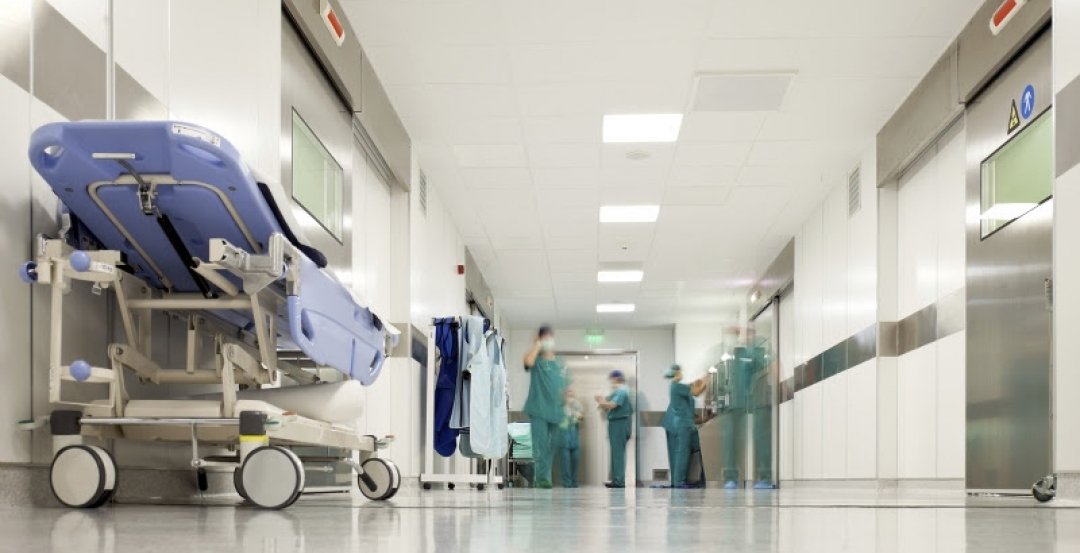
The healthcare analytics market continues to gain traction as the healthcare industry transitions to a value-based care model. Value-based care focuses on quality of care delivery as opposed to volume of patients served. There are great opportunities for data analytics due to the high volume of information that must be routinely collected, tracked and interpreted, particularly in the hospital setting. Examples of value-based care programs in the hospital setting include the Hospital Value-Based Purchasing (HVBP) Program and the Hospital Readmission Reduction (HRR) Program.
According to market research firm Grand View Research, Inc., the global healthcare analytics market is projected to reach $19.5 billion by 2025.
Today, machine learning is emerging as a strategy to help healthcare institutions more efficiently manage healthcare delivery, operations and patient outcomes.
To gauge the role of machine learning in healthcare operations, we researched this sector in depth to help answer questions business leaders are asking today, including:
- What types of AI applications are currently in use for managing healthcare operations?
- How much has the market adopted these AI applications?
- Are there any common trends among these innovation efforts – and how could these trends possibly affect the future of healthcare operations?
In this article we break down applications of artificial intelligence in the domain of healthcare operations to provide business leaders with an understanding of current and emerging trends that may impact their sector.
(For readers with a broader interest in healthcare applications outside of operations software, see our full article about machine learning in healthcare, one of our most popular articles from 2017.)
We’ll begin with a synopsis of the sectors we covered:
Healthcare Operations Software AI Applications Overview
Based on our assessment of the applications in this sector, the majority of healthcare operation use-cases appear to fall into three major categories:
- Hospital management: Companies are training machine learning algorithms to help emergency departments reduce costs and improve quality of patient care delivery (such as reduced hospital readmission rates and patient satisfaction).
- Clinical care management: Companies are using machine learning to help hospitals standardize protocols and implement best practices to improve performance outcomes (such as managing emergency department triage process).
- Risk management: Companies are using machine learning to help hospitals mitigate risk through predictive analytics and evidence-based recommendations (such as reducing the risk of patient and/or staff injuries and its financial implications).
In the full article below, we’ll explore the AI applications of each application by section and provide representative examples.
Hospital Management
Qventus
Founded in 2012, California-based company Qventus claims its healthcare operations platform uses machine learning to help hospitals reduce costs and improve the quality of how they service patients.
