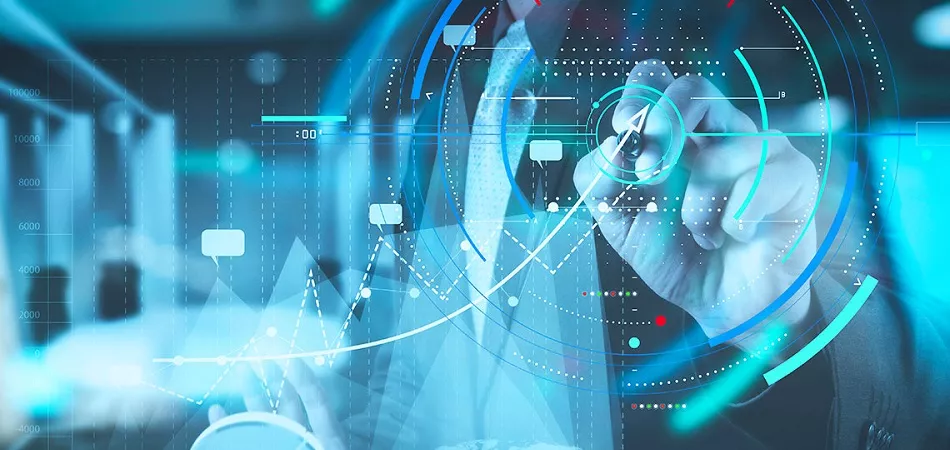
Predictive analytics is perhaps one of the most common AI applications used by financial institutions, banks, insurance companies, and healthcare companies. This type of software allows business leaders across these industries to plan for the most probable outcomes in business areas such as credit, loans, and patient health. Predictive analytics software could make predictions about future business events based on typical company experience using historical enterprise data.
In this article, we define predictive analytics and showcase other definitions from experts in the field. We give context into how AI and ML help predictive analytics serve as a tool for business intelligence. Additionally, we include an example of a predictive analytics vendor and how its AI solutions can purportedly help clients in a variety of industries.
Our explanation of predictive analytics begins with our own definition, along with context into how the software benefits from machine learning algorithms.
What is Predictive Analytics?
Predictive Analytics is a type of software made for using enterprise data in order to forecast changes in an organization’s chosen business area. This allows organizations to plan for the most statistically probable outcomes based on phenomena the organization has observed in the past.
Predictive Analytics Was Not Always AI
Predictive analytics is a type of AI software when it is powered by a machine learning model, but this has only become more common in recent years. Prior to this, the term “predictive analytics” referred to the use of multiple distinct business intelligence techniques to determine the most likely future events.
However, these techniques were not so sophisticated as to provide confidence scores or statistical percentages indicating the most likely outcome. Instead, enterprise data was used to create predictive models that simply showed how the software came to its conclusion and why the predicted outcome might happen.
How Machine Learning Makes Predictive Analytics Stronger
A predictive analytics application powered by machine learning has the capability to utilize a much greater amount of data and make more accurate predictions based on it. Machine learning is able to handle larger datasets because it requires as clear an image of the organization’s business history as possible to work properly. Once the machine learning model is trained on data related to the organization’s chosen business area, it can automate the analytics techniques used to make predictions.
These predictions usually include a list of the most probable outcomes along with a confidence score indicating how accurate the prediction is based on the software’s estimation. If the software makes a prediction that produces a confidence score below a certain number, it will not send that prediction to the user. This confidence level is usually set at a very high interval such as 90 or 92%.
It is important to note that training a machine learning model for a predictive analytics application requires a large amount of structured data as well as time for a trial installation period. This amounts to feeding the structured data into the machine learning model until it is able to recognize trends and patterns in the client organization’s business.
Once the model can recognize the important types of information such as claim amounts or hospital readmission, the organization will need to integrate it into their tech stack and allow it to run in the background. During this time, the machine learning model will be training just as it did with the legacy data, except with the current events of the business. Business leaders can check the software’s predictions during this time to observe their increasing accuracy.
For example, a predictive analytics application made to predict customer churn will need to be trained on large stores of historical data. Then the application would need to be installed into the client company’s network and allowed to run for the trial period. When the trial period ends, the software should be able to make correlations between live customer behavior and historical reasons for customer churn. This allows it to make predictions on whether individual customers will stay with the client company or not.
The vendor that created the predictive analytics application will usually state how long the trial period needs to go on, and if the client wants to move forward with the software it will already be partially installed.
Expert Definitions
“Predictive Analytics is technology that learns from experience (data) to predict the future behavior of individuals in order to drive better decisions.” –Eric Siegel, Author of Predictive Analytics: The Power to Predict Who Will Click, Buy, Lie, or Die
Predictive Analytics is … a combination of different techniques and fields. Basically the purpose is to predict some future event based on past historic events. … If we compare it with Google Analytics, that’s just studying the data. With predictive analytics, there is an automated predictive element [to its problem solving.] ” – German Sanchis-Trilles, CEO and Co-founder of Sciling Information Technology and Services.
