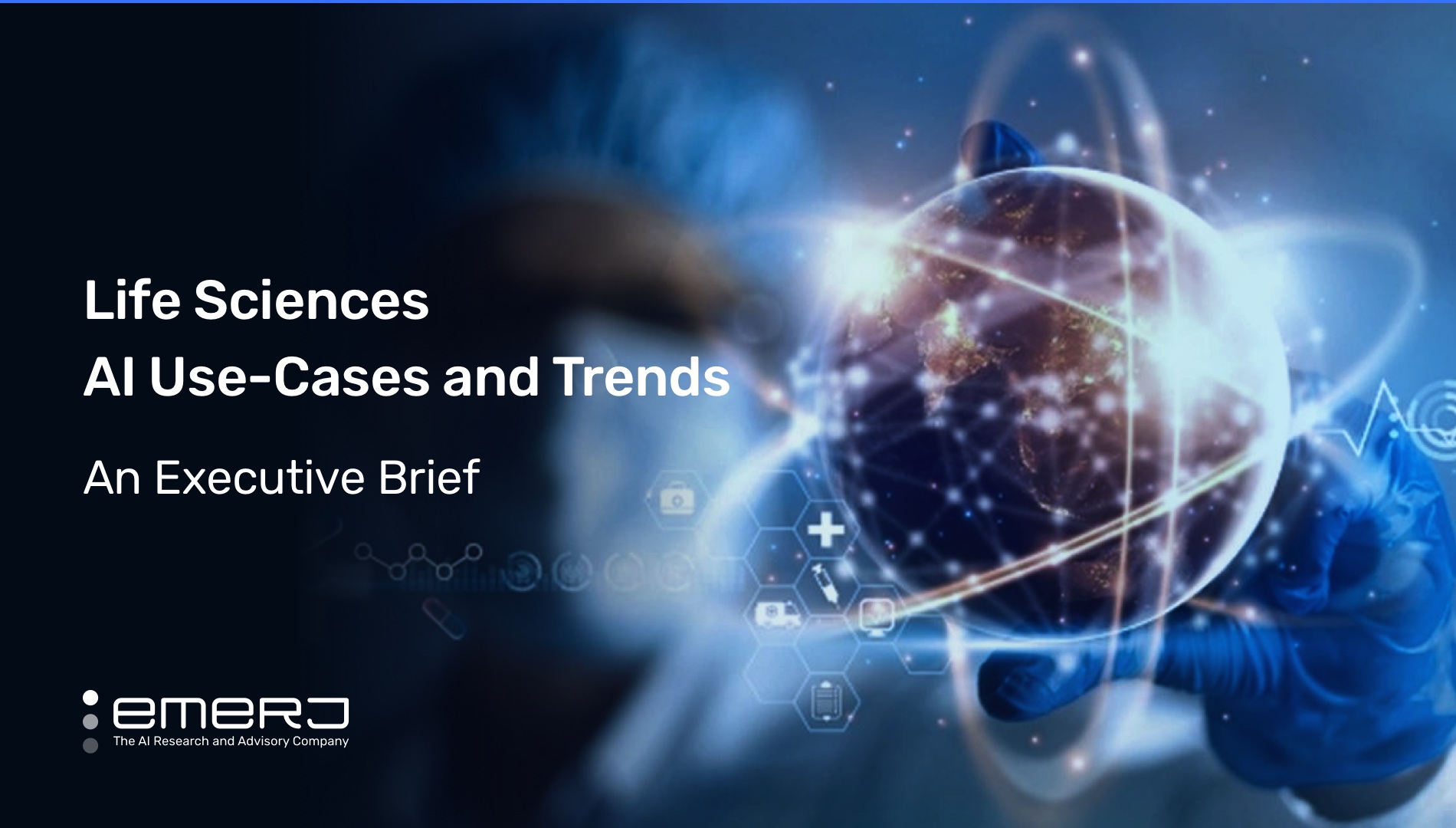
Like many other economic and industrial sectors, AI is beginning to transform operations across life sciences. In a 2021 survey of life science organizations published by the International Data Corporation (IDC), respondents cited a 65% average increase in AI spending in their organizations over the next year.
