There’s a lot of buzz about artificial intelligence shaking up enterprise procurement over the next five years. Procurement leaders wonder what elements of their jobs will be augmented – or even fully automated – by the intelligent systems. Which roles are secure from full automation, and how will more “automate-able” jobs have to adapt in the years ahead?
In this article, we’ll explore the facets of the procurement process that are most likely – and least likely – to be automated or assisted by artificial intelligence. By exploring a wide variety of industry sources and expert perspectives, we aim to vet out AI’s opportunities in driving procurement results – as well as the challenges that these changes pose for businesses.
In order to give the reader an easily digestible synopsis of the impact of AI on procurement, we’ve separate this article into three sections:
- The state of cognitive procurement today
- Procurement functions to be rapidly transformed by AI in the near term
- Procurement functions unlikely to altered by AI in the near term
We’ll begin by with looking at current opinions and surveys in the field today:
The State of Cognitive Procurement Today
A 2017 Deloitte survey of 480 procurement leaders from 36 countries found that procurement leaders believe the impact of robotics and automation will increase from 50% to 88% by 2020, and up to 93% by 2025.
Does this mean that procurement jobs will be replaced by digital labor? Not exactly. As Len Prokopets, Managing Director of KPMG’s procurement practice notes, most AI procurement solutions are “not reducing headcount, but driving improved results.” In other words, current AI market trends are automating specific job functions, rather than eliminating full-time employees.
To understand how this works, Prokopets proposes three classes of procurement AI:
- Class 1: Basic Process Automation
- Class 2: Enhanced Process Automation
- Class 3: Autonomic/Cognitive Automation
To see how this actually works, let’s look at job descriptions for “Supply Chain Analyst I”. The job functions that match Prokopets’ Class 1 and 2 are boxed in black, while the job functions that would fall under Class 3 are boxed in red:
Now let’s look at the list of job functions for “Supply Chain Analyst II” from the same company:
Prokopets goes on to suggest that organizations seeking to drive savings through digital transformation will likely begin by automating Class 1 and 2 job functions for efficiency. However, he also says that the biggest savings will come from the predictive analytics associated with Class 3: Autonomic/Cognitive Automation. The excitement around using AI to support Class 3 decisionmaking is why “cognitive procurement” has become the procurement buzzword of 2017.
Cognitive procurement simply means the application of cognitive computing (e.g. data mining, pattern recognition, natural language processing) to some of procurement’s Class 3 job functions (e.g. predicting market prices, analyzing contracts). Deloitte’s CPO survey reveals that a whopping 57% of CPOs already use some form of “intelligent and advanced” analytics for their supplier negotiations, and 80% believe that analytics will have the biggest impact on their business over the next two years.
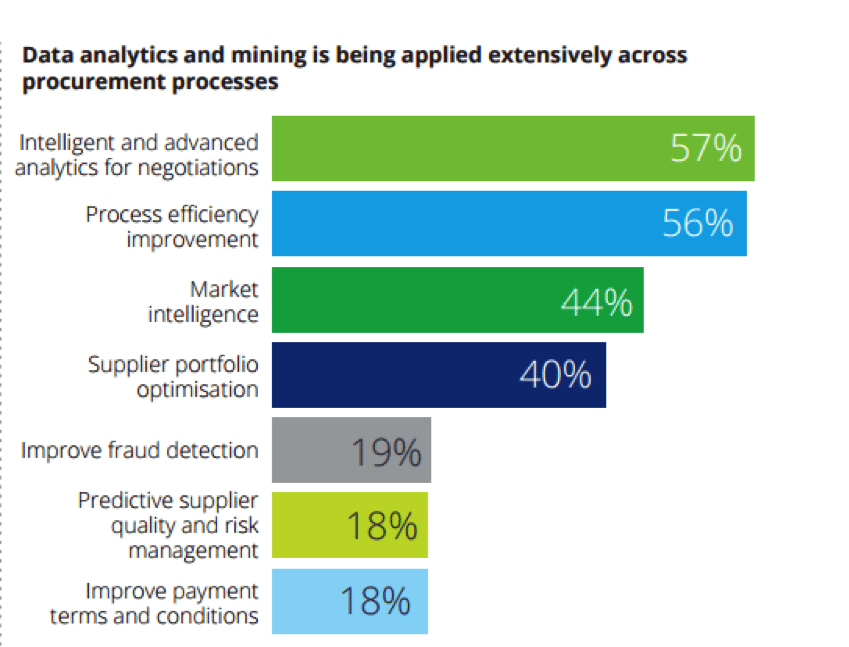
Big players like KPMG, Accenture and IBM are betting that large enterprises will pursue cognitive procurement as the logical next step in their overall digital transformation. But is such a sweeping market transformation realistic?