Qventus uses algorithms trained on millions of healthcare data points (such as administrative data and Electronic Medical Records or EMRs) to identify patterns, predict operational issues before they occur and recommend an appropriate course of action.
For example, based on patient admission and discharge patterns, the Qventus platform could predict when an emergency department would reach capacity. To keep operations running smoothly, the Qventus’ platform would recommend that a triage nurse receive a notification to open up additional rooms a certain number of hours in advance to avoid reaching capacity.
The 2:27 minute video below demonstrates the example above and provides a general overview of how the platform helps meet challenges of patient flow in the emergency department:
Among its case studies, the company reports that it worked with Mercy Hospital Fort Smith (a 336-bed acute care hospital in Fort Smith, AR) to achieve a 20 percent reduction in patient wait time and a 30 percent reduction in the rate of patients who leave the Emergency Department (ED) without being seen by a physician. Qventus projects that these improvements contributed to a projected $1.3 million in additional revenue and cost reductions. However, it is important to note that we did not find a direct statement from the hospital confirming these figures.
Reducing patient wait time is a leading challenge for emergency departments and hospital leadership. A research study evaluating 995,379 ED visits across 187 hospitals found that periods of high ED crowding may have collectively contributed to 300 inpatient deaths and $17 million in additional costs to hospitals.
On its website, Qventus claims that its platform also targets other components of the hospital framework including labs, in-patient care, and surgery.
MedyMatch
Founded in 2013, with offices in Israel and the US, MedyMatch reportedly leverages AI-driven classification of medical images to improve clinical outcomes in emergency departments and reduce healthcare costs.
MedyMatch’s claims its platform is built using deep learning algorithms. These algorithms compare billions of data points in medical images to recognize patterns and support physicians in making accurate patient diagnoses. These findings would be compared with EMR data for a holistic patient evaluation.
For example, subtle bleeds in the brain (often caused by brain trauma or stroke) are often difficult for physicians to detect looking at patient brain scans. The cloud-based analytics solution aims to serve as a “second pair of eyes” during the patient evaluation process in the high pressure ED environment.
A 2014 research study published in BMJ Quality and Safety, a leading medical journal, estimates that 12 million Americans will be impacted by outpatient diagnostic errors each year. Medical misdiagnosis have been estimated as having a $750 billion annual impact on the US economy.
In the 3:14 minute video below, MedyMatch CEO Gene Saragnese discusses the platform and its potential impact in the ED environment:
MedyMatch appears to still be in the early stages of documenting results as no case studies are currently published on the company’s website. However, there is evidence of collaborations with industry leaders including GE Healthcare, IBM Watson, and Samsung Neurologica.
Readers interested in learning more about AI applications in the field of medical imaging are encouraged to explore Deep Learning Applications in Medical Imaging.
Clinical Care Management
Ayasdi
Primary care is transitioning from a fee-for-service to a value-based medicine approach. While the previous model incentivizes the volume of patients receiving care, value-based care incentivizes the quality of care delivery and patient outcomes. Ayasdi is among AI companies attempting to help healthcare providers and institutions deliver value through data analytics.
Ayasdi’s Clinical Variation Management software reportedly uses machine learning to analyze EMR and hospital financial data to improve patient outcomes. Clinical Variation refers to the diversity of services and practices occurring in the healthcare setting. Essentially the goal of effective management is to move from variation to standardization to improve clinical protocols and patient outcomes.
The company suggests that a hospital’s repository of EMR and financial data represents millions of individual events and thousands of patient procedures. Ayasdi’s claims that its algorithms are trained on these data and through pattern recognition can predict clinical workflows that will potentially achieve the lowest costs and best patient outcomes.
For example, in 2014 Ayasdi partnered with Mercy Healthcare Services, part of the larger Mercy healthcare system, to help inform and improve its clinical protocols. In October 2017, the client announced that it had reduced mortality rates by 30 percent or more for patients treated on a pathway, and have reduced patient costs by $800 per case. These improvements have resulted in an estimated $14 million in savings in fiscal year 2016.
