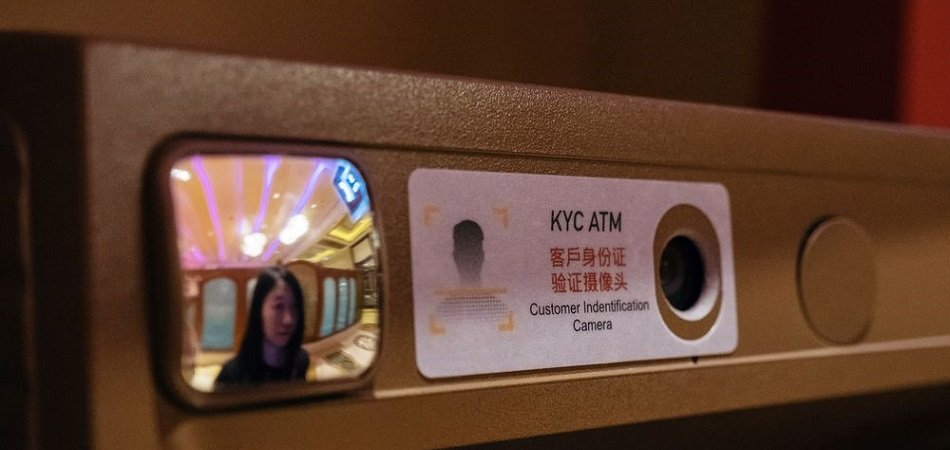
Facial recognition software is making its way into the mainstream, with consumer applications such as the ability to unlock one’s smartphone with their face. The banking sector has been at the forefront of enterprise adoption of AI since machine learning became the hot topic of the business world in the early years of the decade; as such, it makes sense that facial recognition technology would start to make its way into banking.
There are a handful of companies offering facial recognition software to banks that at face value seem to have the requisite talent in their C-suite that we look for when vetting a company on their claims to leveraging AI. These companies offer software with applications ranging from physical security to the ability for customers to make withdrawals with their faces.
Facial recognition is one of numerous ways banks can decrease friction in their customers’ experience and increase efficiency and accessibility. Some experts think that this is how banks can succeed in the future as AI and other technologies make more and more services accessible without any down time.
For example, we spoke with Sindhu Joseph, CEO at CogniCor about how banks may need to transform their business to keep up with evolutions in AI and customer expectations on our podcast, AI in Banking. When asked about why she feels that efficiency and personalized customer service would be deciding factors about which banks would win the market of the coming decade, Joseph said,
“People don’t need banks anymore, what they need is banking. And if banks understand that, I think that’s where everyone is going, and if they provide that I think that is going to be one of the biggest differentiators. … With the likes of Amazon, Netflix, Uber, all of these services, you have instant, … personalized service for all of these. So any service that I sign up for today, I would expect these kind of characteristics. … Since customer expectations are growing at these angles, I think banks need to step up their game to provide this kind of 24/7 accessible, instant, transparent, personalized service.”
The State of Facial Recognition in Banking
That said, what we’ve found is that the application is relatively nascent in banking. Yitu Technology is the largest company listed in this report, a small Shanghai-based enterprise at roughly 200 employees. They employ a number of machine learning researchers and were co-founded in 2012 by a PhD in Statistics from the University of California. That said, it’s unclear how much of their staff works on their facial recognition application, which they call the YITU Dragonfly Eye Intelligent Security System.
The other companies covered in this report are startups with varying levels of funding and talent:
- Cloudwalk has raised $507 million as of September 2018. It’s an impressive feat, but they seem to only now be acquiring the talent necessary to offer the facial recognition software they claim to offer. They also hold contracts with Bank of China and Bank of Chongqing. Aside from their president, who holds a PhD in Electrical Engineering from the University of Illinois – Urbana Champaign, the company doesn’t seem to employ machine learning experts and data scientists at this time. It also doesn’t list any case studies, which again indicates that the company is really just starting to get their bearings. That said, their funding really shouldn’t be understated.
- IntelliVision employs numerous people with Master’s degrees and a few with PhDs in computer science, hard sciences, or statistical fields, which makes it likely that their software leverages machine learning. This bodes well for them and their clients.
- FaceFirst doesn’t seem to employ any data scientists nor people with the academic background that is generally necessary for working with machine learning. We want to make it clear, however, that this doesn’t mean FaceFirst is not genuinely offering AI software. They very well might be. We merely believe banking executives should be aware of the talent density at companies, especially in this day and age when many AI vendors are less than truthful about their claims to leveraging AI.
