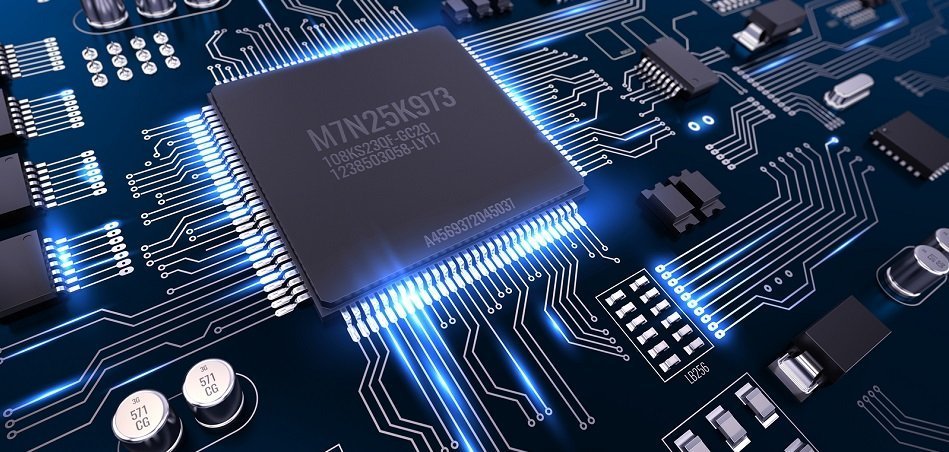
Episode Summary: Some businesses are going to require a sea change in the way that their computation works and the kinds of computing power that they’re leveraging to do what they need to do with artificial intelligence. Others might not need an upgrade in hardware in the near term to do what they want to do with AI.
What’s the difference? That’s the question that we decided to ask today of Per Nyberg, Vice President of Market Development, Artificial Intelligence and Cloud at Cray. Cray is known for the Cray-1 supercomputer, built back in 1975. Cray continues to work on hardware and has an entire division now dedicated to artificial intelligence hardware. This week on AI in Industry, we speak to Nyberg about which kinds of business problems require an upgrade in hardware and which don’t.
Subscribe to our AI in Industry Podcast with your favorite podcast service:
Guest: Per Nyberg, Vice President of Market Development, Artificial Intelligence and Cloud – Cray
Expertise: international market development, go-to-market strategy, sales and partner management
Brief Recognition: Nyberg holds a Bachelor of Computer Science degree from Concordia University. Nyberg has worked at Cray since 2002.
Interview Highlights
(2:30) What are the kinds of business problems or kinds of sectors where this sea change in hardware is going to be borderline necessary?
PN: Yeah. That’s a good question. Maybe a couple of different perspectives there. One is certainly that we look at AI in the enterprise, we kind of think about the journey, if you will. Early on, it’s really all about just understanding the capabilities of machine learning. At that point, it is very much around the skills within the organization and the business problem that they’re trying to address. As organizations kind of progress through their journey, so they convince themselves that there is some value there to their lines of business, and they move into more significant implementations or operationalizing AI, it’s kind of at that point that the infrastructure really does start to become really important.
We’ve seen that in our customer base. We’ve had companies come to us and say, “We’ve been experimenting with AI or deep learning now for nine months, and then all of a sudden, we increase our data sizes and we need faster training or we need faster storage”, for example. We see that arc through the journey where, again, the infrastructure really does start to become a critical and necessary part of their journey.
In terms of specific use cases or problem areas, again, I think that where we see today, areas in like manufacturing, obviously things like autonomous vehicles is the one that a lot of people hear a lot about and it resonates with them, but in the manufacturing space at large, we see everything all the way into really interesting areas that people are looking into, like what they call cognitive simulation, where they’re using machine learning to improve their computational fluid dynamics, for example. You see it permeating throughout that industry. Then obviously, areas like life sciences, healthcare, financial services, oil and gas, so you really see it across that large industrial base.
(6:20) What separates those problems that we may need big deal hardware versus the problems that oftentimes are not as much an emergency with regards to updating hardware?
