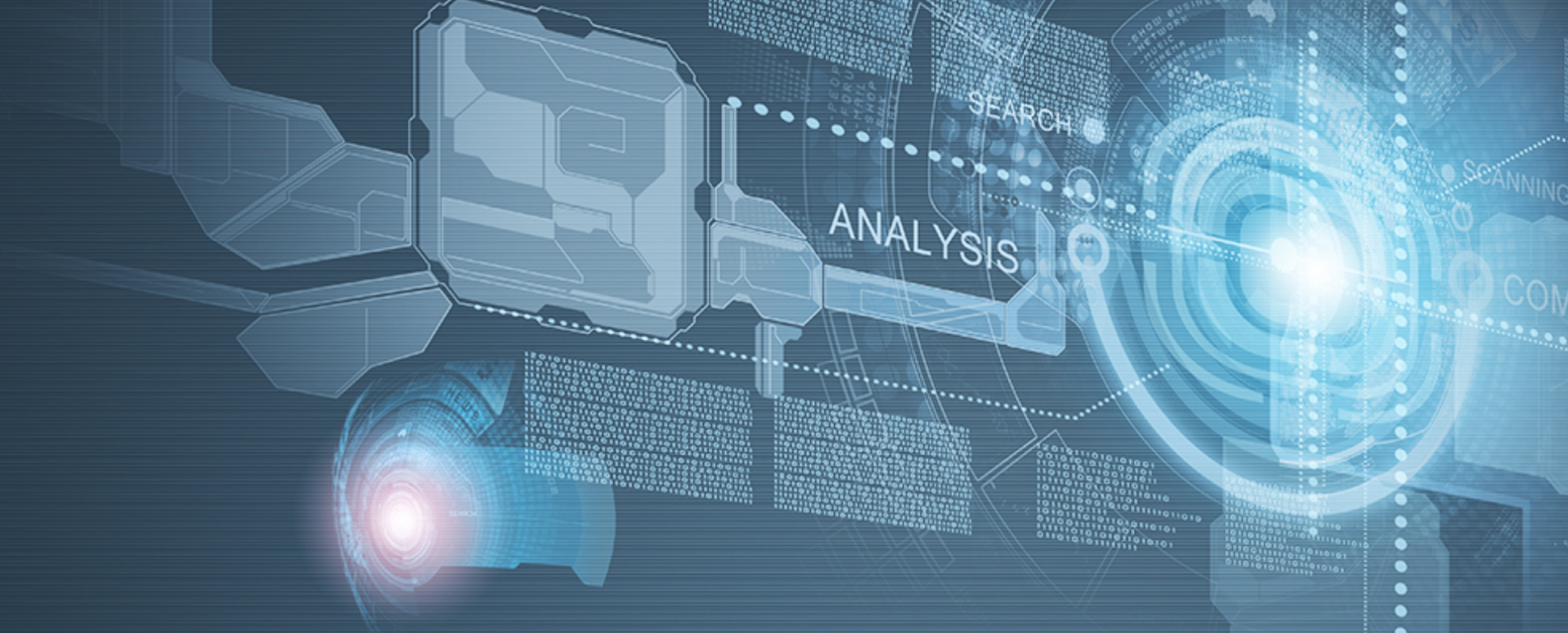
Businesses today around the world have some portion of their operations being automated, which concurrently has meant that a lot of data about these processes is being collected (from sensors or internal company data etc). A combination of AI, big data analytics, and data science techniques seem to be a growing trend in many industry sectors, with predictive analytics being one of the most well-known.
According to a definition from SAS, predictive analytics uses statistical analysis and machine learning to predict the probability of a certain event occurring in the future for a set of historical data points.
Businesses today seem to have a multitude of product offerings to choose from predictive analytics vendors in every industry, which can help businesses leverage their historical data store by discovering complex correlations in the data, identifying unknown patterns, and forecasting. This is hardly surprising considering the fact that predictive analytics can help businesses answer questions such as “Are customers likely to buy my product?” Or even “Which marketing strategies might be most successful?”
We highlight some use cases from the following industry segments with the aim of painting a possibility space for what predictive analytics can really do for business:
- Finance
- Healthcare
- Heavy Industry
- Transportation
- Consumer Goods
Below are five brief use cases for predictive analytics applications across five industry sectors. Each provides a fraction of a glimpse as to how AI technologies are being used today and which are being created and piloted as potential predictive analytics standards in these industries.
