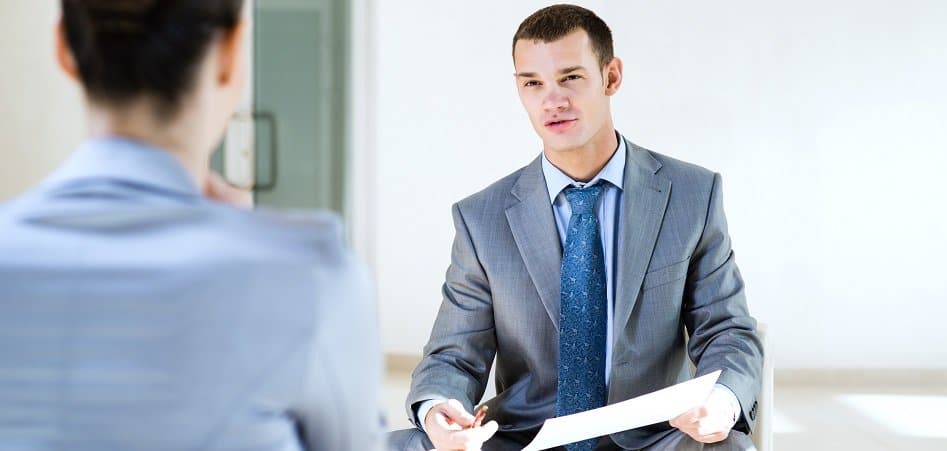
Episode Summary: In this episode of AI In Industry, we interview Nick Possley, the CTO of a company called AllyO, based in the San Francisco Bay area. We speak with Nick about where artificial intelligence and machine learning are playing a role in recruiting today and how picking the right candidates from a pool is in some way being informed by artificial intelligence.
Whether a business leader is hiring dozens and dozens of people or whether they ’re just interested in understanding how AI can engage with individuals on more of a one-to-one basis, this should be a fruitful episode. In addition, the fundamentals of what we discuss in this episode, in terms of taking in data from profiles and responding and engaging with applicants, could be applied to all sorts of cases, such as customer service and marketing.
Subscribe to our AI in Industry Podcast with your favorite podcast service:
Guest: Nick Possley, CTO, VP Engineering, VP Product – AllyO
Expertise: product development, software development, analytics, IT and security, machine learning
Brief Recognition: Possley holds a BS in Electrical Engineering from California Polytechnic State University. Previously, Possley served as a Vice President of Advanced Technology at Jolata.
Interview Highlights
(02:30): Where exactly does AI play a role in recruiting and hiring today? What’s it capable of?
NP: Well, there’s a lot of different aspects to AI in the space, anywhere from creating new ways to engage candidates and automate workflows all the way to doing some kind of special matching engines and searching and sourcing candidates. And I think it’s playing a role in all those different areas today.
And I think from the standpoint of sourcing is where you get a lot more, people would call instead of AI, they might re-term it machine learning algorithms that look for a pattern and then try to predict good candidates or find good candidates based on a specific kind of a pattern that they’re looking for and interpret data under, you might think of it as reading between the lines and looking underneath what’s in the information that is presented on a given person to really search for candidates that would meet a certain job criteria.
If we look into candidate engagement, it’s more along the lines of kind of what we’re doing here at Alio, which is natural language processing in terms of being able to have reasonably intelligent conversations and direct candidates, gathering information from them, and then using that to create a much better experience for them and also not just the experience but even automate many large pieces of the process for the recruiters and make things easier for them.
I think there is certainly matching engines and algorithms and things that are running that are actually able to do these kinds of tasks. The issue then becomes, does it introduce some kind of bias into the system? And that’s kind of the real topical thing that…I think there was a recent article about Amazon created some kind of tool to do something like this and they had to shut it down because it was biased. So you have to be careful on that standpoint of using existing employees because they’ll tend to bias towards them.
(07:00) I think what you’re probably hinting at, although you didn’t quite mention it, is maybe bias for some kind of moral factor that maybe we don’t like.