PN: Yeah. We kind of think along two dimensions. One is, let’s call it, scale, the size of your problem. The other one is just complexity. If you’re looking at some of these workflows in drug discovery, like electron cryomicroscopy, cryo-EM, that is a very complex workflow. Even if somebody’s just starting out experimenting with it, it can still be a computational and an [inaudible 00:06:58] challenge. The scale question’s really interesting because we’ve spoken with a lot of companies where at a small scale, they don’t have enough data to even make deep learning worthwhile. It’s not even a question of whether or not you need it. It can’t demonstrate the benefit, but they can project forward in time as data sizes or data volumes grow, and at that point, they’ll be a crossover. I think it’s an important point, so even before you talk about the infrastructure, is that this machine learning isn’t for everybody just yet.
That’s kind of the first starting point is whether or not you can really see the benefit today. Like I said, a lot of companies we speak to, as they project forward, they absolutely do see this.
Yeah. I can imagine looking forward long enough, all of us are going to be upgrading hardware in some way. The computer that I’m on now is going to be a pretty different computer maybe five or 10 years from now. The same thing with phones. The same thing with all the computation I’m using in business. There’s an inevitable trajectory here. There’s different levels of urgency, as you’ve rightly pointed out.
(9:00) You brought up those two dimensions, complexity and scale. That might be a fun kind of thought “experiment way” of thinking through this for business folks. Is there a better way to think about it as a business leader?
PN: I think one of the other terms that we like to use is heterogeneity. When you look at something about deep learning, I think to date, deep learning has been kind of defined from a processor architecture perspective, but if you’re a data scientist, you’re really focused on the workflow. Even the workflow itself is very heterogeneous, if you will. There’s data preparation and model development, model implementation. AI practitioners will iterate between these steps across the entire workflow. Really, when you look at workflows, they are heterogeneous by nature, but we also see them becoming increasingly heterogeneous, blending data analytics, blending machine learning, simulation. It’s at that point where complexity goes up, heterogeneity goes up, and really, at the end of the day, what ends up happening is that it’s more than any single technology or product. That’s, again, just trying it back to infrastructure is that you really have to look across the various needs of your workflow and choose the right technology which is going to provide the greatest value for that particular portion.
We really see AI as kind of fundamentally a supercomputing problem, but there are certainly … There’s a range along that scale. I think that it’s kind of interesting too when you talk about complexity, I think the rate of change in technology as well is another dimension that’s [inaudible 00:12:04] behind here. A lot of the IT or data science practitioners that we speak to in the enterprise are overwhelmed with the rate of change as well. That’s not only coming from industry in terms of new technologies, but as they go through this journey as I described before, this arc, their problems change there too. What they discover at one point might be very different than they discover six months later. That landscape and how they address these problems, they really are overwhelmed.
(13:30) If you’re speaking with an executive team… where should they be focused if they want to not be completely bowled over by all the new press releases every minute about AI?
PN: That’s a great question. I think that the advice that we provide, and quite frankly, this is why people come to Cray, is that we’ve always taken a system view, focused on the workflow or the application performance. I think that really taking a step back and viewing it holistically is the first step. Don’t get rat holed down any one particular technology, but open up your aperture a little bit and view end to end. Then I think everybody’s journey’s a little bit different. Again, we’ll speak with two different companies that, on the outside, have exactly the same use case, and where they might be in their, let’s say, digital transformation really defines what the right solution is for them. I think that there is a personalized aspect to this as well, and that’s look for people who can look from a system perspective and look at it holistically for your particular organization.
(16:00) How do you ask people to think about scale? How do they get a sense of where they stand in terms of scale of data?
PN: Yeah. That’s a good question. I think a couple of views here. One is that regardless of the size of the organization, I think one of the trends that we see is just towards distributed training, for example. As any problem gets … All problems are getting larger, so it’s all relative to the particular organization. There is this push towards scaling your individual training runs, for example. There’s also a push towards things like distributed inference, let’s say. I think that that thread is common regardless of the size of the organization. Again, after that, it just right sized to whatever their problems might be.
I think computing today, especially supercomputing, fundamentally you have to think parallel. It’s all about running things in parallel. That’s true for anything that you’re doing, whether it’s, again, structural engineering or distributed deep learning training. It’s all about finding parallelism. That’s really how most people should think about the scale question because that’s the only way to really ultimately accelerate their workflows.
Subscribe to our AI in Industry Podcast with your favorite podcast service:
Header Image Credit: Nanalyze