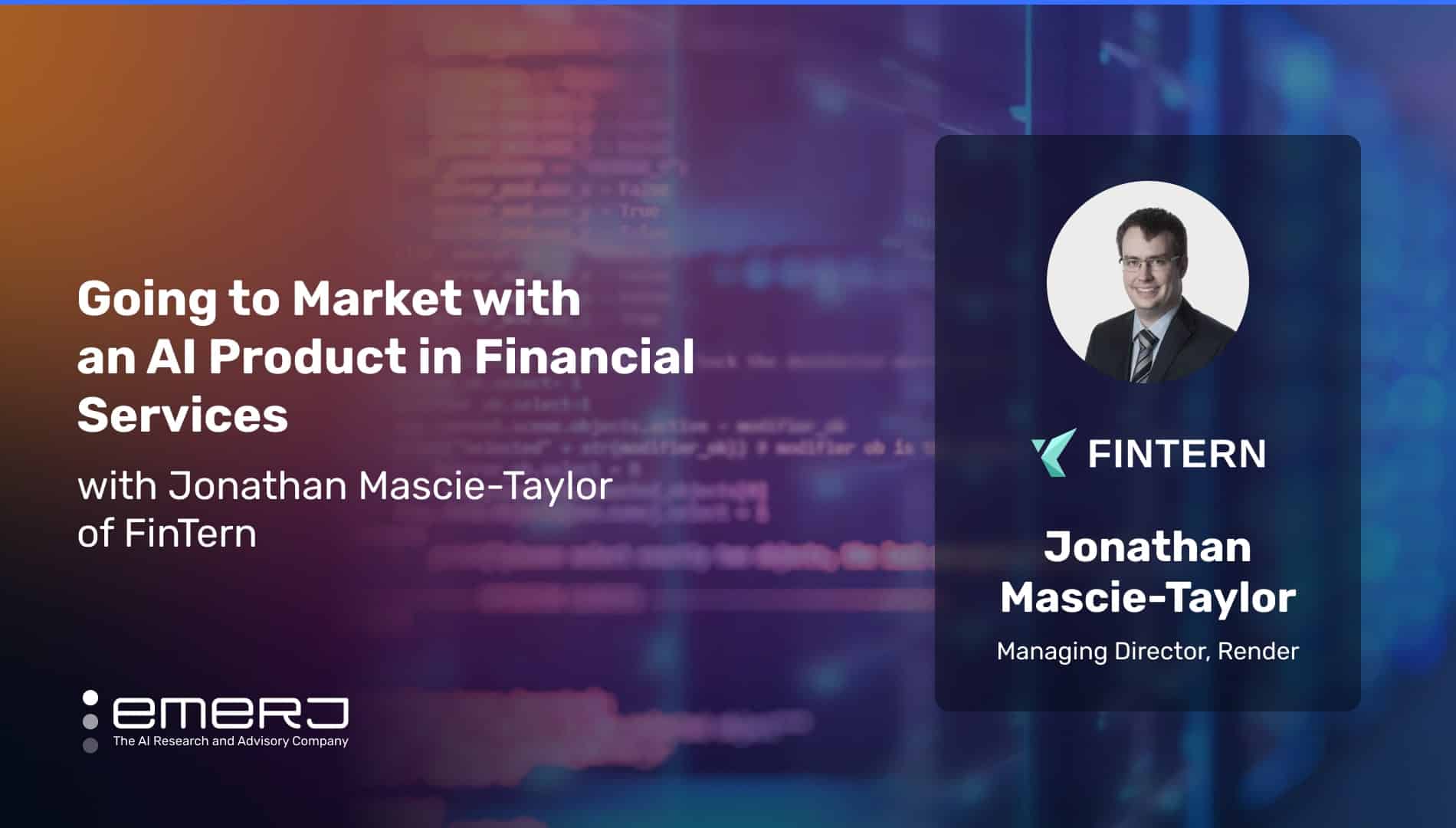
The concept of customer experience is reaching new heights with technological advancements. Mobile technology and applications are increasingly becoming part of consumers’ lives. The impact of technology on customer experience is growing tremendously. Fintech companies are coming up with innovations to serve customers with the best. One such initiative to bring increased value to customers is open banking.
Open banking is a process that facilitates the secure sharing of account data with licensed third parties through Application Programming Interfaces (APIs). It empowers customers with the ownership of their data.
For instance, if someone wants to use a financial product that offers them a complete picture of their financial status, including expenses, savings, and investments. For the mentioned financial product to work, it needs details and data from the customers’ bank. The customer then instructs their bank to share their data with a third party. The fundamental idea is to let customers or borrowers decide if they want to share data with third parties.
