In 2017, Emerj conducted research into the applications of machine learning in marketing with 51 different AI-focused marketing executives. The AI marketing vendors we spoke to named retail and eCommerce as the top sectors ripe for applying marketing AI software. Below is a graphic from our research showing the sectors that AI marketing vendors sell into most:
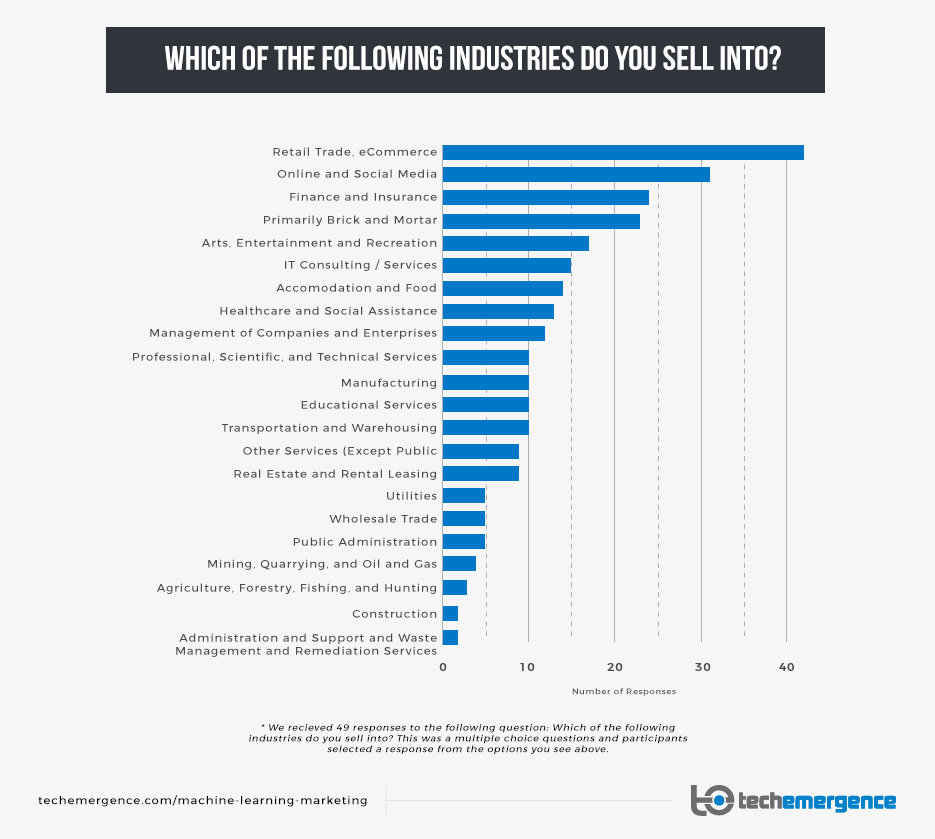
As of now, numerous companies claim to assist retail professionals in aspects of their roles from improving customer service to predicting demand.
We researched the space to better understand where AI-based business intelligence software come into play in the retail industry and to answer the following questions:
- What types of AI-based business intelligence software are currently in use in retail?
- What tangible results have AI-based business intelligence software driven in retail?
- Are there any common trends among these innovation efforts? How could these trends affect the future of retail?
This report covers vendors offering business intelligence software across three applications:
- Improving Conversion Rate Optimization (CRO)
- Demand Forecasting
- Customer Analytics
Improving Conversion Rate Optimization
Sentient Technologies
Sentient is a California-based company with 92 employees. The company offers a software called Ascend, which it claims can help online retailers improve marketing designs in promotional campaigns and improve conversion rate optimization (CRO), increasing the percentage of visitors to an online retail website that convert into customers, using machine learning.
Sentient claims online retailers can test the performance of several different designs for their website using the Ascend software. A team from Sentient first works alongside the client businesses’ marketing and software development teams. The team fleshes out the aspects of the design that can be varied, such as positioning and content in headers, progress bars, pricing displays, and product photos, for a proof-of-concept project.
A control design is then created to compare the impact of any design changes against the control. Then, Ascend automatically tests the changes to customer conversion rates for each of the designs. The software applies a genetic algorithm to create new design changes and tests their performance.
Over the course of a few weeks, Ascend can reportedly test and suggest the best design for the client’s webpage in terms of CRO. The system then provides the best performing combination of design elements in the webpage showing the expected increase in customer conversion rates from the design.
Below is a short 1-minute video demonstrating how Ascend works:
Sentient claims to have helped Euroflorist improve customer conversion rates for their online retail website. Euroflorist and their partner Online Dialogue deployed the Sentient Ascend and, for a proof of concept (POC) on Euroflorist’s Swedish desktop site, focused on their product detail pages.
The project reportedly took place within eleven weeks, during which four generations of genetic algorithms were fully tested. Genetic algorithms use methods similar to the biological evolution process to find the most optimized solution for a problem.
Most genetic algorithms start with a defined objective and several software “agents” whose performance is tested over many generations, or iterations of the software. In each generation, the best-performing agents are chosen and replicated with a few randomized changes added to their behavior (to simulate the passing on of genetic traits and natural selection in evolution). In Sentient’s case, over each generation, design elements are changed and combined to find the features that improve conversion rates.
According to Sentient, this resulted in the fourth generation designs for the product showing a significant increase in conversion rate for Euroflorist. No further measurable results were available, and Sentient further cautioned the right input must be used with the software to show the positive impact on the business case.
