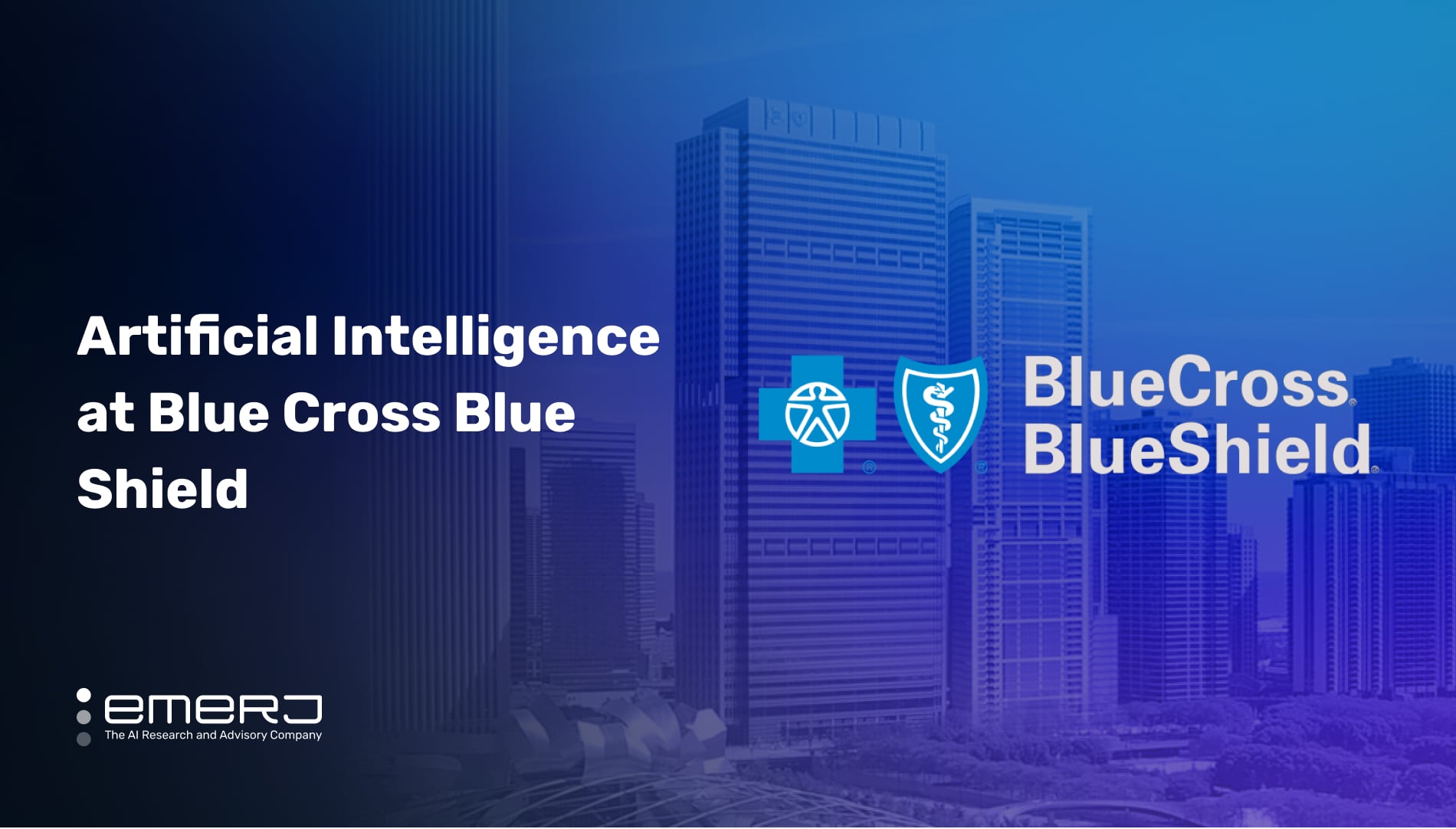
Blue Cross Blue Shield (BCBS) is a federation of 35 independent health insurance companies that provide coverage to over 100 million Americans. The organization’s national headquarters is located in Chicago, Illinois, while individual BCBS companies operate in various states across the country.
