The following statements are all true, but not in the way you might think:
- AI is hard for most companies to adopt.
- Machine learning talent is hard to find and hard to hire.
- AI gives some companies new ways to compete in the market.
AI adoption is harder in some sectors than others. Strategies for AI talent recruiting and training changes across different sectors. The way companies compete using AI today is drastically different from how they will compete in the future – and as I recently explained at a United Nations event in Shanghai – the same goes for countries.
AI today is in its first “phase,” a phase that will change quickly in some industries and more slowly in others, but the shifting of those “phases” will have a dramatic effect on the strategy, adoption, and AI-related talent in companies around the world.
I decided to create this article series (which I’ll be calling “The AI Zeitgeist”) in order to explain to executives the phases of AI’s use in their enterprise or organization and to give leaders a roadmap for their own AI strategy.
While I recommend reading the AI Zeitgeist series in order, you can simply click whichever topics seems most pertinent for your needs.
The series has a total of 7 short chapters:
- The 3 Phases of AI in the Enterprise: Emergence, Adoption, and Dispersion (AI Zeitgeist 1) <— You are here
- How “AI” Will be Discussed in the Future (AI Zeitgeist 2)
- The Evolution of AI Talent and Training (AI Zeitgeist 3)
- The Increased Accessibility of AI in Business (AI Zeitgeist 4)
- Buying and Adoption Readiness for AI (AI Zeitgeist 5)
- The Changing Landscape of AI Priorities of Business Leaders (AI Zeitgeist 6)
- The Competitive Dynamics of AI – Now and in the Future (AI Zeitgeist 7)
