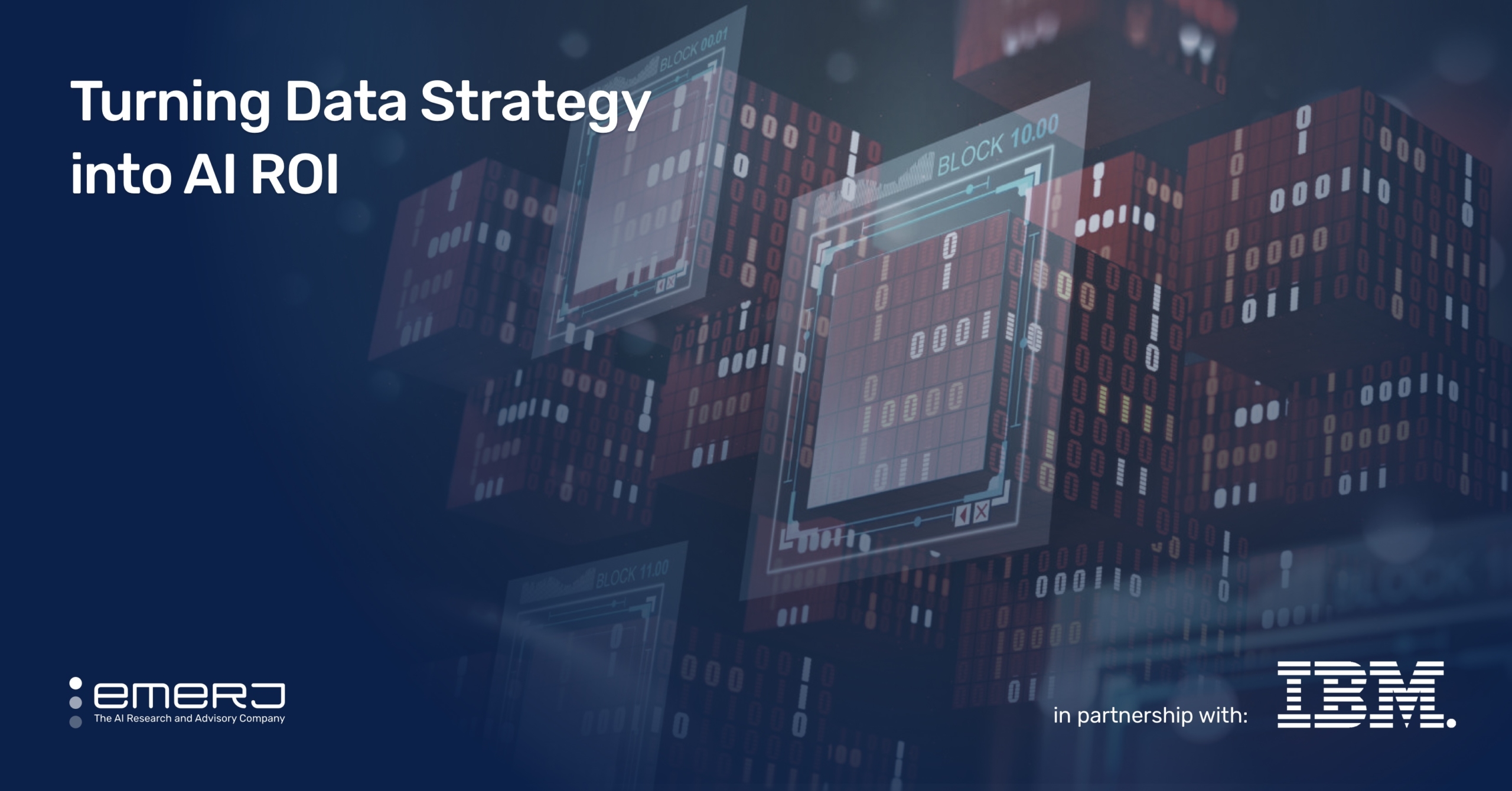
This article has been sponsored by IBM, and was written, edited, and published in alignment with Emerj’s sponsored content guidelines.
When we think about turning data strategy into AI ROI, it could be easy to assume that this comes easy to big companies like IBM. With big companies often come big resources, but they also face big challenges. To confront and address these challenges, IBM follows logical fundamentals that help set up business problems and envision solutions that harness the expertise of its cross-functional teams. There are a lot of ways to turn data strategy into AI ROI and IBM’s advice can be beneficial to companies of all sizes and at all points in their digital transformations.
