This article is based on a presentation originally given by Daniel Faggella, CEO & Founder at Emerj (formerly TechEmergence) to a group of real estate executives at a Grupo4s “Future of Real Estate” event in San Francisco, in March of 2018.
The real estate market in the US is currently a seller’s market, with demand outstripping supply, and housing affordability going down steadily for 2018 (Source: Gallup, May 2018). As more efficient means of buying and selling properties are being made possible with the help of machine learning, other AI-based applications are creeping their way into maintenance, energy management, and more.
In the article below, we’ll explore the applications of machine learning in real estate. We’ll examine the buying and selling process, in addition to a more in-depth look at facilities management and building automation systems. We’ll also examine other industries that might serve as a proxy for future real estate innovation, helping executives to imagine future possibilities before they impact real estate itself.
To help business leaders find the real estate applications and ML insights that matter most to them, we’ve broken this article out into the following sub-sections:
- Why Machine Learning Matters in Real Estate
- The State of AI in Enterprise
- 3 Applications of Machine Learning in Real Estate
- Proxy Application: Consumer Apps, Chatbots and Machine Learning-Based Interfaces
- Proxy Application: Predictive Maintenance in Manufacturing and Heavy Industry
- Application Area 1: Analytics in Building Automation Systems
- Application Area 2: Automation in Property Management
- Application Area 3: Machine Learning in Real Estate Marketplaces
- Deciding “Yes” or “No” on Machine Learning Applications
- Concluding Thoughts – Avoiding Hype, Moving Forward
The slide deck from my original presentation can be found below, and the full article continues further below:
Why Machine Learning Matters in Real Estate
Our interviews with hundreds and hundreds of ML researchers (for our AI in Industry podcast, and elsewhere) show that they agree on very little in terms of big-picture dynamics of ML. There is no consensus on which industries will be transformed first, no consensus about AI risks in the coming 20 years, and no consensus on a definition of artificial intelligence. However, the one thing they do agree on is that AI will change a lot of how business is done in the coming decade or two, as the Internet did in the past two decades.
Tracking the development of AI technology makes strategic sense. However, much of the information available on AI and machine learning is more wishful thinking (or hype) than facts. Business leaders should know what merits attention, and what they should ignore. AI should be on the radar for future-thinking leaders, but it should be the right size of a radar blip.
The goal of this article is to promote a realistic picture of enterprise AI in general, and in real estate in particular. This will enable decision makers to distinguish between the aspects of AI that will have some value to a business and the 95% that will not.
The State of AI in Enterprise
Artificial intelligence was exciting and novel in the 60’s, and went through another period of enthusiasm (in academia and industry) in the 80’s. In the 60’s and 80’s, however, artificial intelligence was not commonly leveraged in business applications, and the largest companies during those decades were not predicated on artificial intelligence to deliver their service or product.
Today, the business is quite different. The most profitable companies in the world are predicated on artificial intelligence and machine learning, such as Google, Facebook, Amazon, Netflix, and Microsoft. While these companies did not start out using AI, the technology has since become the backbone of these firms. They would not have been able to reach their current prominence without machine learning.
In many ways – machine learning is still finding its way into most business applications. Most companies that deal with AI technology in some way are relatively new and are still experimenting with the technologies. Healthcare is excited about AI, in some cases the industry is adopting AI, but by no means has healthcare been “overhauled” as an industry. The same can be said of transportation, finance, and many other sectors. It may be another decade until AI becomes a necessary and important part of most major industries.
Our research shows that, in general, about 1 in 3 AI or machine learning companies have the requisite AI intellectual capability (meaning, an executive with robust AI experience from academia or a previous job). Of those companies, only 1 in 3 will have some degree of traction with a pilot program with documented business value. Even then, this 10% of AI companies that have the capability may not be profitable yet, because they are still trying to figure out how to produce consistent results and solve real business problems.
AI and ML technology today requires a significant investment in resources (more than most businesspeople expect), and there is no guarantee that the end solution will work. Software can be programmed to be stable, but machine learning solutions are probabilistic, and even with sufficient training data, a solution may never produce meaningful results. Business leaders considering AI applications should bear these considerations in mind and do their homework on what AI adoption and integration really requires.
(Readers interested in applying AI to an existing business should refer to our full article titled: Enterprise Adoption of Artificial Intelligence – When it Does and Doesn’t Make Sense.)
3 Applications of Machine Learning in Real Estate
Real estate is far behind other industries (notably: Healthcare, finance, transportation) in terms of total AI innovation and funding for machine learning companies. However, real estate professionals can look at proxy industries to see how they leverage AI to solve similar problems in real estate.
Two major AI application categories to which the real estate industry can look for proxy industries is consumer-facing and predictive technologies.
