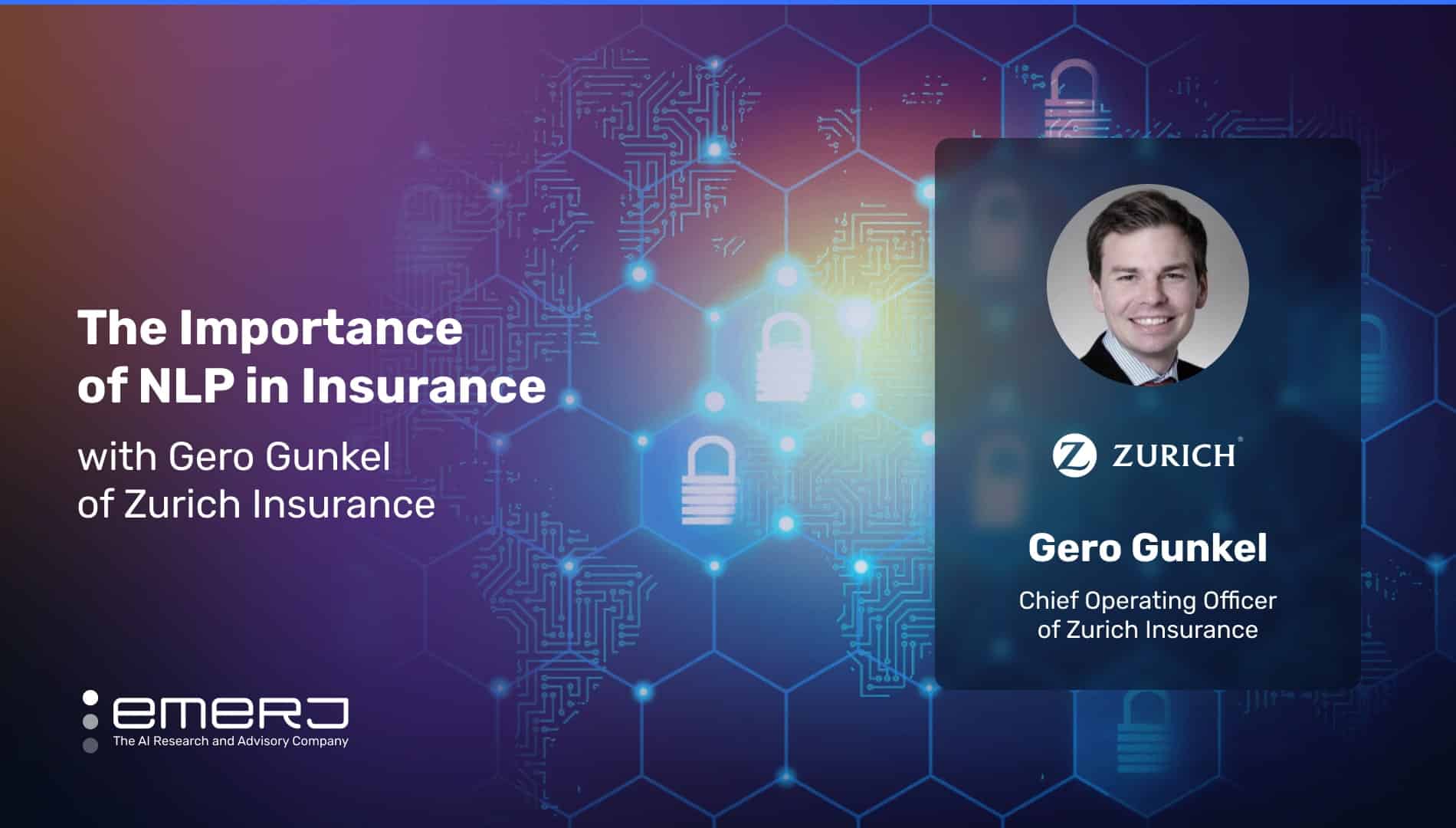
Although not often regarded as a technological first-mover, the insurance industry has recently seen robust, even rapid, adoption and deployment of AI capabilities, particularly in those related to natural language processing (NLP).
