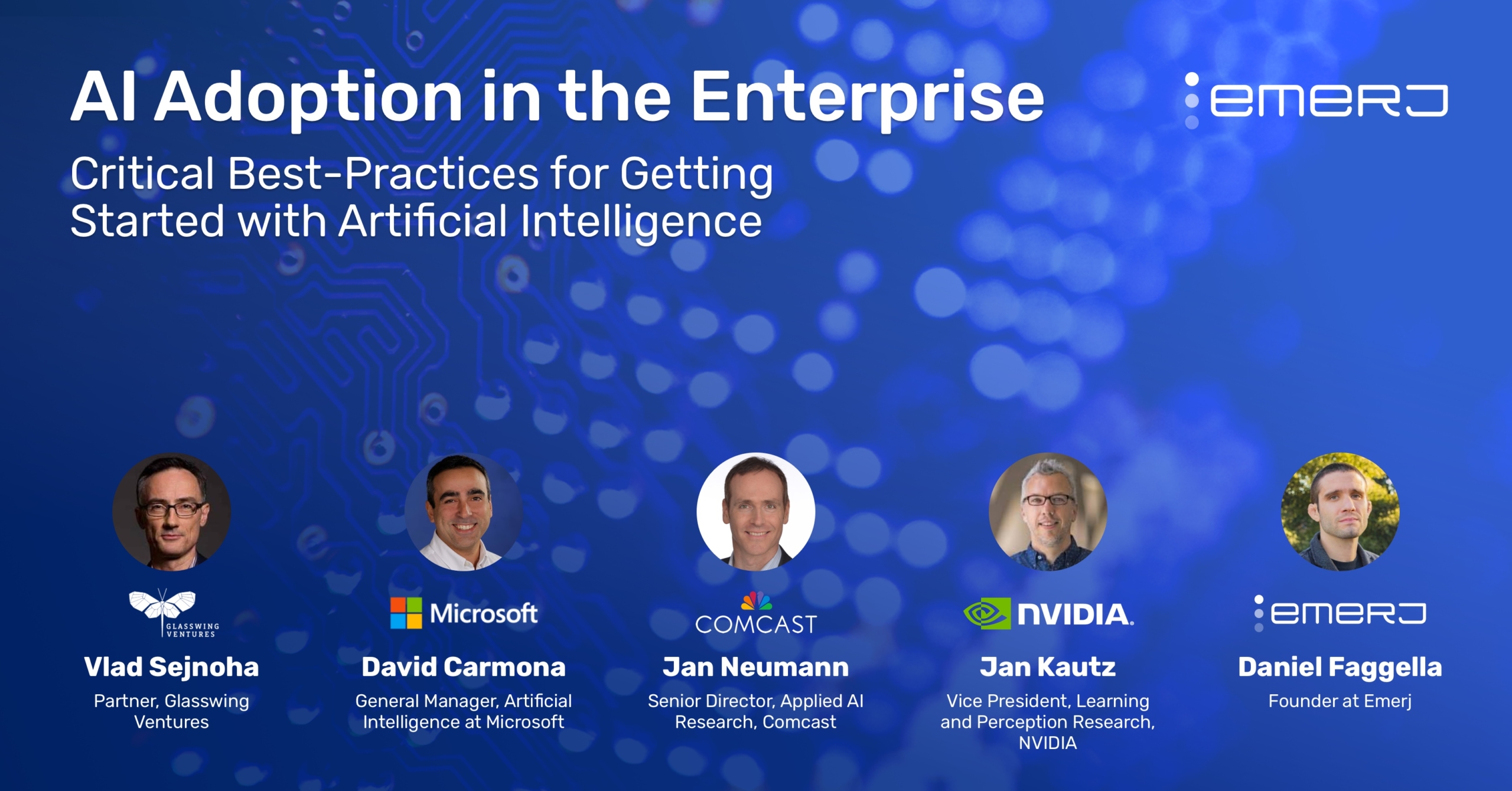
Although there are established use-cases for AI applications in the business world, the claims that AI vendors make about returns from their software are often exaggerated. What is also not apparent amongst the AI hype is that adopting AI and machine learning is far more challenging than it might seem.
News media and vendors sometimes portray AI as a magic “fix-it-all” solution, which it is not. In reality, it’s difficult for businesses to determine a clear measure of tangible returns from AI adoption.