Applications of Predictive Analytics in Different Industries
Finance
Rapidminer
Boston-based Rapidminer was founded in 2007 and builds software platforms for data science teams within enterprises that can assist in data cleaning/preparation, ML, and predictive analytics for finance. The 102-employee company provides predictive analytics services such as churn prevention, demand forecasting, and fraud detection, and they recently worked alongside PayPal. They claim that their predictive analytics software might help businesses with:
- Predicting the impacts of customer engagement for a particular direct marketing promotion in a retail environment using historical promotional engagement data such as customer information, their location, their responses to a promotional campaign or how actively they have been engaging with websites or apps
- Identifying and preventing fraudulent transactions for banks by monitoring of customer transactions and flagging transactions which deviate from a standard customer behavior, identified for each customer of the bank from data such as transaction history and the geographical locations of those transactions
RapidMiner claims that they can help businesses achieve the above results by leveraging the client’s historical enterprise data. For example, In predicting the impacts of customer engagement for a retail firm, RapidMiner would first have to work with the retailers marketing team to gather all historical promotional and transactional data, including any marketing flyers, in-shop promotions, and purchase histories for a particular product.
The data is then cleaned in order to mold it into a structure that can be plugged into the machine learning algorithms. Those algorithms then perform statistical operations such as regression, classification, and frequent item-set mining aimed at identifying patterns in the historical data. These patterns can allow for determining the effect of perhaps promoting hamburger buns over hot dog buns for a particular week.
The system then derives actionable insights by working with a retailer’s marketing and IT teams in order to suggest the potential best practices for new promotional campaigns. The marketing team can then create a dashboard based on these and other insights that provides them metrics and analytics related to decisions such as choosing which products to market in the coming week or to whom they should market based on past history. RapidMiner claims their software can learn more such patterns over time, improving the accuracy of its predictions.
Below is a 3-minute video from Rapidminer giving a brief demonstration of how their predictive analytics software can help businesses:
PayPal collaborated with Rapidminer to gauge the intentions of top customers and monitor their complaints. According to a case study from Rapidminer, Han-Sheong Lai, Director of Operational Excellence and Customer Advocacy, and Jiri Medlen, Senior Text Analytics Specialist at PayPal, wanted to gain a better understanding of what drives product experience improvement. They needed to analyze customer feedback in order to do this successfully.
The challenge for PayPal lay in the sheer number of customer comments they had to analyze. Rapidminer worked along with AI and data science engineers at PayPal to develop a system that could perform sentiment analysis for customer comments in over 150,000 text-based forms in several different languages including 50,000 tweets and facebook posts.
The RapidMiner platform was first used to extract the list of the most frequently mentioned words in every customer complaint from the dataset shared by PayPal. RapidMiner then worked alongside a team of software engineers from PayPal to identify the top two password and PayPal login access-related issues from the list, along with actionable insights on possible resolutions to the issues.
According to the case study, Paypal learned the login issues seemed to spike during November and December (holiday season) when users were more actively making purchases and instances of forgotten passwords were high.
RapidMiner claims they were then able to work with PayPal engineers to design fixes for the login issues. 2 or 3 weeks after integrating RapidMiner into their system, PayPal customers succeeding in recovering their passwords 50% more often than before the integration.
After 2 to 3 months working with the software, PayPal was reportedly able to classify customers as “top promoters” and “top detractors”. This enabled them to arrive at the top complaint areas (customer login issues).
Founder and President of RapidMiner Ingo Mierswa earned a PhD in Data Mining from the Technical University of Dortmund.
For a deeper understanding of the possibilities for AI in finance, read our comprehensive overview of the sector.
Healthcare
Health Catalyst
Health Catalyst in Salt Lake City was founded in 2008 and has around 565 employees today. The company claims to provide predictive analytics services specifically for the healthcare domain through their offerings Catalyst.ai and Healthcare.ai. The company claims they have been involved in several successful collaborations with hospitals and other healthcare companies in projects such as:
- Preventing hospital-acquired infections by predicting the likelihood of patients susceptible to central-line associated bloodstream infections
- Using machine learning to predict the likelihood that patients will develop a chronic disease
- Assessing the risk of a patient not showing up for a scheduled appointment using predictive models
For example, a hospital might use the Health Catalyst software to predict which of it’s patients is most likely to develop a central line-associated bloodstream infection (CLABSI) so that healthcare professionals can act much faster in such cases.
The hospitals historical Electronic Medical Record (EMR) data, along with Health Catalyst’s internal data warehouse records on historical CLABSI cases, can be utilized to gain insights on patterns that might lead to a higher likelihood of infection. A team from Health Catalyst might work alongside hospital staff to gather patient data and, using machine learning algorithms, coax out a CLABSI risk prediction model that is built into a dashboard. The nursing staff might use the dashboard to identify gaps in patient care that might lead to an infection for each patient.
