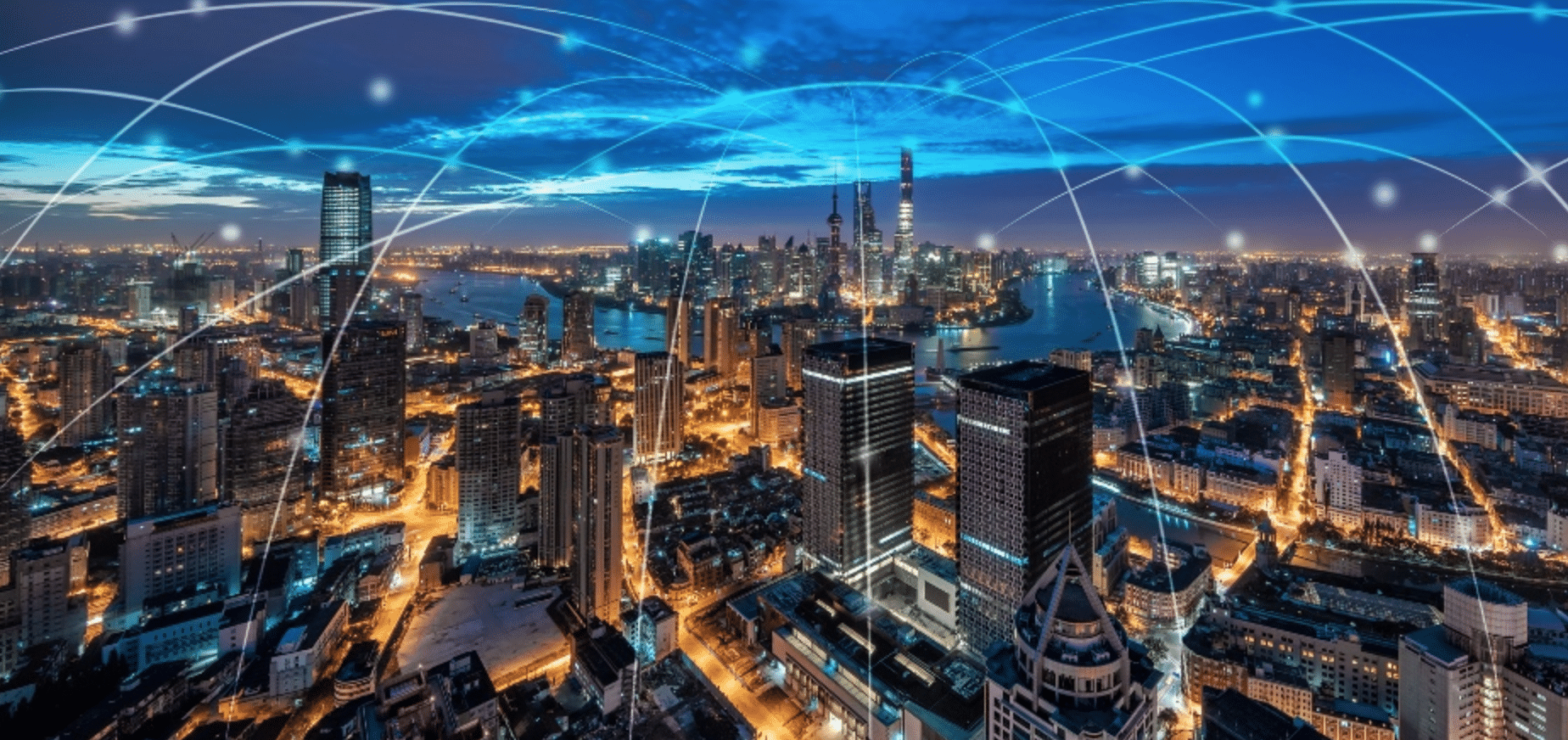
This week on AI in Industry, we explore IoT security with Bob Baxley (Chief Engineer at Bastille). This includes information on how different IoT security is compared to infosec, the unique challenges IoT security presents (for detecting and scanning wireless network traffic that runs on various protocols and for classifying types of cyberthreats), what the future of IoT security might look like, and how deep learning and machine learning tools can be used to better classify and detect threats and attacks in the cyberspace.
Guest: Dr. Bob Baxley, Chief Engineer at Bastille
Expertise: Wireless security, data science, machine learning, software defined radio
Brief recognition: Bob Baxley is a Chief Engineer at Bastille Networks and has been with the company since 2014. He received his doctorate degree in Electrical Engineering from Georgia Institute of Technology, where he served as Adjunct Faculty for over four years.
Big Idea:
One of the biggest challenges in the IoT network security is classifying the type of data to look for anomalies, which are significant deviations in the way data usually behaves over the network. For enterprises and businesses, this poses a huge threat because it means their data on an IoT device–that might be running on any of the several non-standardized wireless protocols–is not secure.
Bob walks us through how this problem is currently tackled, and how IoT security could be improved in the future using deep learning and machine learning applications, such as TensorFlow. Below are some of the important points Bob covers in this particular section:
- Machine-learning tools are already getting more accurate at classifying image categories with only a 3% error-rate as opposed to human error-rate of 5-10%. This is because they get trained on copious amounts of data and become better at identifying categories over time. In the near future, this capability could be extended to the cyberspace for classifying data threats.
- Currently, there is a significant lack of labeled data in the cyberspace from which the machine learning applications can learn. Therefore, identifying anomalies and monitoring cybersecurity involve hours of manual work and effort.
Bob explains how the learnings from image classification can be applied to cybersecurity and how machine learning tools can better identify anomalies in IoT network traffic that could constitute as threats.
Interview Highlights on IoT Security
The main questions Bob answered on IoT security are listed below. Listeners can use the embedded podcast player (at the top of this post) to jump ahead to sections they might be interested in:
- (2:00) How is IoT security different than infosec?
- (4:17) What are the unique challenges when it comes to IoT network security?
- (11:09) Future of IoT security: how can machine learning applications like TensorFlow help in improving cybersecurity?
- (24:44) Can machine learning applications reduce manual intervention in cyberspace in the near future?
Subscribe to our AI in Industry Podcast with your favorite podcast service:
Header image credit: engie.be