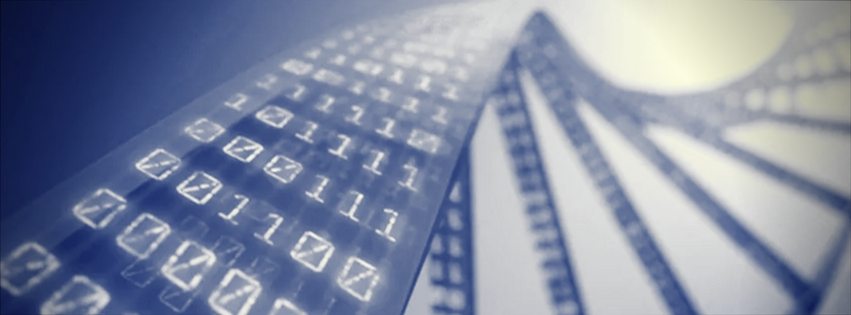
Genomics is a branch of molecular biology focused on studying all aspects of a genome, or the complete set of genes within a particular organism. Today, machine learning is playing an integral role in the evolution of the field of genomics.
We set out in this article to examine the applications of machine learning in genomics to help business leaders understand current and emerging trends within the field.
In this article we will explore:
- Background terminology, and summarized insights from our research
- Current applications of machine learning in genomics
- Potential future applications of machine learning in genomics
- Related Emerj executive interviews
Before diving into present applications, we’ll begin with background facts and terminology about genomics and precision medicine, and a quick summary of the findings of our research on this topic:
AI and Genomics Background and Insights Up Front
The ability to sequence DNA provides researchers with the ability to “read” the genetic blueprint that directs all the activities of a living organism. To provide context, the central dogma of biology is summarized as the pathway from DNA to RNA to Protein. DNA is composed of base pairs, based on 4 basic units (A, C, G and T) called nucleotides: A pairs with T, and C pairs with G. DNA is organized into chromosomes and humans have a total of 23 pairs.
Chromosomes are further organized into segments of DNA called genes which make or encode proteins. The sum of genes that an organism possess is called the genome. Humans have roughly 20,000 genes and 3 billion base pairs. Interestingly, only about 2 percent of the human genome encodes protein and this is a key area of focus in research and the business of genomics.
Genomics is closely related to Precision medicine. With a market size projected to reach $87 billion by 2023, the field of Precision Medicine (also known as personalized medicine) is an approach to patient care that encompasses genetics, behaviors and environment with a goal of implementing a patient or population-specific treatment intervention; in contrast to a one-size-fits-all approach. For example, to reduce the risk of complications, an individual who needs a blood transfusion would be matched to a donor who shares the same blood type instead of a randomly selected donor.