In this first episode of a three-part series, we explore a long-term strategic way to think about data strategy and explore some use-cases that IBM is implementing today. Our guest this week is TJ Shembekar. He is a director of IBM’s Global Financing Division who is responsible for a portfolio of approximately seventy critical applications and for driving transformation initiatives for a core portfolio of leasing and lending applications. He has a background in finance, human resources, and computer science.
IBM provides integrated solutions and products that leverage data, information technology, and its expertise across a broad ecosystem of partners and alliances. IBM’s hybrid cloud platform and AI technology and service capabilities support their client’s digital transformations and draw from the experience and expertise of its globally recognized research organization. IBM generated $73.6 billion in revenue for the year ended December 31, 2020, according to its 10-K.
We cover three distinct topics in this 40-minute interview:
- Use cases and real-world pitfalls that companies hit during the “long haul”
- Constructing a long-term data strategy, and
- The importance of collaboration between IT and the business when putting a data strategy in place
Listen to the full episode below, or skim our interview takeaways and the full transcript below:
Guest: TJ Shembekar, Director of Global Financing IT at IBM
Expertise: Delivery of user experiences using Agile/Designing Thinking principles. Deploying global cloud-native solutions
Brief Recognition: Before joining IBM’s Global Financing Division in January 2018, TJ held positions within IBM as Director, Global HR; Services Integration Hub Leader; Global Account Director; and Associate Partner. Earlier in his career, he was the owner of Premium Capital, Inc., and the owner and officer in a Firehouse Subs franchise with 8 locations and 100+ employees. He also serves as a member of the Board of Directors for the Cirrus Owners and Pilots Association (COPA), a 501 (c)7 not-for-profit organization with 6000+ members.
Key Insights
- AI ROI targets projects that predict, not report. Instead of reporting on customers that have not paid their outstanding invoices or customers whose leases will expire within a set period, the best AI use cases help predict which customers will not pay their invoices or whether a customer with an expiring lease will buy out the equipment, return it, or just extend the term of the lease, for example.
- A close relationship between IT and the business is critical when identifying pain points. Discovering the pain points in the business that AI can best address comes down to developing and fostering a close relationship between IT and business stakeholders. Once you identify those pain points, it’s incumbent on IT to determine what to do about it.
Full Interview Transcript
Daniel Faggella: So TJ, I’m glad that we’re able to have you with us here today. And I know we’re going to be really sinking our teeth into data strategy and some of the core lessons learned there, but I want to start off with what is IBM Financing because I know everybody who listens to the show is familiar with IBM, but they might not be familiar with IBM Financing and exactly what it is and what you do. And then also, how are you folks using AI? So if you could open with that, that would be helpful as heck.
TJ Shembekar: Sure, absolutely and thanks for having me here, Dan. So first of all, IBM Financing is a captive financing company. It’s a wholly owned subsidiary of IBM, and in fact, it’s one of our four key operating segments that we report on externally. Like any other finance company, we face a lot of the similar challenges regarding of massive volume of data, how we leverage that data, how we make sense of that data. My role, I am the director of IT, so I am responsible for the entire application ecosystem, and most recently, my team has been heavily focused on how to apply AI to our portfolio for maximum business value.
Daniel Faggella: And I can imagine, you mentioned a wholly owned subsidiary here, obviously a substantial part of IBM’s business, in terms of what you folks finance and what kind of products are offered, I’d love to make it clear in the mind of the listener. I think when they think about a financial services firm, they might think of mortgages or something like that or obviously there’s an industrial financing where people are financing, let’s say, the purchase of a Caterpillar, giant earthmoving vehicle or something like that. When it comes to your financing operations, what is it mostly composed of?
TJ Shembekar: Sure. So we basically do loans and leases of IBM hardware, software and services. In addition to that, we provide primarily financing for our customers. But in addition to that, we also provide a lot of creative financing for business partners and some of the more complex transactions that involve IBM as well as other business partners.
Daniel Faggella: Got it. So potentially joint ventures, different kinds of creative R&D projects that might require some get started funding. IBM, your financing wing is the one that gets involved and figures out the amount of risk you guys are willing to take and what you could put in it and what kind of terms, etcetera.
TJ Shembekar: Absolutely. And that’s really where the AI impact comes in because we do a variety of different types of transactions, and there, we found that there was a lot of opportunity for us to leverage AI across all of our business processes.