Knowing whether or not to adopt AI is one of the key challenges business leaders face today and in the future.
In this article, we delve deeper into how businesses need to think about AI adoption in order to successfully develop data competencies and what critical best-practices business leaders need to implement to get started with artificial intelligence.
We spoke with four experts on the state of AI in businesses and how they can integrate artificial intelligence and machine learning into existing workflows. We also try to list out how business leaders can avoid some of the common pitfalls associated with AI adoption.
We break down the key insights from the conversations we had with these four experts on our AI in Industry podcast, including discussions about:
- Setting Executive Expectations About AI Adoption
- Picking an Initial AI Project
Special thanks to our four interviewees:
- Vlad Sejnoha, Partner at Glasswing Ventures
- David Carmona, General Manager, Artificial Intelligence at Microsoft
- Jan Neumann, Senior Director, Applied AI Research, Comcast
- Jan Kautz, Vice President of Learning and Perception Research at NVIDIA
You can listen to our full playlist of episodes in our “How to Get Started with AI – Best Practices from 4 Industry Experts ” playlist from the AI in Industry podcast. This article is based in large part on all four of these interviews:
Subscribe to the AI in Industry podcast wherever you get your podcasts:
We’ll begin our analysis of how businesses should approach AI adoption and go about their all-important AI adoption strategies. These decisions will likely impact how they invest in and deploy artificial intelligence to stay competitive.
Setting Executive Expectations About AI Adoption
AI is Not Like Regular Software
Business leaders should understand that they need to fundamentally change the way they think about these projects compared to traditional software automation. Implementing a software automation project that does not involve any AI is usually far easier and takes a lot less time.
Machine learning initiatives are time- and resource-intensive. They generally require vast amounts of very specific kinds of data that the business may or may not have already collected.
They require time from both data scientists and the subject-matter experts that inform their work; a business needs to be okay with taking subject-matter experts away from the routine ways in which they make money for the business to instead collaborate with data scientists to build an AI model. Despite all this investment, the ROI of any given AI project might be minimal in the short term, if there is one at all.
Kautz had the following advice for business leaders adopting AI:
You need to know that going into this is not just buying off the shelf, but you have to invest in data infrastructure and hiring data scientists to look at the specific problem you are trying to solve. The difference from excel spreadsheet modeling to AI is that you will have to hire data science experience and look at that specific problem
Sector-Specific AI Understanding
Businesses also need to conceptually understand what kinds of problems AI can solve. There are several approaches to artificial intelligence and machine learning, including natural language processing, computer vision, and anomaly detection. All of these have their own specific use-cases in industry.
Once a business leader understands what’s possible with AI, they can identify business problems at their organization that AI could solve. Sejnoha adds:
There are a lot of AI applications in the market. It is very important to choose the appropriate applications that attempt to solve business problems with sufficient technology and provide value in measurable ways. Businesses have to consider the overall organizational readiness for embedding new technologies in existing workflows. Finally, businesses must have adequate and relevant data for training machine learning algorithms for accurate outputs.
One of the best ways to research AI in a given industry and learn about case studies from well-known AI vendors and large companies with AI initiatives is to read our coverage of different spaces:
- AI in Healthcare – A Comprehensive Overview
- AI in Banking – An Analysis of America’s 7 Top Banks
- AI in Finance – A Comprehensive Overview
Clarity on Goals
The experts we spoke to seemed to agree that setting a long-term AI objective is critical for success. At the same time, business leaders need to understand that any “reimagining” of business workflows through AI is an enormous project with varying rates of success.
Businesses need to focus on gaining AI capabilities first and then use them as a barometer for defining long-term goals. Jan Kautz agrees that if the goal is upskilling the team’s understanding of AI, businesses should start with small projects and pick an area where a traditional software solution exists:
If you would like to make sure that people in your team gain some competency in AI it makes sense to start small, so don’t overhaul your whole fraud detection example. Pick something where you already have a reasonably good existing model for so that you can at least compare the results of the AI model and know if you are going in the right direction.
While achieving these long-term goals is not an overnight process, the way to approach them is to start with small AI projects that are aligned with the kind of long-term AI skills that a business wants to gain.
