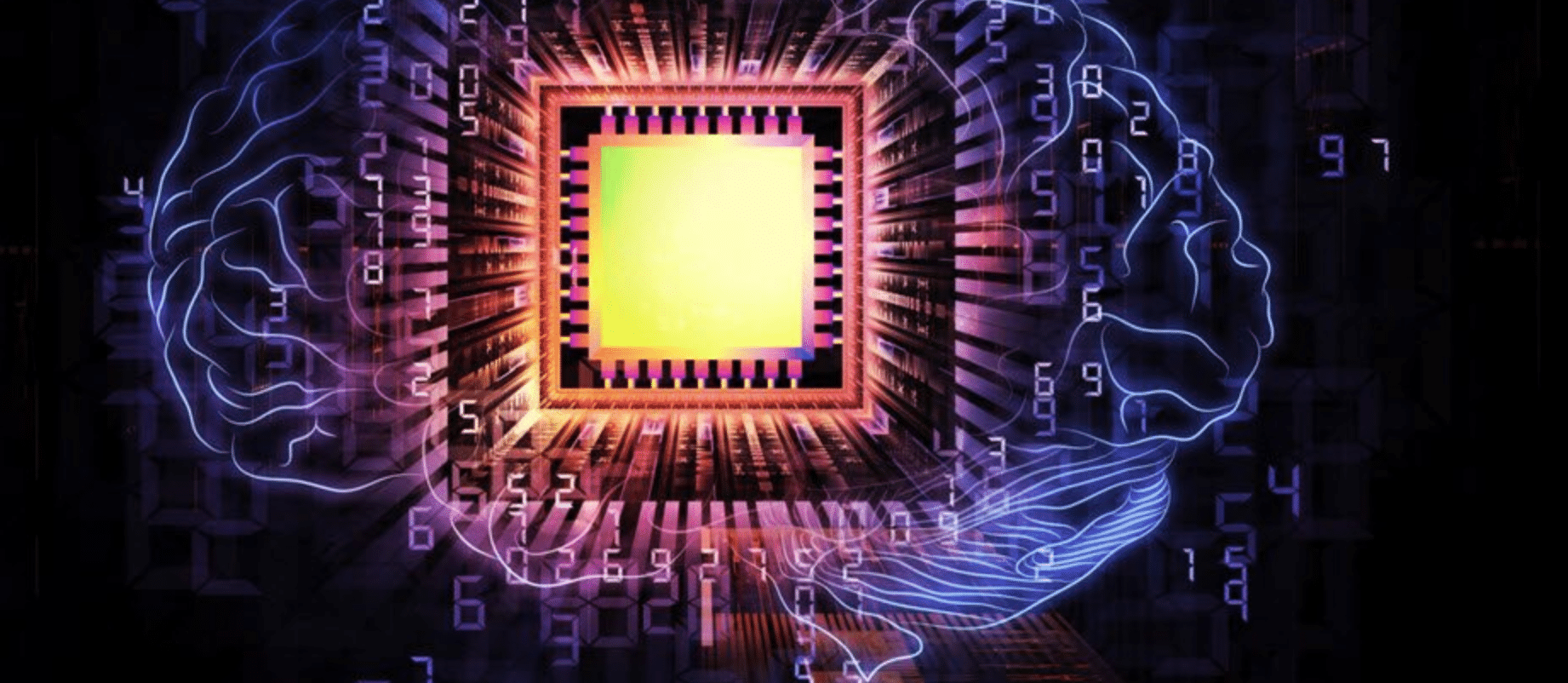
The following article has been written by Josh Sutton, Global Head, Data & Artificial Intelligence at Publicis.Sapient. Publicis is one of the world’s largest. Editing and formatting added by the Emerj team. For information about our thought leadership and publishing arrangements with brands, please visit our partnerships page.
The world is transforming at a faster rate than we have seen before. Richard Foster, a lecturer at the Yale School of Management, has found that the average lifespan of an S&P company dropped from 67 years in the 1920s to just 15 years today.