Daniel Faggella: Got it. And maybe we could dive into a bit of that today. Strategy is going to be a big part of our conversation and clearly there was a lot of strategy going into the rollout of AI in such a large part of IBM’s. business here, but give us a sense. In terms of the kinds of applications that you folks were able to bring to life in IBM financing today, where are we at? What are some examples of what you’re using?
TJ Shembekar: Sure, we’re actually at the beginning of our journey, but we have probably half a dozen really powerful AI use cases in place already and they basically break down into two categories of use cases. The first are use cases that help our internal IT operations where we use data about our ecosystem to help drive activities by our IT production support staff to make sure that we have a resilient rock solid environment that’s up 24/7, problems that may arise in the ecosystem are addressed even before end users notice that they happen. So that’s the first category.
TJ Shembekar: But the second category is the one that is perhaps the more powerful one and this is where in the past, we used to focus on how do we make things more efficient for the business, give them better reporting tools, give them access to more data. But now with the advent of AI, our focus has changed a little bit. We’re not just creating dashboards and reports anymore. What we’re really focused on is figuring out how to leverage the data to evolve our business processes to be more effective. And a perfect example of that, a specific use case is in our accounts receivable function. Like every other business, we have a very robust accounts receivable function. We process tens of thousands of invoices per month and collecting all of that money is a really high focus item because it has a direct impact on our cashflow and our treasury operations.
TJ Shembekar: In the past, what we used to do is we would wait for an invoice to see if it was paid on time. And if it wasn’t paid on time, then we would go take some actions to try to collect the money. Now with the advent of AI, we’ve gotten a lot more effective in this process. So instead of waiting to see if an invoice is paid on time, we use AI to predict whether an invoice is going to be paid on time. And by doing that 30 days in advance of the due date, it gives us an opportunity to take some proactive steps that might help us influence the outcome. So this is a big change from the way our AR function operated before the advent of AI. Instead of being reactive and just responding to something that happened in the business, we’re predicting what we think is going to happen in the business and then actually taking action in advance to influence that outcome.
Daniel Faggella: I like this application. I think that certainly folks that have listened to our show for long enough over the years, but maybe folks in general familiar with AI will understand AI’s applications in lending. Who do we say yes or no to? What kind of terms do we say thumbs up/thumbs down to for what kind of purchases? The collections use case I think is less well known but clearly feels like a somewhat inevitable long-term trajectory for collections operation, certainly at a firm with this as much of that kind of collections activity. As you folks, I would imagine, and tell me if I’m wrong, what I like to do with use case is to paint a picture in the mind of the listener, so that they can imagine workflows in their own business and how some of these ideas might transfer.
Daniel Faggella: What it seems like to me is you have a rolling record of who’s paid, who hasn’t, “We know the geo region. We know the kind of contact. We know the product that was purchased. We know various and sundry factors about the person, the deal, the terms, etcetera. We figured out who’s paid, who hasn’t.” Maybe there’s other factors like communication frequency via email or something along those lines. I’m not exactly sure. “And various historical records of what payments have come in, which ones haven’t can give us an indicator as to which ones are most likely to come in and which ones aren’t.” Am I conceptually on the right page and is there anything you want to clean up about the picture I just painted?
TJ Shembekar: Yeah, conceptually, I think you’re exactly right in your description, but another nuance to the situation is that the first step, this is never a static singular event, this is a continuous iterative process. So in our case, we first came up with an algorithm that allowed us to predict whether an invoice was going to be paid on time. After we did that, then our collectors started taking proactive actions on those invoices that we thought had a high probability of being late. And what we did is we kept track of what actions collectors took. And so the model was fine tuned to the point that not only does it predict what’s going to be late, it tells you the best course of action to take as a proactive measure.
TJ Shembekar: So maybe for some clients, the right thing to do is to send them a statement of account five days before the due date of the invoice. For another client, we may have a different action. Maybe we need a text message. Maybe we need a copy of the invoice sent. Maybe we need some other action. The key is that the AI algorithm is continually evolving based on current activity and current data.