Proxy Application: Consumer Apps, Chatbots and Machine Learning-Based Interfaces
For consumer-facing applications such as chatbots or matching people with properties, the eCommerce and consumer technology spaces are where it comes out first. Their applications in the real estate industry will only be able to adopt it once it has been developed in industries that are moving faster.
Generally, when something is possible to do with consumer-facing AI technology, it is unlikely that it would happen first in the real estate industry. If companies such as Facebook or Amazon have not developed it, then it is unlikely it will happen anywhere else.
Proxy Application: Predictive Maintenance in Manufacturing and Heavy Industry
For predictive technology such as the maintenance or upkeep of equipment, assets, and properties, the manufacturing and heavy industry spaces are most likely to do it first. Efforts in developing the technologies and processes to optimize this function are more likely to give a return in these industries than in real estate. Real estate executives looking into predictive analysis, smart technologies, and the internet of things (IoT) applications, in general, can take their cue from technologies developed in these industries.
Given that these two major categories of AI technologies are representative of the type of innovations that have potential value for real estate in the future, here are three possible applications to improve efficiencies.
We’ll now explore some of the direct application areas of mahcine learning in real estate today:
Application Area 1: Analytics in Building Automation Systems
The first of the applications of AI in real estate is in building automation systems, which serves as a type of IoT system. This involves placing sensors in strategic areas in the building. Sensors may be placed in the lighting or heating systems, elevators, and security systems to collect data that will help the building owner improve energy efficiencies.
If successful, the data collected will support an emerging market for predictive analytics. The problem is machine learning models tend to have difficulty dealing with temporal or time-stamped data that has a wide variance. The challenge is building these temporal data into models so that “sensorized” or instrumented IoT-infused buildings can make consistent predictions when tracking multiple streams of data with seasonal, weekly, or daily differences. This seems to be a friction point for this type of machine learning application.
The technology is not yet able to fully predict what will happen to a building. However, continuing development does open up the potential for future real estate managers to know a lot more about predicting what is going to happen under certain circumstances in their facilities.
Another challenge in real estate is figuring out where to put sensors and what activities to track to maximize data collection without compromising the privacy of the building’s occupants.
In the online world, users understand that all their activities are being tracked, and implicitly consent to it by using a particular website (this is why applications in machine learning and marketing are focused on selling to online retailers more than offline retailers – a trend we explored in greater depth in our machine learning in marketing executive consensus report).
The situation is different in a physical facility. People expect a certain amount of privacy, and a building owner or manager is obliged to protect that privacy. Integrating sensor technology into buildings will involve a delicate balance of functionality, insight, and respect for the people whose behavior will now be tracked and detected.
Security is a similar concern with “sensorized” buildings (it was IoT devices that were hacked in the infamous DDoS attack which took down much of the consumer web in 2016). With “smart” devices and sensors placed in various systems in the building come more opportunities for hack into systems to access sensitive information. A good example of this is the breach at Target in 2013, allegedly via one of its smart HVAC systems, where the financial and personal information of millions of customers was accessed. This is one of the things to keep in mind when considering a building automation system.
Some of the companies doing some promising work in this space include:
- PointGrab – uses embedded-analytics sensors to provide visibility into the behavior and space utilization of occupants in real time; the demo video emphasizes individual privacy by keeping the tracking anonymous
- TellMePlus – its Predictive Objects application uses AI to help heavy machine and real estate companies improve efficiency and performance by embedding sensors in critical assets and making faster, more accurate predictions
- BuildingIQ – enables tracking analysis, aggregation modeling, and reporting energy data within the enterprise at a meter and submeter level to optimize energy efficiency
While these companies may seem to be achieving some traction in building automation technology, it is important to proceed with caution. Many of these companies are small and relatively new, and may show aspirational rather than actual results. For example, the demo videos or use cases published on the websites of these companies may imply that getting positive results is easy. That is most probably not the case.
Installing and integrating AI software and technology at the current stage of development requires extensive hands-on treatment with individual buildings. It is highly probable that the time spent on setup and integration, training, and testing to achieve good results will be quite substantial.
Application Area 2: Automation in Property Management
The second application area is in the automation of property management job functions. The opportunity for savings is that managers will achieve visibility into sales and agent performance data to drive prospect conversion and improve outcomes for the business development team.
The market opportunities of real estate chatbots could potentially interact with applicants through Amazon Echo or other smart devices to help with distributed concierge services to delight and reward commercial tenants. In the future, a possible application for this type of automation is to streamline the initial part of the sales process. It may be to accommodate applications for a potential tenant or setting an appointment for a site viewing through conversation interfaces such as a chatbot.
