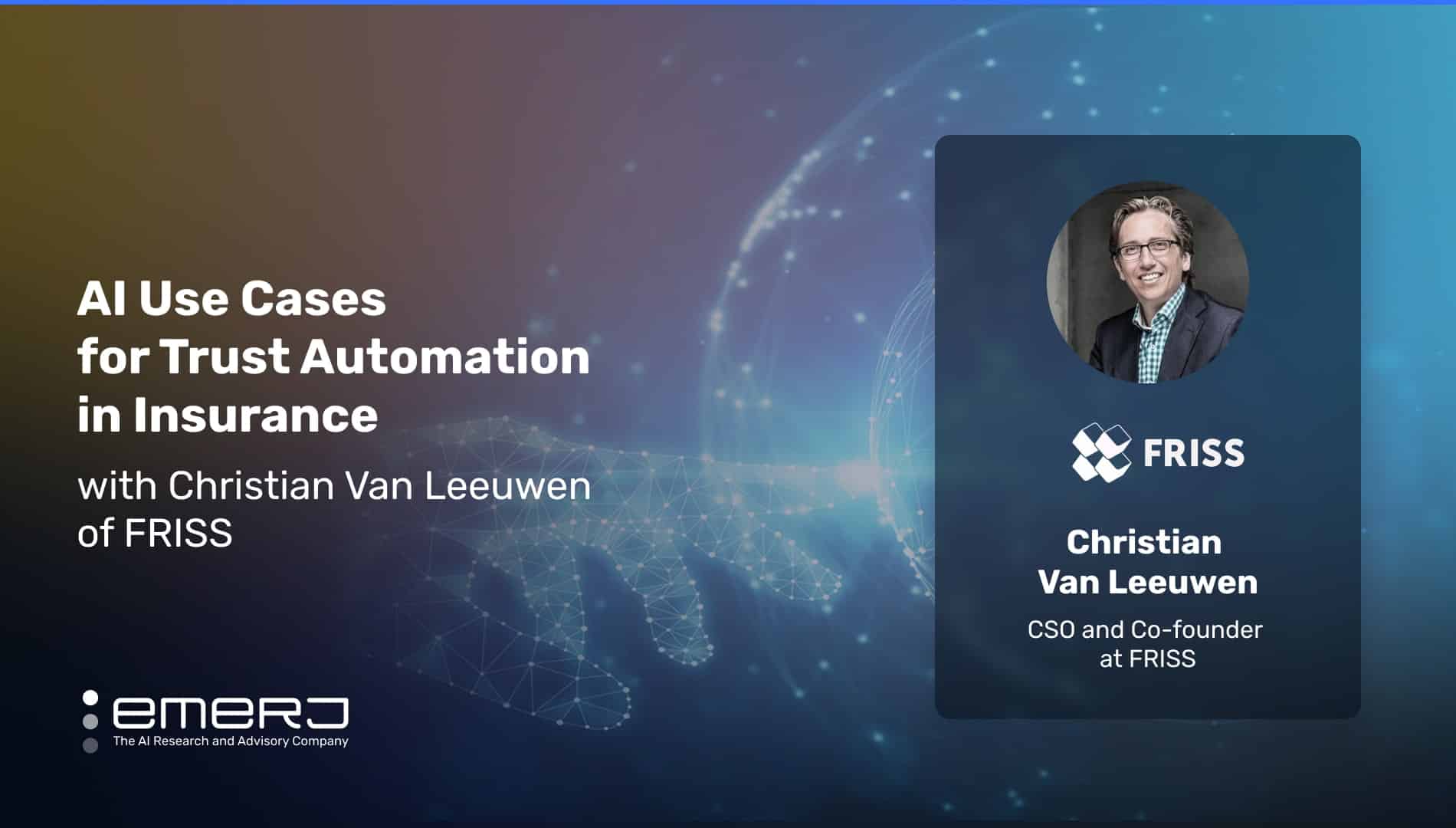
Insurance is a growing arena for AI adoption and in many cases, automation is leading the way to streamline customer experiences and the organizational pipelines behind them along the entire customer journey.
Inevitably, this leads to the importance of ensuring security in insurance workflows – from claims to underwriting and beyond – whereby institutions don’t just know their customers; they have absolute, even autonomous, trust in them.
In our first interview with FRISS Chief Strategy Officer Christan van Leeuwen, he introduced the notion of “trust automation” in insurance to the audience on Emerj’s AI in Business podcast.
The functionality of trust automation relies on a workflow, like many across AI research in compliance and rules-based systems, that embodies a principle of “front-door” verification. In other words: ensuring the security of systems by “checking IDs at the door” in terms of the scrutiny of first-time users of those systems.
This article breaks down a follow-up conversation with Christian focusing on insurance use cases in trust automation, extracting two key insights from his conversation with Emerj CEO Daniel Faggella on the AI in Business podcast:
- Automating claims and underwriting processes: The earlier customers are verified in service funnels like claims and underwriting, the easier it is to streamline workflows in compliance and customer journeys.
- Finding new business opportunities in underwriting and IoT: The data-informed approaches to trust automation in the underwriting process will mean looking at risk and data in new ways to offer customers unique, personalized products and services.
We find beneath his insights the importance of “front-doors” in streamlining compliance and security workflows for those systems and service funnels – something of an organizational approach to secure app development in software engineering – for applying AI capabilities in these insurance functions.
Guest: Christian van Leeuwen, Co-Founder and CSO of FRISS
Expertise: Insurance, underwriting, strategy, risk management
Brief Recognition: Before founding FRISS in 2006, Christian worked across financial services and technology spaces. He began his career in consulting, working for Capemigi and other firms starting in the mid-90s, and as a business analyst for Archmea as FRISS got off the ground.
