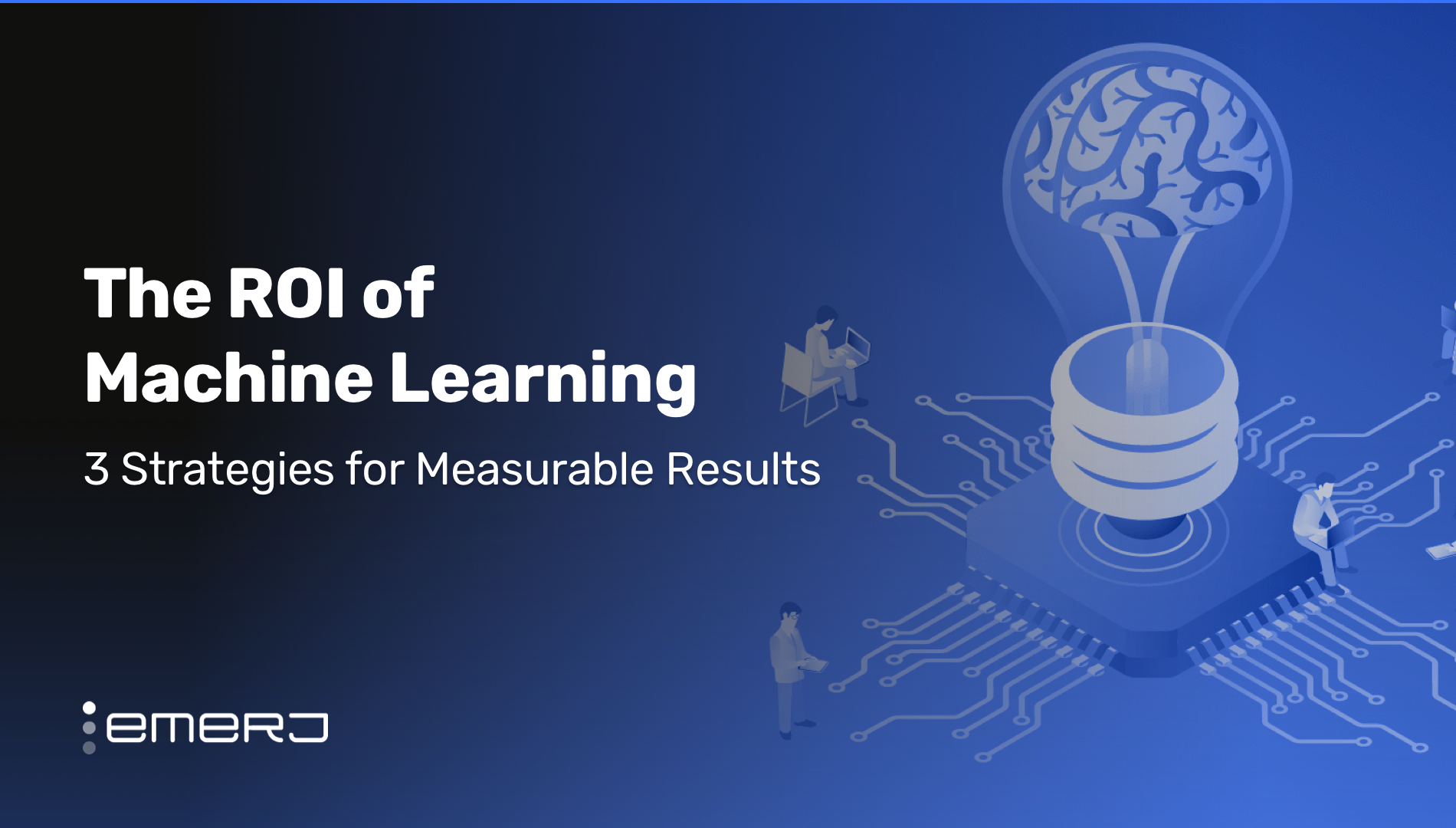
Many business leaders make the mistake of believing that AI and machine learning are like regular IT, but this could not be further from the truth. In large part, this is because, unlike simple software solutions for discreet business problems, it can be very difficult to measure the ROI of machine learning.
