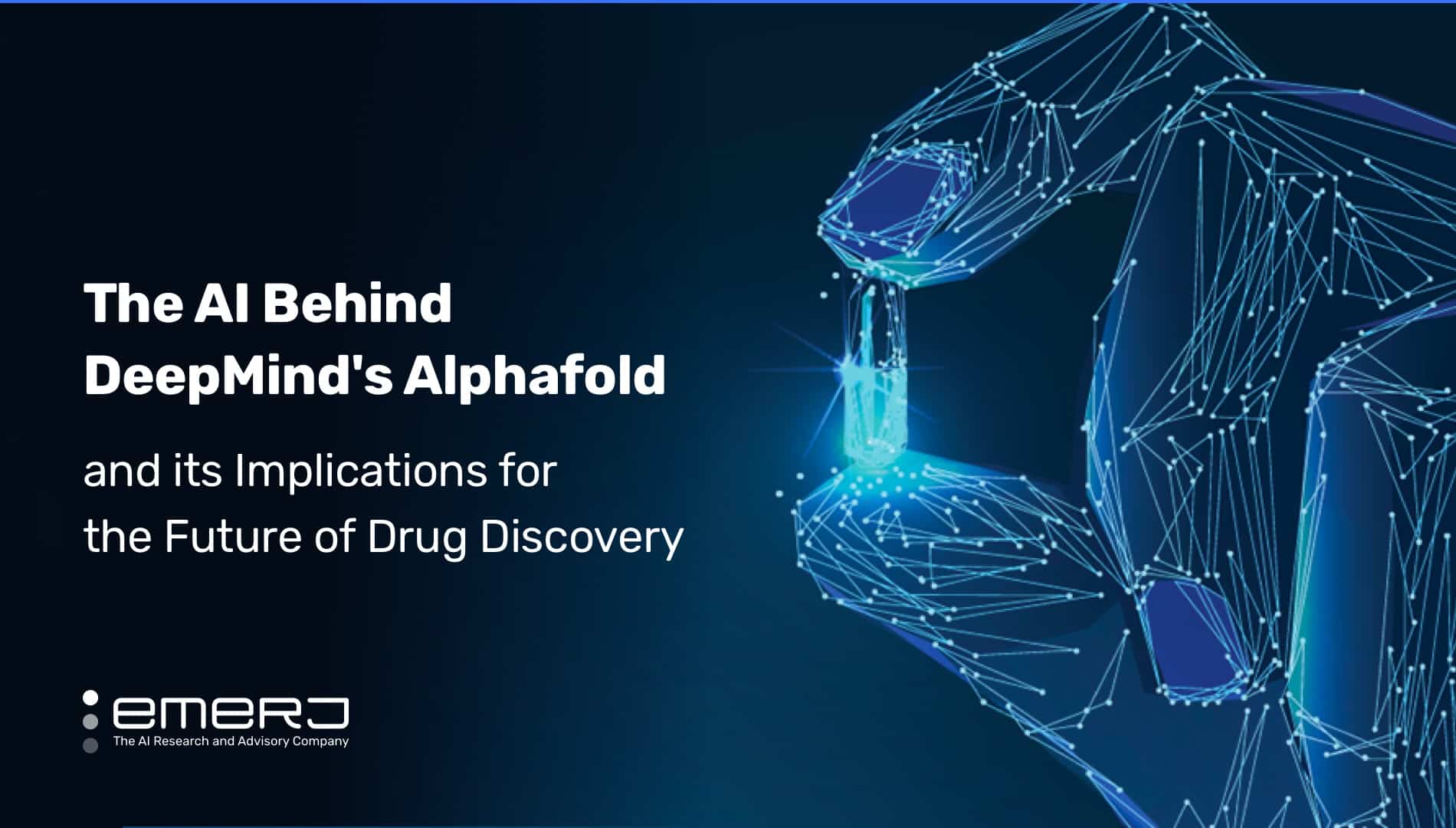
This is a contributed article by Kristóf Zsolt Szalay. Kristóf is Founder and CTO at Turbine.AI, and holds a PhD in molecular biology and bioinformatics. To inquire about contributed articles from outside experts, contact [email protected].
Could you predict how an airplane flies only based on an inventory of its parts?
This – with proteins – is the essence of the protein folding challenge.
Two weeks ago, the organizers of the CASP protein folding challenge just announced that DeepMind’s AlphaFold essentially solved the challenge – its prediction score was just below experimental error.
The fact that this should be doable has been known for a while – live cells don’t have an assembly manual so the section that codes for the parts has to code for the assembly as well. We could even calculate it starting from Schrödinger’s equation and building up – alas, not even the combined power of all computers on Earth is enough to do that for now.