Daniel Faggella: And determining … I would imagine in real time, in an ideal universe here, in real time, we’re learning, “Okay, we made these number of phone calls in this particular way and then this kind of a deal collected,” and if we have, let’s say, 10,000 such bits and pinches of feedback for different kinds of products over the course of a given month, the algorithm might be more trained to say, “Okay, we should lean maybe a little bit more in this particular strategy for this particular account.” Now, it would seem as though it’s very … I would imagine, tell me if I’m wrong, that the bulk of these operations that we’re talking about are for many of your core products.
Daniel Faggella: In other words, the collection strategy that your algorithm can predict and it’s most likely to work is probably not the super unique, really wild deal that you did with like a shipping port in the Philippines one time that was unlike anything else you’ve ever financed. Probably it’s like a company between 5 and 20 billion, they bought this particular IBM product on these particular terms or maybe a company even smaller, maybe you have more deal flow with those kinds of firms, that the pricing was a little bit more standard. I would estimate that the more standard kinds of deals are the ones where you’re able to get more meat off the bone in terms of value from this AI. Let me know if I’m on the right page.
TJ Shembekar: Not exactly because, believe it or not, once we get to the point of an invoice being generated, it is a discrete transaction and what the complexity of the underlying contract, the complexity of the terms of the invoice, those are all input data factor, datapoints into the model. So we may use 30, 35 different datapoints that feed into the algorithm and so it’s not as simple as saying a complex deal is less accurate than a simple deal. It’s actually not. For us, in aggregate, we can predict with greater than 90% accuracy whether an invoice is going to be paid on time, regardless of what kind of invoice it is.
Daniel Faggella: Man, well, I’ll tell you. I would be, again, if I think about analogous use cases, I would be absolutely shocked if your biggest, most wild unique deals were just as accurately predicted as the stuff that’s astronomically common, but I see where you’re getting at, you’re saying that you know, at least according to where you’re at with the tech now, these are all just factors that we enter in on the frontend and we have relatively similar predictions of both collection likelihood as well as the strategy to use. And of course, it sounds like for you guys the goal here is to continue to feed this system and have it evolve over time.
Daniel Faggella: One quick final question on this use cases, I think it’s a very unique one, and in my personal opinion, I think just like lending, collections will inevitably be embedded with AI and this is not something that’s going to go away. So I’d love to tease out one more question for the audience. It seems to me like one of the big, big factors for making this work was determining what are the data inputs. So you’re more aware of this than I, but it’s tough to track everything in an algorithm, right? If I’m a salesperson and I drive over to the golf course where Jim golfs and I buy him a bottle of scotch and I say, “Look, pal. I know it’s been a while, but by golly, it would mean a lot if you could just send Susan another email and see if we can get this thing paid. It’s been 60 days and yadi-yada.”
Daniel Faggella: That kind of stuff which doesn’t happen never, those things occur, is a little tougher to quantify and same thing with a phone call. So, okay, I made a collection call. Well, was it the person in the collections office using some default script or was it the sales guy himself who has a relationship who pulled some strings and talked about how their kids play in the same soccer team? How do we quantify in features the kinds of activities that lead to collections because it’s not as simple as, “Sent email, sent call”? ow do we handle that complexity?
TJ Shembekar: Yeah, and that’s something that we’re iterating on. At a minimum, we start with the type of contact and what was the depth of the contact. Was it targeted to the specific person in accounts payable that we know is the person that triggers the payment or is it to a generic counterparty in the other company? Is the material that we actually send them, is it a phone call or is it an electronically generated thing coming from a machine? All of those factors, we’re keeping track of what we’re trying and what we’re doing is we’re tracking what works and what doesn’t by specific client. And that client, we try to draw parallels to, “This client is similar to this other client and here’s the actions that worked for this other client. So why don’t we try it for this client?”
TJ Shembekar: Those are the things that we’re experimenting with now and that’s really the power of the model, is that whatever actions we take, all it’s doing is creating more datapoints that get fed into the algorithm to predict what the right next best action is.
Daniel Faggella: Certainly, but as you said, there’s a lot of experimentation required. And this is going to lead us very naturally into strategy which I’m excited to talk about with you because you’ve had such a high-level mandate for rolling out AI that you’ve inevitably learned a lot here. But as you mentioned, there’s going to be some tinkering with this because even like you said, “Well, we want to apply it to similar companies,” even that statement, if we want to train an algorithm on that, man, which industry ontology are we going to use? Which geo region ontology … Do we want to say US or do we want to say northeast or do we want to say the state or the city? And so there’s no there’s no obviousness as to how to slice up industries revenue, types of company, if they’re based in Bangalore versus based in Boston. They might have a little bit of a different culture in terms of when and how they pay. Those things might need to be considered.