In this report on facial recognition in banking, we’ll look at companies claiming to offer software for the following two applications:
- On-location Security: Preventing unauthorized personnel from gaining entry to certain areas of a bank and catching suspicious behavior on camera
- Identity Verification and Account Withdrawals: Allowing customers to make withdrawals from their bank accounts at ATMs
For more information on how AI applications can create more varied user experiences that drive engagement, our readers can download the Executive Brief for our AI in Banking Vendor Scorecard and Capability Map report.
We’ll begin by exploring the applications for physical security, starting with IntelliVestion:
On-location Security
IntelliVision
IntelliVision offers software called Face Recognizer, which it claims can help banks offer convenient and secure customer service using machine vision.
We can infer that IntelliVision’s machine learning model for Face Recognizer was trained on thousands of images and bank camera video clips showing human faces at multiple angles and in multiple lighting conditions. The faces within these images and videos would have been labeled on specific facial features.
The names of known customers and staff would also be used to label these images if a bank manager or data scientist uploaded them prior to training. IntelliVision would then have run these labeled images and videos through Face Recognizer’s machine learning algorithm. This would have trained it to discern the patterns of 1’s and 0’s that, to the human eye, form a person’s face as displayed in a picture or live footage from a camera. In this case, the camera is a security camera somewhere within the bank.
The user could then expose the software to faces that are not labeled using a camera attached to a self-service device. The algorithm behind the software would then be able to recognize a customer’s face as shown on their ID card or similar stored verification image. The system can then validate the customer’s transaction once their face is recognized. For example, a customer could insert their card into an IntelliVision client’s ATM, and the facial recognition software would verify that the actual account owner is trying to access their bank account with a camera on or near the ATM.
A user could then expose the software to faces that are not labeled using a security camera. The algorithm behind the software would then be able to recognize a customer or employee’s face when they appear in the camera’s view. The system can then verify their name if it is uploaded into the system and record the person’s face to a shortlist of current patrons for the time they are inside the bank.
IntelliVision claims banks can integrate the software into a database containing images of faces of customers and bank staff.
Below is a short 1-minute video demonstrating how IntelliVision’s Face Recognizer works:
IntelliVision does not make available any case studies showing a bank’s success with the software. IntelliVision does not list any major banks as clients, but they have raised $6 Million in venture capital and are backed by Benhamou Global Ventures and MIT Alumni Angels of Northern California.
Krishna Khadloya is VP of Engineering and Products at IntelliVision. He holds an MS in Computer Science from University at Albany, SUNY. Previously, Khadloya served as VP of Engineering at MobileIron.
FaceFirst
FaceFirst offers a namesake software which it claims can help banks control access to sensitive areas within their buildings using machine vision.
While access control is the most prominent use case for FaceFirst in this sector, the company claims banks can benefit from the software’s alternative purposes. These include authorizing customers’ identity to ensure security, delivering mobile notifications to the security team, and recognizing important customers to ensure they are treated with extra care.
We can infer FaceFirst’s machine learning model was trained on thousands of images and videos of human faces from different angles and in different lighting conditions. The images and videos would then be labeled according to which protected areas of the client’s bank they have access to if any.
These labeled images would then be run through the machine learning algorithm and would have trained it to recognize the sequences of 1’s and 0’s that appear as a person’s face in live footage from a camera or an image. The camera would be attached to a terminal that controls the lock on a door to the protected area.
A user could then approach a security camera using the software and try to gain access to the protected area by looking into it. The algorithm behind the software would be able to recognize the user’s face and discern if they are authorized to access any protected bank areas. The system can then allow or deny access based on how their face is labeled, if at all, in the system.
FaceFirst claims clients can integrate the software into an existing database of images and footage of the faces of important people within their company.
Below is a short 2-minute video demonstrating how FaceFirst’s software can scan faces from a group of people for identity verification. At 1:34, the video depicts a security guard using facial recognition to gain access to a private location within the building he is guarding. This is the same process a bank employee would go through to get to a secure area of their workplace using FaceFirst’s software:
FaceFirst does not make available any case studies showing a bank’s success with the software, nor do they list any prominent banks as clients, but they have raised $9.5 Million in venture capital and are backed by Kayne Anderson Capital Advisors and Innovate Partners.
Gary Brown is VP of Engineering at FaceFirst. He holds an MS in Computer Science from Florida Institute of Technology. Previously, Brown served as Director of Software Development at Guidance Software.
Identity Verification and Account Withdrawals
Yitu Technology
Yitu Technology offers software called YITU Dragonfly Eye Intelligent Security System, which it claims can help banks safely offer facial recognition-based ATM transactions using machine vision.
