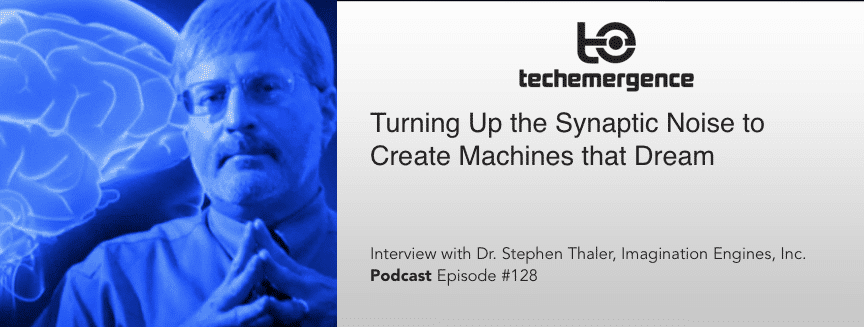
Episode Summary: Neural network – it’s almost a buzz word, but it was looked down on during certain periods of AI development. Nonetheless, most of the public is not aware of what a neural network is, how it works, and how we can create an artificial one.
CEO and Founder of Imagination Engines, Inc., Dr. Stephen Thaler gives us some insight today on how neural networks create what we call creativity, and provides his perspective on how a neural net eventually merges to give way to consciousness.
Guest: Dr. Stephen Thaler
Expertise: Artificial Intelligence and Cognitive Science
Recognition in Brief: After receiving his PhD in Physics from University of Missouri-Columbia, Dr. Thaler went on to work in several diverse technology areas, including nuclear radiation, electromagnetic, high-energy laser interactions, neural networks, and artificial intelligence. After witnessing great ideas emerge from near-death experiences of artificial neural networks, Thaler came up with and created the Creativity Machine, an artificial neural system that discovers and invents new ideas. He founded Imagination Engines, Inc., in 1995, of which he is also the CEO. The Creativity Machine has been used to create new technologies, products, and services for a number of companies and organizations, from NASA to General Electric.
Current Affiliations: Imagination Engines, Inc.; Principal Scientist for Sytex, Inc.
Building a Neural Network
Neural networks – what are they? I asked Dr. Stephen Thaler this question, who suggested we start with the familiar idea of a computer program or algorithm – by definition, these are not neural networks.
Instead, he describes them as “simply collections of cells or switches that can switch either on or off, and they are connected by…connection weights, synaptic connections…these neural systems build models of whatever worlds they are exposed to, and they do that by connecting up the switches, the neurons, into colonies, that are essentially our token representations of things in the external world.”
Using a simple example, in the world of “the farm”, a neural network is busy at work building models of horses, pigs, cows, and any other related object or feature. After building these models, colonies of neurons begin to hook up and form relationships, determining cause and effect between objects and situations, deciding what input and output is included and excluded from a picture of reality, and many other variables.
Stephen explains that all of this is achieved in nature with connection weights, what’s communicated through the ‘synaptic clefts’, the small fluid-filled gaps between two neurons in the brain. In digital computers, those weights are emulated by double-precision numbers.
You might be wondering how this modeling is actually done. While delving into the specifics of how the modeling works is rather technical, Thaler breaks down the basic abstract procedure to give us a better understanding of what is going on in the brain of our archetype farmer.
To start, we are sensory beings, which is how we make sense of the world. Therefore, the farmer might have visual input being received by his or her eyes, while part of the resulting output could be the sounds that the animals are creating.
If the farmer sees an image of a horse – as recognized by the neural network – that propagates through the network, predictive experience and established neuronal relationships might trigger one response to be a “mock up” of the sound of the horse. The connection weights in the synopsis are adjusted to achieve the so-called mapping process of the abstract relationship. When we speak of predictive networks, says Thaler, we’re dealing with pattern-based computing, and arguably everything in the world can be described in patterns.
This foundation of neural network knowledge leads to Thaler’s expertise in “creative computing.” In this domain, the real question, says Stephen, is how do you make a neural network generate an activation pattern that has never occurred up until that time?
The Creative Mind Amidst the Noise
The idea of a “novel pattern” stems back to Thaler’s hobby in the mid-70s, which he describes as playing with rudimentary neural networks. “I remember I was a budding theoretical physicist, and I was taking lattice models, things like ferromagnets and ferroelectric materials, and essentially freezing in memories…freezing the domains of a ferromagnet to represent a smiley face (for example).” Afterwards, he’d raise the computational temperature, which causes a magnet to lose some of its magnetization.
Thaler discovered that after heating, the spins of the simulated atoms would begin to diminish in their interaction, and would produce other kinds of faces, or at least what looked like faces. What was actually happening is the interaction between spins began to dissolve, and Thaler was recognizing a different kind of face.
“It’s more like looking at the man in the moon or that noisy train feature on Mars that everyone thought was an anthropomorphic form, but it wasn’t, and the clue came when I basically shifted the angle with which I was looking”, he said, “It looked like nonsense…what I found was there was perception getting involved.”
Thaler realized that the transition between memory generation at low noise levels and the nonsense perceived at high noise levels held the key to the idea of artificial creativity i.e. the inter-mid regime. Potential ideas stem from this original conceptual space, says Thaler, and there’s a thin skin on the original conceptual space.
At a correctly tuned level of synaptic perturbation, when thousands of other neurons are feeding the connection channel between two neurons, and with many neural networks conspiring together (which are also connected by synapses), the concept of the creativity machine or conscious machine intelligence begins to generate.
This synaptic perturbation can be represented on a computer by double precision numbers. Modifying these connection constraints can lead to creating things or “memories” that didn’t exist, that spontaneously synthesized. At a certain level of synaptic noise within the network, you reach a “glory regime”, a network that starts to “think creatively”, to generate mildly false memories, and “many of those are good ideas”, says Thaler.
This is the basic idea behind Thaler’s patented Creativity Machine, with which users can turn up “the noise”, i.e. the synaptic perturbation, until useful information is generated.
“If you simply take another network…that’s trained to map from the idea to some figure of merit…this is a barebones creativity machine, generating new ideas, that are then filtered out, that can then be used in real-time or archived for lateral perusal, and to make things even more efficient, you can use the outputs of network, in the form of a distance from some target behavior, and use that metric to modulate that synaptic noise, and the results is that if the system does not see a good idea in 100, 300 milliseconds, it can start to wrap up the noise, to make the thinking more twisted inside the noisy neural network, which I call an imaginatron,” explains Thaler.