Daniel Faggella: So the ontology for how we track actions and how we track similarities across customers, it sounds like something you guys put a lot of thought into and something that’s going to be evolving over time as you guys build out and continue to improve the system.
TJ Shembekar: Absolutely. And that’s where the data fabric that underlies all of your AI use cases, comes into play. So when we started with the AR use case, we took literally every scrap of data we could find across all of our systems. And most large companies, we don’t necessarily have one system globally that handles all invoicing and all payment processing worldwide. We have several. And so the first thing that we had to do is we had to create that data fabric at the core that was the starting point and then we used Watson’s machine learning capabilities to experiment with AI algorithms. And we may start with a dataset of a thousand different datapoints. And that’s where it comes down to which ontology matters.
TJ Shembekar: And maybe we find that the industry is not as important as the geography or maybe the industry is more important for some industries. And so those are the things that we use the Watson machine learning tools to help us figure out which datapoints are significant and then whittle down your dataset to the datapoints that are the most important. And that’s what we did with our AR use case. I guess that that is just one of the use cases. Another use case that is very relevant for our business is this concept of what a customer decides to do at the end of the lease.
TJ Shembekar: So a lot of our transactions are leasing of equipment and at the end of the term of the lease, we have to figure out what does the customer want to do with that lease. Do they want to buy out the equipment, do they want to return the equipment or do they want to just extend the term of the lease? All of those things, what the customer decides to do, has a massive impact to us in terms of workload for different parts of our business. So we started experimenting, using the same approach with creating this data fabric, pulling in what we think are all the relevant datapoints and then experimenting with machine learning to figure out, “Can we predict what are customers going to do at the end of the lease?” That’s another use case that’s incredibly relevant to our business.
Daniel Faggella: Oh, man. Well, that throws me back to reading about the history of IBM back when you guys had punch card machines in the old days of Watson himself, where so much of the business and the big boom of growth was in leasing of those big punch card machines, selling more of the cards and then leasing the machines themselves. It’s interesting to hear that still a big part of what you’re doing is you’re leasing out equipment. It’s actually funny to see that there’s some continuity there with 100+ years of IBM.
TJ Shembekar: Well, obviously, the punch cards are long gone. Certainly, the System Z mainframes are still alive and well and they actually are the backbone of quite a bit of the financial services industry.
Daniel Faggella: Indeed they are and I think that might come as a shock to some of you who are tuned in and are working in ecommerce or FinTech proper, but certainly, financial services still got some of the older stuff. So anywho, all right, well, we’re going to swivel into strategy. You’ve been at the helm leading a number of different AI applications heading up it for this division of IBM and data strategy has to be a part of thinking through all this. You and I have already just gone onto the hood very lightly about the challenges of ontology, never mind the challenges of how many different data silos there were and assessing your data assets must have been a big part of picking what use cases to choose. How did you think about data strategy through this adoption process? What was your kind of philosophy there?
TJ Shembekar: Well, the philosophy really is an evolution of the old data warehousing concept. So traditionally, what people would do is, they would have lots of different systems that they had to aggregate data. They would create some warehouse or data lake or whatever the term they would use at the time. And they would feed this source with a whole bunch of interfaces, okay? And typically, for a large organization, that ends up creating an environment with thousands of interfaces that run on various frequencies, whether it’s daily, weekly, monthly, or even near real time in some cases. Ultimately, you end up creating an army of people that have to make sure these interfaces are constantly working.
TJ Shembekar: With the new tools that are available, with cloud native architectures, we are building a data fabric that instead of interfaces feeding a source, we create connections back to wherever the data actually resides and then we create governance rules of how that data gets aggregated. And once it’s there in our data fabric platform, all of this is built on cloud pack for data. Once we have the data connections built up in there, that’s when you have the luxury of starting to think about AI use cases. And that’s when you start to work with your stakeholders and start talking about not just, “What kind of report do they want?” but, “What kind of challenges is the business process fundamentally facing and is there any predictive capability that could help improve the process?” not just speed up the process but improve the process.
Daniel Faggella: Even just thinking about where you want to apply that fabric. I imagine, as you look over, IBM Finances various and sundry silos, functions, departments, etcetera, how did you even make the choice as to which of these sources do we think are going to be worth jacking into in the first place? Which of these we want to get to ground truth on in the first place? Because clearly, the answer would be, “Oh, it’d be great to just have them all,” but of course, there’s a lot of effort here. What was that process like because that’s a huge strategic choice for you.