The 2-minute video below from Health Catalyst gives an overview of some of the applications for their predictive analytics software:
Health Catalyst Analytics reportedly assisted Texas Children’s Hospital in predicting the risk of diabetic ketoacidosis (DKA), a life-threatening complication of diabetes, to allow care team members to intervene in time before patients suffered a severe episode.
According to the case study, Health Catalyst used data from a risk index for children with poor glycemic control who were recently diagnosed with type 1 diabetes to predict the risk of a DKA episode for each patient. This allowed caregivers to monitor high-risk patients more closely.
Health Catalyst claims their software lead to an eventual 30.9% relative reduction in recurrent DKA admissions per fiscal year, although how much of this was solely due to the analytics and how much might have been due to other healthcare measures taken by patients was unclear at the time of writing.
Health Catalyst claims to have worked in projects with customers such as Orlando Health in Florida, Piedmont Hospital in Georgia, the University of Texas Medical Branch (UTMB), Virginia Piper Cancer Institute among others.
Aaron Neiderhiser the Senior Director of Product and Data Scientist at Health Catalyst has earned an MA in Economics from the University of Colorado Denver and previously served as a Statistical Analyst with Colorado Department of Healthcare Policy and Financing.
We explore what AI can do in healthcare in broadly in our comprehensive overview: Artificial Intelligence in Healthcare.
Heavy Industry
Rockwell Automation
Rockwell Automation, one of the largest automation players today, offers the Pavilion8 MODEL PREDICTIVE CONTROL (MPC), which the company claims can analyze historical operational data from industrial manufacturing sectors, such as oil and gas or food and beverage, and predict future values for that operational data.
When compared with desired predefined targets for that data, Rockwell Automation claims their software can help these manufacturers automatically schedule the most optimized points in time to supervise a specific project.
For example, in their offering tailored to the oil and gas industry, Rockwell Automation claims their MPC software can help in maximizing the efficiency and stability of the natural gas liquid (NGL) fractionation process. The challenge in NGL fractionation lies in optimizing the composition of the various components in order to achieve specific quality.
An oil and gas company might use the Pavillion8 MPC software to help its maintenance engineers stay ahead of maintenance issues and improve the process efficiency in the plants. A team from Rockwell would first work with domain experts and IT personnel from the oil and gas firm to gather historical data from any existing sensors in the refineries.
The software has a browser-based user interface which can be used by the oil and gas company’s maintenance managers to monitor key plant variables, such as capacity utilization, and predict the most optimal composition control parameters for the process in terms of end-product stability and process efficiency.
Most industrial plants with any kind of automation in their processes have numerous sensors which collect data about pressures, temperatures, levels of vibration in machines, and so on. The MPC uses this historical data and real-time data from these sensors to find anomalies in plant variables by comparing them to data patterns during normal operating conditions.
The software then prompts the maintenance managers with reports on the anomalies along with a possible recommendation on what might have caused the issue and suggest replacement parts when required.
The 3-minute video from Rockwell Automation goes into more detail about their Pavilion8 MPC offering, specifically tailored for improving NFL fractionation efficiency:
Rockwell claims that their software can help oil and gas companies engaged in NGL fractionation to separate the NGL liquids into component streams of ethane, propane, isobutane, normal butane, pentane, and heavier chemicals in the following ways:
- Increasing process stability and reducing variation in quality of the end product
- Increasing the yield of NGL components by an avg. of 1 – 3%
- Reducing the reboil energy consumption by an avg. of 5 – 10%
- Increasing production capacity by an avg. of 3 – 5%
However, we could find no robust case studies or projects with marquee oil and gas companies on Rockwell’s website for their Pavilion8 MPC software, although Rockwell is one of the largest automation products and services providers in the world. We were also unable to find the data science professionals involved in the development of the MPC software in Rockwell.
Consumer Goods
Presidion (Formerly SPSS Ireland)
Set up as a regional office for SPSS in Ireland, Dublin-based Presidion now offers predictive analytics software for the retail industry in applications such as improving customer engagement, optimization pricing, inventory management and fraud detection to name a few.
Presidion’s Customer Analytics Solutions offering seems to be aimed at helping enterprises target the right audience and identify customer issues by uncovering patterns of buying behavior from historical data. For example, Presidion claims to have worked with Belgium’s second largest insurance provider, Corona Direct, to improve long-term customer profitability. The case study describes the following:
- To improve profitability, Corona Direct needed their customer acquisition campaigns to be effective enough for the first-year revenues generated from new insurance policies to cover the cost of the acquisition campaign.
