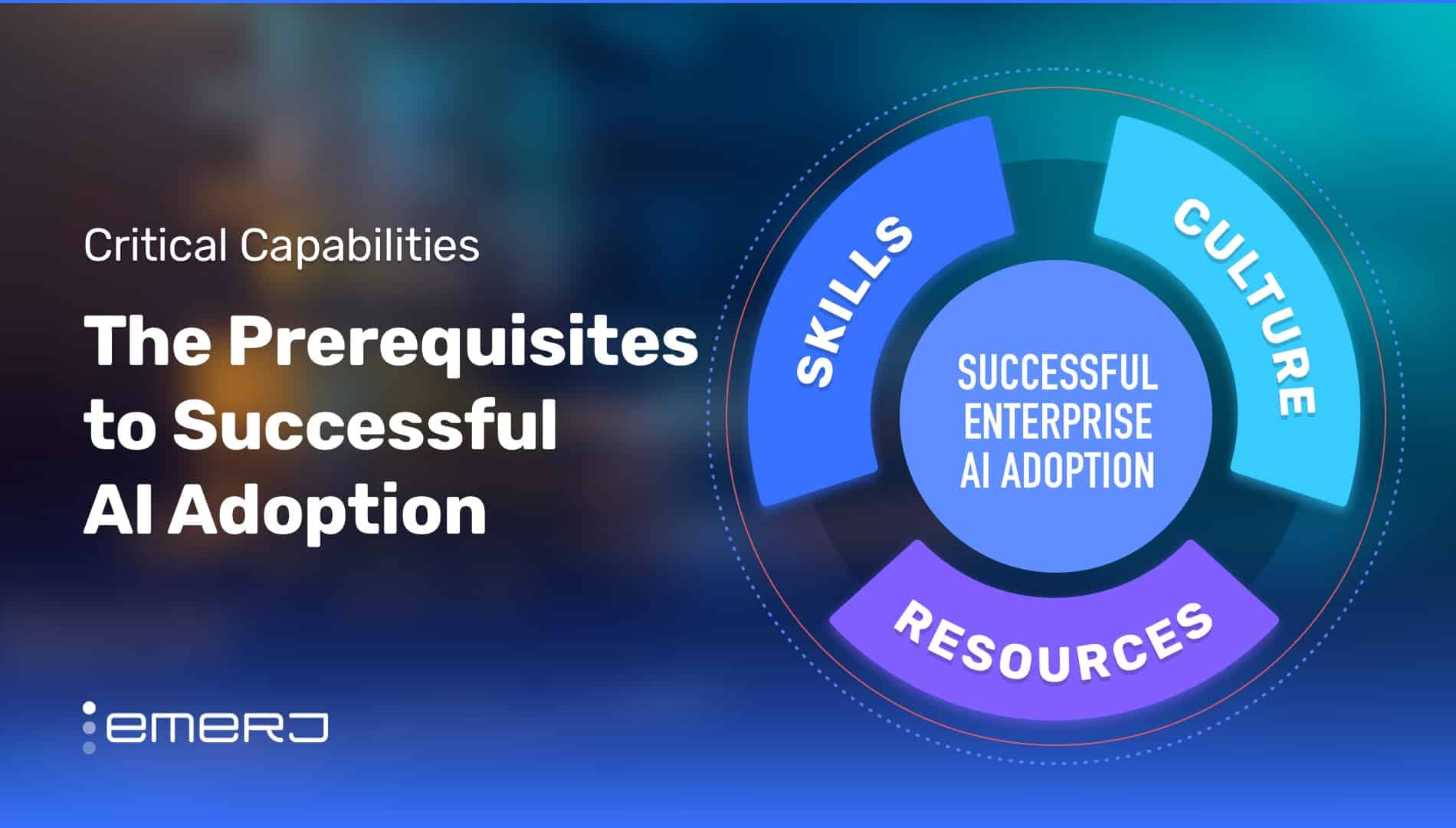
Over the last four years, interviewing hundreds of AI researchers and AI enterprise leaders, we’ve consistently heard the same frustrations about AI adoption said time and time again.
