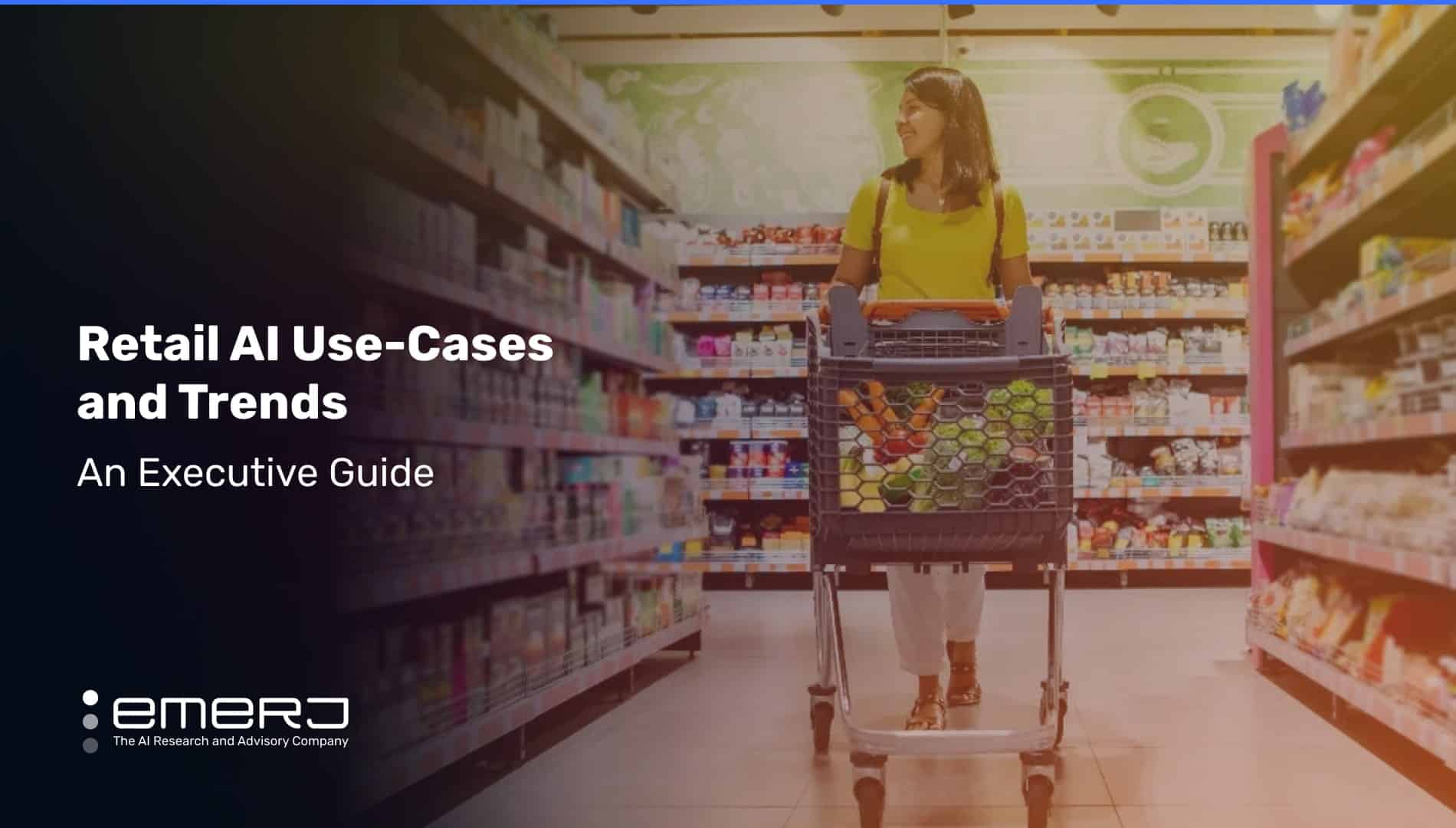
Retail has been undergoing a digital transformation for some time. Most of us have seen the gradual macroeconomic effects of this change, from the increasingly empty malls to the shuttering of once-prosperous brick-and-mortar stores.
To adapt to these changes, retailers seek leverage AI to deliver maximum customer value, forecast demand, and optimize pricing and efficiency. And there’s business value to be had from these use cases. For example, price optimization alone may pad total revenues by an additional 1-5 percent while extending the customer lifecycle by up to 20%.
Realizing these benefits requires that leaders understand the current trends for AI in retail, the value-adding use cases and the challenges of AI adoption.
We created this resource based on several interviews with retail leaders within leading enterprises, along with secondary research. This article aims to help retail leaders navigate the industry’s most influential trends, use cases and factors that lead to successful adoption.
