Chances are you have already been bombarded on social media or in your inbox about all these “revolutionary AI” this and “game-changer AI” that.
Vendors of AI technology are often incentivized to make their technology sound more capable than it is – but to hint at more real-world traction than they actually have. Yes the broader dynamics of how AI can be applied in business are rarely discussed in depth. In this article we’ll take a “helicopter view” of AI’s progress (and sometimes, lack of progress) in enterprise, and whether or not adoption is the right thing for your business.
This article is based on a presentation delivered by Daniel Faggella, Emerj CEO, for the Technology Association of Georgia’s data science event in Atlanta. The findings and opinions in this presentation are based on interviewing and surveying hundreds of AI company founders, and also dozens of interviews with CEOs of hands-on AI consulting companies.
The short story is that AI is being hyped, and that its potential in business is still for the most part experimental. Though there is a genuine sentiment that AI will transform industries, the actual applications and projects that will deliver that value is mostly unknown, and business leaders need to think strategically about where AI may – of may not – make a difference for their company.
By the end of this article you’ll have a better idea of AI’s current applications and limitations – and most importantly – you’ll have a framework for thinking about where AI might have promise for your company.
Below are the slides from the presentation, and the article below goes into more depth about the state of AI in the enterprise, and important considerations for AI adoption.
The Relevance of AI for the Enterprise
The reason it is important to look into AI in terms of business use is that it is very possible that it will likely overhaul entire industries in the next 15 years. Industry researchers do not always come to a consensus, but the one thing they do seem to agree on is that AI and machine learning will – in the decades ahead – drastically change how business is done (you can hear hundreds of our interviews with researchers on our AI podcast).
Through either automation or augmentation, it seems clear that AI will alter “work” as well know it, and much like the internet 20 years ago, AI is a technology factor that must be on the radar of business leaders who are considering the long term.
People with a rudimentary familiarity with AI will not be surprised to learn that artificial intelligence applications are not necessarily a novelty of the 2010’s. AI’s first push into the business world (famously through so-called “expert systems”) began in the 80s, and primarily consisted of hard coding human intelligence into rules and steps.
This strategy succeeded in building some fruitful applications, but it could only go so far and interest and development into it eventually died off (in a period of limited funding and enthusiasm often called the “AI winter”).
With machine learning came the resurgence of AI where, instead of hard baking human knowledge into a technology system, machine learning uses data from the real world to train computer “neurons” to predict outcomes and detect patterns in the future (for a more complete definition see our short “defining machine learning” article).
The most important difference between AI then and now in terms of business adoption is that back in the 80s and 90s, the top businesses in the world were not predicated on artificial intelligence.
Now they are.
You only have to look at today’s most profitable and successful companies to see that this is the case:
- Amazon – A business dependent on recommendation engines built on machine learning and massive piles of user data about products and purchases.
- Google – Built on its search engine, arguably the most ubiquitous and meaningful application of artificial intelligence the world has ever known.
- Facebook – Build on it’s news feed and ad network, structured to maximize user activity and advertising engagement based on trillions of data points and behaviors.
- Netflix – A business dependent on recommendation engines built on machine learning and massive piles of user data about movies and media.
- Apple – While currently seen as a comparative laggard in AI research when compared to its tech giant peers like Google, Apple made voice interfaces mainstream with the advent of Siri in 2011.
These tech giants would not have become what they are today without machine learning, and AI’s impact won’t just be relegated to public tech giants.
Business leaders today should have an understanding of how and when AI might be relevant for their companies (and that’s often not yet). The goal of the rest of this article is to “prime your antennae” to detect the relevant and trustworthy trends of AI’s applications, and to sift out the hype and overblown claims.