From automating customer service inquiries to detecting fraud and analyzing customer sentiment, the ever-expanding range of use cases in insurance is already helping make those insurance companies actively adopting AI smarter, faster and more customer-friendly than ever before.
NLP can also streamline the underwriting process by extracting relevant information from documents, enabling underwriters to assess critical factors such as risk faster and with greater precision.
For those insurance companies prioritizing AI adoption, the stakes are staggeringly sky-high, and won’t be trending downward any time in the future – foreseeable or otherwise:
A 2022 analysis by Allied Market Research projects an AI-powered proliferation of just the insurance market to be upwards of $46 billion by 2031.
Pointing to the industry’s appetite for disruption and transformation overall, many next-gen insurance companies are even self-designating as “AI-first,” and comprise a new subset of the industry, insuretech.
Leveraging NLP, insurance companies are already realizing significant value from their AI investments, according to Gero Gunkel, Chief Operating Officer and Data Science Leader for Zurich Insurance.
Gunkel recently sat down with Emerj CEO Dan Faggella on Emerj’s ‘AI in Business’ podcast for an up-close analysis of NLP adoption that he’s seeing at Zurich and being mirrored in the most disruptive corners of the industry.
From their brief, 30-minute exchange, this article examines two key takeaways in NLP applications in the insurance sector:
- Moving to new, higher-value services: By automating text-heavy processes and document-handling workflows, human jobs will be less centered around repetitive tasks and more focused on tasks that are customer facing, or that require critical thinking.
- Avoiding tech-giant dependencies: The importance of investing in NLP capabilities in-house and diversifying vendors prevents tech dependencies that create monopolies.
Listen to the full episode below:
Guest: Gero Gunkel, Chief Operating Officer and Data Science Leader for Zurich Insurancy Company, LTD.
Expertise: data science, machine learning, artificial intelligence, business development, team leadership
Brief Recognition: Before serving as COO and Data Science Leader for Zurich Insurance, Gero Gunkel served there as global head of AI. He completed his university degrees at Heidelberg University, the University of Bath, and the London School of Economics.
Moving to New, Higher-Value Services
Until relatively recently, despite the insurance domain’s ever-growing volumes of unstructured data – everything from contracts, to customer conversations via phone, and social media – has historically been too cumbersome and costly for insurance companies to extract any measurable value.
Today, NLP-driven automation is proving a strategic entry point for insuring organizations, and particularly, for use cases that can leverage large amounts of unstructured, text-based, data – something the insurance industry certainly isn’t lacking.
“No one has ever counted, but it’s estimated that 80% of all data is unstructured, and potentially, even more, and that usually means either text data, or images, and insurance is rather light on images, but in terms of text, we have a lot,” Gunkel says.
For NLP’s purposes, insurance contains a motherlode of unstructured data: the industry-characteristic proliferation of forms associated with claims, contracts, applications, etc., as well as transcripts of customer interactions – by phone, text, chat, on social media, etc. – and many, many, other sources.
“The automated analysis and the processing of texts comprise probably the most cases at the moment at Zurich, but also in the large insurance sector,” Gunkel says. “It’s very, very, prominent, and you can actually do a lot with it.”
Use cases that can leverage this type of customer information hold immense value potential for improving the customer experience or to otherwise connect with the customer.
Because insurance companies don’t have much contact with their customers outside of claims and contracts, it’s especially important for both sides to have a clear understanding of what’s covered, and what’s not.
This can also be useful for understanding complicated policies or where there may be conflicting language across many different documents.
For simplifying contract reviews, NLP allows for more rapid identification of relevant sections or areas needing further, or human, attention. NLP can also help to identify common mistakes within contracts such as typos, grammatical and spelling errors, all of which have potentially serious legal implications.