Three overarching trends in AI in retail we will discuss in the article include:
- Hyper-personalization is the new norm
- Rising demand for AI chatbots and conversational AI
- AI-enabled supply chain fixes become a pressing need
Three consequent use cases for AI in retail include:
- Curated digital marketplaces
- Automated customer service
- Inventory management and demand forecasting
AI Adoption Trends in Retail
We begin by looking at these trends and their attendant effects on retail investments in AI solutions.
AI in retail is a large market. Per an article published by Yahoo, the value of AI in retail is expected to hit nearly $41 billion by 2030 — up from just $4.8 billion in 2021. A few significant drivers of this market growth include:
- The growing use of chatbots and voice recognition technologies
- The proliferation of AI-driven image and video analytics
- Emerging online sales (e-retailers tend to be prolific AI users)
- Advanced machine learning methods capable of analyzing vast data stores
- Boost in natural language processing (NLP) use cases (e.g., sentiment analysis)
Driving most, if not all, of the above is the desire to hyper-personalize the customer experience.
Trend #1: Hyper-Personalization is The New Norm
Deloitte defines hyper-personalization as “the most advanced way brands can tailor their marketing to individual customers,” adding, “through hyper-personalization, companies can send highly contextualized communications to specific customers at the right place and time, and through the right channel.”
Hyper-personalization goes beyond traditional market segmentation. The former drills the data down to the minutiae of customer data, leading to tailored, predictive, and proactive marketing and sales strategies. Customer engagement is the name of the game. An effective hyper-personalization strategy can:
- Instantly identify customers
- Immediately access said customers’ data about every interaction and on every channel.
- Understand the customer intent or issue and how to solve it based on said data.
Accomplishing this requires a proactive and comprehensive data strategy. Enterprises’ increased access to more openly-shared customer data, primarily via online channels, coupled with a surge in digital engagement across demographics, are supplying this data. Enhanced computing power, machine learning capabilities, and cloud-based solutions convert these data into actionable customer insights.
Organizations must scale to develop highly personalized solutions (see Figure 1 below). According to the Personalization Maturity Curve, the more mature the personalization infrastructure, the more significant the revenue increase. The “Personalization Maturity Curve” begins with individual messaging campaigns and tops out at predictive personalization, as is the case for Amazon and Netflix.
Figure 1: Personalization Maturity Curve. (Source: Deloitte)
As a result of the insights gleaned, companies can promote their brand to the individual level, providing customers with relevant offers with a high probability of conversion.
Retailers should not see a personalization strategy as a “nice to have” but a must. According to a McKinsey report:
- 71% of customers expect companies to deliver personalized interactions, and 76% of customers experience frustration when this interaction doesn’t happen.
- Faster-growing companies drive 40% more revenue from personalization than their counterparts.
- Businesses in the top quartile of personalization across industries generate over $1 trillion in value annually.
- Depending on the execution, personalization revenue spans 5-25% across companies and sectors.
Trend #2: Rising Demand for AI Chatbots and Conversational AI
In a 2021 survey by KPMG, retail business leaders named chatbots for customer service as the area with the third-biggest impact from AI. Customer intelligence and inventory management were first and second; we’ve already touched on the former and will elaborate on the latter momentarily.
Like other industries, chatbot use cases in retail are vast and diverse. These use cases typically involve streamlining workflows, automating processes, or assisting customers.
Customer-facing chatbots are perhaps the most common, as they can answer customer queries sans human intervention. They’re an easy way to automate part of the customer journey and raise customer satisfaction and experience.
AI-driven chatbots, in particular, are finding a home in retail. These intelligent chatbots can answer more nuanced queries and deliver more distinct responses.
Some of the potential uses for AI-enabled chatbots in retail include:
- Inventory management
- Online and in-store support
- Targeted promotions
- Updated product recommendations
- More accessible customer service
- Reduce retail staff workload
Trend #3: The Pressing Need for AI-enabled Supply Chain Fixes
The pandemic demonstrated the woeful inadequacy of many supply chains. As a result of the supply chain shocks, retailers were forced to spend tens of billions of dollars to return to pre-pandemic inventory levels. According to research by McKinsey, unaddressed supply chain disruptions can decrease enterprise EBITDA by 15-20%.
Perhaps the most pressing need is shortening delivery times, mainly the e-commerce “click-to-customer” cycle. The McKinsey report states, “…when delivery times are too long, almost half of omnichannel consumers will shop elsewhere.” However, only one in five US consumers will pay for faster shipping.