In each of the segments of this Zeitgeist series, we’ll discuss how AI will change over the coming decade, beginning with a definition of the three main phases that artificial intelligence will go through (Emergence, Adoption, Dispersion).
Introduction to the “AI Zeitgeist”
“Zeitgeist” is a concept from German philosophy, translated roughly as “spirit of the times.”
Business and government leaders often think about their future AI plans as if the “Zeitgeist” will stand still. Many leaders think about AI talent acquisition, data requirements, and AI integration/adoption as if they will be the same in five years, or even ten years. Nothing could be farther from the truth.
AI is simply in its early phase of the “Zeitgeist” (Phase 1: Emergence), on the way to becoming a ubiquitous, ever-present part of almost all the technology we use at work (Phase 3: Dispersion).
The phases might be visualized as a set of expanding circles, where AI applications go from being a rough hypothesis to a more encompassing swath of functionalities and capabilities over time:
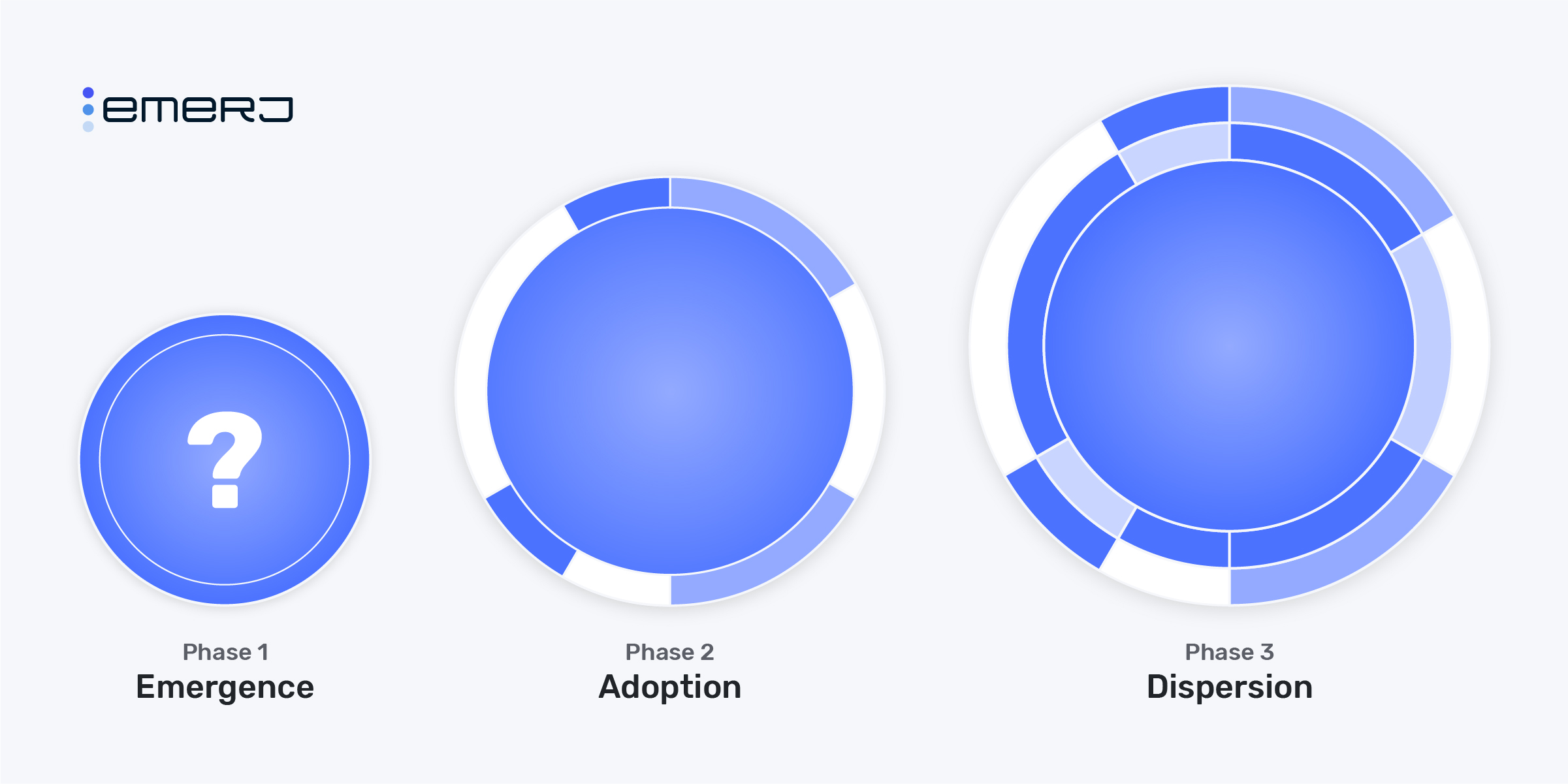
Over time, different sectors and different industries will move through these three phases at different speeds. Some sectors (eCommerce, online media) will likely move faster than others (Manufacturing, Utilities). Some countries (USA, China, UK) will likely move faster than others (India, Brazil). Today, nearly all sectors are in the Emergence phase – but their progress might be represented by the following graph:
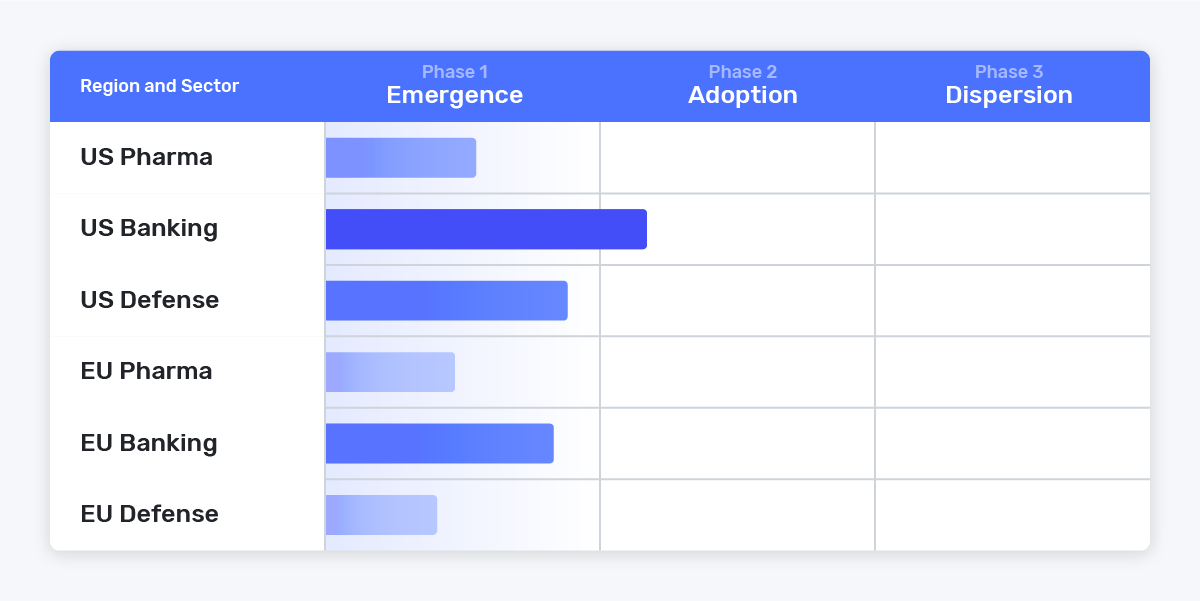
By the end of this article series, you’ll have a better understanding of where AI is headed and what it might mean for your organization. Companies considering a long-term AI strategy (or long-term competitive strategy) should consider these dynamics seriously.