Use Case #1: Front-Door Verification in Claims Automation
Where we see insurance firms establishing new digital processes that ease customer journeys focuses on the moment customers first apply to become fully subscribed users of insurance products. When these customers eventually have a claim, they will inevitably access the firm’s website, answer a couple of questions, and upload documents as evidence for their claim.
When they press the ‘claim’ button, the money is “in the bank account,” says Christian – describing how quickly insurance firms are quick to trust flimsy online processes that are letting fraudulent claims run amok.
But some version of that seamless user experience is what every insurance professional envisions having for their customers. Why? Because that’s what the customer expects nowadays, says Christian: “It needs to be smooth, efficient, and it needs to be paid instantly.”
Firms are on the right track in thinking that automation is a key to end-to-end seamless customer journeys, but not at the risk of security – and even infusing security features and values in the very framework of that customer journey by embracing the right technologies.
Christian cites a use case where a customer was losing thousands of dollars by prioritizing customer experience over automating trust in their claims process, going as far as shutting down the company’s website for weeks.
As it turns out – the bank was the target of a more widespread, organized criminal effort:
“Basically, it turned out that that group of people learned about what the bank was doing, that the process wasn’t really checked,” says Christian. “And if they share the scheme amongst the group online, they will do the same thing over and over again.”
Automating the claims process came down to documentation processing, says Christian – which involves a range of AI capabilities working in concert to de-silo verification processes across the organization so that authentication data can be appropriately stored and processed.
Much of the process of integrating verification into these workflows tends to dismay client organizations’ leadership and their expectations for how they use verification data. But as Christian tells Emerj, one of the primary AI capabilities in this particular use case is in convincing noncoding business leaders to overcome black box issues in the process of building trust automation:
“Armed with that verification data, we can analyze if these claims documents are real. Have they been tampered with? What’s in the document? AI is also instrumental in connecting these questions to the actual claim and checking the persons behind the claims are not related to any other previous suspicious claims already. That’s what we do in the real-time network analytics.
Bringing that technology together is really in the trust automation platform, looking at data, AI, real-time network analytics, image and document analysis. Those are the things on technology that we use to bring greater trust into organizations.”
– Co-Founder and CSO of FRISS, Christian van Leeuwen
Instrumental in streamlining trust automation is ensuring that customers’ data and identity are verified (and in banking terms, the system ‘knows the customer) with the system from their first interaction – or “front-door” verification:
“Every customer wants to ensure trust from the minute they interact with customers,” says Christian. “And then if that’s in place, it opens up to a faster process and helps the customers and at the same time by preventing unnecessary leakage [of false claims].”
The focus on the top of service funnels for verification inherent in trust automation as an AI-enhanced security practice gives business leaders outside of insurance a framework to think about how best to streamline workflows.
In essence, trust automation in the insurance space serves the same purpose as Know Your Customer (KYC) and general BSA-related compliance in banking: guaranteeing that the customer you’re working with is who they say they are.
Business leaders across financial services looking at ways that AI can streamline compliance workflows in fraud and anti-money laundering detection would be wise to keep in mind the “front-door” focus that trust automation builds into insurance verification processes.
Use Case # 2: Commercial Insurance and Finding New Business Opportunities in Underwriting and IoT
In discussing an underwriting use case, Christian starts by citing a recent study from McKinsey predicting that underwriting as a practice we understand today won’t exist by 2030.
That autonomous future involves, as Christian sees it, entirely data-driven organizations that use every scrap of information passing through their organization to the best risks to underwrite and the best way to help their customer understand the underwriting process themselves.
He sees the benefits of that data centralization first being felt in personalized insurance, where conversational and other AI capability categories have broader applications. As that happens, human underwriters will be tasked with more advanced problems to solve – much like human conversational agents are in conversational AI-based business transformations of customer journeys in the banking industry.
In the process, we again arrive – similar to KYC compliance workflows in the banking and financial services industry writ large – at a repetitive task involving verifying a user at the top, or “front-door,” of a service funnel.
Just as in the prior use case, Christian and the team at FRISS are finding, again, that the ultimate result of fully automating underwriting service funnels with AI capabilities also means organizations implementing a ‘front door’ approach to verification of customer information:
“What is presented to me is the company doing that type of business what I expect it to do from the official registration and from the submission that has been done,” says Christian. He explains that sometimes this can register as different kinds of signaling publicly through social media or other public channels.
Christian emphasizes the following vision as the true essence of automated trust processes: First, AI capabilities can build classification models using this data – along with that collected across a commercial client’s entire measurable digital and physical footprint through IoT technologies.
Then based on probabilities and risk assessments using those models, they can make predictions about whether commercial clients will be worth risk underwriting based on their actual facts and circumstances.
In such a way, as we see conversational AI-based adoption projects in banking, having 360-views of the customers enhanced with AI capabilities – whether in the commercial or personal insurance spaces – means customer journeys in insurance can become:
- Profit-generating activities and pipelines worth developing
- Ways to track data that can personalize customer services or enhance the customization of existing services.
While they have different names between different industries, the very task of knowing a customer in the digital space is, in many ways, the heart of the insurance space, where the entire basis of assessing the risk of a particular customer depends on how much a carrier knows about them. Whereas the impetus to gather data on clients in banking, given the privacy challenges involved, tends to come from regulatory forces outside the organization.
For these reasons, the AI applications and use cases go by different names for different industries (BSA-related compliance in banking, trust automation in insurance). Still, the fundamental applicability to the nature of the task remains the same.