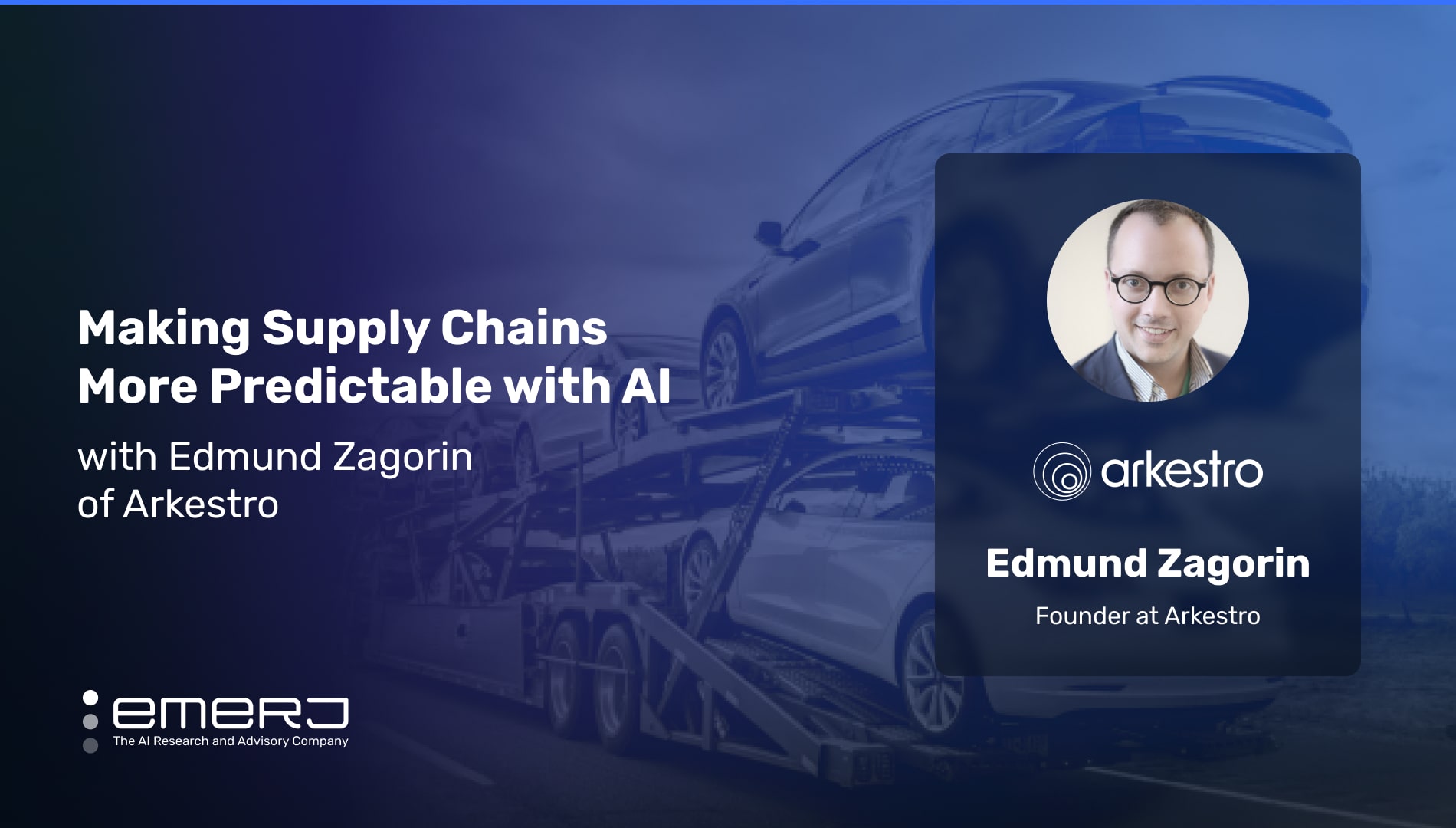
This article is sponsored by Arkestro, and was written, edited and published in alignment with our transparent Emerj sponsored content guidelines.