DeepMind’s AlphaFold Achievement in Context
For proteins, looks are everything.
Like matching keys with keyholes, the shape of a protein defines its interacting partners, conversely its place and function in the intricate machinery of 20,000 different types of proteins that make up a cell.
For some proteins, their shape can be experimentally determined – that’s how CASP’s gold standard came to be in the first place. Others are very adaptable, they don’t really have a well-defined shape. Though there are other investigative methods to figure out how a given protein functions, AlphaFold should help in figuring out how many proteins in the human body operate. In this respect, the discovery is significant.
But this is something that can also be done without AlphaFold. Though 20,000 proteins is a big number, there is a limited set we would eventually uncover.
However, there’s a vast array of proteins that could never have been investigated without a tool like AlphaFold. There’s only one way to make a protein right, but there are a lot of ways, to make them wrong. Such mutant proteins belie many important diseases, most notably, cancers.
AlphaFold and its successors will likely be the only way we could figure out how damaged proteins look like and target them with drugs.
To appreciate the sheer size of the breakthrough, here’s a plot from Nature showing protein folding prediction accuracy over the last 15 years:
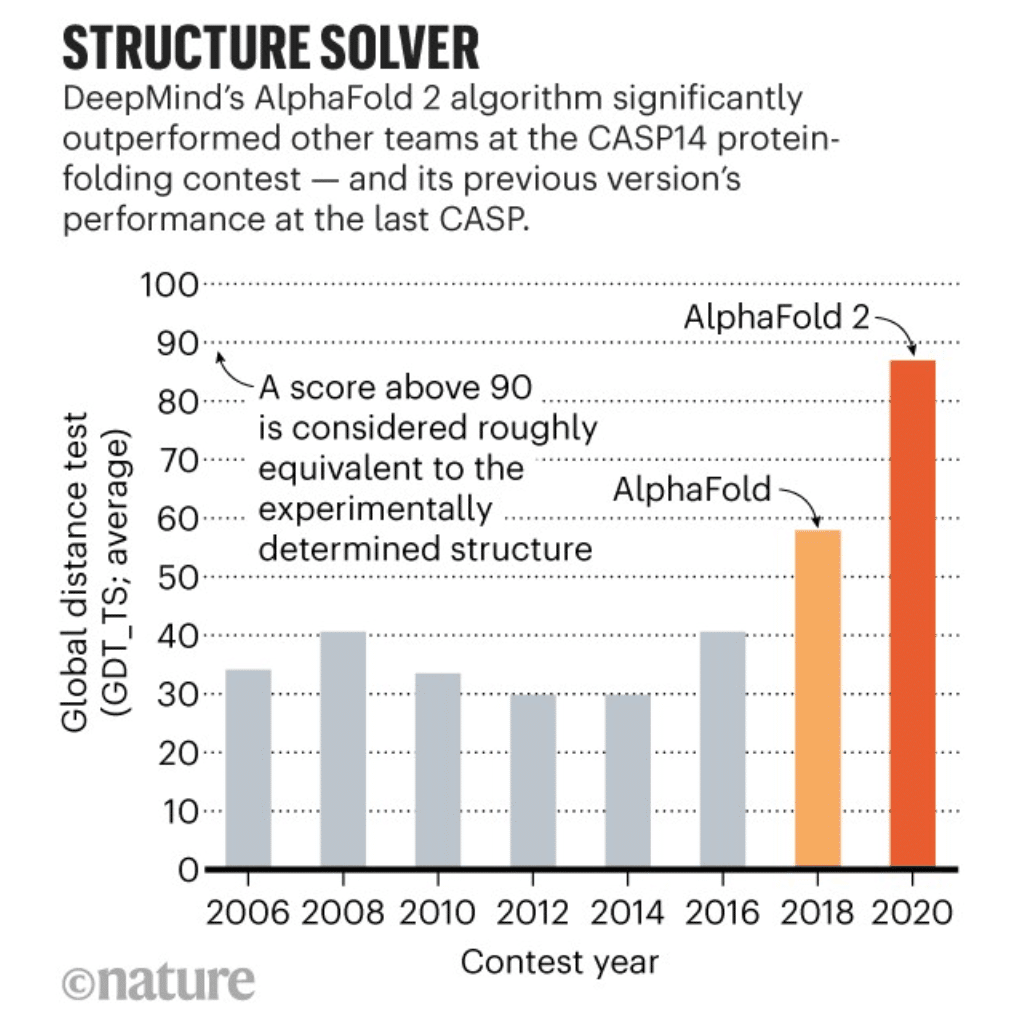
It basically stagnated around 40%, until AlphaFold2 came and in 4 years, dominated every other competitive approach to the task.
