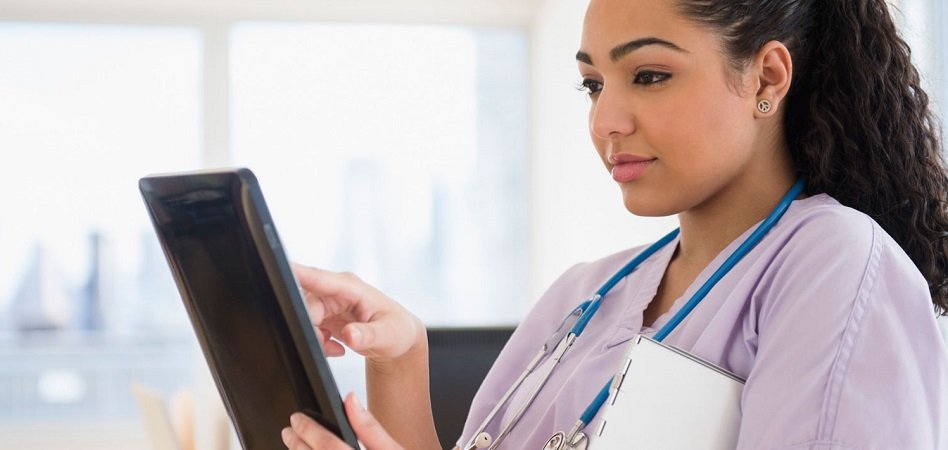
Sensors and mobile devices are in many ways working with AI software for business intelligence purposes in a few industries, including insurance and oil and gas. In the healthcare space, mobile devices and wearables allow patients to receive information on possible diagnoses for their symptoms and to monitor metrics such as their heart rate.
There are several AI vendors claiming to offer machine learning software to healthcare enterprises, including hospitals and clinics, and some that are also offering mobile applications and IoT devices, such as inhalers, to consumers.
We set out in this report to answer the following questions:
- How is the healthcare industry combining AI and the Internet of Things (IoT) today?
- What are the current applications of IoT and AI in healthcare?
In this report, we’ll explore the enterprise and consumer applications of AI and IoT in healthcare. We’ll examine the applications individually, looking at the way they use data, and the unique capabilities that AI enables in them.
(Note: For readers with a specific interest in IoT and AI in medical devices, see our full article on that topic here.)
We’ll begin first by exploring enterprise use-cases, beginning with Microsoft Azure:
Applications for Enterprises
Microsoft Azure
Microsoft offers Azure IoT, which it claims can help healthcare organizations track equipment usage to improve the well-being of patients, maintain key equipment, and reduce readmissions using machine learning. The technology is also applicable to other industries, such as manufacturing, transportation, retail, smart cities, and natural resources.
Microsoft claims that healthcare organizations can integrate the software into their patient monitoring and tracking devices.

In order to learn about and reduce the risk of patient readmission, we can infer that the machine learning model behind the software was trained on a variety of health and medical data such as blood pressure readings; compliance with patient’s personal goals; admit, discharge, transfer (ADT) events; and patient-generated data such as assessment-based depression indicators, among others. The data would then be run through the software’s machine learning algorithm.
This would have trained the algorithm to discern which data points correlate to a certain patient group’s vital statistics readings, adherence to doctor’s post-discharge instructions, health and lifestyle practices, availability of a support network upon discharge, among others. The software would then be able to predict a certain risk group’s potential to be readmitted.
Below is a short 2-minute video demonstrating how different types of businesses, from logistics to food, can use Azure IoT to collect data and perform analytics to improve their operations:
Microsoft claims to have helped Roche Diagnostics deliver services to its customers more cost-effectively. The company needed the analytics capability to:
- Remotely monitor and manage its in vitro diagnostic (IVD) devices as fixed assets,
- Predict potential downtime of any IVD solutions deployed in a customer’s clinical setting.
- Recommend the best IVD solution for a customer’s needs.