Currently, there are two main barriers to greater implementation of precision medicine: High costs and technology limitations. To tackle the vast amount of patient data that must be collected and analyzed, and to help cut down on costs many researchers are implementing machine learning techniques.
Fortunately for researchers and genomics companies, the cost of sequencing a genome continues to drop year-over-year – even after a massive relative plunge in cost between 2007 and 2012:
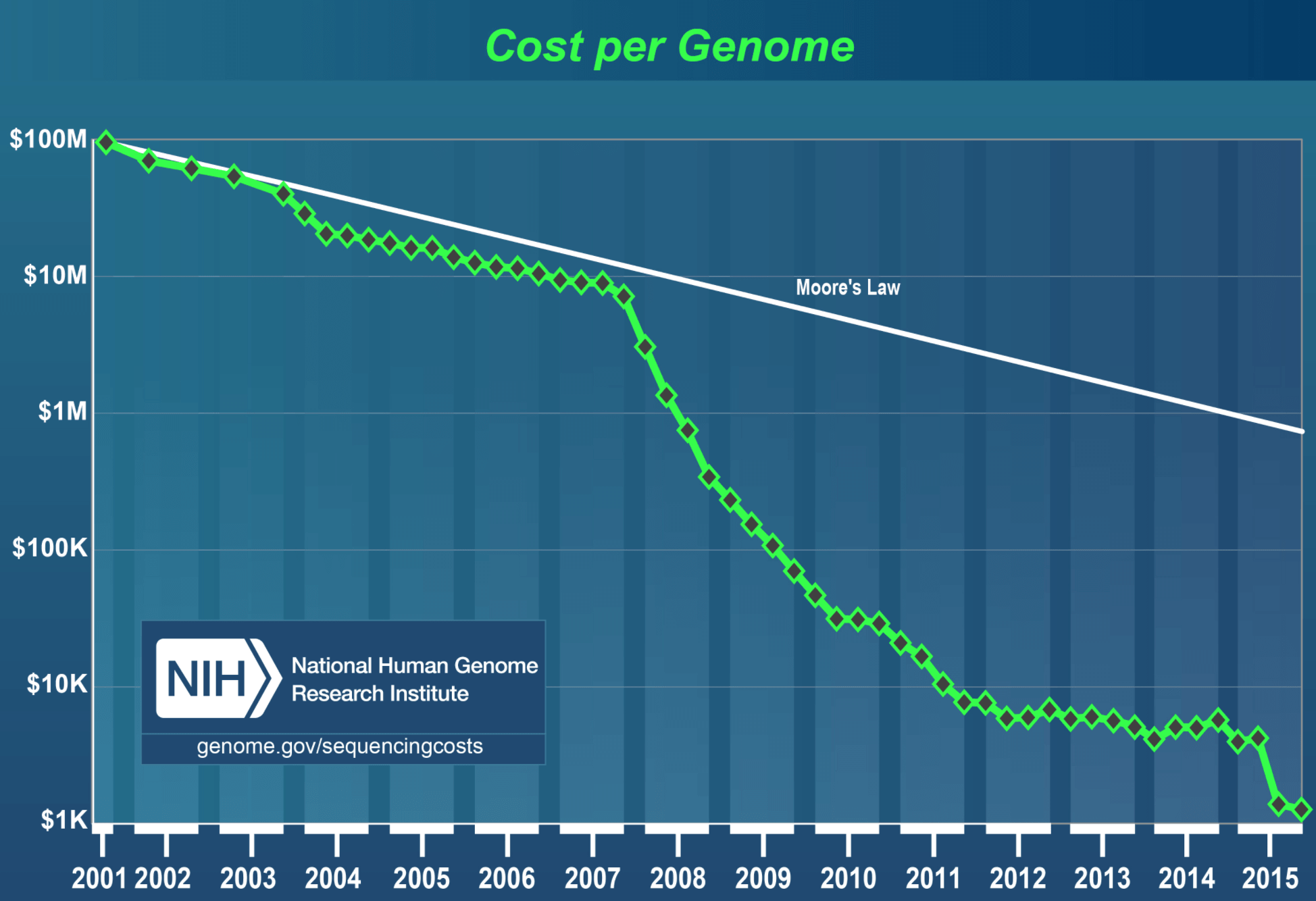
Current applications of machine learning in genomics appear to fall under the following two categories:
- Genome sequencing (particularly as it applies to precision medicine): Researchers are using machine learning to identify patterns within high volume genetic data sets. These patterns are then translated to computer models which may help predict an individual’s probability of developing certain diseases or help inform the design of potential therapies.
- Direct-to-Consumer genomics: This category encompasses companies who offer genomic sequencing services to individual consumers. Companies are using machine learning to achieve greater depth in the interpretation of genetic information such as how an individual’s genes may impact their weight.
Next, we’ll explore four major areas of current machine learning applications in genomics.
AI and Machine Learning Applications in Genomics
Current applications of machine learning in the field of genomics are impacting how genetic research is conducted, how clinicians provide patient care and making genomics more accessible to individuals interested in learning more about how their heredity may impact their health.
1 – Genome Sequencing
Whole Genome Sequencing (WGS) has grown as an area of interest in medical diagnostics. Next Generation Sequencing has emerged as a buzzword which encompasses modern DNA sequencing techniques, allowing researchers to sequence a whole human genome in one day as compared to the classic Sanger sequencing technology which required over a decade for completion when the human genome was first sequenced.
Companies like Deep Genomics, use machine learning to help researchers interpret genetic variation. Specifically, algorithms are designed based on patterns identified in large genetic data sets which are then translated to computer models to help clients interpret how genetic variation affects crucial cellular processes. Examples of cellular processes include the metabolism, DNA repair, and cell growth. Disruption to the normal functioning of these pathways can potentially cause diseases such as cancer.