Blue Cross Blue Shield (BCBS) companies have demonstrated strong financial performance in recent periods. In the first half of 2023, BCBS companies collectively reported $7.5 billion in net income. BCBS has significantly invested in AI and digital health technologies to enhance patient care, streamline operations, and improve member experiences.
Harvard Business Review notes that Blue Cross Blue Shield of Michigan (BCBSM) has transformed from the least to the most efficient user of technology within the Blue Cross system, as measured by technology cost per employee. They have adopted various new technologies, including cloud computing, security protocols, mobile support, and over 20 AI applications.
This article explores two compelling use cases that illustrate how Eli Lily AI initiatives are actively supporting its strategic business objectives:
- Leveraging machine learning to improve medication adherence: Using predictive analytics and machine learning to target and personalize interventions to increase medication adherence.
- Using machine learning to detect fraud: Identifying anomalies, patterns, and suspicious activities with machine learning that indicate potential fraud, allowing for timely intervention and prevention.
Leveraging Machine Learning to Improve Medication Adherence
Medication adherence, the extent to which patients take medications as prescribed, is a critical yet often overlooked aspect of healthcare. Assistant Clinical Professor of Family and Community Medicine at the University of California says nonadherence affects 40-50% of patients with chronic conditions, leading to approximately 100,000 preventable deaths and $100 billion in avoidable medical costs annually.
The American Medical Association believes this complex issue stems from various factors, including patient behavior, treatment complexity, and systemic healthcare challenges. Despite its significant impact on patient outcomes and healthcare economics, medication nonadherence remains an “invisible” problem, lacking prioritization and ownership within the medical community.
A case report from Blue Cross partner AllazoHealth further mentions Blue Cross Blue Shield (BCBS) faced challenges in effectively targeting members for medication adherence interventions. Traditional methods of identifying at-risk members were inefficient and often resulted in wasted resources on members who didn’t need interventions or were unlikely to respond positively.
To combat the above challenge and improve patient adherence rates, BCBS of North Carolina engaged with AllazoHealth, a health tech company. The case report mentions that BCBS uses Allazoengine, the AI-powered platform from AllazoHealth, to drive interventions aimed at improving medication adherence.
According to the case study documentation published by AllazoHealth, BCBS of North Carolina implemented the former’s AI-driven solution, which includes:
- Machine learning algorithms
- Predictive analytics
- Data integration systems to process various member/patient data points
In the same documentation, AllazoHealth also claims BCBS took the following approach to driving medical adherence with machine learning capabilities:
- Step 1: Data Collection
Gathered comprehensive member data, including demographic information, prescription history, and healthcare utilization patterns, to create a robust dataset for analysis. - Step 2: Predictive Modeling
Applied machine learning algorithms to analyze the collected data, predict which members are at risk of nonadherence, determine the optimal timing for interventions, and identify the most effective intervention methods for each member. - Step 3: Personalization of Interventions
Tailored outreach methods based on AI predictions by preparing various intervention types such as live phone calls, interactive voice response (IVR) calls, text messages, and emails to engage members effectively. - Step 4: Implementation of Targeted Outreach
Focused resources on members most likely to benefit from interventions while reducing unnecessary outreach to those who are likely to remain adherent without additional support. - Step 5: Execution of Interventions
Conducted personalized interventions using the selected outreach methods to maximize engagement and effectiveness. - Step 6: Data Collection on Intervention Outcomes
Gathered data on the results of each intervention to assess their impact on medication adherence and overall member health. - Step 7: Continuous Learning and Model Refinement
Fed the outcome data back into the AI model to continuously improve its accuracy and effectiveness based on real-world results.