Vlad Sejnoha discussed how business leaders could approach goal setting when it comes to AI. In his opinion, this can be done in three phases:
The first: business leaders might need to gain at least a conceptual understanding of what AI can do. With this context, they can then bring in AI consultants, not to develop any AI projects, but to identify opportunities in their businesses. Then, in the third phase, leaders need to identify what the requirements are for such AI projects. They must ask questions like, ‘what kind of data is needed? Do we have access to this data, are we capturing the right kind of data from customers and in the enterprise?’
Ways to Organize AI-Compatible Teams
Once businesses have understood what AI can do and have aligned those capabilities with their business goals, the next step is to assemble data scientists and subject-matter experts to create multidisciplinary teams. Subject-matter experts are employees with a deep understanding of business processes in a particular function or division.
Usually, assembling such a team is more expensive than traditional software projects. The budget allocation for such projects usually needs to be approved by the COO or someone in the C-suite. Organizing such a team of data scientists might involve the following steps:
- Ensuring that the data scientists working on the solution are clearly aware of the business problem AI is being applied to. This will help give them context on how much and what type of data they need, as well as what other team members’ skills might be required for the project.
- Subject-matter experts need to identify the business problems that need to be solved. Then, data scientists might be better suited to identifying if AI can solve that particular business problem.
- AI projects are not a one-time investment. When businesses generate new data, the algorithms might need to be fine-tuned in order to incorporate the additional data and still maintain accurate results. Maintaining and updating the AI systems is a necessity, and business leaders need to assemble teams that can accomplish this task even when the project is largely developed and deployed. Again, this process is not one that involves only data scientists. Just like with the development of AI systems, maintaining and calibrating these systems to improve their accuracy also requires inputs from subject-matter experts and other team members.
Carmona provides a simple way for business leaders to understand how to think about giving data scientists business context:
If you ask a data scientist in your organization, what are you being paid for? The most common answer you might receive is probably: “I’m paid to deliver accurate models.” This is a person should ideally say I am paid to reduce workload of customer service executives by X percent or increase customer acquisition by Y percent.
While businesses might easily allocate new AI system-building tasks to freshly hired data scientists, they also need to find a way to redeploy the time spent by subject matter experts in their current tasks in order to incorporate their inputs in AI projects.
This might involve restructuring their work schedule and incentives or hiring external subject-matter
expert consultants for the project. However, if the subject-matter consultants are temporary, their services might be required again when the system requires any updates or fine-tuning.
Carmona seems to suggest that the best way to ensure that AI projects are returning value both in the short- and long-term is to automate a lot of the internal processes involved with machine learning:
The best practice here is automating the life cycle of AI projects. This means making sure that from the moment data scientists create a model to when the model is packaged and deployed into production needs to be automated. In essence, the mean time to resolution (MPTR) ideally needs to be in minutes and not in the order of days.
Data and Data Infrastructure Considerations
While we have spoken about all the essential human components needed to successfully adopt AI, none of these steps are worthwhile unless they are built around an overall data-centered strategy. Data is what makes AI projects run, and this data needs to be cleaned, parsed, and tested before it can be used.
Reimagining how a business is collecting, storing, and managing data is a decision that needs to be made after gaining a certain level of data competency.
Once the data being tested in a pilot is measurably valuable as a proof of concept, businesses can think about the phase in which the entire data infrastructure is overhauled. Below are a few pointers on what business leaders can expect when it comes to data and data infrastructure management in AI projects:
- Businesses will find that accessing data is usually harder than anticipated. Data might be stored in several different formats or might be stored in different geographical locations that have different data transfer regulations.
- Even the data that is accessible immediately is usually not stored in a format that makes it easy to use. The data will often require heavy reorganizing and sorting in order to format it, and, in some cases, purging it.
- The storage hardware for this data might also need upgrading. In addition, businesses might also have to rethink how they are collecting data currently and what new infrastructure might be required to implement AI sustainably
Jan Neumann adds:
Its not just the algorithm that is making AI projects work, it is also the data is being used to train the algorithm and is also part of the eventual outcome. To successfully do AI and ML, you have to make sure that you data pipeline is given the same level of care and attention traditionally given to software development pipeline. This is something that is often unclear for business leaders
Picking an Initial AI Project
Start Small, But With A Long-Term View of AI Skills
AI projects also involve several individual steps that might take days or weeks to complete. In essence, there are several advantages to starting small:
- Small projects can help businesses focus on building skills rather than looking for outright returns immediately. AI projects are technically challenging and require large amounts of initial capital to deploy. They will need two to six months to build, and even then there might not be a successful result in some cases. Starting with small pilots allows businesses to know which AI skills seem to be working and which aren’t valuable.