“Culture is hard to change.”
“Leadership doesn’t know what they’re trying to accomplish.”
“Nobody knows what to do with these data scientists we’ve hired.”
etc…
In our one-to-one work with enterprise clients, we’ve taken the most prevalent, recurring challenges to AI deployment and put them together into a framework of “prerequisites” to AI deployment.
We refer to these as Critical Capabilities, and they break down into (1) Skills, (2) Resources, and (3) Culture:
With few exceptions, there are only two main ways that companies can gain a reliable competitive advantage with artificial intelligence:
- Data Dominance: Monopolizing data within a particular business function and/or industry, to make sure that your product is so much better than your competitors that they can’t compete anymore. Read our full article on Data Dominance if you aren’t yet familiar with the idea.
- Critical Capabilities: Building the skills, resources, and culture needed to leverage AI well into the future. These capabilities provide a company with the ability to wield artificial intelligence towards company objectives quickly, with the highest chance of success, and without costly waste or risk.
There are some companies that have achieved data dominance: Amazon, Google, and Facebook, for example. But the tech giants are also the firms that have Critical Capabilities as a core part of their culture. This is one of many of the AI advantages of the tech giants, only compounded by their massive profits and near-monopoly of AI talent.
Most companies, however, are just starting to learn about AI use-cases in their industries; they haven’t even begun to think through the skills, resources, and culture that their company needs if they want to use AI to win market share and achieve data dominance.
In this best practice guide, we break down these Critical Capabilities and provide guidance on how to bolster them within your organization. Because our many podcast guests and AI mentors have played such a role in helping to flesh out these ideas – I’ve made sure to embed and link to a number of our related AI interviews throughout the article below – for readers who want to explore these topics in greater depth.
We begin our discussion with how companies can develop AI-related skills:
1. Skills
1.1 Data Science Skills and Talent
There are a number of different roles that a company is going to need to hire. One of them is data science or AI leadership. Companies cannot bring in a consulting firm and expect them to be an AI strategist at a high level. Companies must have someone in-house with their interests in mind, aligned with their own motives. Companies will need a leader.
When a company decides AI is going to be a part of the business, it needs to gauge its requirements for machine learning engineers. It also needs people to clean and harmonize the data before providing it to these engineers and data scientists. The company will also need IT staff that understands the role of AI in the business and want to be part of that transformation.
1.2 Cross-Functional AI Teams
A company looking to adopt AI will need data scientists, subject-matter experts, and occasionally functional business leaders, such as the Head of Compliance, to be able to gauge the business requirements for an AI project and what the company is willing to spend. This is a cross-functional AI team, and a lack of one is a big cultural reason why AI is hard to adopt in the enterprise.
For example, an oil and gas company that’s looking to use AI to predict where to drill will need to ask how many of their geologists they want to pull into the project and how they are going to work in the AI workflow.
A company then needs to ask what the phases of deployment are. We cover these phases in our report, the AI Deployment Roadmap, which breaks down the three phases of deployment and the seven steps of the data science lifecycle.
1.3 Functional Leaders That Understand AI
Lastly, a company needs functional leaders that have a conceptual understanding of AI. Functional leaders are non-technical and in charge of strategy. This could be a CMO or a Head of eCommerce. Functional business leaders need to understand three things:
1.3.1 How AI Works
Functional leaders don’t need to learn how to code, but they should understand what makes their company’s data valuable and why its format matters. They should understand how algorithms leverage data and what kinds of results they might expect from those algorithms.
Related Interview: Vlad Sejnoha of Glasswing Ventures explores the topic of executive education in depth in his interview on the AI in Industry podcast:
1.3.2 Basic AI Terms
Functional leaders don’t need to know how to code in Python, but they do need to know that Python is a coding language. They need to know that Python can do certain things that C++, for example, cannot do. They should understand what natural language processing is and perhaps a little bit of the difference between machine learning and deep learning. If they don’t even know these fundamentals, it’s going to be very hard for them to contribute to AI projects.