Founded in 2014, the Toronto-based startup has received a reported $3.7 million in seed funding from three U.S. venture capital firms: Bloomberg Beta, Eleven Two Capital and True Ventures. In fact, the Deep Genomics backers reportedly advised the startup to continue to grow in Toronto instead of relocating to Silicon Valley.
The decision may reflect the Canadian government’s recent allocation of $125 million (canadian dollars) towards a Pan-Canadian Artificial Intelligence Strategy. As of April 2017, Deep Genomics has referenced seven publications related to its technology, the majority of which predict or infer potential genetic variants. However, specific outcomes of this research within the context of diseases or potential therapies have yet to be reported.
2 – Gene Editing
Gene editing is defined as a method of making specific alterations to DNA at the cellular or organism level. CRISPR is a gene editing technology that offers a faster and less expensive way of conducting gene editing. In order to use CRISPR, researchers must first select an appropriate target sequence. This can be a daunting process involving many choices and unpredictable outcomes. Machine learning offers the capability to significantly reduce the time, cost and effort necessary to identify an appropriate target sequence.
London-based Desktop Genetics is a software company at the convergence of AI and CRISPR. Founded in 2012, the company has accrued $5.8 million in total equity funding from 7 investors which include a mix of accelerators, venture capital firms and biotech company and DNA sequencing veteran Illumina.
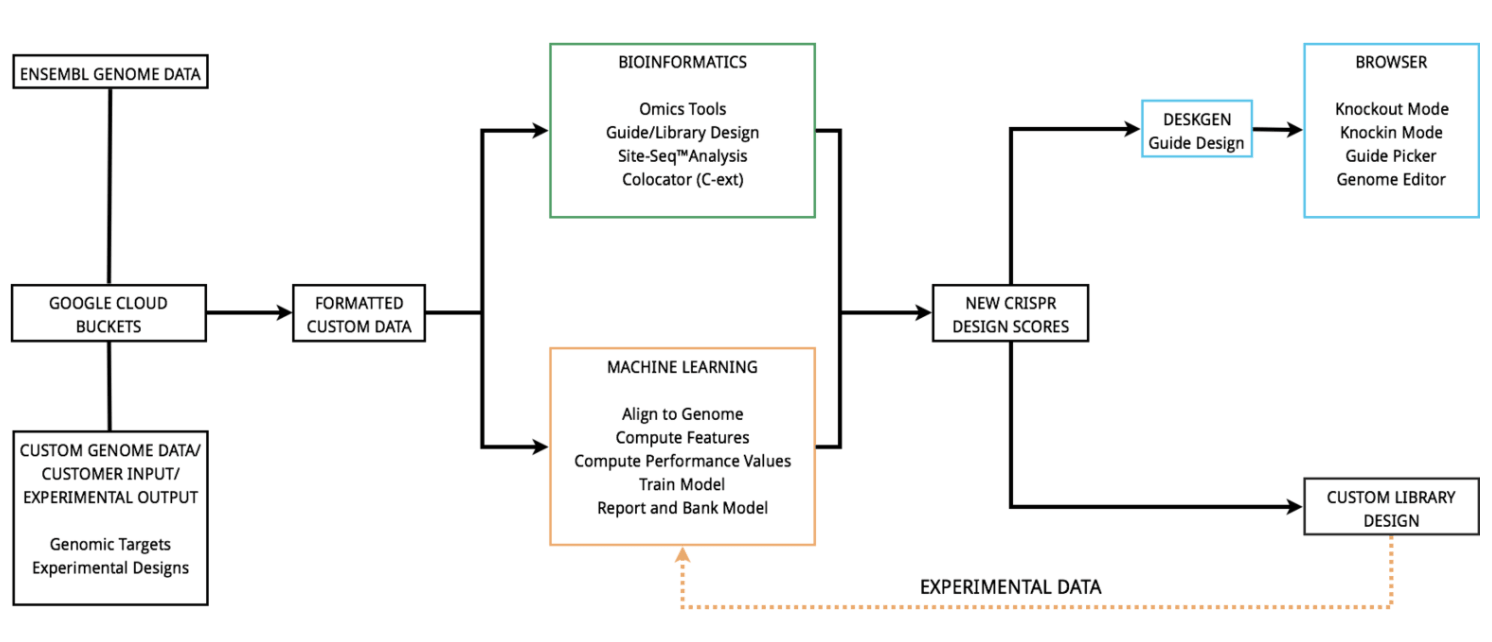
The company reports two key findings from a recent study: 1) an increased amount of training data improves the accuracy of an algorithm in its ability to predict CRISPR activity and 2) the accuracy of the model decreases when applied to a different species, such as humans vs. mice. Neither of these findings are particularly surprising, and Desktop Genetics acknowledges that extensive research will be necessary to continue to improve processes and to push the boundaries of how machine learning can impact CRISPR.
