Episode Summary: This week’s episode of the AI in Industry podcast focuses on two main questions. First, how should business leaders determine the most fruitful, potential applications of AI in their business? Second, how do they choose the right one into which to invest resources?
This week, we interview someone who has spoken with a number of CTOs and CIOs about early adoption strategies for machine learning for customer service, marketing, manufacturing and other applications. He is Madhusudan Shekar, Principal Evangelist at Amazon Internet Services.
Subscribe to our AI in Industry Podcast with your favorite podcast service:
Guest: Madhusudan Shekar, Principal Evangelist, Amazon Internet Services Pvt. Ltd.
Expertise: Cloud, mobility, analytics, artificial intelligence, internet of things
Brief Recognition: Madhusudan Shekar is Principal Technical Evangelist at Amazon Internet Services Private Limited (AWS) and has been working on cloud computing technologies since 2008. He has worked with companies of all sizes to help them adopt and be successful with AWS Cloud. He has over 20 years of experience in developing large scale systems for telecommunications, media, automotive and financial services industries.
Prior to AWS, he built the fleet management system called TATA Fleetman for automotive original equipment manufacturer TATA Motors, as well as the third-party application programming interface gateway for enterprise smart payment systems at Ezetap. Shekar graduated from the University of Madras.
(Note: This interview was part of a broad series of interview with executives and Indian government officials, focusing on the economic impact and opportunity of artificial intelligence in India. That full report is available online, titled Artificial Intelligence in India – Opportunities, Risks, and Future Potential.)
Interview Highlights
(2:43) You speak with developers and business leaders, encouraging them to adopt some of Amazon’s machine learning (ML) capabilities. What ML capabilities do you see making their way into enterprises?
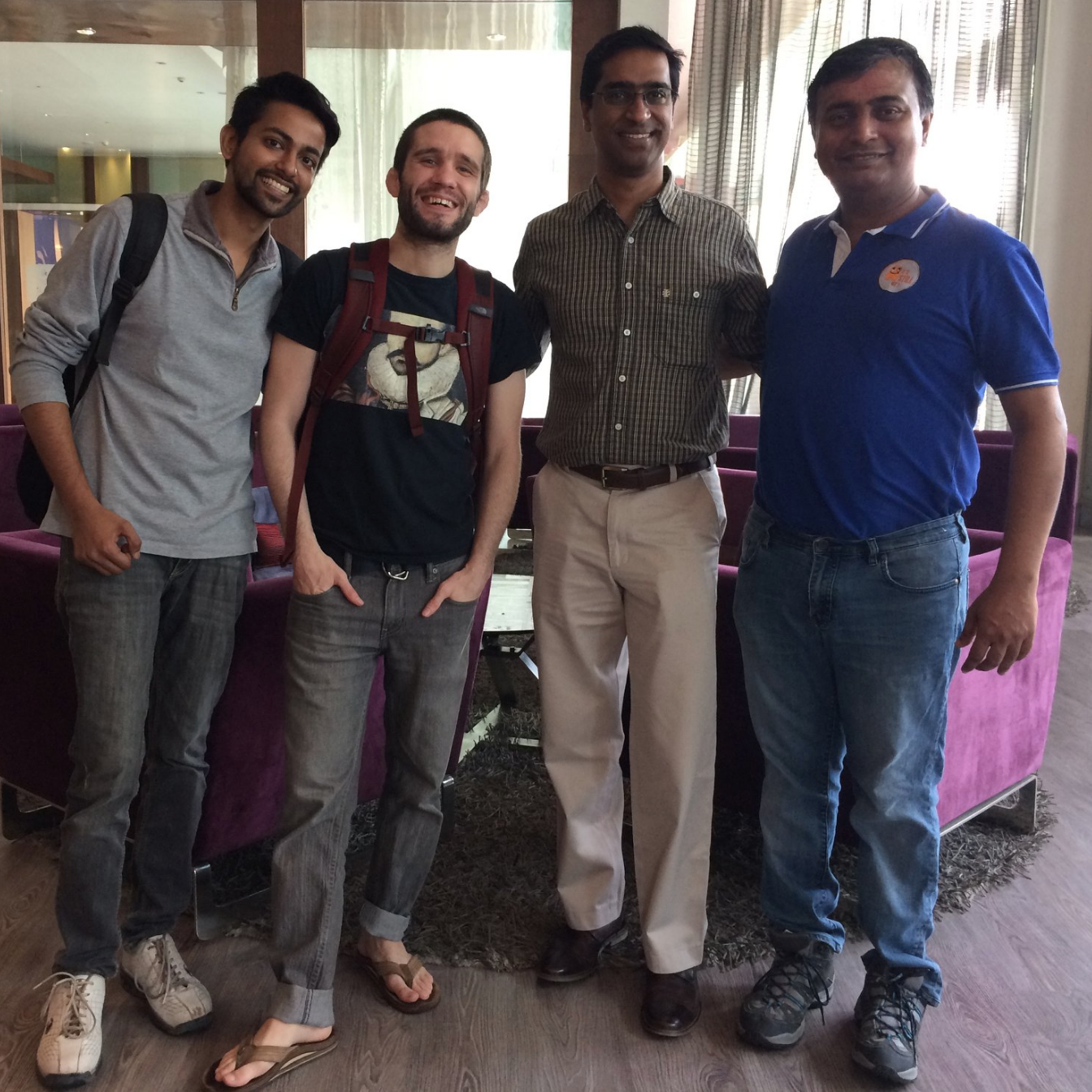
Madhu Shekar: When we look at the challenges that enterprises face, whether it is customer service, sales and marketing, quality control—these are the areas I see ML in. For example, we look at companies using machine learning in customer service or manufacturing companies looking at sales and retail execution. These are the pain points where they can use machine learning.