“We can use AI to analyze our contract wordings to identify wordings that could potentially be understood differently by us and our customers,” Gunkel explains, so in this way, “NLP is being leveraged to actually enhance the core product, which is essentially contract warnings and contracts.”
In claims automation, NLP is being used to read customer documents, such as applications, forms and contracts, to quickly analyze this unstructured data to generate accurate, data-driven, responses, allowing insurance companies to better assess and settle claims. For process automation, NLP is being used in insurance for everything from gathering customer information during conversations with call center agents to creating tailor-made policy recommendations based on a variety of customer input. Use cases extend to flagging documents for manual review, creating personalized policy recommendations based on user input, and onwards.
In addition, NLP is also being used to help improve customer service through sentiment analysis, providing personalized responses tailored to customers’ individual needs.
“The use case is really around finding a process where today we get from internal external source documents – this could be PowerPoints, emails, scanned documents, and finding a way to automatically extract information from it and to avoid rekeying activities for human workers,” Gunkel tells Emerj.
These use cases still require a human to review the output, a reason Gunkel cites as to why he disagrees with the notion that recent advances in NLP and other forms of artificial intelligence and machine learning will supplant human intelligence and human learning. Rather, he says, leveraging NLP means text-related jobs will be less oriented around a collection of repetitive tasks and be more focused on human capabilities collectively leveling up to higher-value services like human interactions, customer-facing activities and creative endeavors.
“Ultimately, a job is a bundle of tasks, and today, human workers have some repetitive tasks like recurring information, and then we have some interactive tasks like engaging with the customer,” Gunkel says.
The first job category of jobs that Gunkel outlines, which are made up of bundles of repetitive tasks that follow a certain structure, make for a prime target for “machines to take over.”
When repetitive tasks, like rekeying information, are taken out of human workers’ hands and moved to the server, those same workers gain significantly more time to level up and focus on more complicated requests that go well beyond the monotony of tasks like rekeying information. “The job profiles will change, but I don’t envision a massive plot of unemployment anytime soon, from AI technology,” Gunkel adds.
Instead, transformation leaders should see this as a perfect opportunity to prioritize their own development pipeline, focusing on high-value use cases that maximize ROI, or handle repetitive tasks so that human workers can level up to newer, cooler, jobs.
Avoiding Tech-Giant Dependencies
A far more salient threat, as Gunkel sees it, is the risk of forming dependencies on certain AI vendors, or the possibility of technological monopolies forming around an AI capability.
Generative models in AI make up the newest class of NLP with capabilities that extend well beyond discriminative classification and automation Generative AI, as the name implies, can create original, net-new text, audio, images, video, speech, code, etc., from existing content.
The paradigm-shifting potential for generative NLP models has driven what’s now being called a Cambrian explosion of AI. As such, it’s already vastly changing the way individuals and businesses spanning every vertical, interact with the world. For instance, in insurance, high-volume claims adjusters can use generative NLP to create short summaries of claims, just like a human analyst would do.
The most powerful AI being applied out there today can only be created and produced by a handful of companies, and they’re getting bigger and more complex every year, so the amount of players in the game is actually shrinking, Gunkel explains: “What kind of future dependencies are being established when there are only four or five companies who can actually create such powerful tools from scratch?”
That’s why Gunkel highlights the importance of applying a portfolio diversification strategy when selecting from your company’s mix of AI vendors and open-source AI projects.
While a well-diversified portfolio can go a long way toward preventing these dependencies from forming, ultimately, fostering those capabilities to train and modify AI learning models in-house are key.
Less about being able to create these models from scratch, and more about having the ability to understand them well enough to train and modify the learning models you have in place without having to rely on a third-party vendor to advance it.
“If you have these internal capabilities, and you work with different open-source models, then I think this dependency can be actually quite reasonably managed,” Gunkel says.