3 – Clinical Workflow
There are often gaps in the patient data available to the different members of a healthcare team serving a patient. This challenge has sparked an interest in using machine learning to improve the efficiency of the clinical workflow process. Intel has designed an Analytics Toolkit which integrates machine learning capabilities into the clinical workflow process. The Transformation Lab at Intermountain Healthcare in Salt Lake City, Utah collaborated with Intel in an effort to more efficiently integrate genetics in breast cancer treatment and patient care.
The partnership resulted in the development of an algorithm to measure factors such as a patient’s level of risk for developing multiple cancers. A workflow model was developed using machine learning with four major components:
- A centralized database of genomic data that is linked to “clinical and patient data”
- All clinicians and genetic counselors have access to Electronic Health Records (EHRs)
- All data from genetic tests are integrated into EHRs
- Clinical Decision Support tools (CDS) are operational and accessible. Examples of clinical decision support include family health histories, screenings and past clinical data.
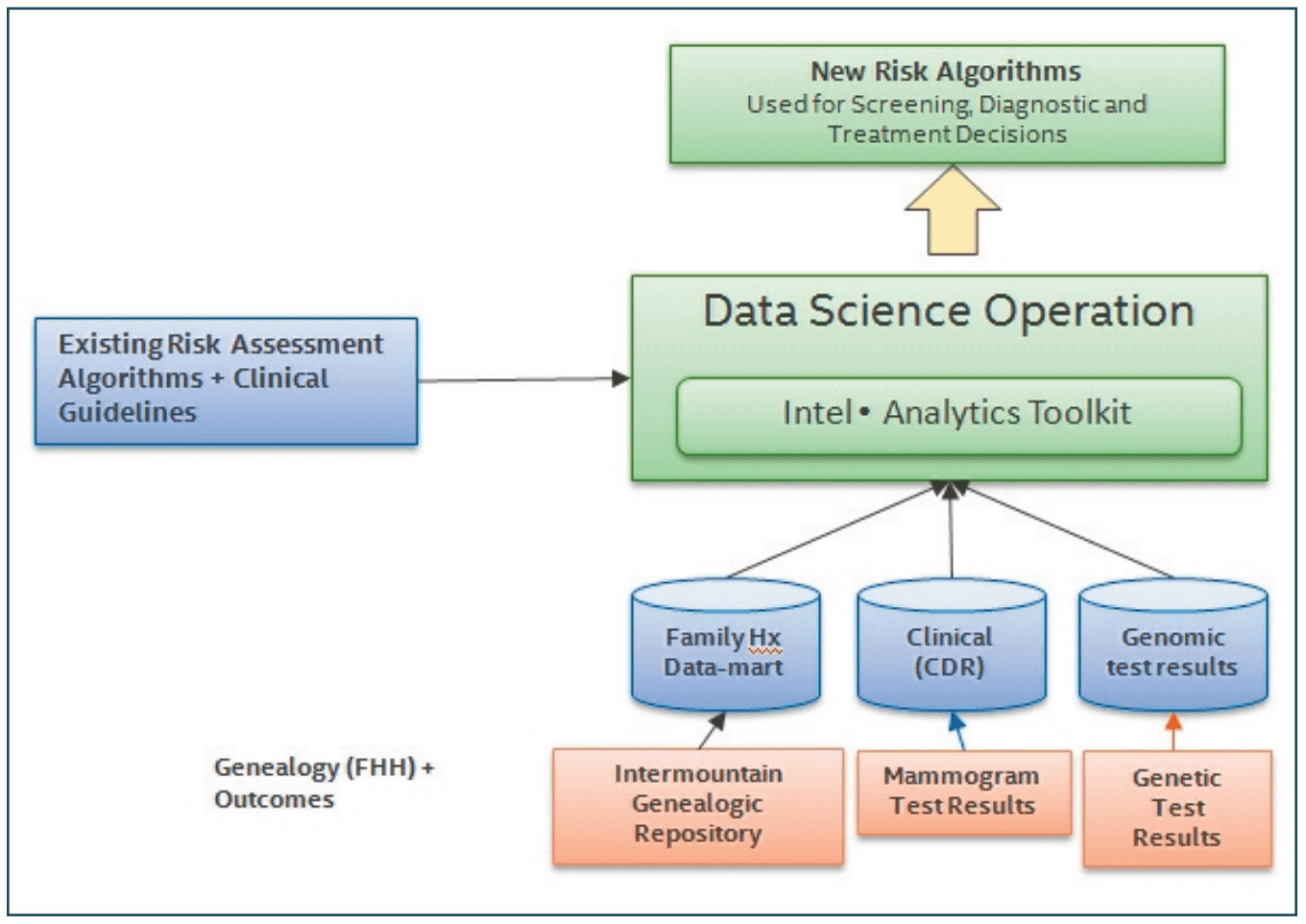
Since the launch of the Transformation Lab in 2013, it has been reported that a patient can be screened for a sample workflow in 3 to 5 minutes.
The workflow model developed using machine learning (described above) contributed to improved data accessibility.
While it can be assumed that the process is now faster based on the fact that data was not previously centralized, it is unclear from the report as to how long the process took before the implementation of the new model.