The State of AI in Enterprise Today
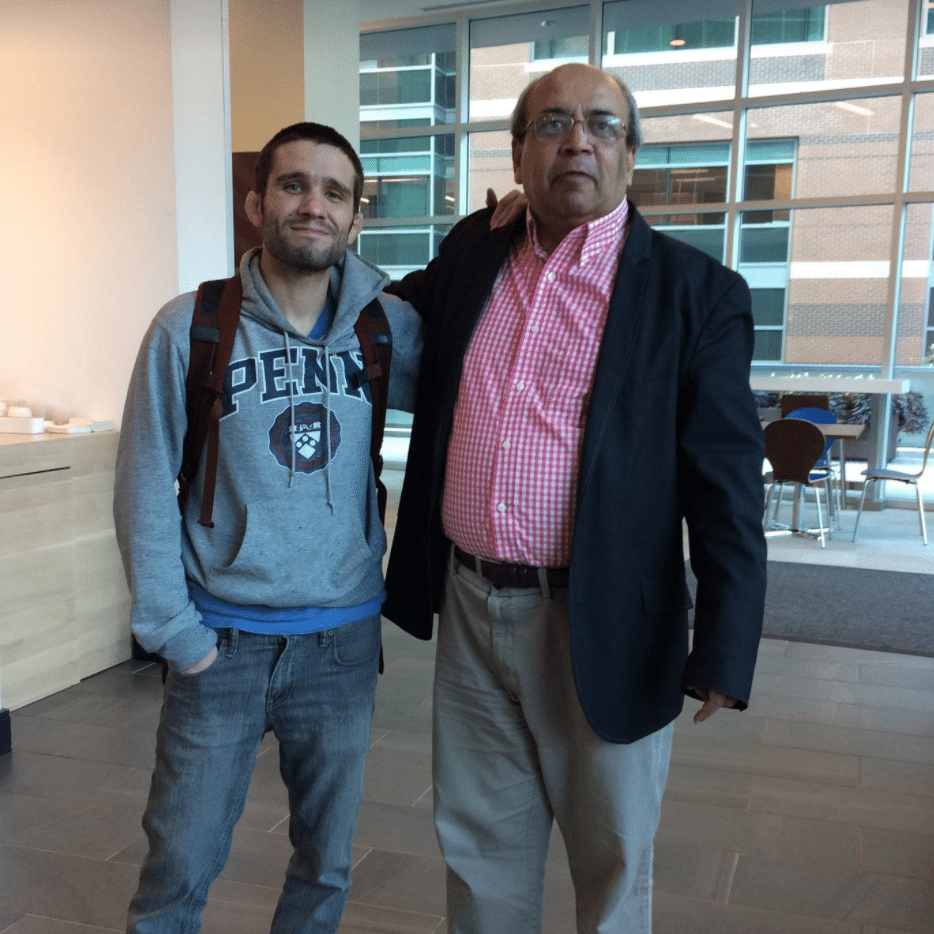
What is the state of AI enterprise now? If you read your social feed you’ll get the idea that if you’re not doing AI in everything, you must be a dinosaur company.
Actually, that is not the case at all; at least, not yet. It is not that it is a good thing if you don’t have AI in your business (or data science talent to draw upon), but if you don’t, you are not alone. Most early AI applications are only beginning to be tried in business, and clear business ROI is still relatively rare for most AI applications.
It’s Mostly Pilots
Most AI applications in the enterprise are little more than “pilots.” Primarily, vendor companies that sell marketing solutions, healthcare solutions, and finance solutions in artificial intelligence are simply trying stuff.
At Emerj we often research hundreds and hundreds of AI companies in a given sector (as we have done in marketing, in healthcare, and in other industries).
In any given industry, we find that of the hundreds of vendor companies selling AI software and technology, only about one in three will actually have the requisite skills to do artificial intelligence in the first place. In other words, only one-third of AI companies have somebody with the legitimate training and requisite mental capacity — whether by education or working for a company such as Google, Apple, or Facebook — for developing machine-learning systems.
Furthermore, our research shows that of those companies with the capacity to produce machine-learning systems, only one-third again are past the stage of “piloting” their products or services. They are at a point of somewhat legitimate traction with their application where it really seems there may be some return on investment for their clients, or are even further along, and are actually selling a real, problem-solving solution to businesses.
All in all, only one in 10 AI companies you might encounter will have something in the AI universe that will have a positive impact on a business.
Avoid “AI Toys”
Another common mistake among AI adopters is going in for the wrong reasons. When businesspeople see their inbox is full of artificial intelligence coverage, and – for example – they see that a competitor in Asia just did a press release about creating a chatbot application, then there is a kind of social pressure to say “let’s do AI, too”. It is AI for AI’s sake, and that makes it a “toy” application.
“Toy” applications are technologies or projects taken on because they use AI, not because they solve a business problem. Vendors play into this because they need guinea pigs to “pilot” products, and they’ll sometimes encourage closing deals even if they are not well organized.
You know this is happening when the question becomes “What can we do with AI?” rather than “How can we best use our resources to meet our business goals?”