Some of the promising companies in this space include:
- VTS – a marketing app that centralizes critical data and process to attract, convert and retain tenants for commercial properties
- Appfolio – software that focuses on marketing to attract and retain tenants for commercial properties
- Buildium – automation technology to improve the tenant-landlord relationship for residential properties
- Zenplace – uses a chatbot via Amazon Alexa to answer basic questions from current tenants as well as enable them to pay their rent easily, manage their energy usage and billing, and even report issues with the property
Companies like Apartment Ocean and Automabots are also heavily leveraging chatbots, but selling to realtors with websites rather than using them to go after buyers directly. However, some brokers have argued that the technology is still too new and risks turning off prospects that may find the bots are rude or unhelpful. This is an ongoing debate that has yet to reach a resolution.
Application Area 3: Machine Learning in Real Estate Marketplaces
As mentioned earlier, the current real estate market is a seller’s market, so matching the right people with the right places at the perfect price point is a profit-driving value proposition. Many real estate platforms such as Airbnb and Zillow are using this type of recommendation application to good effect. Its main function is to match the tenant with the landlord, potentially augmenting the broker’s job.
Property owners and managers can also benefit from these platforms by understanding how they work, and learning to co-exist with them. For example, understanding how recommendation engines work for people searching through Zillow or Airbnb can provide useful insights on how to make a listing on these platforms as attractive as possible to potential tenants or buyers.
Home appraisals are also a big part of a realtor’s job that AI can augment. A Techemergence interview with Zillow Chief Analytic Officer Stan Humphries shed some light on AI in real estate use case. He talked about how to make more accurate appraisals of a home using AI models using visual data and other kinds of information.
These are online technology companies that tend to be AI first, meaning that data science was the main element of these companies from the beginning. This is probably not true for people that own a hotel or two, and may not even be on their radar at all, most probably because it does not need to be. However, AI will certainly be necessary in the future, and some of the more interesting market opportunities are real estate specific AI applications, such as virtual and augmented reality.
Goldman Sachs Research estimates that the market for virtual reality (VR) in real estate alone could generate as much as $2.6 billion by 2025. Given that much AI development, today is in computer vision it is likely to see 3D property visualization creating new tools for brokers and property managers. There is definitely some good potential for people that want to check a house for sale, rental property, or hotel will wherever they are as if they were actually there.
It might sound like just virtual reality, but AI would generally be required to realistically replicate an existing environment (refer to our podcast interview on the topic of machine learning for VR and machine vision). It is only through proper training of machine learning that AI can take a series of images and sensor data and put them together into a virtual world that feels like the real thing.
Deciding “Yes” or “No” on Machine Learning Applications
Determining if AI is something that will provide real value to a business requires a lot of research, and a bit of risk-taking. Smart executives for small and medium-sized enterprises without the resources for either can still be on the cutting edge of their industry by paying attention to what the big companies are doing with AI.
Looking at what seems to be gaining traction in relevance and revenue in the largest companies in an industry is a good proxy of what might actually have some kind of return on investment. Assume then that 90% of the press releases are without value, and focus on the remaining 10%.
It is also important to take note of the major challenges inherent in AI integration in existing companies. One of these is the lack of machine learning talent. The most capable people in this space are hard to find, mostly because they go to big companies such as Google and Facebook, who pay them more than most companies can afford. It is important to have in-house talent as consultants cannot do it all.
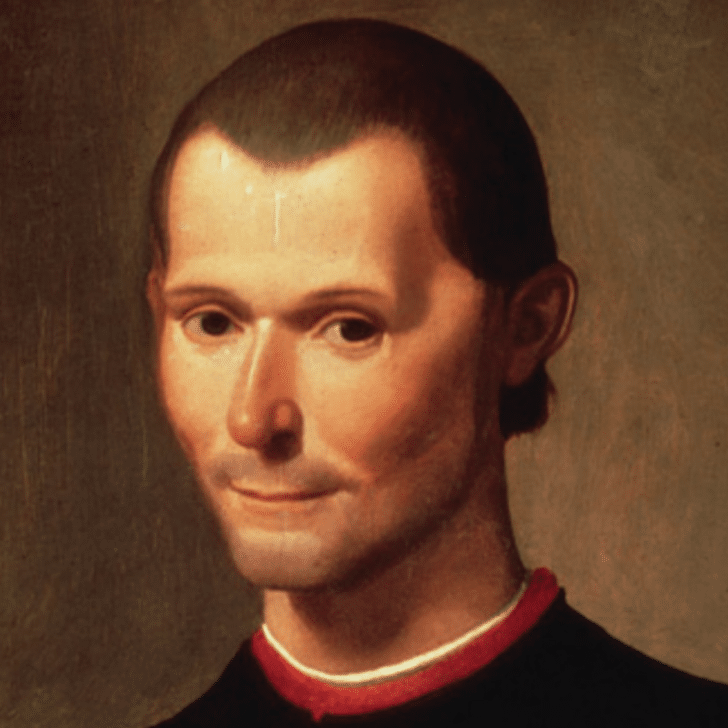
A total reliance on outside machine learning consultants is a dangerous position to be in.