1.3.3 Representative Use-Cases
Functional leaders also need to understand representative AI use-cases in their industry. If it’s eCommerce, for example, they should know what Amazon or Wayfair are doing with AI.
In conclusion, these skills may be the most important of the critical capabilities because they enable all the others.
2. Resources
The second critical capability is “Resources.” Companies need to be aware of two core resources in their business: data access and quality and in-house AI playbooks and guides. We first look at data access and quality:
2.1 Data Access and Quality
2.1.1 Assessing Data Assets
Companies looking to adopt AI need to know which data they have access to and which data they need to bring the AI project to life. For example, an eCommerce company looking to build a recommendation engine needs access to payment data, customer behavior data, historic customer lifetime value, and customer demographic data. It then needs to be able to access this data. Some of this data will be more accessible than others.
2.1.2 Experimenting with Data Assets
A company will then need to begin experimenting with its data assets. The quality of data in part has to do with its value. High-quality data produces some kind of result. Oftentimes, in order to understand how to increase the quality of its data, a company will need to leverage it in some initial projects. That doesn’t mean wasting money on an AI project just for the sake of understanding the data, but companies should use early AI projects as a time to experiment with the data and figure out what facets of it are valuable to them.
2.1.3 Overhauling Format, Architecture, Accessibility
Companies will also need to overhaul the format, architecture, and accessibility of the data in order to create a catalog that allows someone at the company to access the data they need for a given project. We need data governance policies that will go along with this overhaul of format, and architecture, and accessibility. We need policies for who can access what information, what kinds of approvals are required, what kinds of regulatory risks are there, whatever the case may be. So this is another aspect of data access and quality.
Related Interview: Adam Oliner, Head of Machine Learning at Silicon Valley unicorn company Slack, explores strategies for assessing data assets and using them for a competitive advantage in business:
2.2 In-House AI Playbooks and Guides
The second facet to “Resources” in terms of critical capabilities is in-house AI playbooks and guides. Of all of the critical capabilities, this may be the most overlooked.
Realistically, if a company wants to be able to leverage AI well, it needs to have frameworks for maintaining existing AI projects. It needs to know what kinds of teams are going to be needed, what kind of data access is going to be needed, and what kinds of integrations need to take place. For example, if a company is putting together another conversational interface, it should be able to pull up a playbook of how to do it correctly, based on lessons learned the first time around. In addition, a company should have a playbook for how to maintain existing AI applications.
Companies are also going to need a habit of retaining what they learn. When a company launches an AI initiative, no matter if it succeeds or fails, it should ask:
- What aspects of its critical capabilities did it learn about?
- What aspects of its culture made the project harder?
- What aspects of its data are inadequate and made the project harder?
- What made the project succeed?
These playbooks are important because there is no general set of best-practices yet when it comes to AI. It might be another five years or more until the best way to run an AI-enabled anti-money laundering application for retail banking is common knowledge. Building a playbook means that a company retains what it learns from past AI projects, and getting another one off the ground won’t be as challenging.
3. Culture
A lot of the critical capabilities work starts with leadership and incentives. Below are three aspects of company culture that leaders need to set for successful AI adoption:
3.1 Willingness to Experiment and Iterate
This can be thought of as risk tolerance. Business leaders need to understand that AI requires a pretty lengthy experimentation time. Sometimes collecting and accessing enough data to test a project can take five months or longer. As such, business leaders need to be willing to take this risk. This ties to functional leaders having a conceptual understanding of AI. If they have a better idea of the time and resources involved in an AI project, they’ll be more willing to experiment and iterate to achieve ROI.
In addition, a willingness to experiment means leaders at the company understand that AI advantage requires this long term R&D investment, and hopefully, they have a pretty good barometer for when it’s a worthy investment and when it’s not. Also, leaders should accept and encourage deliberate experimentation efforts. They should feel safe letting their data scientists experiment with data and algorithms to achieve ROI.
3.2 Valuing Data
Companies need to think about maintaining, storing, and accessing the data that it already has. There should be protocols for handling and maintaining existing data assets.
