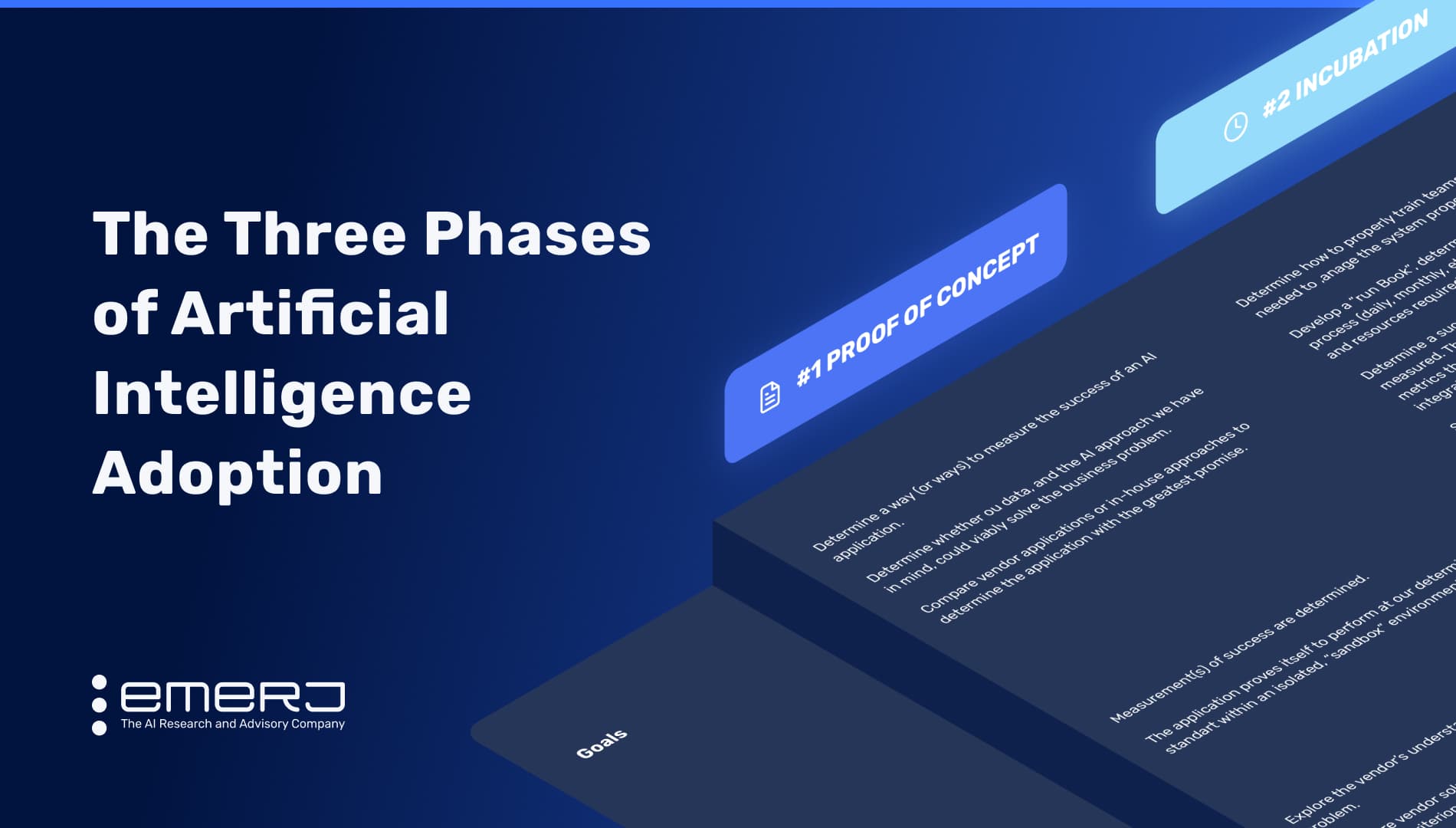
Artificial intelligence projects are more like R&D than they are like traditional IT. It is experimentation as much as it is adoption, and this difference is one of many reasons that AI projects take longer to integrate, and often hit bottlenecks that prevent them from being used in production.