The case study documentation from AllazoHealth also claims that BCBS’s structured approach enabled them to effectively leverage AI in addressing medication nonadherence, leading to improved clinical outcomes and enhanced quality of care for their members.
A quick four-minute video shared below explains how the Allazo engine works to increase medication adherence:
The case report also claims the following outcomes for BCBS North Carolina:
- 5.45 times more efficient in identifying members who would benefit from interventions compared to traditional methods.
- Reduced unnecessary outreach to members likely to remain adherent without intervention.
- Achieved a 1.9 times higher medication adherence rate among targeted members compared to the control group.
- Realized significant cost savings due to improved medication adherence and reduced healthcare utilization (specific numbers not provided).
Using Machine Learning to Detect Fraud
The Insurance Bureau of Canada estimated that insurance fraud cost Canadians over USD 1 billion in 2021. In the U.S., the Sentencing Commission reported that in fiscal year 2023, the median loss for healthcare fraud offenses was $1,416,231, with 4.7% of cases involving losses exceeding $9.5 million. Fraud not only impacts health insurance companies but also financially burdens plan members and sponsors, leading to higher premiums for all.
Alberta Blue Cross may have experienced a high level of fraud activities when it started experimenting with AI to detect and prevent fraud. According to an article published by the Alberta Blue Cross, the traditional rule-based approach to fighting fraud is not enough in today’s age of sophisticated fraud.
Alberta Blue Cross also published a white paper titled “Raising the Red Flag“, which explains that traditional programming to fight fraud is not efficient in detecting unknown fraud schemes or adapting to new fraud patterns.
Per the same white paper, Alberta Blue Cross implemented an advanced AI and machine learning-based system to enhance its fraud detection capabilities.
Here is the approach Alberta Blue Cross took to fight fraud:
- Step 1: Data Integration
Consolidated vast amounts of claims data from various sources, including historical claims, provider information, and member data. Such consolidation created a comprehensive dataset for analysis, providing a holistic view of claiming patterns and behaviors. - Step 2: AI and Machine Learning Implementation
Developed and deployed advanced algorithms utilizing both supervised and unsupervised learning techniques. Implemented natural language processing for text analysis to form the core of the fraud detection system, enabling complex pattern recognition and anomaly detection. - Step 3: Pattern Recognition
Used AI algorithms to identify unusual claiming patterns and behaviors and detected anomalies in service utilization and billing practices. This step helped in spotting potential fraud that traditional methods might miss. - Step 4: Predictive Modeling
Created models to predict the likelihood of fraudulent activities based on historical data and patterns. Continuously refined these models based on new data and outcomes to ensure the system stays up-to-date with evolving fraud tactics. - Step 5: Real-time Analysis
Implemented real-time screening of claims, flagging suspicious activities for immediate review. Real-time screening also allowed for proactive fraud prevention, potentially stopping fraudulent claims before they are processed.
Further, the company also developed intuitive dashboards for straightforward interpretation of complex data. These tools enabled investigators to quickly identify and investigate potential fraud cases, improving the efficiency and effectiveness of fraud detection efforts.
While Alberta Blue Cross does not showcase its AI fraud detection process, it provides educational videos to raise awareness about potential fraud schemes and empower members to protect themselves. The brief video below is from Blue Cross Blue Shield Texas. It advises customers on how best to avoid medical fraud and runs roughly five-and-a-half minutes:
Per the white paper, this dynamic and multi-layered approach helps the Blue Cross identify and prevent fraud.
While the Alberta Blue Cross paper does not mention any specific numbered results for their use case, a research paper by Liberty University, USA, states that machine learning enhances fraud detection by quickly analyzing vast data, identifying subtle patterns, and adapting to new schemes in real time. These technologies may reduce false positives and allow human analysts to focus on complex cases, improving overall fraud prevention efficiency.