Dragonfly Eye is Yitu Technology’s software at the core of their intelligent security solutions. Within this software is Yitu’s generic portrait platform, which allows for static and moving facial detection with video comparison. The software also runs a dynamic portrait system capable of real-time surveillance and tracking passers-by. Clients can also make use of Dragonfly Eye’s static portrait system, which Yitu claims can be used to build a database of faces from an urban population or other select groups for easy identification.
Yitu Technology’s machine learning model for Dragonfly Eye was likely trained on thousands of images and clips from ATM cameras of people’s faces. These would also need to be from various angles and lighting conditions to match the wide array of possibilities during a transaction. Each would then be labeled by name and bank account number. These labeled images and clips would then be run through Yitu Technology’s machine learning algorithm. This would have trained the software to recognize the patterns of 1’s and 0’s that appear as a person’s face in footage from an ATM camera.
A user could then approach an ATM with a camera powered by Dragonfly Eye and try to make a withdrawal from their bank account. The algorithm behind the software would be able to recognize the user’s face and determine if they are a customer or not. The system can then validate or reject the withdrawal request based on if the person actually has an account with the bank that owns the ATM.
Below is a short video in which a woman tries to access Yitu Technology’s facial recognition-based ATM by presenting it with a photo and video of herself. The software does not allow her access to her account until she stands in front of the camera, rejecting the photo and video:
Yitu Technology claims users can integrate the software into an existing database of customers’ faces for verification. We could not find a demonstration video showing how Yitu Technology’s software works.
Yitu Technology claims to have helped China Merchants Bank offer cash withdrawals via facial recognition to their customers. Yitu Technology’s software was the answer to the bank’s major problems surrounding facial recognition. These were the handling of multiple images of the same person, identifying faces in front of complex backgrounds, and discerning between multiple faces in the same image. According to the case study, China Merchants Bank was also able to offer facial recognition for online banking transactions.
Yitu Technology also lists Shanghai Pudong Development Bank, and JD Finance as some of their past clients.
Leo Zhu is CEO at Yitu Technology. He holds a PhD in Statistics from UCLA with a specialty in statistical modeling of computer vision and AI. Previously, Zhu was a postdoctoral fellow at the AI Laboratory at MIT.
CloudWalk Technology
Cloudwalk Technology offers a namesake software it claims can help banks improve the security of their self service transactions using machine vision.
CloudWalk Technology is a Chinese startup led by Xi Zhou, a former professor of the Chongqing Institute of Chinese Academy of Sciences who focuses on artificial intelligence. The company’s facial recognition software is used by China’s state-owned banks and numerous others to identify customers when making transactions at self-service machines. According to their website, Bank of China used CloudWalk’s software to apply facial recognition to self-issuing devices for resident health cards. A resident health card serves as a form of identification attached to a person’s electronic medical records, and is likely linked with health insurance or banking information.
We can infer the machine learning model behind the software was trained on thousands of images, videos, and photo ID cards showing human faces from various angles and in various lighting conditions. These images and videos would have been labeled with points denoting the locations of facial features, such as eyes, eyelids, cheekbones, and lips.
These labeled images would then be run through the software’s machine learning algorithm. This would have trained the algorithm to discern the sequences and patterns of 1’s and 0’s that, to the human eye, form the image of a person’s face as displayed in live footage from a camera or an image. In this case, there are internal and external cameras on an ATM for scanning customers’ faces and verifying their face from their photo ID card.
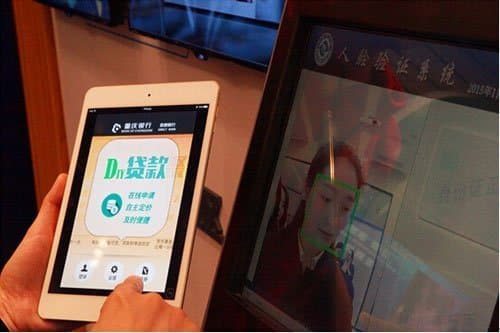
The user could then expose the software to faces that are not labeled using a camera attached to a self-service device. The algorithm behind the software would then be able to recognize a customer’s face as shown on their ID card. The system can then validate the customer’s transaction once their face is recognized. For example, a customer could insert their card into a CloudWalk client’s ATM, and the facial recognition software would verify that the actual account owner is trying to access their bank account with a camera.
CloudWalk does not make available any case studies showing success with the software. CloudWalk lists Agricultural Bank of China, China Constructuion Bank, and China Merchants Bank as some of their past clients.
Xi Zhou is president of CloudWalk. He holds a PhD in Electrical and Computer Engineering from University of Illinois at Urbana-Champaign. Previously, Zhou was a professor at Chinese Academy of Sciences within the Chongqing Institute of Green and Intelligent Technology.
Header Image Credit: Engadget