TJ Shembekar: It absolutely is. And this is where the relationship with the stakeholders come in. The way our organization is structured, is by function. So we have a credit function. We have a capital markets function. We have an area that focuses purely on syndication. We have a whole bunch of different business functions and each of them have different challenges. So we have our applications broken up by functional area where I have a leader in my organization that’s working closely with a product owner and that’s an agile term. Our team is all structured as an agile operating model. So we have distinct squads and tribes that work on individual functions.
TJ Shembekar: So we have a squad and tribe that focuses on the commercial financing team or the credit function. And working with their product owners, they get to know what the pain points are. And for example, in the credit area, one of the challenges that they struggled with was we do business with a lot of large organizations, but if you deal with a large organization, XYZ Enterprise, they might be XYZ Limited Australia or they might be XYZ Mexico Services. And it’s very challenging to figure out, “Is XYZ Mexico Services, is that really part of XYZ Enterprise?”
TJ Shembekar: And so we started trying to figure out how to figure that out and so we started using additional data sources, not just internal, but external data sources to help us figure out how to create an entity match, how to identify who the customer is that’s actually asking us for credit at this moment. And so that sort of relationship with the stakeholder is how we figure out what to go after. The data fabric is the enabling tool that lets us go and create different use cases, but ultimately, the close relationship between IT and the business stakeholders, that’s how you identify where the pain points are. That’s how you figure out what the opportunities are.
Daniel Faggella: Got it and I see a big opportunity. Speaking of opportunities, for our listeners here in terms of learning from some of this experience, so many enterprises are a little farther behind in AI adoption than IBM and they’ve yet to have any kind of, from the top, mandate to really apply AI in a deeper way. So as you guys are well aware, a lot of enterprise projects, the vast majority up until this point, are popcorn projects existing in isolation, not really considering a broader data ecosystem or some grand business strategy where AI plays some important role. They’re more plug and play on the side applications.
Daniel Faggella: You’ve gotten to do what I think the enterprise leaders who get it want to be able to do, which is, “Hey, how could we wake up our data systematically? How could we unlock the pockets of value throughout the business and find the connections between the value and the different flows of data that we have and do so in a way that builds a broad suite of capabilities?” And you even talked about them when we started. You didn’t talk about one or two random isolated use case. You talked about categories of capabilities. This is what our listeners who get it who’ve been tuned in for a year, they’re frothing at the mouth wishing that they were able to do this in their organization. And one day, they probably will, I hope, but you’ve been there.
Daniel Faggella: So here’s maybe a question that will tease out some value for the listeners is when you went about executing on this mandate, you had to then engage, I imagine you have a team underneath you that’s pretty substantial, but you yourself, I imagine were a big part of this engaging with the different parts of the business, the different functional heads, the different maybe subdomains of IT and data science talent in the different parts of IBM Financing. You had to pull those folks together and ask the tough questions to figure out, “Where are the data sources that are valuable and clean and harmonize? Where are the ones that we think have predictive business value?” and figure out how to bootstrap that data strategy based on the expertise of those around you. How did you go about that process?
TJ Shembekar: It is what you said, it is really the relationship by function. So at my level, I would work with my counterpart who’s heading up the credit function and I would try to get to understand what her biggest pain points were. And that’s how we came up with this discovery that matching entities was a big deal for them. And prior to those conversations, I would have never guessed that that was a big deal. I would have thought the credit team would focus purely on how to come up with a more accurate, more effective credit rating. And I didn’t realize that their biggest struggle was when they get a credit application, figuring out if it’s part of a larger enterprise is a big deal for them because that tells them whether they’re analyzing credit for a little regional subsidiary in Mexico or whether it is part of a larger organization that is global.
TJ Shembekar: So that’s an example, but it is nothing more than having deep relationships with your stakeholders, understanding where their pain points are. Now, once you get a sense of their pain points, then it’s incumbent on the IT organization to figure out what do you do about it. And I’ve encouraged my team to develop their skills in this area, so that some people in my team, they’re focused on creating automation tooling. So in some parts of the business, some functions, their biggest problem is that they have a whole bunch of manual steps in a process and they need to automate things.