One of the larger drivers of this accelerated change is the impact the Internet has had on society. Specifically, the Internet has completely changed the way we communicate as well as the ways in which we access information. Not only has this impacted technologically advanced countries, but it has also accelerated the development of third world countries – mobile access to the Internet has allowed countries to accelerate their development in ways that would not have previously been possible. This includes having access to banking, healthcare information, and global communications; all through a mobile phone.
While I do not believe it is possible to overstate the importance of the Internet, I also believe its impact will pale in comparison to that of artificial intelligence. For all of the impact the Internet has had on the world, it has primarily impacted the way we communicate and access information. Artificial intelligence, however, promises to enable people to perform cognitive tasks that were previously not possible.
We are at the very beginning of this period as we begin to see companies apply AI to traditional business problems and not view it as a purely academic pursuit. While it is exciting to see AI solutions win at games like Go, it is even more exciting to see AI solutions improve the way businesses operate. We are beginning to see this across multiple industries. In financial services, EZOps is changing the way that trading organizations manage their middle and back office operations. In healthcare, companies like Spring Health are improving the way that we treat debilitating mental health illnesses such as depression.
AI will become a “cognitive multiplier” – something that enables us to perform more intellectual functions than one person had ever previously been able to achieve. This is similar in many ways to the transformation that happened when steam and electricity enabled people to perform more physical labor than any one person could have accomplished. As AI technologies enable us to leverage this Cognitive Multiplier to perform intellectual tasks, we will see our world change in a multitude of different ways – and we will see it happen over a period of years rather than decades.
I think that there will be at least three major waves of transformation:
- Enterprise-level pattern matching: Extracting insights from data sets with hundreds of different variables at a level well beyond human ability
- Ubiquitous access: Technology that engages with humans on our terms via voice, vision, and conversation – essentially broadening the interfaces where AI becomes part of what we do
- Deductive reasoning: Applying logic to generate new ideas and insights from very limited data sets