Predictive analytics brings together advanced analytics capabilities spanning ad-hoc statistical analysis, predictive modeling, data mining, text analytics, optimization, real-time scoring and machine learning. These tools help organizations discover patterns in data and go beyond knowing what has happened to anticipating what is likely to happen next. – IBM
Vendor Spotlight: Dataiku
Dataiku is a New York startup founded in 2013. They claim to have created machine learning techniques that analyze raw data for building predictive models in many formats. This raw data may take the form of historical transactions for individual products or sales transcripts from customer interactions. Dataiku claims their AI software can help a business identify relationships between certain data points which can lead to higher efficiency and lower company spend.
Major banks such as Wells Fargo generate large amounts of raw customer data daily, and this can come from customer conversations, social media posts, website activity, marketing campaigns, and transaction information. The ability to process this many disparate data types may allow the following benefits for a banking client:
- Marketing departments or fraud detection teams may gain access to new insights via a dashboard that prompts employees with notes about any anomalies in new data.
- The ability to collect, clean, and analyze raw customer data. This would allow the client company to gain insights into the relationships between social media posts and marketing campaign sales. The company may use this to understand trends and predict untapped markets.
- Additionally, patterns in international transactions and customer interactions may help the client identify fraudulent behavior and develop more stringent prevention techniques.
When a Dataiku user logs into the system, they can upload data to be organized by the software. Dataiku claims the data shows up in the form of a spreadsheet and is organized automatically. The software will associate each data point with certain common traits it detects across the newly integrated data. So when the company says it can detect whether specific data is associated with a male or female customer, they mean that the software has come to make gendered associations for certain customer behavior. Additionally, there is a proportion scale at the top of each column in order to communicate the number of missing values related to that type of data.
The user can then click on the header for each column to visualize the data, which may allow them to see this data in the form of a chart or graph. They can also purportedly generate graphs that cross-reference different columns. If the user thinks there may be outliers in the data, the software can give the user a prompt on how to correct them and further train the software.
The following 4-minute video is a demonstration from Dataiku. This shows how businesses can edit, monitor, and see insights gleaned from raw data using this predictive analytics application:
Industry Use Cases
Pharmaceuticals
One of the most prominent uses of predictive analytics in the pharmaceutical industry is design and optimization of clinical trials. An application like this could analyze the medical histories of patients to determine which ones will respond best to the drug being tested. This helps the company find the best patients to try and recruit for the clinical trial.
This type of software solution can help pharmaceutical companies design and organize clinical trials in numerous other ways as well. These include research on possible side effects the drug could have and which patients are most likely to experience them. Additionally, some applications can allow for genetic clustering, or the segmentation of patients based on their likelihood to respond well to the drug.
Below is a demonstration video from vendor Dataiku showing their software platform called DSS. The demonstration takes the audience through the user’s process for utilizing datasets to predict doctors’ prescriptions of different drugs. Although the video is 13 minutes long, the most important sections are also listed below:
- At 0:00 the demonstrator begins by finding the required datasets for the prediction they are going to make
- At 2:30 the demonstrator explains the goal of the data experiment and combines the previously acquired datasets to check for any contradictions.
- 6:30 shows the demonstrator joining datasets and “cleaning” any incongruencies between the datasets. For this specific experiment, the demonstrator needs to ensure that all of the physician’s ID numbers appear accurately or find out why some are missing.
- 8:25 is when the demonstrator populates all relevant information into a single table. Here they can find certain rows that can be combined into one for slightly less granular categories.
- Finally, 9:58 shows how the demonstrator takes all of the cleaned and organized data and uses it to create and predictive model for each physician’s prescriptions.
Healthcare
Healthcare companies can use predictive analytics applications to help prevent patient readmissions to hospitals, predict patient health decline, and predict the likelihood that a patient will miss an appointment. The AI vendor Health Catalyst offers a solution that they claim can accomplish all of these using medical history from patients as data.
Once integrated, hospitals can log into the Health Catalyst dashboard and bring up a patient profile. That profile would show the patient’s likelihood to contract a serious illness, to miss an appointment, or to be readmitted at a later date as percentages. This may allow healthcare providers to keep a closer watch on patients who may be at higher risk due to neglecting their health.
Financial Services
Predictive analytics can help financial institutions predict the risk levels associated with lending money or issuing credit cards, including the likelihood that a customer will default on their payments. This is especially helpful for institutions trying to grow by increasing their number of active loans as well as the amount each loan is for. When these loans come in the form of credit cards, the institution may need a strategy to predict how much risk is associated with each application.
A predictive analytics application could calculate this using the applicant’s credit score, credit history, and overall financial history if it is available. Additionally, this type of credit risk scoring can help a financial institution recognize incorrect payment amounts in real-time. This may bolster the institution’s ability to catch fraudulent payments before they can be fully processed.
Insurance
In the insurance industry, machine learning-enabled predictive models can help businesses prevent customer churn and thus keep customers for longer periods of time. Some applications can score customers on the lifetime value they stand to offer the insurance company. This type of application could be useful for finding new ways to market to these customers and entice them to raise their insurance plan.
Instead of using the customer’s personal financial industry, insurance companies can simply leverage their historical and transactional data with the customer to make an estimate of how much value they will continue to offer in the future. Data that could be considered evidence of likely customer churn could be how often the customer uses their insurance or speaks with customer services to change or improve their plan.
Header Image Credit: Xoriant