TJ Shembekar: So in that case, we may help them with automating the process, but usually, we don’t stop there. Usually, we start asking probing questions around, “Should we really be automating this process or should we be eliminating this process by doing something different? Could this manual effort be eliminated if you had some better reporting capability or if you were able to predict some specific data element? Could that change your process?” And that’s where the real value comes in because then you go from not just, “I’m creating you a report or I’m creating you an automation, but I’m helping figure out how you can leverage the data we have with AI tools to possibly change the process.”
Daniel Faggella: And the part of this that involves a lot of work is not only how much complexity there is in one division, but how many of these different experts and peers of yours that you have to connect with to figure out where those pockets of value are. And also, like you mentioned, it’s not always self-evident like, “Okay, here’s the data we have. Let’s just take all that for granted. Here’s the state of affairs. Let’s take all that for granted and then let’s figure out how to use AI.” Sometimes you’ll look at a workflow and say, “We should have a more unified way of taking in data here in the first place. We should not be solving this by cleaning it up with algorithms on the backend. We should unify how the heck this stuff is flowing into the system in the first place with way less human error because that’s our ultimate problem here.”
Daniel Faggella: So you’ve got to do that level of depth of diagnostics. It’s not just jump in with the cool Python tools. You’ve got to also think about the gradation of, “At what level does this problem need to be solved?” So you go around the circle. You got your peers. You figure out their most important problems and potentially their pockets of valuable data and then you have to figure out at, “What’s the priority of these various potential applications and what’s the level at which I want to address them?” And all of that has to happen, I would imagine, it feels like it’s over years to me, but talk a little bit about the timeframe and the way that you approach such a big elephant to eat.
TJ Shembekar: Sure. For us, we really started in earnest on this journey, this modernization journey at the beginning of 2018. And at the time, we were really focused on trying to deal with the challenges of aggregating data from multiple data sources. That was our initial big challenge. As we started doing that, we realized, once we can get a way to aggregate the data into a consistent platform, then suddenly we can finally get to the conversation, “Wat are we going to do with this data now?” Prior to 2018, honestly, we were focused on aggregating. We were struggling with aggregating. And part of it is just the complexity of the systems, the volume of data, the sheer amount of data.
TJ Shembekar: But ultimately, once we overcame that hurdle, then we got to the more powerful, the more meaningful discussions about what you do with the data. And the tempting thing is to say, “Great, now you have all this data in a way that I can get to it. That’s great. Let me just do what I used to do with seven different reports and let me just make it faster with one report or a better report or something.” That’s the temptation, but the business may ask for that, but that’s not necessarily what will truly help them. And that’s why it’s incumbent upon the IT group to help them understand some of the possibilities. And a very famous quote that somebody told me, I don’t know who, “If you ask somebody at the turn of the century when Henry Ford was tinkering with automobiles, if you ask the customer what they wanted, they would say, ‘I want faster horses,’ but you don’t want to give them faster horses, you want to give them a better solution to what they’re trying to do.”
Daniel Faggella: And that was Henry Ford himself, so an eminent person behind that quote, and certainly, you have to steward that strategic role yourself. And frankly, that’s a tough one because you’ve got very powerful people who are not necessarily underlings, right? These are peers, these are big leaders who’ve got important agendas, but there has to be a shared level of trust where you can step in as what we call the catalyst to not only say, “Yes, I’ll take your order,” but, “Hey, here’s what this capability means. Here’s what we’re building towards. I want to talk about how we could think about this, right?”
Daniel Faggella: Instead of slapping on whatever the Band-Aid is and doing the popcorn project strategy we were talking about, you’ve got to have enough trust to be able to actually share ideas in a way that without framing it any other way in a way that educates these leaders, right? Because they might not know that, “Oh, there’s a broader capability to build, right?” They might just be thinking in terms of plug and play. So it feels like there’s a lot of trust and tact in those conversations.
TJ Shembekar: Yeah, absolutely. And we’re fortunate at IBM Financing that the IT organization and the business functions have a really tight working relationship and there’s a tremendous amount of trust that goes back and forth. As we’re dealing with, for example, our sales enablement area, this is an area that is always a high focus area because sellers always are motivated to, “How do I get the information I need to close the next deal? How do I know which deals I should be working on?” Being able to provide them with tools and predictive capabilities that they can rely on that truly gives them outcomes, that’s something that requires that level of trust. So we ask them, “What do you struggle with?”