In the 3:47 minute demo below, the company demonstrates how the Ayasdi platform to help manage care variation for a surgical procedure:
Qualaris Healthcare Solutions
The health IT company offers a cloud-based software platform QualarisAudit™ which reportedly uses machine learning to help hospitals analyze their data and improve their implementation of best practices. Machine learning algorithms are trained on data for specific protocols allowing the platform to measure compliance. Qualaris claims that it has a library of over 100 solutions or applications that help users track and improve their compliance with a given protocol.
For example, in one case study an unnamed 350-bed hospital aimed to measure its compliance with best practices for preventing falls among patients. Staff collected compliance data on 14 best practices such as accessible call buttons and patient use of slip-proof footwear.
Qualaris claims that six months of using its platform resulted in a 90 percent reduction in falls and the hospital maintained a 56 percent reduction over the fiscal year. The company claims that a single patient falling in a hospital can cost as much as $33,000 on average.
In the 2:32 minute below, Qualaris’ co-founders provide a walkthrough of their platform with examples of challenges and solutions in the hospital setting:
Risk Management
Lumiata
While effective best practices and clinical protocols exist, there is always some degree of risk in the healthcare environment. Examples include injuries to patients or staff or errors in patient case management.
Founded in 2013, California-based startup Lumiata uses AI-driven analytics to identify & manage risk in healthcare settings. Lumiata claims its risk management platform, the Lumiata Matrix Suite, uses deep learning algorithms drawing on data from 60 million patient records.
The platform identifies patterns within the data and predicts the likelihood a patient will develop a chronic conditions and associated healthcare costs. The platform then recommends a unique plan of action or “chase list” based on those insights.
The platform also integrates data from PubMed, the leading biomedical literature search engine, to provide users with evidence-based rationale behind recommended courses of action for treating patients.
In the 2 minute video below, Lumiata CEO Ash Damle explains the rationale behind his company’s platform and how it may simplify a physician’s ability to make decisions regarding patient care
Lumiata claims its platform helps service over 1 million patients and has provided a 50 percent increase in revenue for its clients. This percentage translates to roughly $600 in revenue per patient. However, a search for individual case studies yielded no results on the company’s website.
CloudMedX
Sharing some similarities with competitor Lumiata, CloudMedX is a health IT company which reportedly uses machine learning and natural language processing to generate clinical insights from patient data in real-time.
The CloudMedx Clinical Analyzer is the company’s platform for optimizing patient care delivery. The platform reportedly uses algorithms trained on a high volume of clinical data to identify potential risk factors for medical conditions in patients and identify a course of action. The company claims that its software can seamlessly integrate into an EMR allowing for a holistic view of the patient in real-time.
The company offers the following example to explain how the Clinical Analyzer helps identify risk of disease in a patient:
When a provider types a diagnosis such as “hypertension” or “HTN”, the [platform] detects the data and immediately puts it to use by:
a. Recording the ICD-10 code for it [a standardized medical code to categorize diseases]
b. Generating the risk using evidence based algorithms that use hypertension as a component
c. Returning a probability for the patient having other associated diseases (such as obesity and diabetes)
Case studies did not appear to be available on the company website.
Future Trends for Healthcare Operations and ML
Successfully managing healthcare operations is complex and involves the implementation of standardized protocols, risk mitigation and adaptability for innovative advancements in the medicine. AI is participating in the growing healthcare analytics sector by helping to improve the quality of healthcare operations and reducing costs.
Hospital management platforms appear to be delivering value and ROI for the hospitals investing in this technology. AI applications that can deliver results within the demanding and fast-paced emergency department environment are more likely to be seen as valued assets for healthcare providers over the long haul.
Applications focused on improving clinical care are showing promise but long term patient studies will be needed to evaluate how these machine learning platforms will impact rates of chronic diseases and high risk patient populations.
Healthcare providers will generally be more likely to consider evidence-based applications as in the case of Lumiata’s platform. Emerging competitors will need to consider a similar approach to attract potential clients in this market.
Risk management is a broad category and we can anticipate more AI applications to emerge in in this sector in the near term. The limited amount of data on outcomes suggests that applications in this space are still in the early stages. Evidence of sustainable improvement in performance metrics will help to establish the validity of risk management platforms.For a broader look at the applications of AI in Healthcare, readers are encouraged to reference Machine Learning Healthcare Applications – 2017 and Beyond.
Header image credit: BDC Network