In this article we’ll explore the three phases of AI adoption:
- Proof of Concept Phase – This is the preliminary phase of experimentation, where we determine if value (predictions) might be gleaned from the data we have available. In this phase we’re answering the question: “Can this application deliver results in a sandbox of historical data?”
- Incubation Phase – This is a phase of experimentation closer to the core of the business. This phase often answers the question: “Can this application work with a controlled cohort, and more real-time data?”
- Integration Phase – This is the phase where a project goes from experiment to deployment. In this phase we’re answering the question: “Can this application work at scale in the business?” (Hint: The answer is not always “yes,” AI is an iterative, experimental process).
Notice that in each phase, experimentation is ongoing.
While we’ve covered these phases in a previous article, this guide will focus specifically on the Incubation Phase. This phase is designed to ensure that sandbox experimentation has a chance of actually workin in the business itself. Skill the Incubation Phase can lead to embarrassing and expensive failure in deployment. Getting the Incubation Phase right, however, will provide an AI project team with the necessary confidence and understanding to give the AI initiative the best chance of successful adoption.
This article should help AI project leaders to (a) better prepare for the experimentation and iteration requirements of enterprise AI adoption, and (b) assess any AI project along the three phases of AI adoption, with clear distinctions between each.
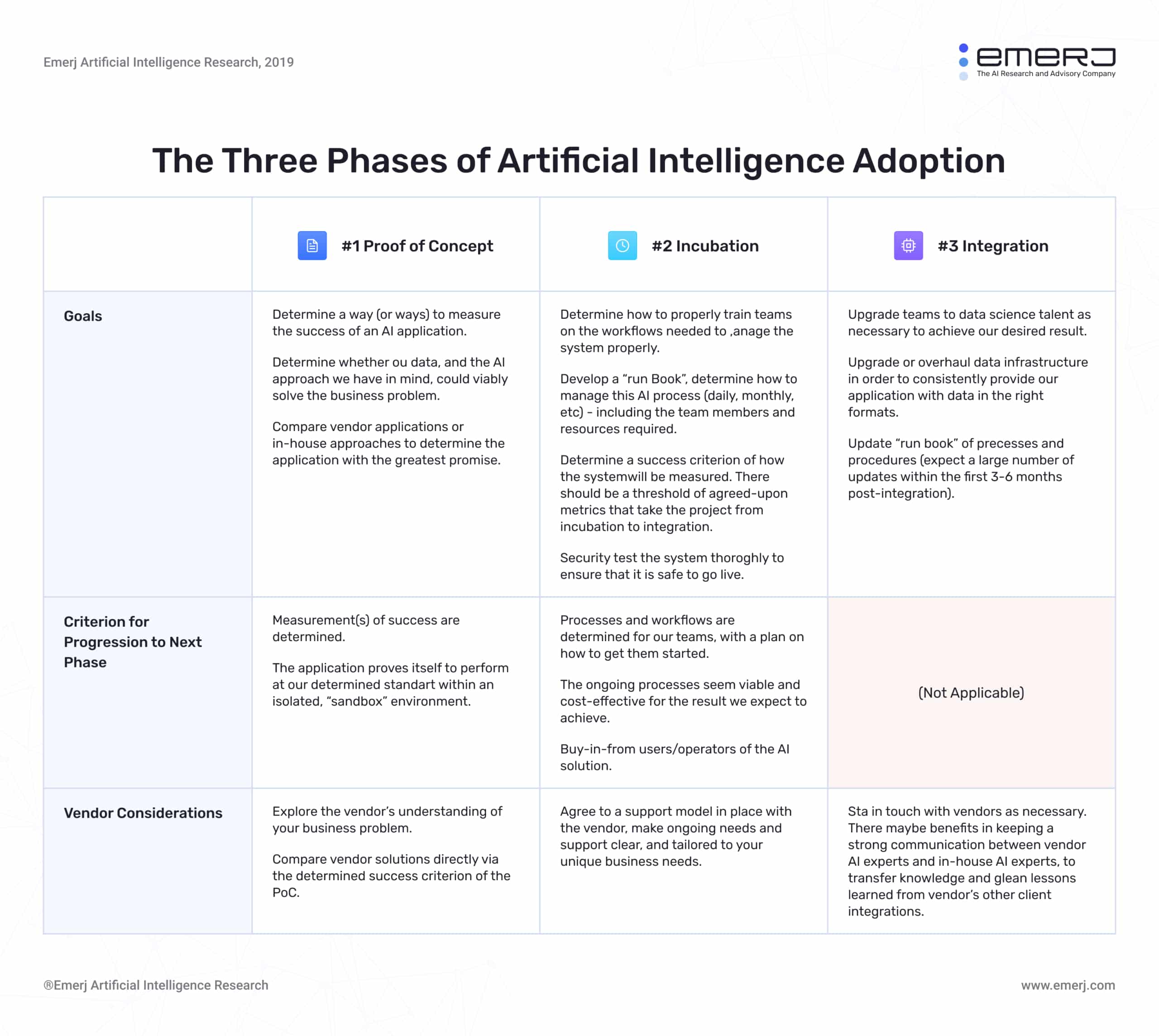
Referencing the phase-by-phase table above, we’ll go through each of the three phases in depth, describing the goal of the phase, and – where relevant – the critical questions to be answered before moving to the next phase. We’ll begin with the Proof of Concept Phase.