NP: Yes. I think the specific case that I was mentioning for Amazon was they found it to have a bias against women. So I think that was one issue that came up for them. There’s a general case that that may be a general issue in the industry, but there’s also problems of taking performance existing workers and then trying to compare that against what their profiles look like.
A lot of it heavily depends on the kind of target job market, right? If it’s the professional market, maybe they have a well done LinkedIn profile, maybe they don’t. So you may be missing out if you’re solely relying on that.
(09:30) When it comes to engagement, what I think is, okay, initial messages to candidates that might be a fit, maybe initial assignments and responses to answer basic questions, but there’s probably a lot more to it. Where does NLP really fit in there?
NP: Well, you can think about NLP is the way we think about it is our goal is to make recruiting delightful and efficient for everyone, including recruiters, talent management, and the applicants or candidates themselves. And it’s all about scale and automation from that standpoint. But I have to have a way to have a friendly, call it even delightful, type conversation with the candidate so they get a good sense, and it represents the brand of the employer that’s trying to hire them but does it at scale. I guess I take an example. If I have to hire 10,000 people, I can’t have 10,000 recruiters talking to everybody every day.
And the problem that you have is that since I can’t [inaudible 00:10:39] somebody every day, then people just get left behind. So you’re losing talent right there that could be entered into your talent pool and then somebody that may be better for the job doesn’t get lost. So if you, though NLP, you’re able to automate those conversations, represent the brand of the employer, and also engage all those candidates that you would normally not be able to talk to, screen them and assess them for their capabilities and skills, and then utilize that to have the recruiters talk to the best fit out of the entire pool. So that’s kind of where we’re folding in, but I’m seeing that that’s also a common trend to be able to do that more broadly.
Typically, if you go to somebody’s career site, they have a list of FAQs. Hey, what is the culture like at the company? Somebody could, in terms of NLP, you can put any sort of list of questions that somebody can come up with and you have set up the answers for those.
In fact, I think with a very broad range of the way they ask the question. Any way that they can ask the question, the whole point is to determine what the intent of the question is, which is something that you do utilizing AI from that standpoint. Then I can map that to, oh, they’re asking this question, and I have that question in my list, and I know what to give the answer.
(14:30): There’s some feedback process here, I can imagine. Is that right?
NP: Yes, absolutely. And so that’s part of it, is that we can gather up more information over time. As people ask more questions, we can add that to our list of questions, identify what all those intents are, and then be able to answer all of them. So over time, it learns and it gets better. And that’s a very key part of AI, is that it’s able to learn and improve over time. Another piece of it as well is on the screening and assessment side, there’s being able to go look at a job requisition that somebody may have on a website and figure out, what are the set of questions I should be asking for that? What kind of role is that?
That brings up a whole other section of technology and capabilities that you can use something more along the lines of what we call semantic web or ontology-based technology. There, you have basically an understanding of how all the information in there is related to a broader set of information and use that to determine, what set of questions should I ask to determine the skillset for a given job? So that’s also very interesting to help autogenerate a way to be able to screen candidates for a job.
(16:30): You mentioned kind of the semantic web, but it’s almost like other folks hiring for this may have found it useful to ask. Would it function in kind of a similar sense here, potentially?
NP: Well, there’s a lot of information that may already be available as like NAI and CS as an example of categorizations and understanding of what are all the attributes that are associated with a given job? So maybe a good example would be, you talk about Arby’s. Well, there’s a cook. Well, the cook has to have, maybe it needs a cooking school, maybe it has to be a certain age. There’s a set of attributes that are associated with that, a set of skills.
In the case for me, having a background in technology, hey, if I were to hire a software developer, I would want somebody, I need somebody who knows Python, I need somebody who knows Linux. So there’s kind of a list of things that I can come up with that would then represent that job, but that also ties in, I think as you said, to a lot of other types of similar types of jobs out there that are classified broadly for the industry.
Subscribe to our AI in Industry Podcast with your favorite podcast service:
Header Image Credit: Recruitment Agency Now