There also should be protocols for establishing new data assets. For example, suppose a company starts interacting with customers via chat. It would need to know what data within that interaction should be tracked and where that data would be stored. This is similar to building an in-house AI playbook or guide. Part of that playbook should include ways for collecting and accessing new data.
Another part of valuing data is consistently using it. Companies need to use the data they have if they want to figure out which data is valuable or not.
Having a set of protocols for these scenarios is important. Otherwise, a company might store or format data in ways that actually aren’t useful. As such, valuing data involves deliberate efforts. It involves asking how it’s going to be used and how that use is informing the way it’s stored and made accessible.
3.3 Valuing Cross-Functional Collaboration
Company culture also needs to be one that values cross-functional collaboration. It’s important to have team members hired or opted in for AI team participation.
To explain how this may be done incorrectly, we’ll use an eCommerce company as an example. This eCommerce company is setting up a new fraud detection system. Its data scientists may ask subject-matter experts, in this case, fraud specialists, to join them in meetings about setting up the fraud detection system. As a result, these subject-matter experts are getting less done in their normal jobs, and they feel pressured by that because they feel like their boss is expecting them to do their normal job. Sometimes, these subject-matter experts may just return to their work, and the data scientists are left without the subject-matter expertise they need. This is a case in which cross-functional collaboration isn’t valued at the company.
If it was, upon reaching a certain threshold in the project, the data science team would speak with management, and there would be an opportunity for fraud specialists to be part of the AI team. Their full-time job would be working on the project, and they wouldn’t feel the pressure of fulfilling the duties of their normal job.
Similarly, the company should be able to hire for AI projects. This company may start looking to hire in fraud specialists folks specifically to be part of the AI team. This will set the expectations of any potential hiree for the kind of work they will be doing. They won’t be doing the duties of a regular fraud specialist; instead, they will be educating the data scientists on how fraud detection works.
Related Interview: Shane Zabel, the Cheif AI Officer at Raytheon, explores the dynamics of AI team development and establishing AI project leaders in his interview on our AI in Industry podcast:
Applying the Critical Capabilities Framework
Building these critical capabilities is essential for bringing AI projects to life.
Many (or most) AI proof of concept projects won’t yield a financial return on investment. Their ROI will often be the lessons learned, and the Critical Capabilities developed. The true ROI of early AI projects is learning, skill-building. If this sounds pessimistic, go talk to 10 buyers (not vendors) who have run AI pilots in your sector and see how it went for them. Most pilots are run without any context on Critical Capabilities, without much chance of taking the AI application into deployment, and – worse yet – without any tangible, important lessons learned to help deploy future projects faster.
Before beginning an AI project, ask:
Which Critical Capability Skills, Resources, or Culture, might we need to substantially improve before undertaking this project?
or
What Critical Capabilities can we make a point to substantially improve over the course of this project?
At the end of an AI project or proof of concept, ask:
Have we in any way improved our Critical Capabilities of Skills, Resources, or Culture, over the course of this project?
If the answer is “yes”, then that early AI project could – in some sense – be considered a success. When companies don’t see a financial ROI, they will still be able to have the confidence in knowing that they’re building on these critical capabilities that are going to allow them to leverage AI in the future. While companies should always aim for and ardently measure financial ROI when possible, learning ROI is the only return that is practically guaranteed with early AI projects.
Plug: If your firm is in the process of building an AI strategy or preparing for AI adoption, contact us through our Research Services page, or at research [at] emerj [dot] com.
Special thanks to our many podcast guests who helped to flesh these points out over the last twelve months. Guests with a background in enterprise AI applications helped to mold and improve these points immensely – including Dr. Charles Martin of Calculation Consulting, Vlad Sejnoha of Glasswing Ventures, Sankar Narayanan of Fractal Analytics (one of our most popular podcasts of 2019), and Ian Wilson – former Head of AI at HSBC. Without our longer, open-ended interviews with these guests (and others), the ideas above would be much less succinct and refined.