The venture capital arm of Intel, Intel Capital, has reportedly invested in over two dozen AI entities. The firm’s latest three AI company investments totaled roughly $133.35 million in Series A and B funding, perpetuating a trend of relatively high AI investment in the healthcare sector (compared to other industry verticals). Despite it’s regulatory issues and complex sales cycles, many of the biggest players in artificial intelligence seem to be affirming the massive economic value of AI in healthcare.
We’ve looked at the relatively high investment in AI in healthcare in our article analyzing “AI industry” market segments.
4 – Direct-to-Consumer Genomics
One particular estimate postulates that by 2025 the predictive genetic testing and consumer genomics market worth will reach $4.6 billion. Contributing factors to the anticipated market expansion include a growing awareness of how genomic tests can be used to help determine the likelihood of developing a particular disease and may with proper guidance.
Despite concerns around regulation and the role of health professionals in helping individuals interpret their test results, direct-to-consumer genomics is a rapidly growing industry and leading companies such as 23andMe and Ancestry.com are becoming household names.
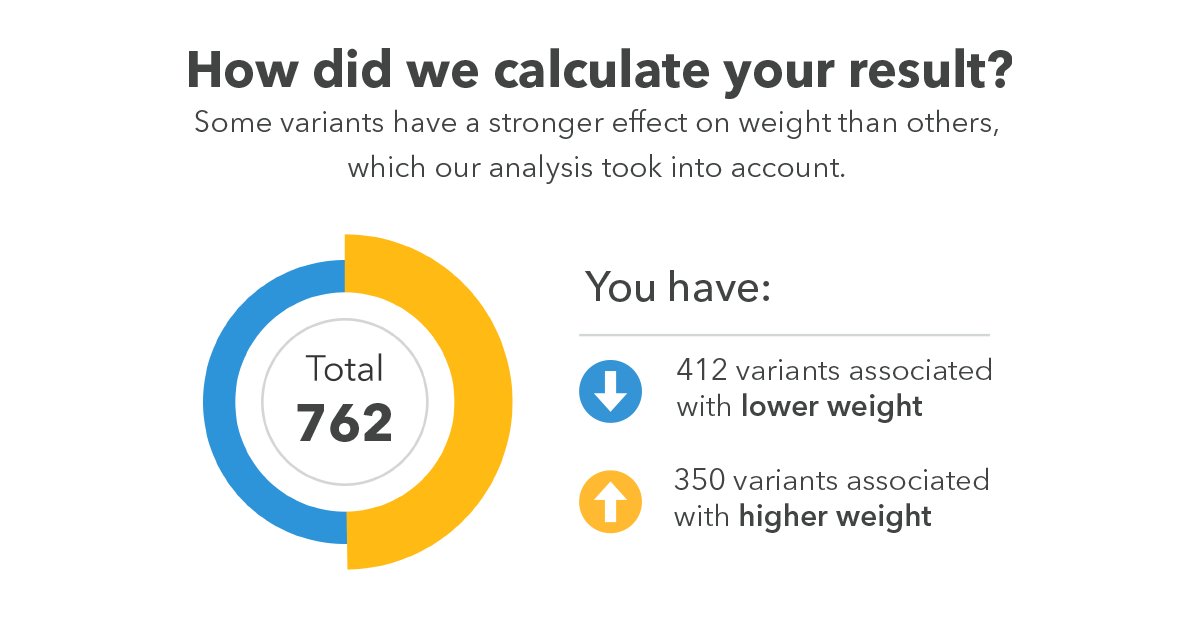
23andMe recently combined data from 600,000 research participants with machine learning to develop a model for a Genetic Weight report. The report is designed to provide personalized analyses of how an individual’s genetic material may impact their weight.
Unique factors used to develop each report include “genotype, sex, age, and self-identified primary ancestry.” These factors would be determined either from a customer’s genetic information or derived from a survey that would be administered prior to accessing the report.
With over 2 million customers to date, it will be interesting to see what economic impact the Genetic Weight report will have on user lifestyle habits, the weight loss industry in general and on the company’s business model going forward. Founded in 2006, the human genome research company has raised a reported $232.97 million from 16 investors which include Johnson and Johnson, Google and Illumina.