- Provide data visualization and analytics for better decision-making
- Establish a foundation for future maintenance
Roche Diagnostics contacted Cleidon International, a Microsoft IT partner, which integrated the software into the client’s (IVD devices. In turn, these capabilities have enabled the client to collect operational data, such as location, in near-real time from the IVDs, and to assess the system’s health data, troubleshoot issues, and dispatch supporting teams for service.
At the time of the case study’s writing, Cleidon had planned to build and deploy a preventive maintenance model for the IVD installed base, once the IoT application had gathered enough data from the devices. The data was expected to help ensure the IVD system’s reliability and availability to our customers. Microsoft also lists Ruppiner Kliniken, 365mc, Rolls Royce, and Schneider Electric as some of their past clients for its IoT applications.
Sam George is the Director of IoT Engineering at Microsoft. He has served at Microsoft for more than 21 years, starting in 1997 as a Software Developer – WPF, Hotmail, Microsoft Learning Technologies and Windows 98, rising to Principal Development Manager, Principal Group Program Manager to his current role. His profile does not reveal his educational background.
Medidata
Medidata offers cloud-based mobile health (mHealth) technology, which the company claims can help healthcare organizations collect data to that can be used to learn about the quality of life of patients with cancer using machine learning and predictive analytics. Medidata claims that healthcare organizations can integrate the application into sensors and other activity trackers.
We can infer that the machine learning model behind the software was trained on data related to different types of cancer, the contributory factors, therapies that could potentially help inpatient treatment, types of fitness activities, and nutrition, among others. The data would then be run through the software’s machine learning algorithm. This would have trained the algorithm to discern which data points correlate to which treatments could or could not enhance the quality of life of cancer patients.
The software would then be able to predict appropriate treatments for patients. This may or may not require the user to upload information about their new treatments into the software beforehand.
Below is a short 2-minute video demonstrating how Medidata Patient Cloud is able to aggregate data collected from mobile devices such as sensors, patient wearables, tablets, smartphones, and mobile applications to create more patient-focused databases:
In 2016, Medidata claims to have helped Memorial Sloan Kettering Cancer Center (MSK) bring together its data on the Medidata Cloud and conduct a study in cancer treatment using mHealth technology.
The study had planned to use wearable sensors and mobile technology to monitor the quality of life of patients who were being treated for multiple myeloma through induction chemotherapy. MSK used activity trackers, mobile apps and Medidata’s cloud technology platform to track patterns in patients.
The data collected from the fitness trackers were also to be collected in the Medidata Cloud, and used by researchers to identify multiple myeloma treatments that improve quality of life and life expectancy. Among the factors that MSK would track were patterns of fitness activities and other movements, and quality of sleep.
Patients were to be asked to wear the trackers for 1 to 7 days prior to chemotherapy session to determine a baseline, then continuously through four chemotherapy cycles. MSK researchers were to use Medidata’s visualization and analytics dashboard to monitor if patients adhering to the treatment instructions, and to identify trends and anomalies. Results of the study have not been made available.
Medidata also lists Cancer Research UK, Danone Nutricia Research, Worldwide Clinical Trials, PSI, Transcend Trials, Karyopharm, PhaseBio Pharmaceuticals, Zosano Pharmaceuticals, Teijin, and Onconova Therapeutics as some of their past clients.
Medidata has raised $20 million in funding and generated $545.5 million in revenue in 2017.
David Lee has been Chief Data Officer at Medidata since May 2014. He holds an MS in Statistics from Columbia University. Previously, Lee served as VP, Head of Science at AIG, where he worked for 10 years.
Applications for Consumers
Senseonics
Senseonics offers Eversense, a continuous glucose monitoring (CGM) system that uses a sensor implanted below the patient’s skin that can collect data about the patient’s blood glucose levels for 90 days. The company claims the product helps individuals with diabetes to actively manage their condition using machine learning and predictive analytics.