From the outside, the problem with automotive purchasing seems simple and ubiquitous enough: supply chains are – after inflation and the Ukraine invasion – every economist’s favorite scapegoat in the post-pandemic global economy. Yet underneath the headlines about supply chains – or more recently, a shortage in superconductors – is a central economic dynamic that might be more obvious to those in heavy industry purchasing and procurement.
Vale and General Motors’ recent long-term contract to seal their trade of battery-grade nickel sulfate shows how far major automotive players will ensure the supply chain integrity of the materials their products need.
Arkestro Chief Strategy Officer and Founder Edmund Zagorin recently put a finer point on what he’s witnessed in automotive purchasing and procurement spaces during his appearance on a recent episode of Emerj’s AI in Business podcast.
He tells the audience of enterprise AI leaders that we are experiencing the residual effects of suppliers realizing their purchasing power. Zagorin cites Tesla’s approach to the superconductor shortage — one of many examples of how firms can ‘weaponize’ supply chains.
In this analysis of his conversation with Emerj CEO Daniel Faggella, we will explore two use cases Edmund discusses on the podcast that showcase how AI adds value to the purchasing process:
- Streamlining purchase orders: Decreasing the oversight and time spent in workflows to manually review and verify mundane details in purchase orders and verify their accuracy.
- Predicting trustworthy suppliers: Empowering embedded platforms to predict which suppliers and prices are the best for specific purchase orders while circumventing time-consuming approval processes.
We will explain how each comes as a result of the decreased reliability of suppliers by purchasers in post-pandemic supply chains, beginning with the promise of machine learning in streamlining the purchase orders process.
Streamlining Purchase Orders
Among the most time-consuming processes in automotive purchasing is “cutting POs,” or when purchasing professionals finalize purchase orders and have their built-in prices go through the proper approval processes by budget holders.
What makes the process of cutting POs so time-consuming is that it is:
- Fundamentally a multi-step validation cycle that involves multiple lines of business.
- Ultimately siloed to the single purchasing professional who has to make a decision, usually with never enough information.
That verification process can involve cross-referencing potential suppliers with master databases of preferred supplier lists and price changes over the previous year with that supplier over the last year. All of which require a massive data collection effort by the entire organization.
To achieve fluidity in ordering processes with built-in verification, platforms must be embedded throughout the organization’s existing data stack, and malleable enough to pull all relevant purchasing information from across legacy systems.
Only with enough data from across the organization can companies de-silo the procurement verification process to where human intervention is most needed: the verification of a purchase order completely filled-in through automated processes, according to Melissa Drew – Associate Partner at IBM and a data veteran at the crossroads of automotive procurement and emerging AI capabilities.
“I don’t need my procurement person to go in and manually create [a purchase order] and then route it to the business stakeholder. The system should automatically create that,” Melissa says of the ideal ways to automate the purchase order process with cross-organization data collection.
Such a solution should have all data entries typed in the price of the contract being pulled into the purchase order in a way that’s automatically routed to the business stakeholder. Melissa says the process should be easy as “where they just look at it validated, click approve, and you’re done.”
Then, not only does the model have enough context to self-verify prices and suppliers instantaneously, but leveraging insightful data from a wide range of organizational sources provides additional value to end-user decisions, no matter how small.
“That’s where we see one of our customers – a large German automaker that has recognized tens of millions of dollars in savings in under six months, as a consequence of implementing the solution into their requisition-to-purchase order, and then payment workstream, inside of SAP. In many cases, suppliers aren’t even aware that they’re using this technology. They’re just experiencing the ability to get a faster purchase order, simpler, cleaner process, and we call it predictive procurement orchestration.”
– Chief Strategy Officer and Founder of Arkestro, Edmund Zagorin
The RPA and machine learning-based solutions in predictive procurement orchestration show that these technologies can streamline purchasing related workflows from repetitive tasks. It also shows the larger solution needs to collect enough data to incorporate real-time monitoring of associated processes to simulate the creation of purchase orders based on routine purchases.
Once enough data is being collected across the organization to determine which purchases are so routine that they can be predicted, machine learning-enhanced platforms can fill in many of the blanks in an early PO process – allowing the process to take minutes rather than days to verify.
