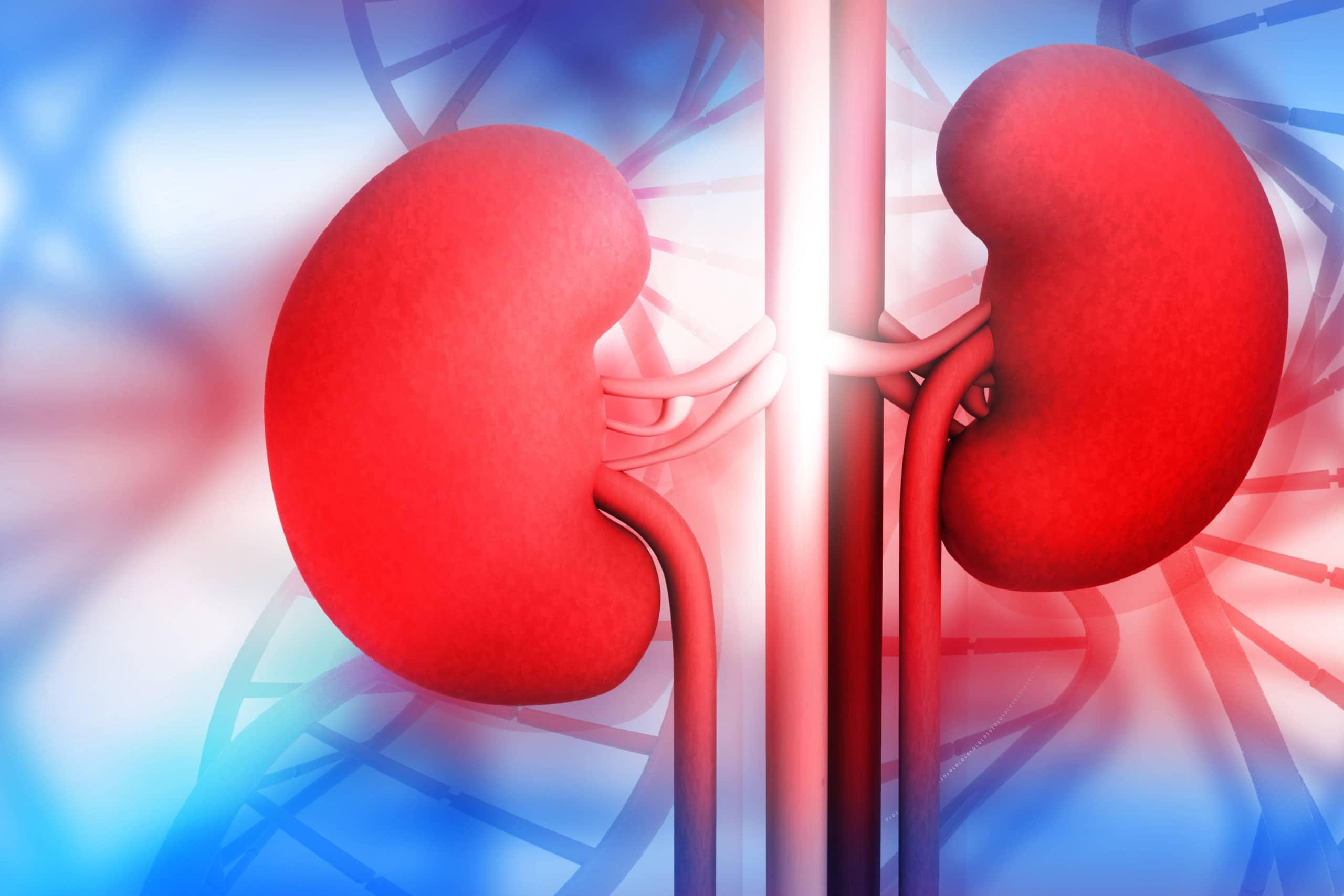
Chronic Kidney Disease (CKD) affects approximately 1 in 7 adults or an estimated 30 million Americans. CKD is characterized by kidney damage which impedes proper filtration of the blood. As a result, other health problems may occur due to excess fluid and waste in the body. According to the American Society of Nephrology, annual health care costs associated with treating kidney (renal) failure are estimated at over 32 billion.
In an effort to improve quality of life for individuals living with chronic kidney disease and in turn reduce the economic impact of this medical condition, researchers are testing the possibilities of AI applications.
Some of the questions that need answering to better understand the role of artificial intelligence in efforts to treat chronic kidney disease:
- What types of AI applications are emerging to help treat kidney disease?
- How is the healthcare market responding to these AI applications?
Kidney Disease AI Applications Overview
The majority of AI use-cases and emerging applications for treating kidney disease appear to fall into two major categories:
- Patient Monitoring and Prediction Models: Companies are using machine learning to monitor patients and to predict and prevent the onset of kidney failure.
- Medical Image Analysis: Researchers are developing software using machine learning to analyze kidney biopsy images to help prevent disease.