Life sciences is an enormous umbrella that covers numerous scientific disciplines. This article will present leaders with three overarching trends of AI adoption within the life sciences space, primarily focusing on for-profit companies in pharmaceuticals (pharma) and biomedicine:
- AI-driven drug R&D is taking off
- Data sharing is driving AI innovation
- Many firms could be gauging AI ROI poorly
We then will examine three use cases directly related to these trends:
- Drug research and development: Reducing the oncological drug development process by several years through reviewing drug performance and patient outcomes with natural language processing and predictive analytics.
- Automated diagnostics: Developing interactive apps using models trained in diagnostic data that lets patients perform their own screenings and answer essential questions on symptoms.
- Supply chain management: Reducing waste in manufacturing active pharmaceutical ingredients using deep learning techniques and transformer anomaly detection models to identify critical insights from temporal patterns in the production data.
AI Adoption Trends in Life Sciences
We’ll first look into these trends and their concomitant effects on life science investments in AI solutions.
Trend 1: AI-driven Drug Research and Development is Taking Off
Drug research and development (R&D) is perhaps a biopharmaceutical enterprise’s most common and promising use case. The reason is that the process is so arduous, expensive, and uncertain. According to a 2021 report by the Congressional Budget Office (CBO):
- In 2019, the pharmaceutical industry spent $83 billion on R&D, ten times the per-year amount in the 1980s.
- The cost of developing a new drug – including capital costs and expenditures on failed drugs – is estimated to be anywhere from less than $1 billion to more than $2 billion. (Independent, mostly non-profit sources such as the American Chemical Society tend to estimate costs on the lower end of this $1-2 billion scale, while research from within the industry may exaggerate expenses. For example, PhRMA, a big pharma trade association, estimates an average development cost of $2.6 billion.)
Companies are already investing big in end-to-end AI for R&D. Per a press release by the American Chemical Society (ACS), “… companies are committing to R&D-wide AI for support along the entire continuum of drug discovery, from identifying targets to designing drugs to analyzing clinical trials.”
The release lists companies that have invested heavily in related AI tech. Some of them have exceeded billions of dollars.
This “continuum of drug discovery” encompasses:
- Biology: target discovery and disease modeling
- Chemistry: retrosynthesis, small molecule generation, virtual screening
- Clinical development: clinical trial design, patient stratification, prediction of trial outcomes
Experts state that AI is not yet mature enough to serve as a fully functional, end-to-end solution. “Much AI has focused on chemistry, whereas biology is a far more complex, difficult-to-predict field,” the release reads. However, the end goal remains the same: use machine learning to analyze vast data stores and develop models capable of autonomous improvement.
Trend 2: Data Sharing is Driving AI Innovation
While drug and vaccine development is perhaps the most ubiquitous application of AI, it is far from the only one. There exist many potential applications of AI across the life sciences value chain, including:
- Clinical setup: e.g., designing the trial protocol, trial planning, trial setup, and management
- Manufacturing and supply chain: e.g., defect management, order and inventory management, packaging and labeling
- Marketing: e.g., market research, advertising and promotion, product lifecycle management
- Medical affairs (department tasked with communicating accurate information to clients): e.g., literature research, medical queries, regulatory filing, scientific documentation
- Research: e.g., developing novel compounds, molecule identification, and targeting, lab data management
Figure 1: AI Applications Across Life Sciences. (Source: InfoSys)
IDC estimates that computers will have stored approximately 270 gigabytes of healthcare and life sciences data on every person worldwide by 2020. This data trove drives many insights and innovations in healthcare and life sciences.
According to a Deloitte 2022 report, life science enterprises are sharing non-competitive, HIPAA-compliant data. Moreover, these enterprises are supposedly able to do so without concerns for data privacy. Deloitte states that this is possible through an application programming interface (API)-centered data strategy.