Coming up with ways to use AI because other people are doing it makes it a “toy” application because it is not really the best use of your resources. It takes a lot of time, training, and tinkering as well as specialized skills, and often goes nowhere. Even if there is progress in adoption, chances are halfway through somebody at the top realizes it is not the best use of available resources.
When it comes to “toy” applications, whoever championed the AI adoption in the first place (for the wrong reasons) ends up looking a more like a fool than a champion, and the consultants and stakeholders involve all end up disappointed.
The Biggest Challenges to AI Adoption
There are some significant challenges in adopting AI for enterprises, most of which is structural. AI and machine learning are not turnkey solutions, at least not yet. They are not plug-and-play, so adopting it to your business requires a particular set of skills and resources. There is still some “wizard skill” involved.
The AI Talent Gap
The first challenge is finding talent. You need people on your team with the requisite technical ability to train AI systems, such as using customer support data to automate answers, or marketing data to refine and define your campaigns in the future. This type of training is a nuanced skill, and not a learning-over-the-weekend type of ballgame. Unfortunately, the number of folks with those skills is relatively few and far apart.
Cultural Challenge of Bringing AI into Existing Businesses
However, while talent is certainly a major challenge, it is not the preeminent challenge in bringing AI into an enterprise. The preeminent challenge is the structural challenges, which involve research and development to adopt it to an actual enterprise environment.
Most enterprise IT procurement more or less to some degree is simply choosing software or hardware that you want use, plug it in, and eventually get it to do the things you want it to do. The trouble with AI is that there is a lot of initial training and working with data in the early days that you have to do so it will work with your existing system, and calibrate it in order to deliver the result.
Integrating a very large and robust artificial intelligence solution in business involves a level of R&D that is just not something most companies can bear psychologically. They never had to do that kind of long tinkering in-house to make technology work. There are some serious costs involved in AI adoption that most companies are simply not equipped to bear.
Using Data Scientists – and Keeping Them Around
In addition, companies that use AI without thoroughly understanding what it can do often underutilize it, even if they can afford the talent in the first place.
If a data science team is hired in order to help solve business problems, it’s unlikely that the teams they engage with will know how to work with them. For example:
- A marketing team may not know what kinds of marketing problems AI can solve, so they never talk to the data science team
- A business intelligence team may have many misconceptions about AI, and after being frustrated with how hard it is to get the data science team to solve problems (mostly because the business intelligence team doesn’t understand the iterative process required to use AI), they stop making suggestions altogether
- When the data science / AI team does create an AI solution (after long periods of tinkering and research), the IT teams aren’t familiar with how to integrate this new solution into its regular workflows, further frustrating all parties involved
The additional downside of having frustrating communication channels is that a bored data science team probably isn’t going to stay around very long. Data scientists can get jobs (and great salaries) wherever they go, and there’s almost always someone willing to pay more.
We’ve heard many times (including in our interview with Equifax’s Chief Data Scientist, titled “Building and Retaining a Data Science Team”) that without having meaningful, useful, interesting projects to work on – they’ll probably be sending their resume to other potential employers (or simply responding to the recruiters who are already emailing them daily).
Examples and Use Cases
While AI is a tough nut to crack for many industries, there are some areas with a good deal of traction in machine learning in AI in industry already.
Below, we’ll explore some broad business functions that are integrating AI more and more, with existing enterprise traction.
Cyber Security
A key area where AI is way past the pilot stage across industries is data security, because security is important for everybody. Traction exists in a robust form in that area and people believe there will be significantly more traction in enterprise across industries.
Machine learning is peculiarly suited to data security. Hard coding rules to detect when somebody is hacking into your stuff is very challenging because it is adversarial. It requires a fluid approach.
To illustrate this, consider marketing. People using AI products for marketing are not training the systems the wrong way to sabotage the process. In data security, there are large teams of very smart people who are working to bypass what you are doing. The ability to detect anomalies and detect behaviors or accesses that are a bit different is something AI can handle. In the next two to five years, most people in enterprise that works in security or sells into that space will think you are not doing security if you are not in machine learning.
Additional context:
- See our full article titled “Artificial Intelligence for Security Applications”
Customer Service and White Collar Automation
Two other areas that are reasonably common across industries today is customer service and white-collar automation. In customer service, you have the usual support Q&A, technical support, and ticket routing, where automation saves a lot of time. For white-collar automation, these are the tasks that for decades people have wished a machine could do. These are rote tasks such as data entry, processing, and sorting.