Phase 1 – Proof of Concept
A proof of concept is intended to determine whether an AI application could potentially deliver a specific goal or outcome. It is not intended to replicate an actual production environment. It is merely intended to prove that the AI approach is potentially valuable.
This phase is important because, frankly, many AI approaches are purely experimental. Determining whether such approaches, whether for fraud detection, for recommendation engines, or whatever else, could be used on the specific data and specific business problem of a specific business is uncertain.
Often a proof of concept is run in a “sandbox,” a controlled environment, with historical data, without any actual integration into core business systems, and without any actual security concerns.
The vendor, if one is involved, is often doing the project themselves without significant involvement from the in-house data science talent of their client.
There are no end-users to please, no day-to-day operations that can be interrupted or challenged. If the vendor has run many such sandbox tests, they may be able to confidently set up and run this very controlled test. If the vendor is solving a new problem or working with new kinds of messy data, they may be learning as they go. There may be no walk-through of the user interface for the client; the interface and work is often being done by a vendor.
The aim of a PoC is to determine some measurement of performance, for example:
- False-positive, false-negative, true-positive, and true-negative rates
- Time to results (per the processing of one loan application, for example)
- Cost-per-item (for a chatbot, this might be per reply or per satisfied user query)
- Ease of use
By coming up with some kind of standard measure, a company can compare vendors (or a vendor versus in-house approach) side by side. A company can also look at the time it takes to complete the process.
Goals to achieve in the proof of concept phase:
- Determine ways to measure the success of an AI application
- Determine whether your data and the AI approach you have in mind could viably solve a problem
- Compare vendor applications or in-house approaches to determine the application with the greatest promise
Phase 2 – Incubation
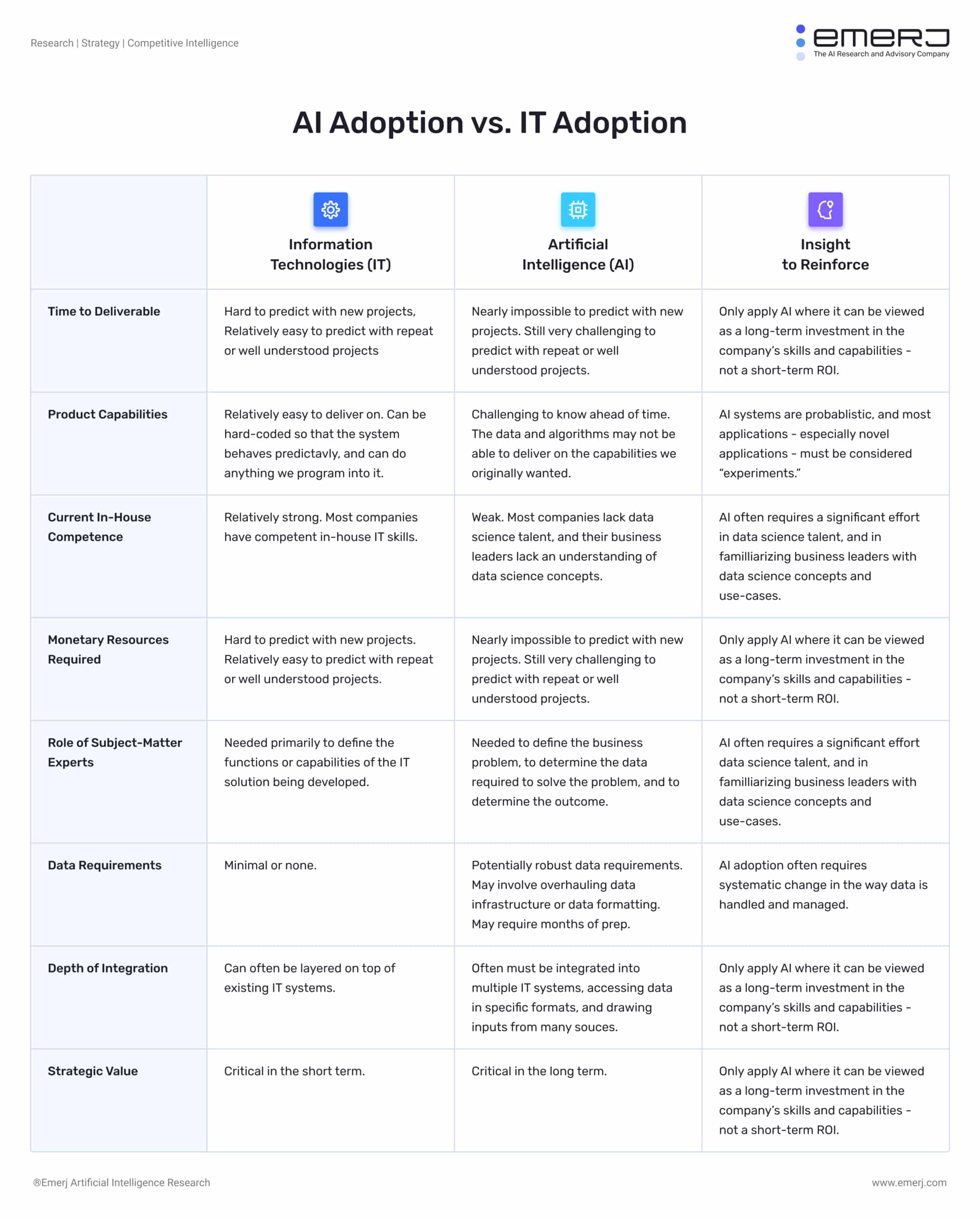
With traditional IT, a proof of concept can often provide enough insight to determine whether or not a solution should be integrated fully into a business. Because of the iterative nature of AI (calibrating data and algorithms to achieve a goal) and because of the novelty of AI workflows and skills, an incubation phase is often required in order to flesh out the challenges and potential of the technology.
