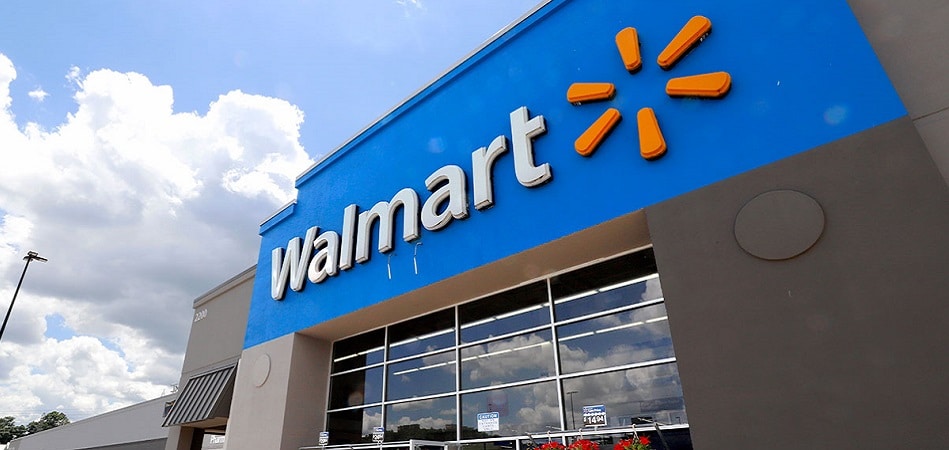
Many of the top Fortune 500 retailers have begun using AI and ML to solve business problems for various departments. Walmart and Costco share one in grocery stocking, which includes the freshness and condition of the products along with timing the restocks for peak hours.
It can be difficult to keep track of every new tech initiative from the leading retailers as they do not keep all of their information public. That said, they still announce enough of their initiatives to get a good idea of the way they are currently developing.
In this article, we discuss three of the top retailers in the U.S. and how they are leveraging AI, ML, and data science to solve business problems. These problems range from sales recommendations to trying on products virtually. We also use the information the company provides to make inferences about how these companies may want to develop their AI in the future.
The companies we cover include:
- Walmart and the Intelligent Retail Lab. This is their largest attempt at instrumenting AI into a brick and mortar store.
- Target and their initiatives to boost eCommerce recommendations along with a virtual product-trying application.
- Costco and their “future state bakery experience,” or ML-powered method of keeping track of fresh food stock and reducing waste by baking a recommended amount of product.
Our look into these top retailers starts with Walmart and their latest attempt to fully digitize a store.
Walmart’s Intelligent Retail Lab and Robot Monitors
The Intelligent Retail Lab is a Walmart store equipped with thousands of cameras that relay information about the stock or condition of products as well as customer shopping behavior. The cameras send this information to a database for a machine learning model that is constantly learning more about managing the store. Walmart’s tech incubator Store No8 developed the lab as an attempt to explore the possibilities of AI in retail.
A Walmart newsroom reporter asked Mike Hanrahan, CEO of Intelligent Retail Lab, about how the employees of the instrumented store felt about the changes. With regards to working with the AI’s alerts and recommendations, Hanrahan said:
“We think it’s something our associates will be excited about. The technology has been built to improve associates’ jobs, to make their jobs more interesting, to help them alleviate some of the mundane tasks. AI can enhance their skillset in a very rapidly changing world.”
Walmart seems to be exploring a few major AI functions by instrumenting a 50,000 square foot store with cameras and sensors. These include:
- Product inventory and availability
- Opening and closing registers
- Determining the availability of shopping carts
The lab is able to detect if certain products need to be restocked as they are taken off the shelves by customers. Once the stock becomes visibly low to any of the many cameras in a given aisle, the system will alert the staff to restock the products that are running low.
Additionally, the system can keep track of the discoloration of bananas across the day. Once the system recognizes that the bananas have become too brown to keep on the shelves, it sends a similar alert to the staff to send someone to throw them away.
Walmart claims the Intelligent Retail Lab’s system can determine when more cash registers need to be opened. Though they do not elaborate much further on this, we can infer that the cameras watch for a light-up signal above each register to determine whether it is open, and compare these numbers with the volume of customers waiting to check out. This may result in an alert for a human employee to come to operate a register if not all of the registers are automated.
The following video is a short walkthrough of how the cameras placed around the Intelligent Retail Lab communicate product and stock information back to the large data center in the back. The speaker also emphasizes the utility of experimenting with using AI across the Walmart network, as well as maintaining customer privacy:
Additionally, the company claims the lab can check the store for abandoned shopping carts which can also trigger an alert to have an employee gather them back to their designated area. This is likely accomplished the same way as it would detect a “register open” signal. It would need to be trained on high resolution images of shopping carts both from historical data and real-time data from the lab’s cameras.
Then, the lab could detect the presence of abandoned or empty shopping carts and keep track of how many there are. The staff may set the system to alert them when the amount of carts reaches a certain number.
Target’s Current AI Initiatives
Target Corp. has three active initiatives that involve using machine learning or AI to improve business processes or increase customer satisfaction. They work on new AI applications and algorithms to drive sales, acquire smaller tech companies to hire on their leadership, and partner with other AI firms to offer new services to their customer base.