Enterprises anticipate the more open sharing of this data, particularly by younger digital natives, resulting in a valuable stream of actionable data points. The intended result is interoperable data sharing across the organization and in collaboration with partners, patients, payers, and providers.
According to a report [pdf] by the U.S. Department of Health and Human Services (DHHS), the sharing and utilization of copious health data has fueled the development of algorithms and machine learning and has accelerated the development of AI applications. The DHHS report cites six major health data types that can be used for AI development:
- Administrative and claims data
- Clinical data
- Clinical trials data
- EHR data
- Genomic data
- Patient-generated data
- IoT data
- Social media data
- Social determinants of health data (defined as “the conditions in the environments where people are born, live, learn, work, play, worship, and age that affect a wide range of health, functioning, and quality-of-life outcomes and risks.”
Trend 3: Many Firms Could Be Substandardly Gauging AI ROI
The IDC survey respondents report the three most popular AI uses in their life science firms as:
- Improving employee productivity
- Developing new products
- Improving risk management
However, aside from improved risk management, these uses are not those with the highest ROI. Per the survey respondents, the use cases delivering maximum returns were:
- Gaining competitive intelligence (i.e., market research)
- Improving customer experience (CX)
- Increasing margins
- Improving risk management
- Improving product quality
Secondary research appears to strengthen the argument for this trend. In a report published by Deloitte, leaders cited “difficulty identifying use cases with the greatest business value” as the top challenge to AI initiatives.
We must bear in mind the limitations of this assumption:
- First, the product cycle in life sciences is much slower than in other industries.
- Second, given the massive expenses typically incurred in product discovery and development, turning a profit on pharmaceuticals can take several years following FDA approval.
Therefore, respondents likely would not have seen any ROI regarding AI-augmented product development.
However, it is telling that there appear to be very few use cases related to competitive intelligence, customer experience, and improving product quality. Relative to the highly complex, highly costly AI infrastructure needed for product creation, an AI-enabled platform capable of producing actionable market insights appears both cost-efficient and capable of producing short-term returns. The same can be said for any AI-enabled software that enhances CX.
Three AI Use Cases in Life Sciences
Next, we will discuss three potential use cases within the life sciences sector. We begin with drug discovery, perhaps the industry’s most common AI use case. We follow this up with disease diagnosis before finishing up with automated supply chain management.
Our first use case examines the application of machine learning and natural language processing to help expedite cancer drug research.
Use Case #1: Expediting Drug Development
Cognizant was approached by a large pharmaceutical company that needed a new, more efficient process for reviewing and referencing information on drug performance and patient outcomes.
The two and a half minute long video below details how Cognizant approaches expediting clinical trial operations:
Before implementing Cognizant’s solution, the company had relied on a manual cross-referencing process. This process was apparently costly and time-consuming.
In the clinical trial phase of drug development, scientists must understand and predict how a specific patient’s body will react to their new compound. To accomplish this, they cross-reference vast internal and external data stores. This data includes:
- Patient profile data
- Novel compound data
- Medical literature data
The client company was conducting trials for acute myeloid leukemia (AML). It was amassing large data stores from its clinical trials, medical research, and data from the Cancer Cell Line Encyclopedia (CCLE).
The software appears to be trained with the client’s internal data stores and scientific and medical literature related to AML.
Cognizant states in the case study that its automated solution uses text mining to review online medical journals and scientific research publications. The software uses natural language processing (NLP) to convert unstructured online data into a normalized dataset for analysis.
In the case of this particular client, Cognizant states that its solution is designed to analyze data in clinical trials research during clinical trials. The company states that it used an Agile development model to build an automated data pipeline that intakes this extensive research data, standardizes and analyzes it, and constructs an outcomes report for the researcher.
Cognizant clearly states that the model’s output is a report, but it does not elaborate on its contents.
Regarding outcomes, Cognizant claims:
- The solution can scan over 10,000 online resources simultaneously.
- 97% faster drug outcomes review
- Up to a 4-year reduction in the 10-18 year oncological drug development process
- 8% to 10% cost savings per clinical trial patient
Use Case #2: Automated Diagnosis
Ada is a Berlin-based company that produces an AI-enabled diagnostic application.