Goals to achieve in the incubation phase:
- Determine how to properly train teams on the workflows needed to manage the system properly
- Determine how to manage this AI process (daily, weekly, monthly), including the team members and resources required
- Determine a success criterion of how the system will be measured. There should be a threshold of agreed-upon metrics that take the project from incubation to integration
- Security-test the system thoroughly to ensure that it is safe to go live
- Agree to a support model with the AI vendor if you worked with one
Often the goals of the incubations will be tailored to your unique business and business problem. Relatively mature vendor companies will have proficient strategies for proving a proof of concept, but they are unlikely to have a succinct process for the incubation phase. In many cases, the vendor companies will be learning as much in this phase as the client.
Questions to ask in the incubation phase:
- How will this work into the workflows of your teams? Who will do what to keep the system running regularly?
- How do you collect new data? Who tags it? Where do you put it?
- What do you do if the model seems to be wrong?
This incubation period will fade as vendors and use-cases become more capable and as teams become more used to data science and ML systems, but for the foreseeable future, incubation is necessary for the integration of most robust AI systems.
Phase 3 – Integration (Deployment)
Integrating artificial intelligence into an existing business environment is challenging but can be improved by having the right set of expectations, as we’ve tried to cover throughout this report, and by going in with the right set of questions.
Questions to ask when moving an AI application from incubation to integration:
- Do you have a team leader or champion who understands the basics of artificial intelligence and is willing to take risks and iterate?
- What team members will need to be involved in keeping this AI systems working?
- What additional in-house talent or consulting help do you need in order to implement and run this system?
- How do you need to prepare these team members to work together and change their current workflows?
- What are the additional security considerations you should take into account before going live?