Each of these “waves” could be seen as a lens to look into any given industry and imagine AI’s future changes. Below we’ll explore each of them in depth, with representative examples to help readers extrapolate what these dynamics might mean in their own industry in the years ahead.
1 – Enterprise-Level Pattern Matching
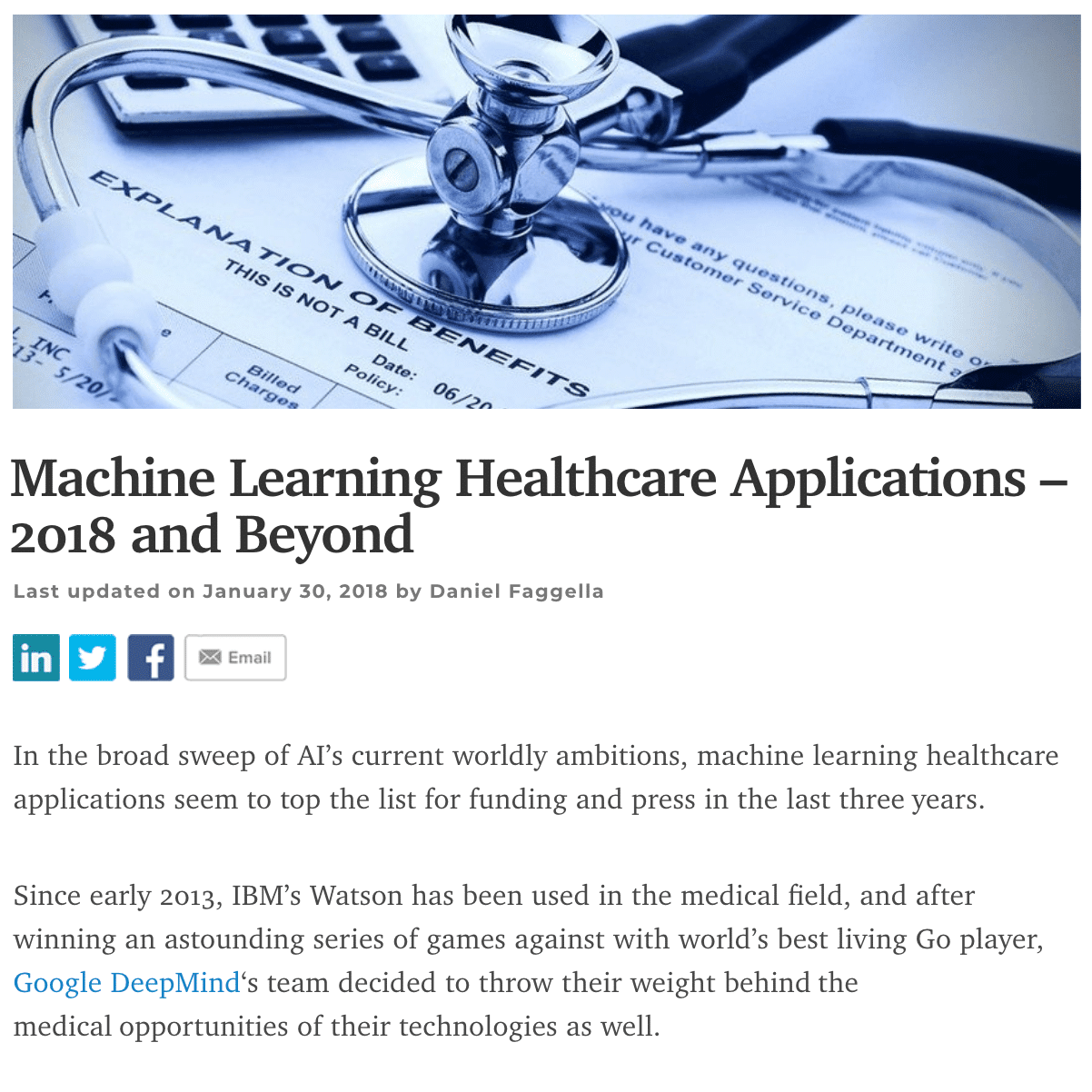
recently updated with new examples.
AI-enabled pattern matching is already starting to impact business around the world today. Humans have the ability to analyze situations and identify likely outcomes based on recognition of similar patterns in the past.
When a person catches a ball that has been thrown to them, they don’t perform calculus in their head to determine exactly where the ball will be so that they will be able to catch it – instead, they rely on their memories to match patterns of previously thrown objects to determine a rough approximation of where the ball will be and how they can get there in time to make the catch.
Machine learning is enabling this “pattern matching” to occur at a larger and faster scale than has ever been possible. Machine learning is a concept that originated as early as the 1950s. Professor Geoffrey Hinton demonstrated that backpropagation could be used to create multi-layer neural nets in 1986, effectively giving birth to deep learning.
Current AI thought leaders such as Andrew Ng and Yann LeCun studied with Hinton and have continued to advance the applications of deep learning at companies such as Google, Baidu, and Facebook. While backpropagation and deep learning are still central to many AI advancements, we are now seeing a wide range of other approaches to developing intelligent systems being explored.
Some of the areas where we are seeing the impact of this first wave include healthcare, finance, and advertising.
- In healthcare, the combined power of man and machine has the potential to outperform traditional human-only diagnosis for various diseases and conditions (particularly with respect to medical imaging).
- In finance, fraud detection is orders of magnitude more effective than it was before the integration of machine learning (Editor’s note: See our interview on the simplified science of ML for fraud detection with Kevin Lee of Sift Science).
- Advertising is undergoing a massive transformation as brands are able to use individual-level data to determine how to best engage a person to generate the desired results rather than relying on a single big idea.
At Publicis, we have seen improvements in advertising efficiency, as measured by actual purchasing, thanks to AI leveraging more data than would have been previously possible. In other words, machines aren’t just pattern matching the same way that humans do – in many cases they doing it better than us.