Surely behind a breakthrough of this magnitude, there must be some seminal scientific insight, right?
An Advantage in Architecture and Compute
There is a breakthrough here, it’s just not related to proteins.
AlphaFold2 is built on the same Transformer machine learning architecture as another breakthrough – Generative Pre-trained Transformer 3 (GPT-3) – the OpenAI language model that generates text in many cases indistinguishable from human writing.
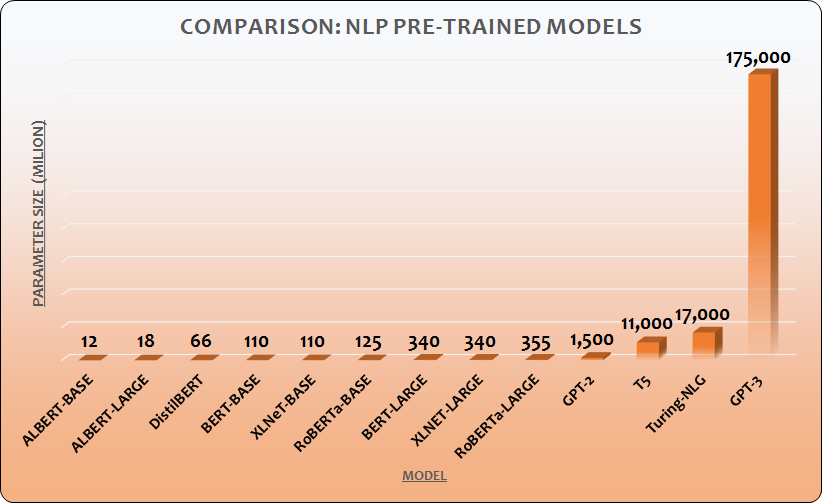
Transformer was designed to reduce machine learning training times. It is not an AI approach that somehow generates better or “smarter” results from the same number of parameters. Rather, what Transformer does is allowing to train models with billions of variables in parallel, using hundreds of thousands of machine-hours.
To that effect, AlphaFold2 could be argued to be much more of an engineering breakthrough than a scientific one. Since DeepMind merged with Google, they can use the resources and approaches from the infrastructure that powers a lot of the internet itself. I’ve heard it from multiple scientists that it doesn’t even matter if AlphaFold2 releases their source code as long as it just starts with “import Google,” a massive competitive advantage.
IBM’s Deep Blue supercomputer, upon beating Kasparov in 1997, was accused by some of not really mastering chess, but of simply apply brute force compute power to a range of possible moves and combination. Even an AI pessimist could still say that the achievement was significant.
We might say the same about AlphaFold. It didn’t “master” protein folding, it simply marshaled near-unlimited compute power (albeit in a remarkably sophisticated way) to find combinations that might have taken millions of years to compute without the hardware and AI approaches that DeepMind has access to.
As with Deep Blue, AlphaFold didn’t change drug discovery – it changed science. We can expect these engineering breakthroughs to make their way into other discrete problems in the sciences and industry, and we’ll likely see AlphaFold or GPT-3-like applications spring up in other scientific or industrial domains in the year ahead.
Implications for Academic and Private Sector Research Teams
I believe the main near-term impact of AlphaFold will be a flurry of new drugs, targeted against specific mutations, resulting in a lot of additional living years gained for patients.
The protein folding scientists were stunned by DeepMind crushing their field in just a few years, but this applies to all of us. Not many research teams or institutes are in a position to even pay for the training of the model, let alone recruit or retain a technical team that could improve on it.
Those of us not working at a Google-backed firm might still need to be scientifically smart, but we must build the muscles to be able to compete.
Academic research is not necessarily doomed. However, I also think it needs to change to stay relevant. I imagine the ones who stay will adopt a structure like CERN – a huge number of people working largely on the same goal, sharing a common infrastructure, just running different, related projects upon it.
Ironically, COVID-19 might have helped. To even fathom a remote scientific collaboration of this size was unheard of, but now we’re much more intimately accustomed to working over Zoom, Slack, and their friends, so it might just be possible. Heck, we have ways for thousands of people to write code together remotely, we just don’t really have the same systems set up for research. Maybe it’s time we start working on them.
Editor’s Note:
Private sector firms can benefit from learning about the architectures, tools, and training methods deployed by giant firms like DeepMind (Google) and OpenAI (Microsoft), but they can’t possibly compete on compute. They’ll also be unlikely to compete for raw ML engineering talent. Deep and narrow subject-matter expertise will be vastly more likely to bolster the profitable discoveries and breakthroughs of firms outside of big tech.