The company offers an app of the same name, which they claim can help healthcare providers improve diagnosis accuracy using what appears to be machine learning. Enterprise users, such as clinics, contact the company to inquire about incorporating the platform into existing screening systems.
Individual users can download the app via Google Play or the App Store. The user interaction is as follows:
- The application is downloaded for free on Google Play or the App Store.
- After creating a profile, the user takes a “symptom assessment.”
- The user types in the most prevalent symptom; as the user types, the software auto-populates a list of suggested symptoms, along with a short description.
- The user is asked how to enter symptom duration and given five choices, ranging from “less than one day” to “more than one year.”
- If the software algorithms detect a potential match at this point, the user is provided with the following data:
- Summary: A paragraph describing the next best course of action, e.g., seek advice from a doctor or visit the emergency room.
- Possible causes: A list of one or more potential underlying ailment(s) causing the symptoms and the likelihood (in the form of “# out of # people with these symptoms had this condition.”)
- “Tell me more”: Additional information on possible causes.
- A longer description of the possible cause
- A graphical representation of “# out of # people” and a list of additional symptoms
- “Tell me more”: Additional information on possible causes.
- Less likely causes: A list of one or more unlikely potential underlying ailment(s) in a similar “# out of # people” format.
The model is trained by an in-house group of general practitioners and specialists using thousands of medical conditions and many symptoms. The model includes a knowledge base of risk factors and a reasoning engine, which “asks” [1] What possible known ailments may be responsible for the user’s symptoms, and [2] What are the best questions to ask to narrow these ailments down to a probable cause?
The user functionality of the app is on display in the following 40 second video:
An algorithm calculates possible conditions’ likelihood and presents the users with this data. It also provides the user with information about actions to take; for example, scheduling an appointment with a primary care physician or going to the emergency room.
Regarding outcomes, Ada states that while the app is free, the company earns revenue through partnerships with health providers, who integrate the platform into their screening systems. However, as the company is private, it is not obligated to release financial statements.
However, we can get an idea of the company’s – and the app’s – growth by looking at recent funding. In May 2021, the company raised $90 million in seed funding. In a February 2022 Series B round, the company raised an additional $30 million to bring its total capital raise to $120 million within nine months.
The company says:
- Its app is the #1 medical app in 130 countries
- 26 million symptom assessments have been completed
- 99% of known medical conditions covered
- 35% more accurate than other symptom checkers
Use Case #3: Automating Supply Chains
Nexocode is an international AI software development company based in Poland. The company was approached by a large manufacturer of active pharmaceutical ingredients, volatile substances responsible for medicines’ beneficial health effects.
According to Nexocode, this manufacturer struggled with the efficiency of its repeatable batch production processes. Over 50% of production went to waste due to quality deterioration. The challenge was identifying critical insights from temporal patterns in the production data. To solve the problem, Nexocode says that it needed to find which parts of the time series data could explain the malfunction. The lack of data on post-batch production complicated the issue.
Nexocode case study documentation states that it trained the solution using data from the production line. The model eventually analyzed new data in real-time, identifying potential outliers using anomaly detection.
The company claims to use deep learning techniques, such as recurrent and convolutional neural networks (RNNs and CNNs, respectively) and transformer models in its anomaly detection model.
The company also applied predictive analytics to track product batches as they move through the manufacturing process. In this way, issues can be resolved in real-time, minimizing production disruptions when possible.
The workflow appears to be as follows:
- Data is gathered and aggregated from the production line and then stored.
- A time-series analysis is conducted on the imported data
- Algorithms and predictive analytics are applied to the data
- Alerts and adjustments are made on the current batch, with recommendations for the next production cycle.
The goal of the model was to detect outliers that could lead to quality deterioration. Nexocode states that the model identified critical processes that accounted for the decay in quality.
Nexcode solution workflow (Source: Nexocode)
Regarding outcomes, the company gives only generalizations. Nexocode states that its solution led to “improved efficiency, predictability, and quality assurance of manufacturing operations and yields.”