One more recent example of the application of artificial intelligence in the advertising world is a Publicis project partnered with Dove. Dove wanted to connect with their customers around a social issue that mattered to them and they decided, as part of their “My Beauty, My Say” campaign, to target sexism in the media. They used natural language understanding technology from IBM’s Watson to read and understand thousands of articles per day to identify and call out when journalists focused on a female athlete’s appearance instead of their performance. The results were impressive with over 315 million social media impressions.
2 – Ubiquitous Access
Over 10% of US households have an in-home voice device such as Amazon Alexa and Google Home according to NPD Group’s report: “Digital Voice Assistants: Ownership & Applications.” To put that in perspective, it took smartphones 5 years to reach the 10% penetration mark that in-home voice devices achieved in just 2.5 years. This is just the tip of the iceberg as consumers are becoming increasingly comfortable with the concept of an always-on conversational interface or device being part of their life.
Similarly, the new iPhone X is leveraging facial recognition to unlock the phone. The common theme behind these shifts is that AI is enabling us to interact with technology the same way that we interact with one another – through conversation, vision, and, eventually, other senses. Many companies are eager to capitalize on this trend. Qualcomm, for example, recently announced a focused effort to make on-device AI ubiquitous across their products. Below is a video of Apple’s unveiling of the facial recognition unlocking feature:
We are at the very beginning of this wave, but the implications are profound. Just as mobile phones changed the way the world communicated, ubiquitous and seamless integration of technology will forever change how we live and work. Conversing with our household appliances will become normal. Knowing the answer to questions such as “What are the favorite activities of people that buy this brand?” will become easy to answer as we can now use machine vision platforms to identify a specific brand’s product in imagery and identify what activities people in those images are engaging in.
If a picture is worth a thousand words, the billions of images posted daily on the Internet are truly the greatest source of consumer insight that we are just beginning to tap into. Perhaps most importantly, the concept of having to check something on your computer or phone will quickly become an antiquated notion as you combine this insight generation capability with the ubiquitous AI interfaces discussed previously. Imagine:
- Living in a household that can call 911 for you if it sees you having a heart attack
- Being able to ask a factory building where production bottlenecks are happening and what can be done to mitigate them
- Asking your golf bag what club you should use to get on the green
The possibilities are endless and range from life-changing to incremental improvements in day-to-day life. Time will tell which of these applications or ideas will garner mainstream adoption, but there’s so many of them in development (and so many with traction like Siri or Alexa) that it seems evident that AI will make it’s way into our regular interactions.
3 – Deductive Reasoning
Just as humans do more than pattern match, AI is becoming capable of performing more complex tasks. This is a relatively unexplored part of the AI landscape – at least in recent years. That seems to be changing, however. As people have become more and more excited about what is possible via deep learning, they have also become aware of its limitations (Hinton himself has openly addressed some of the flaws of neural networks).
Cris Ross, Mayo Clinic’s CIO, recently compared AI system’s ability to learn to that of a two-year-old child. One of the drivers of this is because humans don’t rely on a single means of ingesting and analyzing data but a combination of different approaches. The implication of this relatively simple statement is having a profound impact on the AI world. Specifically, the concept of “Transfer Learning” is becoming one of the most exciting areas of new research.
One example of research in this area is happening at Google DeepMind, where the team is developing “PathNet” as an approach to enable transfer learning. Simply put (and I apologize in advance to the data and AI scientists that are reading this), transfer learning enables AI systems to combine the incredibly powerful pattern matching capabilities of deep learning with a more structured understanding of how the world works, often in the form of ontologies and semantic AI.
For example, if a person knows how to ride a bicycle as well as drive a car, it’s not a far-fetched scenario to envision that the same person could learn how to ride a motorcycle very quickly – they understand how to ride a two wheeled vehicle as well as how to operate a motor-powered vehicle. While there will still be some learning curve, it will be nothing compared to somebody that has no relevant knowledge to transfer over to the new activity.