TJ Shembekar: And when we talk to a seller, one of the things they would struggle with is that, “Well, we use this CRM system that highlights our opportunities that are out there.” And generally for an IBM Financing seller, they’re focused on IBM opportunities, whether it’s hardware, software, infrastructure, whatever and they’re figuring out which deals they should help drive from a financing standpoint. It would be really helpful for them if they could predict which opportunities not only that are going to close, but are going to close where the customer is likely and receptive to use financing. That whittles down the opportunity pool to a much more manageable level for a seller. So that’s another example of another use case where, by working with the stakeholders, we found out what their pain point was and then we came up with a way of leveraging the data we had to help with that.
Daniel Faggella: Got it. So this is cool. So we’re getting to crack open a number of the individual use cases you’re working with. And one thing I knew I wanted to chip in on before we wrap up here is the idea of a data fabric as a concept. In terms of summarizing the concept for business people, how do you nutshell it so that it can click? Because we’ve talked about it. I think I have certainly a bit of a better picture than when we started, but how would you frame it for the listeners?
TJ Shembekar: I’d say the main differentiator is that instead of creating a warehouse where interfaces feed a static place, a data fabric is creating modernized cloud native connections to data at their source and making them available to leverage.
Daniel Faggella: Got it. So this is the idea of a data fabric. And the advantages here are closer interfacing with the ground truth data as opposed to pulling from some other aggregation and having that obfuscation in the middle. Is that a good way of nutshelling it or how would you frame the benefit there?
TJ Shembekar: That is one of the benefits. The second even more powerful benefit is that by having a data fabric that has AI machinery plugged into it, that allows you to leverage the data once you have that unified view from the true source.
Daniel Faggella: Got it. Cool. So two bigger upsides to bear in mind. Final point here, as we wrap up, we’ve talked a good deal about some of the challenges of coming up with ontologies and how that’s, by no means, a solved problem. We’ve talked about the process. And I think many businesses don’t have the patience to do what you folks did … It sounds like you went through the big hard long journey of wrangling and aggregating and finding and sorting on some level, maybe even harmonizing a lot of your data and then you went through the process of enacting a real strategy and starting to build capability on top of that. You didn’t just find little pockets and run little spin up, a little spin up AWS project here or there or the other place where we see a lot of enterprises go.
Daniel Faggella: So you’ve gone through that long process and there was clearly a lot of issues there with data. When you think of considerations outside of data that made this process fruitful for you guys, that you would consider to be a success factor in bringing AI to life, into ROI within IBM Financing. What are some of those takeaways? Outside of technical data concerns, what are some of the factors that made this a win for you?
TJ Shembekar: Well, I’d say, as we work with our stakeholders, one of the things we were always on the lookout for are elements of the business process where we react to some event happening. In AR, they reacted to when a bill was late. In end of lease, they reacted when a customer told us what they want to do. Any part of your business process that has a reactive element to it could benefit from predictive AI capabilities in advance of the reaction point. It happens very much in the business side. It also happened for us on the IT side where we were, instead of waiting for an outage or a server space problem, we were predicting when failures would happen and then proactively doing something about it in advance. So that’s, I think, the big challenges.
TJ Shembekar: In the past, we were never trained to look for that. What we were trained to look for is look for a process that has manual steps and see what you can do to automate it or look for a process that takes too long and find a way to make it faster. We’re not looking to just improve purely the speed of a process. We’re trying to look for a way to use AI to improve the overall outcome of the process.
Daniel Faggella: Well, so this is a maybe a mindset shift point for the listener. And those of you who’ve listened in for a long time, you’re well aware that we do not beat the drum of AI equals automation. That’s a very, very limiting scope of mind. And clearly, you had to go in with a much broader scope of, “What are the new capabilities we can bring to life?” And I like the idea of, in terms of finding projects, what you’re saying is not just, “What’s a long process we can make short?” but, “What’s a process where we’re reactive and how can we leverage the power of the data in those workflows to become proactive?” I think that by itself, it sounds like for you guys, it’s been a source of finding a lot of fruitful use cases.
TJ Shembekar: Absolutely.
Daniel Faggella: Excellent, cool. And hopefully, it’ll be the same for some of our listeners who are tuned in. I know we went a little bit over time, but, TJ, this was all excellent material. I really appreciate you sharing your experience and I appreciate you joining us on the show. Thanks so much.
TJ Shembekar: Thanks for having me. Take care.