In the article below, we present representative examples from each each category, as well as the current progress (funds raised, pilot applications, etc) of each example.
Patient Monitoring and Prediction Models
The Anemia Control Model (ACM) Trial (ANEMEX)
In July 2017, a clinical trial was posted on the website of the U.S. National Library of Medicine where Fresenius Medical Care researchers are aiming to use AI to assist in managing anemia (lack of healthy red blood cells in the body) in patients with CKD.
The trial is anticipated to run from December 2017 to September 2019 and will enroll an estimated 240 patients. Specifically, Fresenius Medical Care claims that its software program is built on an algorithm trained on high volumes of patient data and can recommend medication dosages based on patient demographics and dosage history.
According to the clinical trial description, the artificial neural network architecture (ACM) used to build the algorithm “complies with the European requirements for medical devices.” Three dialysis clinics managed by Fresenius hosted a proof of concept trial in the Czech Republic, Portugal, and Spain.
The main goal of the trial to assess the effectiveness of the software program on anemia management in a routine clinical practice setting. If successful, the trial would help to prove the validity AI application tools for managing CKD. Currently, preliminary data on the progress of the trial is pending.
Through its global dialysis clinics, Fresenius Medical Care claims that it services 210,191 patients through 2,716 global dialysis clinics across the globe. To date, the company has raised $168.8 million.
Lytics
Originally founded in 2011 under a different name (Expertmaker and then Expertlytics), as of 2015, Sweden-based LYTICS claims to be using machine learning to help clinicians manage the care of patients with end stage renal disease (ESRD). Typically these patients are required to consistently undergo dialysis (process of excess water and waste removal through a machine) and may require a kidney transplant.
In an effort to improve quality of life, LYTICS algorithms were trained on thousands of patient data records from partner organizations and companies such as Boston Scientific, Lund University and Sony. As a result, the company claims that the system learns average patient health trends (for individuals living with ESRD) and performs analyses to identify or predict unusual changes.
The company’s suite of solutions includes applications for making predictions about hospital admissions and readmission rates and a patient monitoring system which alerts clinicians when the status of a patient makes any unexpected changes.
Wearable technology is worn by the patient to monitor vital signals and information is continuously relayed to computer monitors. Alerts may also be sent via text message directly to members of the healthcare team. It is not clear from the company’s website if the LYTICS solutions are cloud-based and include a mobile app component as opposed to the data being stored on a local hospital computer.
The animated 6:47 video below provides an overview of ESRD management and the role that LYTICS solutions may play in improving patient care:
Case studies on patient or healthcare system outcomes is not currently available on the company’s website. To date, the company has raised $1.1 million in private equity funding.
Western Chronic Disease Alliance
In May 2017, the Department of Health and Human Services in Victoria, Australia reported that AI was integrated in a pilot program for the detection of CKD.
The pilot was conducted at Western Health, Victoria hospital and led by researcher Craig Nelson, PhD, director of the Nephrology department. The pilot ran for a year from 2012 to 2013 and enrolled over 170,000 primary care patients.
The Western Health hospital system services an estimated population of 800,000 people. Results from the study indicated a 300 percent increase in diagnosis of CKD as a result of using the software program. However, the report does not specify the number of actual cases.
The software is built on algorithms trained to identify at-risk patients and to order relevant screening tests for CKD. The report also claims that the number of patients who reached benchmarks for health improvement nearly doubled as a result of the study.
The researchers currently aim to expand the software to other chronic diseases including heart disease and diabetes.
Medical Image Analysis
In January 2018, a team of researchers from Boston University published their findings on applying deep learning to improve the analysis of kidney biopsy images. In the paper published in Kidney International Reports, the researchers claim that six convolutional neural network (CNN) models were trained using images derived from kidney biopsy samples from 171 patients treated at the Boston Medical Center.