In the coming decade, AI will become more accessible to non-technical teams, certain kinds of AI talent will become more plentiful, and the way that companies use AI to compete in the marketplace will change radically. This Zeitgeist series was written to help leaders think through that transition.
First, let’s lay out the definition of each of the three major phases of AI in the enterprise, beginning with phase 1:
Phase 1: Emergence
Defining qualities of an industry in the “Emergence” phase:
- “Artificial intelligence” is a buzzword that becomes prominent and seems important (even if hardly anyone can define artificial intelligence)!
- Very little adoption happens with AI outside of some lead-edge companies with huge sums of money and/or tremendous AI talent
- Most use-cases are experimental, and most vendor companies pay their bills with VC money, not with real revenue from clients
- AI-related events, training, and thought leadership flourish, largely focused on future potential, not on realistic current applications
During the phase of Emergence, AI is getting onto the radar, but not into the business. Since 2015 I’ve said that “The real money in artificial intelligence today is in selling AI-related event tickets.”
This is a joke, and something I admit to being an exaggeration, but it points to a truth of the Emergence phase. In most sectors, I can safely say that “AI companies, “even ones who have raised $40MM or more, are usually still unsure of exactly what they’re selling and to whom. There are plenty of pilot programs, plenty of vendors convincing large firms to do trials or a new service or technology, but there isn’t much robust evidence of clear and powerful use-cases in most sectors.
Certainly, there are exceptions, and some AI capabilities have a clear business case today. However, the vast majority of AI applications have no case studies or robust evidence behind them, and the future of these sectors is still up for grabs.
Due in part to this uncertainty around ROI, in part to the lack of AI technical talent, and in part to the lack of AI conceptual understanding from non-technical leadership, we find that there is a much more general curiosity in AI as a topic than there is actual effort to procure AI solutions. Business leaders, even in large enterprises, spend many thousands on event tickets before they deign to spend millions on AI vendor companies.
While it’s often presumed that our Emerj Advertising work involves lead generation for B2B AI companies (this is indeed usually the case), we have had months in the last two years where the majority of our advertising revenue came from events, and not companies offering actual AI solutions.
If you’re a long-time reader, you’ve seen banners for O’Reilly Media, IQPC, and other prominent event firms on the site, and it’s true that basically all AI events with ticket prices over $1,000 eventually email us to ask about advertising.
For the time being, the money is in curiosity, not in commitments—events, not vendors. I suspect that as AI shifts more into the “Adoption” phase, we’ll see the percentage of our advertising revenue from event companies go lower and lower with time.
Phase 2: Adoption
Defining qualities of an industry in the “Adoption” phase:
- “Artificial intelligence” is no longer a selling point in-and-of itself, healthy skepticism around AI hype persists
- AI adoption occurs not just with small pilots programs at the largest companies, but across many of the major players in the industry
- Many AI use-cases are still experimental, but many are fleshed out and useful, with strong ROI evidence and clear traction in industry
- Business leaders are no longer just curiously asking, “What can AI do?”, they’re also asking, “What is working, and which solution is best?”
Reaching the adoption phase in an industry is a challenging chicken-and-egg problem. In order to get enterprises to adopt artificial intelligence, we need case studies and some proof of ROI. In order to get proof of ROI, we need business to adopt the technology.
While we saw this dynamic clearly in our machine learning in healthcare consensus, the challenge isn’t limited to one industry.
This chicken-and-egg problem creates a consistent dynamic within major sectors:
- AI vendor companies have to convince enterprises to try their experimental solution, often with short trial programs or pilot programs
- Only relatively large enterprises have the budget, data science talent, and data volumes to do these pilots; SMBs are unable to participate
- AI vendor companies slowly begin gaining traction with their pilots and figuring our their core value proposition and business processes
- Gradually, evidence of ROI peeks through with a variety of AI applications in a given sector, and more companies feel comfortable adopting AI
Business leaders often make the assumption that the AI solutions being offered by vendors are already constructed, and the process to integrate and gain value from these solutions is already determined. Nothing could be farther from the truth.