Ecommerce Sales Recommendations
According to their corporate website, Target has worked on machine learning algorithm to match a product’s webpage with other complementary products to be advertised on that page. The company claims one of their interns is working on developing this algorithm, however it is likely that a larger team would be required to fully develop and implement such a model. It is because of this that we can infer that Target is at least attempting to develop a larger scale ML-powered solution for optimizing their ecommerce presence.
In theory, Target could use machine learning to drive sales where the site lets the customer know “people who enjoyed this also bought …” for example. The company likely would need a significant amount of ecommerce sales data, which they would use to train the machine learning model to recognize shopping patterns in the customer base.
Targeting Advertising Spend and Acquihires
Another of Target’s initiatives is building their data science teams through acquiring smaller AI firms and adding them to their team. Acquiring consists of buying out the smaller company and then finding the best business areas for their former leaders to work at within Target. The company has been making these types of strategic acquisitions since 2014, and they may see improvement in their ability to innovate on and maintain their current AI solutions.
One example is Target’s most recent acquired company, Kvantum. Prior to the buyout, Kvantum developed machine learning models for finding the best ways to spend advertising budget. We can infer that Target sought out Kvantum in order to improve on this capability for themselves.
This would allow Target to decide where to advertise and to which types of customers more easily. For example, the machine learning model could predict and compare ROI of spending on TV or Youtube advertisements if a marketing leader specified the consumer demographic they wanted to market to.
Virtually Trying Products with Target Beauty Studio
Target is also leveraging AI by allowing their customers to interact with their products virtually using augmented reality (AR). On desktop and mobile, Target customers can visit the Target Beauty Studio to virtually try on makeup and other cosmetics such as false eyelashes.
The customer uses a smartphone camera or a webcam to allow the studio app to scan their face. Then it will be able to direct or “map” high resolution images of the products onto the user’s face. Target claims this gives the customer a good impression of how the product will look when used on their face.
Target worked with PerfectCorp, a Taiwanese machine vision firm known for their face mapping technology that combines AI with AR. They claim to be able to map colors and tints of liquid products onto a user’s face in order to give them an idea of what it might look like when applied to their skin.
This is likely accomplished by judging the size and shape of the user’s face before applying the shade to it at a realistic level of opacity. The opacity would depend on the product’s coverage as well as the customer’s skin tone, and would ideally result in an accurate test image.
Costco Bakery Demand Forecasting
Membership warehouse club retailer Costco uses machine learning to maintain productivity and sustainability in their fresh foods department. Costco donates all of its unsold or damaged food, and because of this it is even more costly to produce more fresh items than are necessary. They worked with SAP to solve this problem with a demand forecasting algorithm that purportedly helps managers ensure the right amount of fresh products available when customers pass by.
Costco bakery managers need to anticipate the demand for each item on the menu that needs to be produced each day. Before SAP’s solution, these managers would need to create a production plan on paper by reviewing sales and trend reports. They would also need to research local events and the past experience of their coworkers.
Costco refers to this experiential knowledge as “tribal knowledge.” The plans were adjusted daily based on carryover from the previous day, damaged items, or destroyed items before bakery teams could get started in the morning.
The design for this solution was led by the SAP AppHaus, an offshoot of SAP’s solutions that focuses on ease of use and collaborating with end-users during development. When Costco was ready to start working on a new solution, they brought in Jeff Lyons, Senior Vice President of Fresh Foods, and several bakery managers and supervisors from their bakeries.
These Costco bakery experts would go on to be end-users of the solution, so AppHaus wanted their input on when customers buy certain products and how often. They interviewed each employee in-depth and shadowed some of them during work. Once SAP had enough data on the daily tasks of a bakery manager, they were able to co-create a new bakery experience with the Costco employees.
The company calls this solution a “future state bakery experience,” which is a tablet app that displays data and insights to bakery managers and digitizes manual processes. The app uses machine learning to provide a planning forecast for each bakery menu item. This forecast determines how much of each item is to be baked when, automatically adjusted for carryover and damaged goods.
This way, the bakery staff always has an ideal “future state” to have the bakery be in for when new customers arrive, and the plan to put the bakery in that state is automatically generated and updated.
The machine learning model used to develop the solution app was initially trained on seven years worth of sales and promotional data, and also leveraging influencing factors such as weather, holidays, and sporting events taking place near the store. Additionally, SAP likely trained this model on the data they created when collaborating, interviewing, and shadowing the bakery managers. This purportedly allowed Costco to accurately forecast demand for each bakery item made.
Below is an image of SAP’s solution app running on a tablet. It displays an overview of sales and demand projections while charting sales as they happen in the middle of the screen. The user can also navigate to the top selling items and which items need to be restocked the soonest:
The software that makes SAP’s app functional is likely a predictive or prescriptive analytics software powered by the machine learning algorithm they developed with Costco.
This is because it is able to make predictions on sales from past historical data, and is made to recommend a specific course of action depending on the state of the bakery. While neither SAP nor Costco make this clear, we can infer that the app is at least based on these types of artificial intelligence technologies given the use of a machine learning algorithm.
SAP claims the solution is still helping Costco meet the demand to always have fresh and available products while reducing waste involving unsold food. They also claim to be able to find specific insights from enterprise data such as when customers will visit the store in relation to a sporting event.
Costco is currently integrating this new solution in over 500 bakeries in the US. They are also exploring similar solutions for business challenges in the deli and food court areas of their stores.
Header Image Credit: Transport Topics