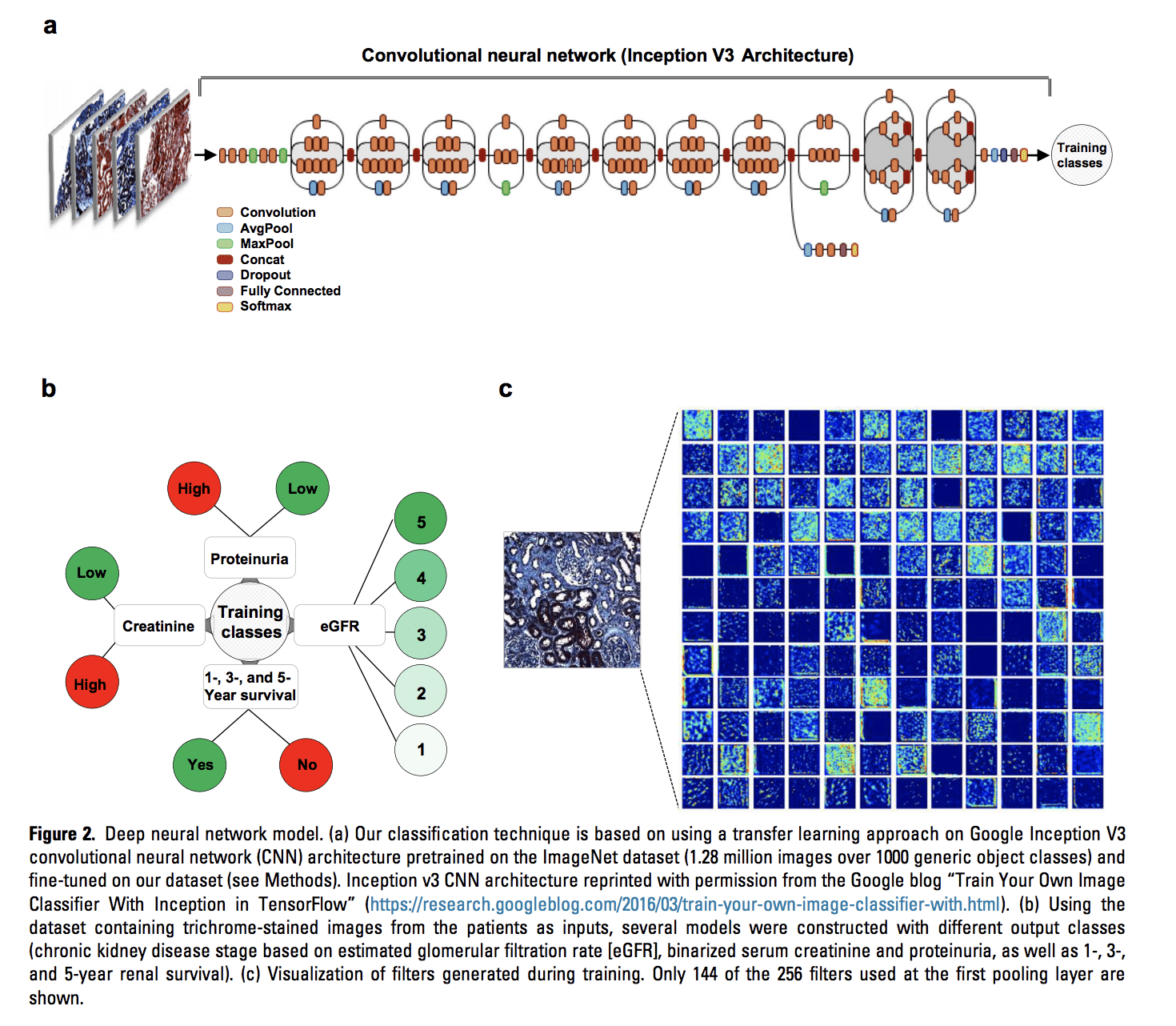
The goal of the study was to improve how the images are classified and thus optimize how images are assessed for their level of damage. For example, classifiers included 1-, 3- and 5-year renal survival. Renal survival is defined as the period of time between disease onset and kidney failure.
In comparison to traditional methods, results of the study conclude that the AI system performed better at predicting the stage of CKD; improving from roughly 78 percent to over 90 percent accuracy in the 5-year renal survival category.
In the following quote, researcher Vijaya B. Kolachalama, PhD, provides additional context on the current challenges facing clinicians in analyzing kidney damage and the potential for his team’s model in the clinical setting:
“While the trained eyes of expert pathologists are able to gauge the severity of disease and detect nuances of kidney damage with remarkable accuracy, such expertise is not available in all locations, especially at a global level.
Moreover, there is an urgent need to standardize the quantification of kidney disease severity such that the efficacy of therapies established in clinical trials can be applied to treat patients with equally severe disease in routine practice. When implemented in the clinical setting, our work will allow pathologists to see things early and obtain insights that were not previously available.”
Concluding Thoughts
Applications of AI for the treatment and prevention of CKD are showing some promise for bringing valuable insights to this medical sector. The balance of current efforts appears to lean more heavily toward patient management strategies.
Dialysis within the treatment center setting typically occurs an average of three times per week, ranging from 3 to 5 hours per session. The inconvenience and burden of traveling to the dialysis clinic multiple times each week can be a challenge for managing patient health.
AI has the potential to personalize patient care and to help clinicians identify intervention points in at-risk patients before they would require dialysis or an organ transplant.
The focus on prevention is important for helping to reduce the number of cases and treatment costs associated with CKD and ESRD. For example, the staggering annual medical costs have been estimated at over $98 billion for Medicare beneficiaries.
With the first efforts towards developing an artificial kidney currently in development, AI applications are poised to complement such advances in the medical field by providing users and healthcare teams with automated support to monitor performance and reduce the risk of organ rejection.
These AI applications may also reduce the amount of medication that patients are normally required to take to prevent the body from rejecting a donated organ. The waiting list for kidney transplants is estimated at over 100,000 individuals, with an average wait time of 3.6 years.
Header image credit: Adobe Stock