Senseonics claims that the sensor is implanted under the patient’s skin of upper arm by a trained physician. No part of the sensor protrudes from the skin. We can infer that the machine learning model behind the software was trained on data related to high and low glucose levels. The data would then be run through the software’s machine learning algorithm. This would have trained the algorithm to discern which data points correlate to normal glucose levels.
The software would then be able to predict if the user’s glucose level is moving to a high or low point. This may or may not require the user to upload information about their medication, food intake, and physical activities, among others into the software beforehand.
A transmitter then sends alerts to the patient every 5 minutes on their mobile device as well as on-body vibration alerts from the transmitter itself when the mobile device is out of reach. The patient will also be able to view reports to better understand their glucose history and patterns.
Below is a short 2-minute video demonstrating how Eversense collects, transmits and analyzes the data about a patient’s glucose levels. Because the data resides in the cloud, it can be shared with the patient’s health care provider or a family member who helps take care of the patient:
Senseonics claims to have undergone a 90-day study participated in by 90 patients who had Eversense implanted in their arm. The participants had the Eversense sensor implanted under their skin. According to the case study, Eversense achieved an 8.8% mean absolute relative difference (MARD).value against reference glucose values, which was significantly lower than the predetermined 20% performance goal for accuracy. The study also showed that 99.3% of samples’ predictions were within the acceptable error rates in A (92.8%) and B (6.5%). A total of 91% of the tested sensors continued to work until day 90. Among the 90 participants, one serious adverse event occurred during the study, needing the sensor to be removed.
Senseonics is a consumer product and does not reveal its individual patient clients, but lists distribution partnerships with TypeZero, Roche, and Rubin Medical. The company has raised $325 million in funding from Roche Finance, New Enterprise Associates, Oxford Finance Corporation, Silicon Valley Bank, Greenspring Associates, and Anthem Capital Management.
Abhi Chavan is the VP – Engineering, R&D at Senseonics. He holds a PhD in Electrical Engineering, specializing in Analog Circuit Design and MEMS Sensors from the University of Michigan. Previously, Chavan served as VP – Research, Product Development & Manufacturing Operations at Coventis, Manager II for Product & Technology Development, Boston Scientific, and Project Manager for Medical Applications at Delphi.
Propeller Health
Propeller Health offers the Propeller, a device with sensors that attach to asthma inhalers, which the company claims can help patients with asthma or chronic obstructive pulmonary disease (COPD) track their medication usage, identify triggers, manage symptoms, and achieve more control over their disease using machine learning.
Propeller Health claims that the device, previously known as Asthmapolis, works with most kinds of inhalers and Bluetooth spirometers.
The company states that the machine learning model behind the software was trained on data about levels of air quality, temperature, wind speed, and humidity, as well as the number of times the patient uses their inhaler, among others. The data would then be run through the software’s machine learning algorithm. This would have trained the algorithm to discern which data points correlate to an asthma or COPD attack.
Over time, the software would then be able to predict when an asthma or COPD event is impending, based on the patterns of attack and other factors. This may or may not require the user to upload information about their quality of sleep, state of immune system, nutrition, level of fatigue, etc into the software beforehand.
The patient’s healthcare provider also has access to the data to help the patient monitor and manage the disease.
Below is a short 2-minute video demonstrating how the Propeller determines the time and place when the inhaler is used and what environmental triggers were present at that time. The video below explains that the device helps patients, physicians, and government health officials better understand the disease:
Propeller Health is a consumer product and does not reveal its individual patient clients. Nor does it feature case studies on its website, but has raised a total of $69.9 million in funding from social capital, SR One, 3M New Ventures, Safeguard Scientifics, and Hikma Ventures. It was acquired by Resmed last December 2018 for an undisclosed sum.
Greg Tracy is the CTO and Co-founder at Propeller Health. He holds an MS in computer science from the University of Wisconsin-Madison. Previously, Tracy served as President at Sharendipity and Director of Engineering at Emageon.
Header Image Credit: British Nursing Association