Additional context:
- See our full article titled “AI for Customer Service – Current Use Cases”
- See our full article titled “Two Examples of Automating White Collar Work”
[tecarousel]
Hottest Current Industries for AI Enterprise Traction
In terms of specific industries, AI is most robust is in healthcare and finance because these are the sectors that provide the most financial support for its research and development. The people working in these fields realize the richness of data in there. In finance, this is mostly in fraud and security, in healthcare; this is mostly in discovery and diagnosis.
Diagnostic technology in general is among the top venture capital recipients, because it has many benefits in terms of healthcare. For example, a system can map genomic data on top of a patient’s specific condition to see if there is a precedent for treatment. Doctors may not be able to make the connection, but a machine learning system might if it runs through the available research in the field, and map a particular drug or therapy combination, which doctors may not have thought of.
Additional context:
- See our full article titled “Machine Learning in Finance – Present and Future Applications”
- See our full article titled “Machine Learning in Healthcare – 2018 and Beyond”
Adopt or Wait?
Given that most AI requires significant resources, yet is inevitable in the decades ahead, should business leaders adopt AI now, or wait for the technology to mature?
The situation is not black and white. As I mentioned above, there are five types of adopter: innovators, early adopters, early majority, late majority, and laggards. As a rule of thumb, most business leaders will want to avoid being an innovator (because of the uncertainties, talent, and costs involved) or a laggard, where the rest of the industry has already harnessed the ROI of AI.
Being an innovator requires a unique set of capabilities – and a unique set of business needs. Companies such as Microsoft, Google, and Facebook are part of this exclusive club, and doing basic research on AI in deep learning itself.
Some other “innovator” companies (companies applying AI early, in an experimental phase) are not going so far as to move forward the science of AI, but they are often using massive resources to flesh out AI’s business applications to find AI. For example, JPMorgan Chase is working to analyze legal documents with AI, and Delta Airlines is using facial recognition at some of it’s kiosks – but neither of these firms are pushing forward in basic AI research like – say – Google or Amazon.
Let the Big Firms Sort Out the Bugs
For most firms, the best path will be middle ground – being among the early or late majority and pay no attention to buzzwords and marketing ploys that claim AI implementation to be simple or inexpensive.
Most business leaders should simple pay close attention to real case studies and real applications of industry-leading organizations. When these organizations are clearly delivering ROI from these applications, then they might be worth looking into and exploring.
As the technology becomes more accessible in the years ahead, more vendor companies will aim to replicate and offer the AI applications of industry-leading firms, making them more accessible to SMBs and companies without a massive bench of AI talent (i.e. most companies).
When it Might Make Sense to Innovate in AI
As a caveat, some companies may indeed be able to make a case to innovating in AI, or at least for becoming an early adopter. In general, these companies:
- Have a strategic plan to compete in their market which involves specific advantages over competitors, and specific value for customers
- The best (or only) ways for this advantage or capability to be leveraged is through artificial intelligence
An example of such a firm might be a merchant account business that needs to find new ways to detect fraud in order to stay competitive in the market, or a logistics company that needs to better predict demand to stay competitive in the market.
It’s important to note that in such a situation, a company should never assume that AI or machine learning is the answer to their company’s problem, or the key to its differentiation. Rather, a company should explore all possible technology options (possibly with the help of an outside consultant) in order to determine if AI is in fact the right solution. AI used for its own sake is almost destined to fail.
In order for companies to consider this route, a company must:
- Have the budget, time, and resources to bring on consultants and new hires to flesh out AI applications, without expecting any kind of near-term return on investment
- Have a clear understanding of their problem, and robust understanding to validate why AI is being used as the solution
- Have a leadership team who believes in AI, and is willing to learn new terminology and methods to foster the growth of AI in their firm
Take All Claims with a Grain of Salt
The sources of one’s information should be called into question when reading about AI innovation. In our experience, the opinions of business leaders are often swayed (away from objectivity) by the following sources:
- Vendor companies (they want customers). Vendors often drastically overrepresent what their technology is capable of, and drastically underrepresented how long it takes to integrate their technologies. Also, vendor firms are notoriously vague with the tangible results of their case studies – particularly if they have no real results to speak of (we have tried to solve this problem with our case studies section here at Emerj).
- Consulting firms (they want enterprise deals). The IT consulting giants know that AI has the potential to harm their people-heavy firms (via automation), but also that AI presents an opportunity to generate huge sum of money from enterprise customers who want to “jump on the wave.” We believe that consulting firms often fool companies into believing that if you aren’t using lots of AI in lots of areas of your business, you’re sure to be eaten alive by competition. While in some cases this is true, it is only true in the long run. In the near terms, companies should be very skeptical and discerning about AI, and no more eager to “throw money” at it than at any other technology.