In the sections that follow, we’ll assess the aspects of procurement that are – and are not – likely to be altered by artificial intelligence and automation, and what that means for companies today.
Procurement Functions Susceptible To Rapid Automation
Here are some ways that AI could rapidly transform enterprise procurement organizations over the next five years:
- Cognitive systems replace supply chain assistants
- Cognitive systems replace purchase order systems
- Cognitive systems replace supplier on-boarding workflows
- Cognitive systems forecast prices, generate contract templates and evaluate suppliers before a human does
If you regularly input procurement-themed keywords into search engines, then chances are good that you’ve been shown ads for IBM Watson Supply Chain:
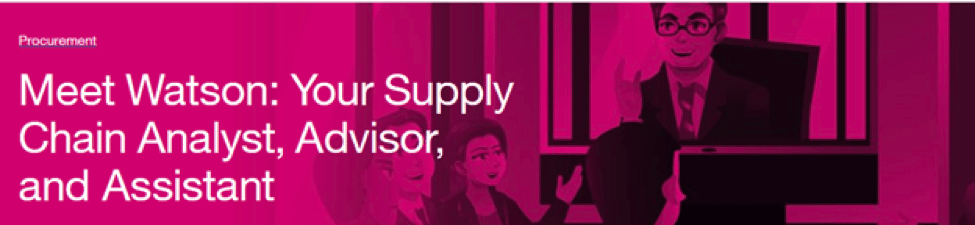
Under the umbrella of cognitive procurement, IBM suggests that Watson will become your organization’s “Supply Chain Advisor, Analyst and Assistant”. If we take the marketing of cognitive procurement seriously, then it’s likely that creating a purchase order, paying an invoice, verifying the location of an order or checking on inventory levels may soon involve far fewer keystrokes. With the help of cognitive procurement systems, human workers will simply approve or modify transaction documents that are entirely generated by virtual machines.
The view that procurement professionals perform essentially rote tasks underlies the NPR’s Planet Money automation forecast for the job title “Procurement Clerk”:
NPR’s Planet Money provides readers with a perplexed 1960s-style robot as a mascot for their “definitive” guide to automation. Planet Money’s caveat: “The researchers admit that these estimates are rough and likely to be wrong.”
The easiest way to think about how cognitive procurement will change procurement’s day-to-day business operations is by looking at AI consumer trends. As consumers become comfortable with ordering electronics using Amazon’s Alexa, procurement buyers may similarly begin to delegating supplier communications to virtual assistants or chatbots. Following the personification trend of Siri, Alexa and Watson, Tradeshift’s chatbot Ada Lovelace offers an “instant response to any requests related to transactions, procurement, spend, payments etc.,” according to Tradeshift CEO Christian Lanng. Depending on the level of integration with inventory management systems, the decision to purchase or re-order a product could also become automated.
Cognitive systems will affect each segment of the strategic sourcing cycle differently. For example, Ada Lovelace’s “instant response” completes tasks within Purchase to Pay segment. Source to Contract, by contrast, is about using key insights from spend data to drive system-wide contract savings during a supplier negotiation.
Deloitte’s CPO Survey summarizes the high impact outlook of transformations in these segments succinctly, “Source to Contract is becoming predictive, Purchase to Pay is becoming automated, Supplier Management is becoming proactive, and these are all empowered by analytics and strong operational management.” This is the story you’ve probably heard, and in discussions of AI procurement the notion of rapid market transformation taking place in the next five years is already being treated as gospel.
Readers with a strong interest in understanding what job types are most likely to be automated in the coming decade may want to tune into our workforce automation interview with Martin Ford, or our recent Silicon Valley Job Automation Survey.
Procurement Functions That Resist Full Automation
The idea that AI might replace many procurement job functions entirely in the next five years is perhaps overly optimistic. Other experts believe that due to the limitations of legacy enterprise IT, the impact of cognitive systems on procurement could be less than transformative.