This is because the costs associated with adopting AI are often amorphous. They are never as simple as the sticker price of a vendor solution. For example:
- How much does it cost to have a VP of wealth management collaborate with a team of data scientists for three months? Six months? Eighteen months?
- How much does it cost to rearrange a bank’s technology infrastructure and reinvent the way it collects and stores data on its wealth management clients?
- How much does it cost to go back to the drawing board if an AI software that suggests investment decisions actually fails to invest as optimally as actual wealth managers?
As a result, many companies will struggle to gauge how much revenue they are likely to generate, how much money they are likely to save, and how much risk they are likely to reduce by adopting AI software. That said, it is imperative for companies to determine some measurable benchmarks by which they can hold AI projects accountable, even if they aren’t perfect.
In many cases, these benchmarks aren’t even metrics; they are cultural shifts, guidebooks for future AI adoption, and other Critical Capabilities necessary for adopting AI.
Companies should determine these benchmarks by looking at precedents of how their competitors have applied AI for the use-case in which they are interested. Our enterprise clients do this with our AI Opportunity Landscape service, which allows them to focus their AI efforts into business areas that are already benefitting from AI.
In this article, we lay out the three core ways we advise our clients to consider for measuring the ROI of AI:
- Revenue
- Efficiency
- Risk Reduction
We provide examples of each in industries like eCommerce, insurance, and financial services, but first, we discuss how to measure AI in terms of revenue.
AI ROI Measurement Method 1: Revenue
The most obvious way to measure the ROI of AI is revenue. Executives at large companies often jump into their first AI projects eager to generate a financial ROI, but this is much more difficult to achieve than they think, let alone measure it. In some cases, measuring the financial ROI of an AI project can take years.
There are, however, some use-cases in retail and eCommerce that can be measured in less time. These use-cases include recommendation engines, the AI software that allowed Amazon to achieve data dominance.
The ROI of Recommendation Engines in eCommerce
Recommendation engines are machine learning software that use past purchase and demographic data to recommend products to site visitors and customers they might be likely to purchase. Past purchase data includes transaction amounts, total cart values, and the products that customers buy.
These data points are all measured numerically within a company’s CRM. eCommerce companies necessarily track this data, which allows them to more easily compare revenue before and after adopting a recommendation engine. More specifically, an eCommerce company could measure the ROI of a recommendation engine by:
- Percent of new site visitors who become customers.
- Average cart value of a new customer before and after implementing the software
- Customer lifetime value (CLV) before and after implementing the software
In order to measure these metrics, an eCommerce company needs to split test its customers’ experiences. It needs to expose a percentage of its users to the recommendation engine and a percentage of its users to its traditional means of marketing and recommending products to customers.
Then, the company could compare the conversion rates, cart values, and customer lifetime values of each cohort to each other. If the recommendation engine cohort generated higher conversion rates, cart values, and CLV, the software may be generating a positive ROI.
The ROI of Underwriting in Lending
Underwriters can use predictive analytics software to determine the creditworthiness of loan applicants with thin credit profiles or who lack traditional credit scores. Oftentimes, these applicants include young people and people in developing nations.
Although machine learning is often understood as a risk reducer in underwriting, lenders could measure the ROI of this software in terms of revenue. Predictive analytics could help lenders win business from these underserved segments of the population; thus, underwriters will be able to make more money for the business.
However, this is a much more difficult AI use-case to measure in terms of ROI because it won’t be immediately clear which applicants will or won’t default on their loans.
This data needs to be collected over several years, which means lenders will have to keep an AI-enabled underwriting software pilot running until they collect enough of this data to determine whether or not the software is generating a positive ROI.
The insurance carrier could measure the ROI of this AI software by measuring how profitable each customer approved by the predictive analytics software is to the company in comparison to how profitable each customer approved by underwriters is. This measurement would have to be taken over the course of many years as profitability data on machine learning-approved applicants becomes available in the company’s CRM.
The lender would need to set up a process of measuring that ROI over time because within one year, for example, the lender is almost certainly not going to know if a loan will be paid back or not.
As such, the lender would need to set up benchmarks over time to gauge the relative success of the predictive analytics software. In doing so, the lender would be able to measure what sort of new revenue it was able to drive and how profitable that revenue was.
AI ROI Measurement Method 2: Efficiency
Efficiency gains are another way to measure the ROI of AI. Many large companies want to use AI to automate key business processes, such as customer service, fraud detection, and manual data entry, with the express goal of cutting costs.