- Tech press (they want clicks and shares). A common headline might read “XYZ Company Revolutionizes Self-Driving Trucks.” With 5 minutes of digging a bit deeper, it’s easy to learn that this company is just a few guys out of college who raised a few million dollars. No traction, not evidence of “revolutionizing”, no product. Business leaders see their social feed and inbox full of these kinds of statements and think that AI is making much more progress than it is. We’ve built objectivity into our company DNA (see our “about” page), and while it means we get less hype-induced social shares, we believe that in the long term our goal of establishing “trust” is one of the most important tenets of fulfilling our mission.
It’s important to note that none of the above parties are particularly malicious in their intentions, they are merely following their incentives and doing what it likely best for their own survival – which isn’t something we can necessarily call out as a moral failing. That being said, it’s important for business leaders to be wary of where they get their information, and the incentives of said information providers.
How to Cut Through Hype When Considering AI’s Importance for Your Business
When I am called on to give presentations to business audiences – it’s almost never just for the sake of “finding interesting use-cases.” Maybe the marketing audiences want to learn about marketing use-cases, and maybe healthcare audiences want to learn about healthcare use-cases – and in both cases I’ll deliver – but there’s a deeper point that all groups are looking for. They all want to answer this question:
“What should I pay attention to, and what should I ignore?”
More than anything else, answering that question is the value proposition of Emerj, and it’s also the end point for most of my speaking engagements.
Train your “attention antennae” to tune out the 95% of AI hype that is not actually going to be relevant to you and to be able to consider serious things that matter. Here are the steps that I advise:
- Have a firm conception and flesh out the possibility space of AI within the sector of your business to understand what is viable, and what is possible. Get a basic understanding of the types of problems that AI can solve by making a quick Google search or jaunting through our site for a little to give you some idea of the kinds of problems that map well onto artificial intelligence that it potentially could solve and for which it is suited. The more you look into the space, the more you learn. Unless you know that, everything will seem viable, and that is not true.
- Familiarize yourself with the precedence and use cases within your own industry. Find out who are the industry leaders in your space that are using these kinds of things. You can find some interesting case studies on our site, such as what the top 5 pharma companies by revenue are doing with artificial intelligence. You can also go on Google and find any government source, which usually use some cutting-edge IT.
- Talk to companies who have developed and implemented similar applications and get a realistic understanding of what it would take to implement them yourself. Find someone who has done something analogous to find out how long it took to adopt AI successfully. What kind of in-house expertise did it take? How long did it take to train, tweak these systems, and actually shake a dollar ROI?
- Think about your strategic initiatives. Think about your major goals. Think about the benchmarks you have to hit, the technologies you have to develop, and the most pressing areas for development. Decide then if investing in AI is the best way to achieve your business goals and strategic objectives, and remain competitive in your market. At some point, there will have to be adoption, but you should not do so from FOMO or a fear of missing out.
Early adoption is probably not right for most enterprises unless you have the requisite talent in-house, and your competitiveness predicates on the dedication to some particular cutting-edge technology using machine learning. If that is the case, then seriously contemplate AI adoption now.
Otherwise, it is advisable to wait and see when there is some traction in bigger businesses or some other industry where there is some semblance of return. Do not dive into the deep end of research because cause it is not an easy undertaking.
When considering AI for your business, ask vendors specifically about what companies similar to yours that have gotten the results for which you are looking. You commonly see AI companies in pilot mode pivot from finance to some other categories like insurance and claim that it works for different industries. This can be a costly assumption.
You should really have a firm understanding of the concept of AI as you would in any other IT procurement situation and see if these people are delivering some kind of a result. Vendors are not necessarily trying to trick you. It is simply everybody’s trying to figure AI out. Enterprisers like yourselves and vendor companies are just trying to connect the puzzle parts. Most companies are not there yet.
A special thanks to Dr. Charles Martin for helping to highlight and make salient many of the critical points in this presentation. I spoke with Charles before my trip to give this speech, and his insight into AI’s “adoption friction” helpful in making this presentation complete.
The original transcription and writing for this article (based off of Daniel Faggella’s presentation at the Technology Association of Georgia in September 2017) was done by Tini Abadicio, with later editing and additions by Daniel Faggella.
Header image credit: Technology Association of Georgia