In The Prince, Machiavelli writes at length about the danger of relying on mercenaries and auxiliary troops in winning battles or conquering strongholds:
“A prince or republic, then, should adopt any other course rather than bring auxiliaries into their state for its defense, especially when their reliance is wholly upon them; for any treaty or convention with the enemy, however hard the conditions, will be less hard to bear than the danger from auxiliaries.”
Consultants (in Machiavelli’s day, “auxiliaries”) have different incentives than full-time team members, which might lead to drawn-out projects, disjointed priorities, and unnecessary expenses. Machiavelli might be overboard in his assessment of the same, but his words are more fun to read than my own:
“Mercenaries and auxiliaries are useless and dangerous; and if one holds his state based on these arms, he will stand neither firm nor safe; for they are disunited, ambitious, and without discipline, unfaithful, valiant before friends, cowardly before enemies; they have neither the fear of God nor fidelity to men, and destruction is deferred only so long as the attack is; for in peace one is robbed by them, and in war by the enemy.”
Of course, machine learning consultants aren’t useless – such expertise is a necessary addition to a talent-starved ecosystem – and in previous articles, we’ve gone into depth on the value of machine learning consultants and training events. They certainly have their place – but many consultants themselves will admit that having an outside party serve as both subject-matter expert and head AI wizard is a dangerous place to be, and not a viable long-term strategy for making AI part of the DNA of a company.
Another challenge has to do with the management structure. An existing company will not be set up to deal with the uncertainties of AI applications or the length of the R&D processes. There is a lot that goes into bringing AI and machine learning into existing businesses, and the people from the top down have to fully engage with the integration.
Management has to know the real capabilities of AI or ML in solving problems. They cannot hand the AI team problems that 90% of the time machine learning is not designed to solve, and expect positive results. Then once an application is built, somebody has to figure out how to integrate it into the rest of the company. The length of time it takes to tweak and modulate AI or to build something out within an existing enterprise is very challenging.
Perhaps the biggest problem with any AI integration in an existing company is accessible data. Most companies that adopt AI in their operations often do not have enough data available in the proper format to train machine learning applications. The data may be there, but not organized for easy use with AI applications.
Most vendors believe that big companies will not be adopting AI directly because of these difficulties, particularly the management of data. Most of the bigger companies will adopt AI through acquisitions, and those that do not figure out a way to manage their data moving forward will not have much of a future.
The same scenarios will apply to the real estate industry. AI is not revolutionizing necessarily everything now in every single way. It has much potential, but it is mostly just a lot of noise right now. A discerning executive should be able to train their brain to differentiate what is relevant and not, and when and where to focus their time and money.
Concluding Thoughts – Avoiding Hype, Moving Forward
Many exciting prospects exist today for real estate applications using AI. However, it is a work in progress, and many real estate executives fall into the trap of “toy” applications. “Toys” are AI-related projects taken on simply because they use AI – not because they solve an actual business problem. This is part of a hype trap, a way of thinking which is warped by enthusiasm in the marketplace.
The best way to avoid the toy application trap is to ignore persuasive marketing tactics employed by vendor companies and to think about the adoption of AI like any other technology (i.e. pretend that machine learning isn’t “cool”, and make the best decision for your company’s goals and strategy). Think about the problems, direction, and goals of the company, then consider the technologies that can help. If it happens to be AI-based, then it is not a toy application.
Certainly, business leaders should be considering the impact of AI’s inevitable impact in their industry. This might be enough to keep AI “on the radar” for strategic planning. It should not, however, translate to impulsive and disjointed “projects” that involve AI for AI’s own sake. As a nascent industry for AI use, real estate leaders are as likely as business leaders in other industries to fall into this trap – and it should be avoided.
It’s perfectly fine to accept that most new IT integrations in real estate will not involve machine learning. Most companies will not have the resources to use AI and expect a reasonable return on investment, and may actually create more problems than it will solve.
The real estate industry is not an exception to the rule. The three applications described above show how AI applications may potentially provide some real-world solutions to problems. However, they may come at too high a price for many real estate companies. AI applications and solutions will become more accessible to more businesses in the future, but not just yet. Machine learning adoption is shouldn’t be taken lightly.
A special thanks to Emerj contributor Ed Zagorin for conducting the initial research on our previous AI in commercial real estate article from late last year, and helping to generate many of the ideas for this article.
Header image credit: Dan Faggella