In other words, the onus falls on the retailer to ensure fast and efficient product delivery. Unfortunately, many retailers still rely on disparate, legacy supply chain methods.
In a Reuters survey of 400 supply chain executives, the report lists the top four shortcomings of current supply chain systems:
1. The lack of integration between the supply chain and other systems
2. The lack of automation planning and optimization
3. The inability to incorporate all necessary supply rules
4. The inability to test multiple supply/demand scenarios
A common thread runs through these shortcomings: they are all potentially solvable with an AI- and machine learning-enabled supply chain solution.
Three AI Use Cases in Retail
Next, we will discuss three concomitant AI use cases relative to the abovementioned trends.
Use Case #1: Curated Digital Marketplaces
Vue.ai is an end-to-end software company based in Redwood, California. The company’s flagship product is a retail automation platform. Per the company’s website, “Vue.ai allows every retailer to customize AI personalization models to work best for their business.” According to the company, the platform:
- Builds nuanced style profiles for every shopper
- Maps shopper site actions to “rich product data.” (Data is not defined)
- Recommends products the shopper is most likely to purchase
Purple Style Labs (PSL) is a luxury fashion retailer based in Mumbai, India. The company has both an online and offline presence. According to Vue, PSL is one of India’s largest luxury fashion houses.
PSL wanted to improve customer engagement and conversion rates. Vue proposed that the company integrates its retail automation platform into a digital marketplace that features curated fashion items. According to Vue, one of the goals for this new model was “to find new use cases for recommendation strategies across the shopper journey based on insights derived by putting data front and center.”
Vue.ai appears to have implemented one or more of the following solutions across the home page and six subpages (see Figure 1):
- ‘Similar products’
- ‘Brand-based similar products’
- ‘Complete the look‘ (Recommends different ways the source product can be matched and worn with other products in the catalog.)
- ‘Recently viewed’
- ‘Recommended for you’
- ‘Trending products’
Figure 1: Vue.ai implementations in PSL’s pop-up shop. (Source: Vue.ai)
Vue states in its case study that the company first started by gathering and analyzing shopper data to understand shopper trends on the site better. The company then used this data to suggest which solutions to implement. Per the personalization engine solution page and video, the model uses and produces the following input and output data (see Figure 2):
- ‘Product intelligence’
- Input data
- Product image tagging and categorization
- Product metadata (e.g., brand, discount, price, size, etcetera.)
- Output data
- Product tags
- Product associations (i.e., recommended products)
- Input data
- ‘Customer intelligence’
- Input data
- Shopper preferences (historic activity)
- Attribute preferences
- Long-term buying behavior
- Affinities (price, brand, company)
- Customer intent (current session activity)
- Output data
- Targeted product recommendations
- Shopper preferences (historic activity)
- Input data
- ‘Customizable AI’
- Change algorithm weightages
- Add/remove filters based on business goals and inventory levels
Figure 2: Personalization engine elements. (Source: Vue.ai)
Below is a short 3-minute video demonstrating how Vue.ai works:
https://www.youtube.com/watch?v=v-fsHs9UJqk
Vue.ai reports the following business outcomes for PSL (in % of website visitors):
- 44% viewed a Vue.ai-generated recommendation
- 17% clicked on a recommendation
- 1% converted to a sale
Vue.ai reports the following revenue contributions (in % of total site revenue):
- ‘Direct attribution’: The user clicks on a product and purchases the same product within seven days – 21.3%
- ‘Assisted attribution’: The user clicks on a recommendation and buys the product in the same session – 31.8%
Use Case #2: Automated Customer Service
Ada is a Canadian software company that offers a chatbot-based platform to retailers. The company claims its app is the #1 AI chatbot in retail. Ada has designed solutions for several marquee companies, including Shopify, Facebook, Zoom, and Square.
Ada offers an AI-powered chatbot, which the company claims helps retailers anticipate customer interactions using machine learning, natural language processing (NLP) and natural language understanding (NLU).
Ada lists the following retail use cases for its solution:
- Order tracking
- Troubleshooting
- Lead capture
- Product recommendations
- Sales handoff
- Account details
- Conversions, promotions, and referrals
Raise Marketplace is a mobile payment platform that helps consumers earn instant cashback and save money with sales and coupons from over 4,000 brands. The company faced delays in its email and phone customer service interactions and needed to adapt to meet customer demands.
According to Ada’s case study report on the use cases, the company’s turnaround time for email communications was 12-24 hours, which was considered too lengthy. The company wanted an AI chatbot to implement its FAQ content and deliver it in a way that did not disrupt the customers’ typical flow of using the e-commerce site.
