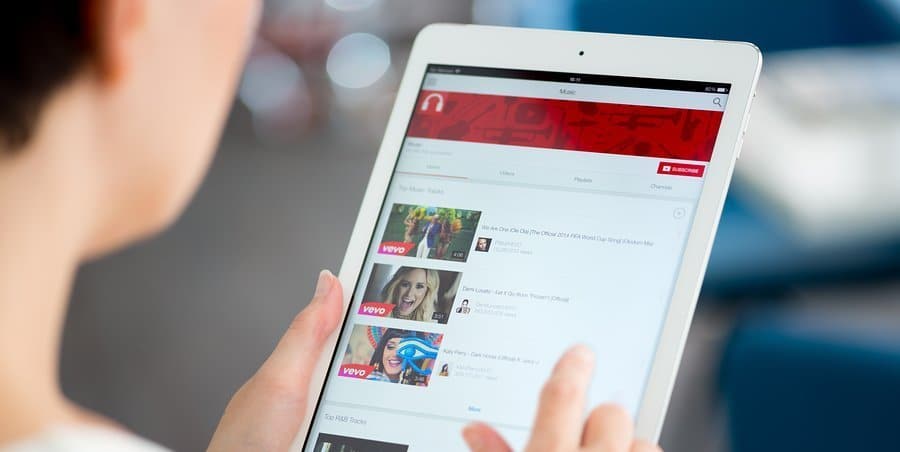
Episode Summary: AI, specifically natural language processing, has made it easier to understand text as a medium in a deeper, more efficient way and at scale. With video, the situation is quite different. AI is already being used to help industries that work in the video medium. However, searching for content within videos is more challenging because video is not just voice and sound, it is also a collection of moving and still images on screen. How could AI work to overcome that challenge?
In this episode of the AI in Industry podcast, we interview Dr. Manish Gupta, CEO, and co-founder of VideoKen, as well as Infosys Chair Professor at the International Institute of Information Technology, Bangalore, about the future of video search as machine learning is increasingly integrated into the process. Dr. Gupta talks about how video is becoming more searchable and discusses his own forecasts about what that will look like in the future. He also predicts what machine learning will allow Youtube to do as people continue to search for more specific video content.
Our content lead, Raghav Bharadwaj, joins us for this interview.
Subscribe to our AI in Industry Podcast with your favorite podcast service:
Guest: Dr. Manish Gupta, co-founder and CEO, VideoKen
Expertise: high-performance computing, compilers, distributed systems, virtual machine optimizations
Brief Recognition: Dr. Gupta is also Chair Professor at the Infosys Foundation at the International Institute of Information Technology Bangalore. Previously, he served as Vice President and Director of Xerox Research Center India. He has held leadership positions at IBM, including that of Director at IBM Research India and Chief Technologist at IBM India/South Asia. As a Senior Manager at the IBM T.J. Watson Research Center, Dr. Gupta led the team developing software for the Blue Gene/L supercomputer.
He earned his PhD in Computer Science from the University of Illinois at Urbana Champaign. He co-authored 75 papers, and has more than 6,000 citations in Google Scholar related to high-performance computing, compilers, and virtual machine optimizations. Dr. Gupta has also been granted 19 US patents.
Interview Highlights
(3:13) In What role does AI play in video data that is productive in a business context?
Manish Gupta: Videos are powerful but tend to be opaque, unlike text which you can quickly visualize. You usually have to play through the whole video to figure out the content. But people don’t have the patience to go through a 30-minute informational video.
VideoKen is looking at a class of videos, such as lectures, information videos, presentations, and trainings. Our starting point was to to automatically build features such as a table of contents and a glossary at the end into videos. We have leveraged AI techniques that analyze the content of the video. Out of the thousands of words in the video, which are the most important words?
(6:30) Is there an analysis of the images?
MG: Yes. Particularly for the table of contents, we feel the visual data in an informational video, especially a lecture to be extremely rich, much richer than the data we get from audio. Our application identifies which traits of the video contain that visually rich text. This is possible with AI and is a classification problem where the technology tries to identify the product trait. Does it have rich information in the form of text? Once that is determined, you will have to identify the salient text.
Take, for example, lectures in the form of slides. The change of topic would typically happen at the slide boundary. That part is very rich with information. The application extracts the important words from this part. The richer content also comes from those titles.
(9:00) In focusing on developing AI for videos, you need to find common features of informational videos, such as lectures and slides. How do you train an algorithm for this kind of video?
MG: We are at the starting point, just like a textbook. But potentially you could do an analysis of different segments of the presentation and discover the concepts that are covered there. There are views that we might get from the audio. Different instructors have different ways of changing topics.
(09:55) You have to find a new set of patterns to train the algorithms about a new mode of teaching.
MG: There is often a bigger gap or silence (between topics or slides). But you can’t pre-program all of these nuances or variations. They have to be learned from the data.
(10:28) In creating a table of contents or a glossary, is there a process of checking how well the algorithms distill the information or how they can be trained to do better?
MG: We created an editing tool to give the end user the ability to modify. Producing a video is labor intensive. But the amount of effort it takes to edit the table of contents that has been created by the application is simplified by being able to edit or change a few entries.
(12:15) As corporate users edit the table of contents or the glossary, that serves as feedback for the system. It seems the goal is to drive the machine learning from the side of users, rather than from a team of natural language processing experts.
As an example, I am looking for oil and gas drilling developments in tundra environments in the year 2015. I just want to see the parts that show the drilling. The goal would be to find a way to query those specific parts. Is that the objective?
MG: We are trying to make the search process easier. Not just searching among the videos, but also searching within the video. You don’t want a person to go through an entire hour-long video.
(15:00) Youtube’s platform is a mix of music, entertainment, and education. It’s not their niche to find business topics. Your niche is organizations that need to educate their people in a time-efficient way.
MG: That is the starting point. One of our realizations is that there is no company formally utilizing this resource. Most companies have classroom lessons or buy content to use in their employee trainings. One of the things VideoKen is enabling is utilizing this wealth of information already contained in freely available videos on Youtube. There are 3 million educational videos on Youtube alone. But companies aren’t able to find the high-quality videos because it is a pain. How do companies separate the high-quality from the low-quality videos? From there, they can choose the appropriate content for their context.
Most companies have customer events, with the content posted on Youtube. Chances are people will only view the early part of the video. What our application lets the user do is process these videos and make them more consumable and more impactful.
The applications of these videos go far beyond learning. We can take our customer’s videos and we can index them with our application.
(17:55) The analysis of video could become possible in the next two or three years. Where do you see this going?
MG: The next step is developing a deeper understanding of videos. One challenge is recognizing nouns and verbs. So the work is going to noun (name, object, person, place) recognition and verb (activity) recognition. This is applicable for creating captions.
Subscribe to our AI in Industry Podcast with your favorite podcast service:
Header Image Credit: Optometry CEO