- Small projects might not require total data infrastructure overhauls in order to successfully test and deploy. For instance, deploying a chatbot might not require a business to overhaul their entire data infrastructure, and yet it will give them a point of entry into AI. Businesses can keep small AI projects in a closed loop in terms of internal data flows thereby not disrupting existing processes.
- Small projects can help build confidence in data management capabilities
Gaining confidence in working with data will help continue the development of future AI projects in the future, and gaining data competency as a critical capability will permit businesses to stay ahead of the competition.
Carmona also reiterated the importance of splitting AI objectives into short and long-term goals. He adds a crucial insight in terms of profitability in the initial phases as well:
Businesses need to think about AI in two ways: tactical projects on one side and strategic initiatives on one side. An overall long-term goal also needs to be established, which represents the size of opportunity, such as increasing revenue by a certain percentage or improving customer acquisition numbers. In the short term goal might be similar to increasing revenue for a particular division in the organization or reducing the workload for customer service. Sometimes the ideal first smaller project might not be the most profitable immediately, but the ultimate goal should be the bigger long term bubble.
Developing AI In-house Vs Working With A Vendor
A likely decision that business leaders might have to make when they decide to get into AI is whether to build a system or to work with a vendor’s solution.
In this section, we address the relative advantages and disadvantages of buying from an AI vendor and building AI solutions in-house. The question of whether to build or buy can be assessed based on the following factors:
Building an AI Solution In-house
Organizations that have access to the right kind of data, existing automation efforts, budgets and have identified that deploying AI is needed to stay competitive will likely be incentivized to build the application in-house. For instance, in applications such as fraud detection in banking, AI solutions might be required to combat the more sophisticated cyberthreats.
Businesses that don’t have access to historical data records might need to start collecting the data now in order to build the solution in-house. Businesses that identify a business problem AI might solve, need to first analyze if there are any existing vendor solutions for that challenge.
Leaders need to be careful to identify only vendor solutions that solve the exact problem in their own industry preferably. This is because vendors are very likely overestimating the capabilities of their software to suit any problem. If a vendor solution does not exist, business leaders need to know that this might be an experimental use-case that comes with new challenges that even consultants might not be able to help with.
Working with an AI Vendor
Businesses that are entering into small AI projects that might not drastically overhaul their bottom lines might be more likely to work with vendors. Sejnoha suggests this would give them the opportunity to transition into an AI project without really needing to disrupt any of their existing business workflows too much:
You dont have to transform the enterprise before you know where you are heading overall. You require a lot of data for AI projects. Identify of the data assets that you have access to what is good small pilot test that can help you understand what is possible and what is not.
Businesses that do not have access to large volumes of historical data might benefit from working with vendors that provide pre-trained AI solutions that might be helpful in cases where businesses have little or no access to large volumes of training datasets.
Finally, businesses looking for an immediate return on investments or those applying AI to problems that need rapid resolution might be better off working with a vendor that has a strong track record of solving that exact business problem.
Smaller projects and projects that are less time-sensitive can be successful if developed in-house. The additional time can be effectively used to develop and test a robust solution in-house.
Related Articles
- Buying and Adoption Readiness for AI (AI Zeitgeist 5 of 7): This article looks at how the process of buying artificial intelligence solutions will change in the years ahead.
- Machine Learning Still Getting Sea Legs in World of Midsize Business – A Conversation with Charles Martin: In this podcast, we talk to Charles Martin, PhD, about the areas in AI that he believes are ripe for implementation in a business context, and where he sees businesses getting AI ‘wrong’ before getting to the hard work of implementing systems that work for them.
- How to Determine the Best Artificial Intelligence Application Areas in Your Business: Amazon’s Madhu Shekar details what the early adoption strategies might be for machine learning for customer service, marketing, manufacturing and other applications
- Enterprise Adoption of Artificial Intelligence – When it Does and Doesn’t Make Sense: Readers of this article can gain a better idea of AI’s current applications and limitations – and most importantly – a framework for thinking about where AI might have promise in their businesses.