There seems to be some evidence that Sentient Technologies collaborated with MIT’s Computer Science and Artificial Intelligence Laboratory team (CSAIL) for a one-year period in a project in the healthcare space. The company also lists Rakuten, Mobly, and National Debt Relie as some of their past clients.
Sentient was founded by current CEO Babak Hodjat. He holds a PhD in Machine Intelligence from the University of Kyushu University. Hodjat also served as founder and CTO of three other AI startups before starting Sentient.
Demand Forecasting
Blue Yonder
Blue Yonder is a German company that offers a predictive analytics software, which it claims can help retail businesses gain business intelligence insights to improve customer service and identify new opportunities using machine learning.
Blue Yonder claims users can first upload historical transaction and marketing data into the software. For instance, the software might be used by grocery retailers for demand forecasting applications. The grocery retailer would need to organize their internal data, such as point of sale records, promotional campaigns, and product information.
Then, Blue Yonder’s software combines the internal data with weather, holiday, and special event data for the retailer. The software uses machine learning to predict the demand for each product daily. The system then provides inventory managers with inventory replenishment notifications for every product in each store, daily on their dashboard.
Blue Yonder claims to have helped Morrisons optimize inventory replenishment and automate the ordering of 26,000 product SKUs in all its 491 stores. Morrisons deployed the Blue Yonder Demand Forecast & Replenishment solution, which uses AI, according to the company.
The grocery retailer uploaded historical product, promotions, and sales data into the software to predict the demand for each product in all their stores. According to Blue Yonder, this resulted in a 30% reduction in shelf gaps over the course of 12 months and like-for-like sales rose 1.7% in the final quarter of 2016.
Below is a short 4-minute video demonstrating how Blue Yonder’s software works:
Blue Yonder also lists Otto and Selgros as some of their past clients. Blue Yonder was founded by former CERN scientist Professor Michael Feindt and acquired by JDA Software in 2018.
Jan Karstens is CTO at Blue Yonder. He holds an MS in Computer Science from the University of Potsdam. Previously, Karstens served as Development Architect at SAP AG.
IBM Watson
IBM offers customer engagement solutions for retail using their Watson AI platform, which it claims can help retail businesses improve customer engagement using machine learning.
IBM claims users can design conversational interfaces to gather customer feedback on purchased products. Retailers can use this data for customer analytics and find ways to improve customer engagement on their websites using AI. Watson provides customer service teams at retail and eCommerce companies with the top customer issues for each product on a dashboard.
IBM claims to have helped 1-800-Flowers.com develop their new product, Gifts When You Need (GWYN), an “AI gift concierge.” According to IBM, the software tailors gift recommendations by comparing specifics provided by the customer in their search query with gift products purchased for similar recipients. As a result, 70% of online orders were completed through GWYN. The case study is described in video format below:
IBM also lists Boots, UK as one of their past clients.
Customer Analytics
RetailNext
RetailNext is a California-based company with over 200 employees. The company offers a software called Traffic 2.0, which it claims can help retail businesses improve operational efficiency by benchmarking store traffic using machine learning.
RetailNext claims users can integrate RetailNext’s SaaS with legacy systems in retail stores to access IoT data. RetailNext also provides its own IoT hardware sensor called Aurora. RetailNext’s solution uses video analytics, on-shelf sensors, and data from point-of-sale systems.
The system uses machine learning to draw out insights on customer engagement from this data. The system then provides access to the RetailNext platform from a web browser or its mobile app. Users can see a graphical visualization of store trends.
Below is a short 1-minute video demonstrating how Traffic 2.0 works:
RetailNext claims to have helped 100% Pure, a cosmetics retailer personalize shopper experiences. 100% pure deployed RetailNext’s solution across all its stores in the US. RetailNext used historical POS data, Promotional campaign data and data from in-store IoT sensors to analyze individual customer shopping trends. According to RetailNext, this resulted in 100% Pure being able to better understand the core factors affecting customers’ shopping journeys. We could find no further measurable results for this case study.
RetailNext also lists Bloomingdale’s, Club Monaco, peach Johnson, TSI Holdings, and Busckfeet as some of their past clients.
Mark Jamtgaard is Director of Technology at RetailNext. He holds degrees from Stanford and Santa Clara University. Previously, Jamtgaard served as Technical Lead at Cisco.
Takeaways for Business Leaders in Retail
Creating customized experiences for retail consumers, such as targeted marketing or personalized promotions, are areas where AI can directly affect business outcomes. At companies that have access to large volumes of customer data, such as customer purchase history, the data science talent at the company could implement AI-based business intelligence projects to drive value. In terms of real business returns, retailers could save on time and costs as compared to manual processes by using AI.
In our 2017 AI research, we were told by executives at AI vendor companies that large global retailers like Target and Walmart might have the budgets and data volume required to successfully implement AI technologies. But, due to the tight profit margins in the retail industry, it may be another three to five years before most large retailers invest in AI applications in manufacturing, logistics, or customer service.
Looking at the use cases for the software we covered for this report, it seems as though AI applications that have a high likelihood of adoption are those that have decently well-established use cases and ROI. RetailNext has raised over $184 million and are backed by Activant Capital and NGP Capital.
As with other industry sectors like healthcare or finance, the future adoption for AI-based business intelligence applications will likely be driven by the larger retail players. We might very likely see a retail ecosystem with large retailers following strategies and use-cases established by companies such as Amazon. Small and medium-sized businesses might be better off investing in business-critical AI applications that have well-established case-studies.
Header Image Credit: BoF