Regarding inputs, Ada claims in its promotional literature that its platform is “system-agnostic” and capable of integration with over 100-plus platforms via:
- Handoff integrations (transfer workflow to live chat and ticketing apps, e.g., Salesforce)
- App integrations
- API integrations
Ada’s platform uses a drag-and-drop interface to build conversational flows and is no code. The workflow to build out a simple conversation on the platform looks like this:
1. Ada prompts the user to type a question received by customer service, e.g., “What is my order status?”
2. The software recommends additional training by adding 7-10 different ways a person may phrase a particular question, e.g., “What is my order status?”, “Where is my order?”, “Where’s my order?”, “Why is my order late?” etc.
3. Ada suggests pre-programmed training questions to help improve the platform’s accuracy. The user clicks the “Add” button to select additional training questions.
4. The user is prompted with an option to add “List options,” a multiple-choice answer format (see Figure 1), instead of the traditional open-ended form.
5. The platform also offers scripted answers. For example, when training an order status conversion, the platform provides the following script: “Hello <First Name>! Order no <Order-Number> has <Order-status>.”
6. After additional platform-driven recommendations, including tweaking, troubleshooting, and testing, the user is prompted to save the conversation. It is then ready to go live.
Figure 1: Screenshot of Raise’s AI Bot. (Source: Ada.cx)
Raise Marketplace states that they are using Ada on their website and web app, which is the first customer contact point.
Ada reports the following business results achieved:
- An 85% containment rate (the percentage of interactions solved by the bot sans human intervention)
- $133,000+ savings in six months, resulting from 22,000 bot-contained customer cases
- 45% decrease in support phone calls
Use Case #3: Inventory Management and Demand Forecasting
Retalon is a Canadian software company specializing in AI-enabled solutions for the retail industry. The company offers an end-to-end inventory management platform that Retalon claims can help retailers uncover lost sales, reduce inventories, and increase revenue using machine learning and predictive analytics.
In its platforms’ promotional literature, the company lists some of its marquee clients, which include Columbia Sportswear Company, Moss Brothers (UK), Gamestop, Hard Rock Cafe, Laura (Canada), and Sandals Resorts (Jamaica).
Simons is a fashion retailer headquartered in Quebec City, Quebec. The retailer has been offering Canadian fashion products since 1840 and is Canada’s longest-running private, family-owned business. Besides manufacturing men’s and women’s clothing and accessories, the company also produces its own home decor line.
In a customer story video, Gary Craig, VP of Information Technology at Simons, states that the enterprise required a better fulfillment solution to manage store-destined product distribution. Moreover, the company faced difficulties aligning its “fashion boutique customer experience and [product] assortment” business model with its inventory management approach.
Challenges apparently faced by Retalon in implementing the solution included:
- Accurately forecasting demand spikes
- Automatically adjusting shipment quantities based on calculated spikes
- Adjusting for demand changes due to promotions
- Accounting and adjusting for product displacement due to promotions and cross-promotion
- Knowing when to start and end a promotion
The solution integrates with the client’s ERP or OMS to provide the input data. Either company did not offer specific input data, but at minimum, it likely consisted of store-level:
- Product metadata
- Historic order data
- Promotional data
- Other inventory data
The inventory management platform is supposedly comprised of six functions:
1. Purchasing and order management: The demand forecast model generates optimal suggested quantities
2. Auto-replenishment: Demand forecast determines shipment quantities and allocates said products to respective locations
3. Smart allocation: The model performs allocation optimization based on the following:
- Location
- SKU performance
- Existing terminal stock
- Assortment distribution
- “Other business-specific factors” (not defined)
4. Inter-store inventory balancing: Predictive analytics engine analyzes “every … influencing factor” of the retail supply chain and recommends optimal inter-store product transfers. Per Retalon, these influencing factors include:
- Seasonality
- Events
- Promotions
- Lead times
- Product cannibalization
- Geodemographic diversity
5. Micro-merchandising: Clustering of products and stores based on user-set quantity minimums and SKUs
6. Optimal safety stock: Calculation of optimal safety stock quantities using the demand forecasting model and variables such as:
- Volatility of demand
- Lead time variability
- Vendor fill rates
- Company policies (e.g., “desired customer service level”)
According to Retalon, its solution:
- Increased Simons’ promotional forecast accuracy by 40%.
- Generated a highly-accurate demand forecast model
- Significantly reduced associated resources, time, and manual labor costs
No specific data were provided for the second or third benefits listed above.
According to a press release by Retalon, its solution for Simons also won the Retail Council of Canada’s “Supply Chain Innovation” award for “Synchronizing the Supply Chain to Support Growth & Expansion in an Omni-Channel Environment.”