Machine learning could be the most powerful tool that can take companies to the next step. I always focus on identifying areas where they have competitive advantage. What is the next level? That’s the area where you have the deepest strength. Because machine learning requires a huge volume of data, determine the areas where you have the best—not the most—data and start building your competencies and mechanics.
Once you have achieved that, you can start expanding that capability into other areas of the business. If you are a fast-moving consumer goods company with a great amount of data on retail distribution or consumer buying behavior, focus the machine learning in that space.
(5:20) I interviewed someone that said prioritizing efficiencies may not be the best way to apply ML, and here you are saying “think about competitive advantage first”. Not merely starting small by automating some processes. You are saying, “let’s see where the business is going and let’s take ML to make that leap forward.”
MS: If you have an advantage over your competitors in the same area, applying machine learning could potentially give you a generational advantage. To do that requires a lot of clean data.
(6:40) When people come up with ideas for their business, what advice do you give them to help them achieve these advantage areas? How do you prompt them to look in the right direction?
MS: When I talk to business leaders at the C-level, I ask them to understand why they think they have an advantage. If you are building a new business opportunity or a new model, what is it that you want to do with this? How are you collecting data? What kind of data are you collecting? Then I take them to the next step and start building machine learning models.
Some of these conversations can go differently. For example, one executive asked “do you want to get started with machine learning because you want to achieve advantages in, for instance, customer services or sales where they want to use ready-made solutions such as Amazon Polly or the Amazon Lex chatbot engine. These are ready-made services that you can consume and quickly deploy to get the results that you want.
Another way is to look at areas that offer competitive advantages. What reliable data set do you already have? Is it your manufacturing data? Is it customer data that you are confident can bring results? How do we take advantage and support that in the machine learning process?
(9:45) Understanding what kind of data is currently available data seems to be an important first step in ML and to finding the best application. Do the exercises you conduct with business leaders emphasize how the importance of organizing data because it is the foundation of their advantage?
MS: When a business faces challenges in data organization, we start looking at the data lake to determine what is clean and unclean data. Once the data is cleansed, the business now has the ability to process it. Put the data in a single space and define schema as you start building and reporting from that.
Machine learning, for all the coolness it is known for, first is about data. Eighty percent of the work in machine learning is getting the data organized, structured, trustworthy. Because the machine is now going to make decisions for you.
(12:39) Three years from today, what kind of themes do you see around treating and handling data? What other things do you hope to see more of so enterprises can adopt machine learning faster?
MS: I think there will be more data lakes. Businesses will start to structure data to make it as less expensive as possible. That’s what Amazon business is all about: being able to store data at $27 per terabyte. The goal is to be the most viable per dollar. Once this is set, the next step is to build the data pipelines to process and cleanse the data, and make it available across the entire organization. Democratizing the data gives the business the capability to use the data as quickly as possible and benefit from machine learning every day.
Each one is going to make that data better for their respective business use case. Does the quality person need access to some of the customer data? That’s the kind of feature you want to build as well to avoid data leakage. You also want to have data security.
One of the things we recognize in Amazon is data patterns. If someone is accessing the data too frequently when they should not, the organization needs to be alerted. Amazon Macie looks at the packets of data, analyze it in the back end to determine patterns and raise alerts. Your data lake should have these capabilities that democratize on one end and control on the other.
After this, comes the next step. You will have layers of data: raw customer data, first-level cleansed data. A product manager will build a particular cohort and market segmentation of the data suitable for a specific use case. Someone will build another off that cohort, build hierarchies of these dependencies, evolving through a natural progression. That is the organization’s data platform. Now you start building machine learning on top of this.
Simple binary classifiers, multiclassifiers can solve a lot of issues. A lot of things can be solved using traditional algorithms. Build models on top of that and take it to the next step. Specific business use cases have tremendous returns. Having the wrong data set and processes can be more expensive than the investment itself.
Header image credit: Smartdatacollective.com