Future Applications of Machine Learning in Genomics
Future applications of machine learning in the field of genomics are diverse and may potentially contribute to the development of patient or population-specific pharmaceutical drugs, help farmers improve soil quality and crop yield, and contribute to the development of advanced genetic screening tools for newborns.
While the possibilities might be endless, we’ve chosen three applications that seem promising and are probably worth keeping on the radar for business leaders with a keen interest of the business of genomics:
1 – Pharmacogenomics
A natural progression of precision medicine, pharmacogenomics is an emerging field that looks at the role of genetics in the context of how an individual responds to drugs. While the field is still quite new, there is evidence of research involving machine learning. For example, what is regarded as the first study to apply machine learning models to determine a stable dose of Tacrolimus in renal transplant patients was published in February 2017. Tacrolimus is commonly administered to patients following a solid organ transplantation to prevent “acute rejection” of the new organ.
2 – Newborn Genetic Screening Tools
Analysts anticipate that newborn genetic screening will become standard practice over the next decade. Data collected at birth would be seamlessly integrated into the individuals EHR, and non-invasive screening capabilities for particularly diseases such as Down Syndrome would be available to women during a pregnancy.
The Newborn Screening Center at the National Taiwan University Hospital implemented machine learning to improve the accuracy its web-based newborn screening system for metabolism defects. Results of the study showed that instances of false positives were reduced “from 21 to 2 for phenylketonuria (PKU), from 30 to 10 for hypermethioninemia, and 209 to 46 for 3-methylcrotonyl-CoA-carboxylase (3-MCC) deficiency.”