Emerj CEO and Head of Research Daniel Faggella recently spoke with Jonathan Mascie-Taylor, Managing Director of FinTern B2B, on the AI in Business podcast about the potential of open banking to streamline financial services workflows. In the following analysis of their conversation, we examine how leaders can execute two organizational capabilities by integrating open banking technology:
- Developing seamless customer experience: Using API-enabled open banking to provide more customized and better prize loans.
- Building data models for personal and business loans: Estimating risk on loans with credit risk analysis models using a probability of default, loss given default, and exposure at default.
Listen to the full episode below:
Guest: Dr. Jonathan Mascie-Taylor, Managing Director of Fintern B2B (Render)
Expertise: Mathematical modeling and statistics.
Brief Recognition: His previous roles include Head of Data Science at Capital on Tap, Associate Partner at McKinsey & Principal at Oliver Wyman. He has worked with a global set of clients, from banking to retailers, providing strategic, analytical, and technological support.
Leveraging Open Banking For Seamless Customer Experience
As Jonathan details on the podcast, credit unions and lending companies expressed a need for a tool to make their credit and finance decisions easier. Another business asks from the lending and financing companies were:
- How can they grow the market size?
- How can they help the under-served?
- How can they make the customer experience better?
- How can they make the process simple and easy for the customer?
“When customers request a mortgage or a loan, they are asked questions on their rent, expenses, holidays, savings, and investments. They are supposed to fill out a long application form with these details. The details are estimates that could be different from potential reality.”
– Managing Director of FinTern B2B, Jonathan Mascie-Taylor
Expressing this business problem, Jonathan says that many of the solutions lie in emerging technologies known as, and described previously, as open banking.
With open banking and APIs, the lending company can pre-populate all the information with the customer’s consent by using open banking data. Jonathan further shared that this approach is more effective in small ticket-size loans.
Via an open banking system, customers can use the financial services from service providers available on the network by giving consent to their banks to share their data with a third party. While APIs allow sharing of information between the systems, in open banking, APIs provide access to the third party to the financial institution’s customer data with customer consent.
APIs are also crucial for banking as a Service (BaaS) which is a vital part of open banking. BaaS is an end-to-end process that enables fintech and customers to use banks’ frameworks using APIS.
Open banking helps lending companies reduce their business costs through a reduction in manual processes. More than banks and fintech companies, open banking has made it easy for the customer. As a value proposition for the customer, open banking provides more customized and better-priced loans due to a complete data picture of their finances.
The ecosystem requirements for successful open banking are:
- APIs: APIs are the mechanism that connects banks with other parties in the digital ecosystem.
- Enabling assets: Assets enabling open banking include digital identity, access management, and data and analytics.
- Use cases: To prioritize use cases, banks must look beyond compliance to develop core objectives for open banking.
- Applications & partnerships: Banks will need to offer seamless customer experience through various applications and deep strategic service partnerships.
One of the successful examples of open banking came from India, where the model adopted is based on a hybrid approach. The market and the government have active roles in developing open banking. The motive behind India’s initiative for open banking was to explore the usage of accessed customer data to benefit both customers and banks.
Building Data Models for Business and Personal Loans
Banks assess the risk to ensure that the loan recovers at the maximum rate and the losses are lower when it comes to lending. The best way to monitor and measure risk is by building and implementing credit risk models.
The credit risk models help banks to make a forecast of expected losses. The information available for risk modeling at the loan application time may vary. Factors like age, gender, occupation, level of education, income level, investments, and many others help the bank assess the risk involved in extending the loan. It is known that the riskier the loan, the higher the interest rate.
“Banks are at risk for both USD 2,000 and USD 2,00,00 loans. Fundamentally the risk parameters stay the same in the credit risk analysis models, but what changes is the demographic and the need of people applying for those two different loans.”
– Managing Director of FinTern B2B, Jonathan Mascie-Taylor
Jonathan emphasizes that while the estimate of risk in a $2,000,000 loan and a $2,000 loan are different but the parameters to define and analyze the risk remain the same. Further, he says that the risk models of smaller ticker-size loans may work on the large ticket-size loans, but the efficiency will be impacted.
Banks look at three main components to ascertain the risk involved in lending. These are:
- Probability of Default (PD)
- Loss Given Default (LGD)
- Exposure at Default (EAD)
The probability of default is a crucial risk parameter financial companies use while analyzing credit risk. It measures the probability of the customer’s default within a specified time. It is a measure that assigns a value between 1 and 0.
Loss-given default is the percentage of exposure that will be lost and cannot be recovered. An LGD value of 100 percent indicates that the recovery rate will be zero; hence the bank will fail to recover the loan.
Exposure at default is the total amount the bank will lose when the customer can no longer pay the debt.
While sharing his learning from the project, Jonathan says that the difference between small ticket size loans and the larger ones is not only in the estimated risk but also in the consumer journeys. For instance, the solution that fits neatly into the consumer loan space may need to be revised to fit into the housing/mortgage loan space.