Companies often raise tens of millions of dollars without robust proof that their application will be useful for clients, or that their method of integrating and setting up the solution with clients will be scalable or even viable. These hard lessons are learned in the real world, and we often see companies with large sums of venture capital money change their product description or set of product offerings every 4-6 months. It’s all an experiment.
Getting over this experimental phase is key to bringing a sector to the “Adoption” phase. In this phase, we’ll see a set of companies emerge who are able to fully fund their multimillion-dollar operations with just revenue. Revenue is a proxy for the actual value being generated in the world.
While venture capital is often raised to help scale an existing solution, the initial 2-3 rounds of capital are often provided before there is any “sure thing” to scale. For example, the funds are raised to aid in experimentation, to iterate and find a useful, scalable product.
Phase 3: Dispersion
Defining qualities of an industry in the “Dispersion” phase:
- “Artificial intelligence” isn’t spoken about overtly, but is rather assumed to probably be part of any mature technical solution
- “AI adoption” is an antiquated term, as AI becomes a component part of all things “tech”
- A broad set of new capabilities are opened up by AI, widening the use and enhancing the value of technical solutions immensely
- Business leaders no longer think about “AI”, they think about the widening capability-space of technology, of which AI is one part
As a sector reaches “Dispersion” phase, artificial intelligence will be seen as a normal part of any business, or of any robust technology solution.
Back in 1996, an “internet company” was unique. Few people used the internet, few people understood the internet, and it wasn’t a part of day-to-day business life. Today, the internet is taken for granted. People within a company understand what the internet is, what the internet allows them to do, and they’re reasonably familiar with many internet tools. In fact, many businesspeople in all technologically mature sectors couldn’t imagine a business world without the internet.
In some ways, artificial intelligence will be the same. By the time a sector hits the “Dispersion” phase, employees throughout the company from marketing managers to executive assistants don’t need to know how to “do AI;” they simply use the latest tech tools, and they take for granted that these tools use artificial intelligence in order to deliver value to users—the same way many apps use the internet today.
Here’s a potential snapshot of how AI will become “normal” within a sector in the “Dispersion” phase:
- CRM software might automatically scrape the web (or even send emails to people) in order to gather complete data on incomplete prospect records
- Company email systems would automatically be capable of replying to routine emails, even initiating simple tasks (sending a package, booking a meeting, finding travel accommodations) all autonomously
- Software would automatically troubleshoot a user’s issues, detecting their frustrations and prompting them with preemptive help when the user seems lost
In the “Dispersion” phase, everyone will be using artificial intelligence, but it will just be “technology.” It won’t be unique, special, or particularly scary.
What will set artificial intelligence apart from other technology norm transitions (such as the desktop computer, or the internet) is that artificial intelligence enables such a broad set of ever-evolving new capabilities.
Someone could argue that desktop computers have evolved, too, into laptops, smartphones, tablets, and with more and more features and capabilities. Someone could argue that the internet has done the same, evolving from old bulletin sites, to search engines, to social media, to video sharing, and beyond.
We believe that artificial intelligence will be fundamentally different than these previously dispersed technology shifts. The fundamental difference lies in the ability for artificial intelligence not merely to enable new actions or capabilities, but to automate tasks and make decisions at massive scale.
At Emerj, we view artificial intelligence as a “capability-space,” with new capabilities constantly emerging and older capabilities moving through phases of being “possible” (no ROI, but hypothetically useful) to being definitely “useful” (clear and present current ROI).
Most of our Research Services work with companies and governments involves mapping out the “capability-space” of artificial intelligence across important business sectors (life science, insurance, etc) and functions (customer service, process automation, etc) in charts and graphs like those you see below:
Leaders in the “Dispersion” phase won’t care about “AI,” they’ll care about the new capabilities that AI opens up and the traction/proven ROI that these new technologies develop.
We don’t see ourselves as a company focused on AI—at all. We see ourselves as a company focused on the expanding capability-space of technology and how government and business leaders should use these capabilities to reach their goals.