- Corona Direct input historical customer acquisition data, such as that from promotional campaigns, into Presidion’s IBM SPSS software.
- Presidion claims their software helped Corona Direct’s marketers to efficiently create, optimize, and execute their outbound marketing campaigns by churning out a predictive analytics dashboard.
- The dashboard helped the marketing team at Corona identify customer groups that were more likely to respond to a particular campaign and to predict the most balanced growth targets for optimal profit margins.
- Presidion claims that Corona was able to reduce campaign costs and improve long-term customer profitability and eventually the cost of the implementation was covered by new insurance policies taken out within six months after the integration.
Presidion also claims to have worked with O’Brien’s Sandwich Bar in Ireland to assist with customer satisfaction, product development, and product marketing. O’Brien’s needed a way to track their customer feedback (which was being done through comment cards) more efficiently and to digitize the process. This led them to adopting Presidion’s predictive analytics platform.
The system was set up so that information from the comment cards was directly entered into Presidion’s SPSS-IBM Statistics and SPSS-IBM Text Analysis for Surveys. Presidion claims this change aided O’Brien’s in leveraging predictive analytics to ensure a fast turnaround time in identifying and resolving customer issues. The way they claim to have done this is described below:
- Each of their stores received a monthly report on their performance detailing the top issues that customers faced during that month.
- The information received from the comment cards was also used to inform the development of new products and campaigns.
Presidion claims to have worked in projects with companies such as Daimler, HONDA, and banks like Bancolombia and Rabobank, among others. However, we could not find any evidence of previous AI-related experience in Presidion’s leadership team.
Transportation
Dataiku
Dataiku is headquartered in New York and offers Dataiku DSS (Data Science Studio), which the company claims can be used effectively in many applications for air freight, sea freight, road freight, and passenger transport. According to Dataiku, their DSS software can aid in some of the following applications:
- Predictive Maintenance: Using vehicle sensor data (for cars or trucks), DSS can potentially help customers develop a predictive analytics solution, which can take this raw data and cleanse, format, and model it to predict which components might fail or not perform as required.
- Dynamic Pricing: Using Dataiku DSS predictive analytics, transportation businesses might be able to optimize the end-product costs based on real-time changes in operating factors such as fuel costs, security-related delays in shipments, and external factors, such as weather.
Dataiku’s software might help supply chain managers for a truck-based transportation company reduce the downtime that results when trucks break down. Dataiku’s DSS is used to create a data pipeline of both historical and ongoing maintenance data and the data from the electronic control unit (ECU) inside the trucks.
The software then parses the data automatically using machine learning techniques to identify patterns which lead to the failure of a particular part on the truck, such as when a defective or poor quality spare part is installed in the truth and leads to an engine failure during a delivery in rough terrain. DSS then provides insights that transportation maintenance managers can use to proactively order the right kind of spare parts for a particular issue in case of a failure.
For example, Dataiku worked alongside French company Chronopost, a member of the La Poste group, which provides express delivery services. Chronopost’s differentiation strategy revolved around ensuring the delivery of all parcels before 1 PM the next day, and with increasing scale, especially during holidays or festivals. The company needed a way to ensure that their delivery promise was met even during peak hours. According to the case study, Chronopost used historical internal delivery data and retrieval data (such as shipping data for each geography) to create a predictive model that continuously optimizes production costs and delivery times.
Chronopost claims they were able to ensure delivery of all parcels, even during peak post-traffic, after integrating Dataiku’s predictive analytics software. However, the study did not go into further detail.
The 14-minute video below from Dataiku explains how to use Dataiku’s DSS software:
Louis-Philippe Kronek the VP of Data Science at Dataiku earned a PhD in Operations Research from the Grenoble Institute of Technology, and the company claims to have worked in projects with companies such as Kuka, FOX Networks group, GE, Unilever, BNP Paribas among others.
Readers with a deeper interest in transportation may be interested in our complete article about AI applications in transportation.
Concluding Thoughts
Near-Term Impact
- Predictive analytics is being applied to many existing and new use cases across industries, especially in the healthcare, marketing, and finance domains.
- In the manufacturing sector, predictive analytics also seems to be leading more industries to adopt predictive maintenance best practices.
Takeaways for Business Leaders
- Predictive analytics requires the use of historical data which has to be cleaned and parsed before any analytics algorithms can be used to analyze the data.
- Predictive analytics also requires a great deal of domain expertise for the end results to be within reasonable accuracy levels and this would involve enterprise employees working alongside AI vendors or consultants.
A typical collaboration for an AI predictive analytics project might last around 2-3 months. Prediction results would incrementally become more accurate over time after the integration is complete.
Header image credit: metering.com