As humans, we not only leverage this combination of different types of cognition to learn new things, but we also apply these different types of cognition to justify our answers. When you ask an expert in a given field a question, they will often have an immediate answer for you because they have seen similar things in the past.
When you ask them why that answer is correct, they leverage the years of training that they have built up to provide you the logical rationale that supports the answer that they originally provided to you. This process is one that AI platforms are beginning to mirror to explain answers that are provided by deep learning solutions – a potential solution to the frustrating “black box problem” of machine learning.
This ability to think logically and apply things that we have learned in solving one problem to address another has led to some of the greatest scientific advances in history, such as George Westinghouse’s realization that he could leverage Nikola Tesla research on alternating currents to transmit power over long distances. At the AI Summit conference in San Francisco that I attended this past fall, there were a number of standing-room only presentations on the topic of integrating semantic AI with deep learning.
The Financial Industry Business Ontology (FIBO) team explained how they are using this combination of technologies to drive increases in productivity across a wide range of activities such as trade processing. A year ago this would have been heresy, as people hadn’t yet begun to realize the limitations of deep learning as a stand-alone approach to cognition.
This is clearly changing. Deep learning has already proven beyond a doubt that machines can outperform humans when it comes to many pattern matching problems – both in the scale at which they can ingest and analyze data as well as the speed at which they can deduce answers (image recognition is one of many domains where this is the case).
Albeit a bit technical, the Google Developers YouTube channel features a 7-minute primer video on the fundamentals of image recognition, which readers unfamiliar with the technology might find useful:
When you combine this capability with platforms that can process information about how the world works in a way that resembles what we would call logical deduction, you are left with a platform that can process information at a relatively unlimited pace and deduce new approaches that can be applied to new areas of research, yielding an acceleration in idea generation unlike any that we have ever witnessed.
The real world impact of this is yet to be seen, but it promises to be monumental. Some early examples of the combination of deep learning and semantic AI have led to breakthroughs in genetic research as well as the best performing natural language understanding solutions in the world (which seem to have improved significantly in just the last year).
In the future, it is difficult to imagine an industry that won’t be transformed by a platform that can both identify patterns faster than a human as well as deduce what the implications of those observations are for a specific business. I expect to see examples of this that range from the simple, such as identifying that a marketing message isn’t driving the desired behavior and proactively identifying what should be said instead and adjusting the campaign automatically, to the very complicated such as setting direction for product development and R&D investments as companies seek to build products that will resonate with consumers in the coming years.
Moving Forward
When it comes to AI, Frank Sinatra put it best when he sang that “The best is yet to come.”
In every major transformation that we have gone though in our history, the impact has been felt over progressively shorter time periods. The World Economic Forum describes the period we are entering as the Fourth Industrial Revolution. Each of the previous Industrial Revolutions, driven by steam, electricity, and digital technologies, have changed our world over a shorter and shorter time period.
The same appears to be holding true for AI and this Fourth Industrial Revolution. Over a few short years, AI has already begun to transform most businesses on the planet via dramatically outperforming humans at pattern matching. As we move into the second and third waves of transformation it is reasonable to expect that we will see progressively more impactful changes to the way that businesses and society work.
This will create substantial impacts on society – some of which could be negative in the short term (including major concerns about job loss, among other serious near-term risks). There is also the challenge of how humankind will adapt to a world in which some of the most original and insightful ideas originate from a machine – a struggle for meaning that may be difficult to adjust to.
Despite all of the challenges that transformation at this scale and speed will create, I believe that humankind will be better off as a result of AI. Just as every prior step-change in how we work (including steam, electricity, and digital technologies) has improved our lives, I firmly believe that we will look back at the world before AI and wonder how we lived without the benefit of the amazing technology that we are just beginning to reap the benefits of today.
(Editor’s note: Readers of this article may be interested in our full podcast interview with Josh Sutton on the applications of AI in advertising.)
Header image credit: Big Think