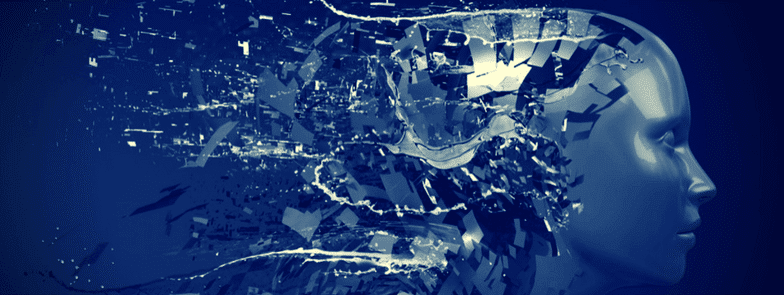
Machine learning offers an opportunity to leverage competition and new forms of collaboration in order to yield new products, services, and entire business models… but machine learning misconceptions run rampant.
In such a nascent niche, it’s helpful to gather expert consensus in how best to apply machine learning in business.
As a company, it’s tempting to see other businesses yielding tangible results from applying ‘machine learning’ technologies and to want to immediately jump on the boat. But good business sense also dictates not getting involved in a new venture before having done due diligence in understanding what a technology is – the potential capabilities and limitations, risks and rewards, and relevance in context to what a particular company produces or provides.
This is exactly why Emerj set out to ask the question:
“What do you believe to be the biggest misconception that executives and businesspeople have in applying machine learning to business opportunities?”
Tapping into our hundreds of interviews (on our podcast and otherwise), as well as reaching out to other experts in the field, allowed us to glean valuable insight from researchers and executives across the globe. We reached out to 30 experts and asked them to share their thoughts on this trending topic. Thirty of those participants responded to this particular consensus question.
You can see an abbreviated version of the answers to our “Deriving Value from Machine Learning in Business” question below in our full infographic.
Misconceptions in Applying Machine Learning: Expert Consensus
Note: The data for this graphic is no longer available publicly and are only available for Emerj research members. This change was enacted in November 2018.
Four categories emerged from participant responses, which were grouped based on similarities or parallels according to an overarching idea.
“Wrong expectations of capabilities” refers both to the misconception of the limitations of machine learning technologies, as well as to an underestimation of the possibilities that this technology can yield (as illustrated by the selected responses in the graphic). Perhaps the most common sentiment in this category is that machine learning can serve as a guide for finding solutions, but that it’s not a “magic bullet”, as termed by Ben Waber of Humanyze. A more pragmatic way of viewing machine learning technology: as a middle-of-the-road solution that’s not miraculous but far from useless. Amir Shapiro of Ben-Gurion University of the Negevood explains the concept this way – that machine learning is currently proficient at identifying patterns and making categorizations of data, but that there’s plenty room for innovation in integrating factors like “real-time control and motion planning.”
Danko Nikolic, a researcher with CSC and the Max Planck Institute, reminds us that machine learning systems are wonderful tools, but that’s all that they are at present. In other words, machine learning systems are not autonomous entities sometimes sensationalized by the media, and they won’t be for the foreseeable future. Anima Anandkumar of University of California at Irvine reinforces this point by reminding executives that machine learning tools present valuable opportunities for collaboration between machines and human, but they are there to augment and expand our knowledge, not to replace us. Joscha Bach, a researcher at Harvard and MIT, expands on this idea, describing the machine learning systems that we’re creating as new systems or paradigms within which to live and work, as opposed to creating separate “intelligent” entities.
A final but important point gleaned from this pool of responses is that many businesspeople also make a mistake when they sell machine learning short; in other words, there exist many more opportunities to use different kinds of data in innovative ways, as voiced by Patrick Ehlen, head of Deep Learning at Loop AI Labs. This idea was recently demonstrated in creative fashion by the technology and consulting firm Accenture, which created an AI-inspired symphony with the explicit goal of attracting the attention of business investors in the untapped possibilities of machine learning and artificial intelligence technologies.
Fewer responses fell into the bucket of technical misunderstandings, but these responded tended to be more diverse in content. Perhaps one of the most pertinent insights, shared by Professor James Hendler, is that it’s important to remember that results gained from machine learning and predictive analytics tools are correlative by their very nature and not causative. Other valuable perspectives include knowing what matters in the analysis of data (most researchers stress size and quality of data set over algorithm choice), and staying abreast of what machine learning is and is not – this is common sense, but it can be all too tempting to pass on the responsibility of knowing how the technology works to the next “guy” down, and in the end be misinformed or even misled by the “experts.”
We close by again driving home the importance of datasets; it’s a point that some may get tired of hearing, but most researchers will argue that having clean, relevant data is of paramount importance in designing and choosing the “right” machine learning model. As AI Expert Steve Omohundro states, a poorly-trained machine learning model can have blind spots, or simply be inaccurate.
Keeping educated and taking best-practice steps from the beginning when implementing machine learning is essential for the executive and company that does not wish to waste valuable assets. It’s inevitable that mistakes will be made, particularly when first implementing machine learning solutions, but having as good an understanding of the technology and as clear a roadmap from the beginning helps ease the transition and opens more quickly doors for innovation.
Related Emerj Articles:
If you liked this article about machine learning misconceptions, you might also enjoy some of these other fundamental machine learning articles from Emerj:
Image credit: IT Gurus of Atlanta