Companies that want to use AI to save time and money will need to start measuring the time it takes their employees or outsourced workforces to complete the task they want to use AI to automate.
Once they collect this data, they can compare it to the time it takes employees or outsourced workforces to do the same task after adopting the AI software into their workflows.
The classic example of AI for efficiency gains is in cutting customer service overhead.
The ROI of Chatbots for Customer Service
If a company can measure what percentage of new messages from customers can be handled by the conversational interface, then it may be able to determine how much fewer call center resources have been required to handle customer’s concerns since implementing a chatbot.
Another example of measurable efficiency ROI is proper support ticket routing. When a new call or text comes in, a company could adopt a machine learning system that directs the call or text to the right person. The right person to handle a refund is different than the right person to handle a product inquiry. The latter person might be in sales, for example.
The ROI of a conversational interface differs depending on the specific use-case. When a company adopts a conversational interface, it won’t be measuring its ROI, but the ROI of the discreet use-case in which it is being applied.
The ROI of Claims Fraud Detection
Anomaly detection is most commonly used for automating fraud detection and cybersecurity. Large banks have been working with vendors such as Feedzai and Ayasdi in these domains since the early 2010s. The ROI of fraud detection is most obviously risk reduction in banking and financial services, but insurance carriers are often under less regulatory scrutiny than large financial services companies.
They might instead understand the ROI of anomaly detection software as efficiency. The software could allow them to reduce the amount of time that claims adjusters spend investigating claims fraud.
An anomaly detection software could be trained to handle obvious instances of fraud, freeing up fraud specialists at the insurance carrier to focus on more nuanced investigations. In addition, insurance carriers may be able to spend less on fraud specialists if the software is handling low-priority fraud instances on its own.
In order to measure the ROI of this software, an insurance carrier could first measure the amount of time it takes claims adjusters at the company to investigate if a claim is fraudulent. They could then compare this against the amount of time they spend investigating fraud after implementing the anomaly detection system.
The insurance carrier might find that claims adjusters spend much less time determining if a claim is fraudulent, or they might find that claims adjusters is spending even more time determining if a claim is fraudulent because they are spending additional time reviewing all of the false positives the software is flagging.
AI ROI Measurement Method 3: Risk Reduction
In order to avoid heavy fines from regulators, executives at banks and financial institutions have become very interested in using AI to automate risk management processes, including compliance and fraud detection.
AI vendors understand this, and they often market their products for risk reduction if they don’t have another measurable ROI to reference.
For example, when they can’t reference a case study explaining how their software increased revenue in a bank’s wealth management department or reduced the overhead cost of an insurance carrier’s customer support department, vendors might say that their products can help enterprise clients prepare for the sunset of LIBOR or remain GDPR compliant.
In some cases, vendors will market their software for regulatory compliance when financial ROI metrics are unattainable given the use-case, such as enterprise search, which we discuss further in this section.