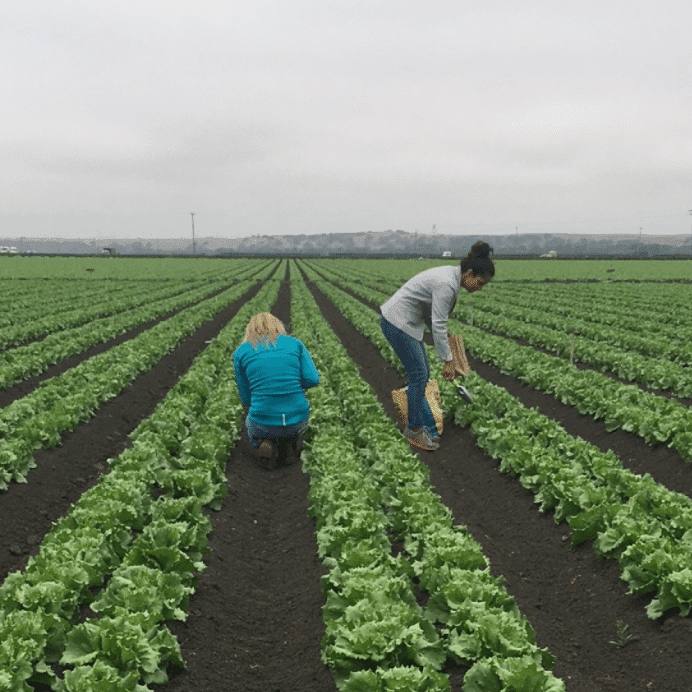
3 – Agriculture
The potential for genomics to help improve soil quality and crop yield is an emerging area of interest and promise within the sphere of agriculture. Through its Illumina Accelerator, Illumina lended support to California-based startup PathoGn, Inc. in 2015. The startup is described as a combining genomics and machine learning to build diagnostic tools aimed at predicting and preventing diseases in crops.
Today, the company is know as Trace Genomics and seems to have shifted its focus more towards soil health.
If genetic data can be used to predict the yield or health of crops (and the resulting impact on soil) could help farmers better predict and optimize yields. Such innovations used at scale could also ramp up the global improvements in crop yields that have resulted from past genetic alterations.
Concluding Thoughts on AI, ML and Genomics
Machine learning in genomics is currently impacting multiple touch points including how genetic research is conducted, how clinicians provide patient care and the accessibility of genomics to individuals interested in learning more about how their heredity may impact their health.
Efforts to implement AI to help accelerate the path from bench-to-bedside and make precision medicine more commonplace is smart business (readers will a deeper interest in this topic may want to explore our recent article on the applications of machine learning in medicine and pharma). These efforts may also prove profitable for businesses that are able to deliver tangible and sustainable solutions to the challenges facing precision medicine.
While there is great promise, making the case for precision medicine is still an uphill battle with many clinicians seeking greater clarity around clinical utility and insurance companies not viewing it as a necessity.
Therefore, the data interpretation capabilities accessible through machine learning will need to be complemented by education and clear explanations of the utility and value of this technology.
Pharmacogenomics is a main area of emerging applications of machine learning in genomics but this is just one example and potential future applications are diverse. However, with limited data on outcomes, time will tell which fields stand to gain the greatest benefit from investing in AI.
We will continue to follow the field of the genomics closely as we suspect this will be an active field for more machine learning applications in the near future. It’s possible that the world’s largest drug companies (whose AI initiatives we have tracked and written about) will be among the biggest financial backers – and acquirers – of the innovative AI genomics companies that emerge in the coming years.
Related Healthcare Interviews on Emerj
At Emerj, we serve a very specific audience: Business leaders who care about the real economic and strategic advantages of AI. Not “tech fans” or “startup junkies,” but people with companies and departments to run, profits to be made, and competitors to be outwitted.
That’s why our coverage focuses so much on real-world applications, quotes from real experts, and hard numbers (dollars, percentages, timelines, etc). One of the ways to serve our business readers best is through our podcast called “AI in Industry,” where we interview real executives, investors and researchers, and probe their minds for the real trends and challenges of applying AI, and it’s consequences on companies in the present and the 2-3 years ahead.
While the podcast can be found easily on iTunes, and while it’s easy enough to search the “Interviews” section of Emerj.com, we wanted to single out some of our recent “AI in healthcare” interviews that might be of interest for readers who’ve enjoyed this article on genomics:
- Ayasdi’s Sangeeta Chakraborty explores the “success factors” among top hospitals who are adopting AI, and explores strategies for actively bringing AI into traditionally technology-resistant organizations
- Eleven Two Capital’s Shelley Zhuang discusses some of the unique regulatory challenges of AI healthcare innovations, and how smart companies are overcoming them
- Your.MD’s Matteo Berlucchi shares predictions about the future of the healthcare experience, with both consumer and hospital uses of artificial intelligence