Bonus Phase 4: Singularity
Defining qualities of an industry in the “Singularity” phase:
- The most powerful first world governments and largest tech companies are fighting for control of the computational substrate that houses the most powerful AI (read: Substrate Monopoly)
- Urban human beings spend over 50% of their time living, working, and relating in digital environments (read: Programmatically Generated Everything)
- The most pressing political issues are almost exclusively about the creation of post-human intelligence and managing the control over its powers (read: Political Singularity)
- Super-intelligent, super-capable machines gain the ability to construct their own values to support their own decisions, and humans run the risk of being eviscerated (read: Moral Singularity)
Nah, just kidding.
We might all be killed or eventually dissolved by artificial general intelligence, but we can worry about that another day.
The rest of this series will be focused squarely on the business applications of AI, I promise.
Timeline Considerations
The speed at which different sectors in different countries are progressing through the three AI Zeitgeist phases is hard to predict.
In the United States, the eCommerce sector might reach “Dispersion” within 8-10 years, while the US manufacturing sector might not get to “Dispersion” until 20 years from now.
Sectors vary by region, too. Banks in India aren’t going to adopt AI in the same way – or at the same speed – as the largest US banks. There are a number of regional and sector-specific factors that will impact the speed at which AI moves through “phases”, including the following:
- AI talent density and talent attraction – Cities with computer science-oriented universities, existing AI talent, and fast-growing venture-backed companies are more likely to build a “virtuous cycle” of adoption than sectors without those factors. This gives a place like San Francisco an edge over a place like Bangalore, even if Bangalore eventually eclipses SF in raw number of engineers.
- Sectors of focus – New tech sectors like eCommerce and online media are bound to not only have younger, more tech-savvy employees, they’re also likely to have access to (and understand the value of) data. Gathering minute-to-minute data about an oil rig isn’t nearly as easy as doing so for a website or online platform. Regions with more tech-focused businesses and younger workforces are likely to run through the phases more quickly.
- Labor costs (high cost = high automation incentive) – Where there is tech talent and AI engineering skill, there is probably high labor costs. Eastern Europe is doing it’s best to be a middle ground, but the talent density for machine learning-related skills still isn’t robust combared to major US tech cities. We can expect the developing world to have less initial incentive to adopt AI aggressively. For example, India may simply accept its position as a low-cost service provider, instead of turning its IT services sector into an engine to build scalable, valuable AI products (for more detail on our assessment of India, see our full AI in India ecosystem report).
These were just a few of the major factors influencing adoption speed – there’s likely to be many more.
A Note on Why I Chose Three AI Zeitgeist Phases
Any definitions of technology adoption are arbitrary.
There is probably some reasonably useful way to break down AI’s adoption phases into four phases, five phases, or ten phases. The decision to break the process down into three phases came from two considerations:
- First, my independent conversations with AI PhDs (including my technical advisor, whose opinion I value greatly) seemed to break down roughly into three broad sets of phases: AI being totally new and novel, AI gaining traction, and AI becoming a nearly required part of any tech solution. Congealing each of these into simple definitions made things easy.
- Second, three phases seemed more reasonable to understand and explain than breaking the process down into vastly more phases. Because the lines between the “phases” are somewhat grey as it is, adding additional phases and more “grey lines” seems disingenuous.
All in all, an understanding of this transition in three phases is what seemed best to explain what we wanted to communicate in this AI Zeitgeist series, and I hope they’ll serve as useful references for you as well.
What’s Next
This has been week 1 of the “AI Zeitgeist” article series.
In the coming weeks, we’ll be exploring the following Zeitgeist topics, in order:
January 7th – The 3 Phases of AI in the Enterprise: Emergence, Adoption, and Dispersion (AI Zeitgeist 1) <— You are here.
January 14th – How “AI” Will be Discussed in the Future (AI Zeitgeist 2)
January 21st – The Evolution of AI Talent and Training (AI Zeitgeist 3)
January 28th – The Increased Accessibility of AI in Business (AI Zeitgeist 4)
February 4th – Buying and Adoption Readiness for AI (AI Zeitgeist 5)
February 11th – The Changing Landscape of AI Priorities of Business Leaders (AI Zeitgeist 6)
February 18th – The Competitive Dynamics of AI – Now and in the Future (AI Zeitgeist 7)
Next week we’ll explore how AI will be discussed in the future and when executives will even need to refer to “artificial intelligence” and “machine learning” (good news: These terms won’t be fodder for hype forever).