Attivio is an AI-enabled enterprise search vendor that claims its software can be used to reduce risk. Below is a screenshot of the company’s risk and compliance page:
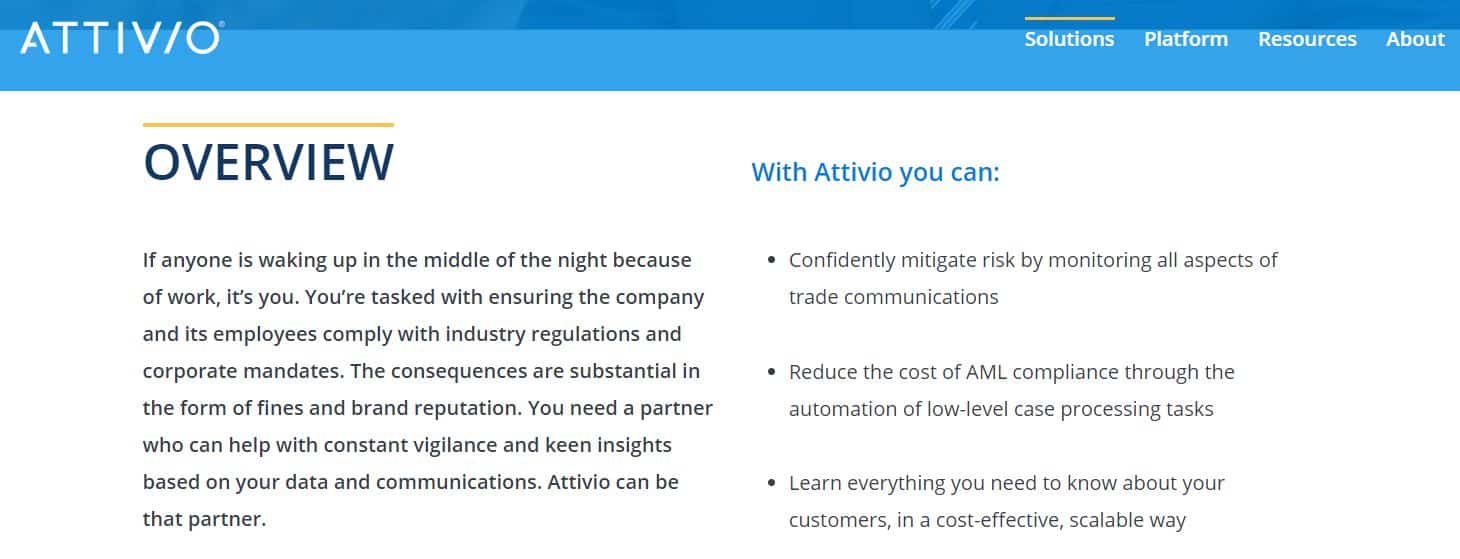
Anxiety about regulation can often be enough to push large companies to buy from vendors.
Although risk reduction is oftentimes the hardest method of measuring the financial ROI of AI projects, there are ways to measure proxies for how much or little a newly integrated AI system is reducing risk.
The ROI of Anti-Money Laundering
For example, in anti-money laundering, companies can first measure the number of false positives and negatives generated before implementing an AI system. Then, they could run the AI software for six months to a year to gather enough instances of false positives and false negatives.
They can then compare the number of false positives and false negatives generated before adopting the software to the number of false positives and negatives after adopting the software.
The ROI of Payment Fraud Detection
AI could also help with payment fraud detection in the financial services and retail industries. Companies could measure the number of instances of fraud that fraud specialists were able to flag in a given month prior to adopting an anomaly detection software for the use-case. They could then measure the number of instances of fraud the anomaly detection software flags within a given month.
Barring a difference in false positives and negatives, companies could determine the success of the anomaly detection software by how much more or less fraudulent transactions it successfully identified compared to fraud specialists.
The ROI of Complying with Data Privacy Regulations
A much more difficult risk reduction-related ROI to measure is how much more or less compliant a company is with regulations before and after adopting AI software. Data privacy laws like GDPR and CCPA require that large enterprises remove customer data from their systems upon customer request. But companies may not be able to access all of their customer data.
In order to fulfill data privacy requests from customers, they may need to digitize some of the data they have stored in paper documents, such as past loan files.
A document digitization software may help with this, and an enterprise search and discovery software may allow companies to then search for customer data that exists in multiple digital locations like an intranet or the cloud. Companies may not be able to gauge the ROI of these software beyond being able to access their data when they weren’t before.
They could measure the time it takes employees to provide documents to auditors or fulfill customer requests to have their data deleted before adopting the software and then compare it to the time it takes employees to provide documents and delete data after adopting the software.
This, however, is impractical; it’s unlikely that any company is timing how long it takes its employees to comply with audits or data privacy requests, and these processes can vary wildly depending on the documents the employees need to find. This is one use-case for which vendors may default to telling clients they could reduce risk without providing metrics of success signifying that their products work.
Emerj Helps Clients Generate ROI From AI Projects
Financial services firms come to Emerj for our AI Opportunity Landscape service, which allows them to discover the highest ROI artificial intelligence projects that align with their priorities. We provide clients with
We provide clients data on areas of the financial services industry that are seeing the most traction with AI and Emerj Strategy Guides that clients use as decision support tools for picking their first AI projects and developing long-term AI visions for their companies.
We encourage professionals that want to deliver an ROI from AI projects at their company to learn more about one of our AI Strategy Guides, Generating AI ROI – Best Practices and Frameworks.