Predicting Reliable Suppliers
The ‘weaponization’ of supply chains is a symptom of industry-wide reactive mentalities regarding automotive purchasing, says Melissa Drew. “Over time, procurement supply chains have constantly been reactive,” she tells Emerj. “And reactive means: What do I need to do right now to solve the immediate problem?”
According to Zagorin and Drew, all the decisions made over decades based on reducing costs caused ensuing “supply chain interruptions” in the aftermath of the pandemic – the amount of which only revealed how little purchasers know and can rely on their suppliers in a crisis like COVID.
The fundamentals of the problem consist of the following:
- Since suppliers know they don’t have to reward smaller purchasers with discounts, a chasm permeates between these suppliers whose price has effectively skyrocketed.
- These suppliers’ regular purchasers, now left out in the cold, are left with products that cost a small fortune to produce now sitting in warehouses and storage with just a handful of parts missing – and, therefore, unable to be sold or used.
One form that ‘chasm’ can take that Zagorin discusses on the podcasts is the parking lots in southern Michigan state full of cars and buses that are “building shy” – as in, missing only a handful of essential parts.
“By [purchasers] demonstrating that you have some of the power to dictate the price in that situation, if you can’t come to an agreement on that, then you will see consequences, both sides,” says Zagorin of the ensuing stalemates in the global economic headlines.
On top of these challenges, most supply chains are optimized for cost. In other words, if a supplier goes out of business or has delays, it can mean:
- Massive disruptions in operations.
- These enterprises are not used to diversifying their supply options, just as one would an investment portfolio.
Given that, until recently, data in these areas is so difficult to both procure and leverage to the point of putting it in the hands of pricing professionals – the problem at the heart of supply chain disruptions is that:
- Suppliers and their reliability have always been taken for granted.
- Now that the scale of risk for buyers has been set in stark relief thanks to the global pandemic, automotive leaders are looking to increase their options.
As Zagorin further explains on the AI in Business podcast, COVID supply chain problems are forcing automotive and heavy industry purchasers to reinforce the reliability of their suppliers with actionable intelligence.
Only a global crisis like COVID could have put into perspective the scope of data necessary to truly build systems – AI-enhanced and otherwise – that can truly understand this data, says Melissa Drew.
“If it had been isolated in one geographical area or another, we wouldn’t have been able to get as far as we are now,” says Drew in regards to AI capabilities. Where these technologies enter the process is in global, real-time information to solve “problems we’ve never had before,” says Drew. That includes the many previously unprecedented supply chain disruptions that ensued at the onset of COVID.
Among those AI capabilities, Zagorin tells Emerj that machine learning is instrumental in reinforcing that intelligence with predictive capabilities that find the next best options if preferred suppliers are unavailable. He emphasizes that wherever purchasers can give suppliers intent scores based on previous experience and general reputation in the sector based on public information, there is enough data to train a model.
“When you talk about the role of machine learning and AI,” says Zagorin. “It’s really about creating the benefits of optionality for your purchasing organization without the administrative process and overhead costs associated with managing that complexity.”
Additionally, and just as in the previous use case, embedded platforms armed with models trained on information from across the organization’s tech stack will be able to reduce the time spent on oversight and verification processes drastically.
To implement such a solution, stakeholders need to ask themselves a few questions, says Zagorin:
“I really look to business owners and ask, ‘Of the parts and materials that you buy, what’s your dual sourcing coverage? In other words, where do you have another option in your supplier base that gives you some ability to load balance? In other words, to balance your demand across multiple suppliers and have that diversification. It’s true of investing too, right?”
– Chief Strategy Officer and Founder of Arkestro, Edmund Zagorin
Zagorin underscores that asking these questions is about ensuring supplier prediction models to overcome significant black box problems in automotive procurement based on the sector’s reliance on qualified category managers – a scarcity in the current talent shortage.
The transparency barrier means the solution’s capabilities must build trust with subject matter experts by design — category managers need to see how and why a supplier is predicted to be more reliable than another.
Among the range of AI capabilities, Zagorin also notes that machine learning-enhanced solutions convince category managers by cutting “through a vast, complex network, get the right price with the right supplier, not going through a whole long approval process. And do it at that moment when the order is placed.”