Here’s another view of what could happen in the next five years:
- Cognitive systems rely on bottlenecked human supply chain assistants
- Cognitive systems struggle to ingest inaccurate legacy spend data
- Cognitive systems remain hamstrung by proliferating data silos
- Cognitive systems provide an interface for humans to benchmark prices, solicit information from suppliers and access contract templates
In contrast with the bullish ROI envisioned by IBM and KPMG, one might consider the market position of a firm like Tamr. Founded by Turing Award recipient Michael Stonebraker of MIT CSAIL, Tamr is betting cognitive business processes will face massive hurdles due to legacy enterprise IT systems. Stonebraker and his investors believe that large firms, anxious to catch up in the analytics race, will turn to Tamr to cleanse and structure their data using a combination of machine learning and human expertise.
Stonebraker uses the term “data lake” to describe how executives have idealized the process of unifying their enterprise data within a single, integrated ecosystem. Unfortunately, if multi-source data isn’t verified and cleansed first, then any unification efforts amounts to what Stonebraker jokingly dubs a “data swamp”, unable to realize the promised ROI of analytics. Writing on the ACM blog, Stonebraker illustrates this problem with the following example:
Consider two very simplistic data sources containing data on employees. The first data source has record of the form:
Employee (name, salary, hobbies, age, city, state)
while the second contains data with a layout of:
Person (p-id, wages, address, birthday, year-born, likes)
An example record from the first data set might be:
(Sam Madden, $4000, {bike, dogs}, 36, Cambridge, Mass)
An example from the second data set might be:
(Samuel E. Madden, $5000, Newton Ma., October 4, 1985, bicycling)
A first reasonable step is to assemble these records into a single place for subsequent processing. … The two salaries of Sam Madden are different numbers. One or both may be incorrect. Alternately, both may be correct; for example one could be total wages including consulting while the other could represent the W-2 salary of an individual. Similarly, Sam can only have a single age. Hence, one (or both) data source have incorrect information. (My emphasis added).
Stonebraker’s conclusion is that “some data curation will require human involvement.” If data curation will continue to rely on the inevitable bottleneck of human expertise, then it’s hard to imagine cognitive procurement systems running as smoothly as they’re being advertised.
Another way of thinking about this is through the problem of “data silos”, when data remains only usable by a single department or business unit. Let’s say that in this case the data is accurate and verified, but it is structured in such a way that functional cross-referencing of datasets isn’t workable. Given the piecemeal approach of business units to digital transformation and the persistence of M&A as a strategy of corporate expansion, data silos are the rule, rather than the exception.
This is why, despite the vast excitement surrounding analytics, the 2016 Data & Analytics Report by MIT Sloan Management Review found that “analytics is now a mainstream idea, but not a mainstream practice.” Disputing the fashionable notion that all global business decision making is now “data-driven”, the Report’s authors observe: “Despite the hype, the reality is that many companies still struggle to figure out how to use analytics to take advantage of their data.”
This reality is reflected in the widespread agreement of CPOs on the leading barriers to digital transformation:
Image from The Deloitte Global CPO Survey, 2017
Therefore, implementing cognitive procurement solutions will remain greatly dependent on mature pipelines of spend data. Gathering and structuring that data from diverse sources is already a daunting task, and it remains to be seen whether or not cognitive systems will make that task any easier.
Given this reality, it’s hardly surprising to see a flourishing marketplace of solutions aimed at overcoming the many bottlenecks associated with spend data. Platforms that explicitly advertise these solutions as “artificial intelligence” include Orpheus, Zycus, Tungsten Network, Keelvar, Smart by GEP, Vroozi, IBM Watson and Coupa’s Spend360, to name but a few. But are all of these systems truly examples of “cognitive procurement”, and will these AI applications live up to the hype? Agreeing with the sentiments expressed by MIT Sloan Review, the authors of Deloitte’s 2017 CPO survey cautions against overly aggressive forecasts:
“Only a small number of teams are attempting to answer the question “what might happen?” by using data to drive decision making, deploying online analytical tools, and integrating data from ERP and some external tools to provide insight. The application of predictive and cognitive analytics is almost non-existent….”
Conclusion
Evaluating the ROI of cognitive systems for procurement depends greatly on an enterprise’s level of digital transformation. More than humans, cognitive systems insights will only be as good as the spend data that drives them. There is certainly a strong outlook for AI procurement solutions to deliver value from process efficiency (e.g. chatbots, virtual assistants, automating purchase orders and invoices). However, in order to unlock the greatest savings from cognitive systems, enterprise procurement must first tackle the problem of data silos and legacy IT infrastructure.
To realize the greatest opportunities in the evolving AI procurement marketplace, leaders must evaluate the following factors:
- Unified Data Availability: To what extent is enterprise spend data continuously available in a digital form? Is there a cost associated with retrieving spend data from IT infrastructure on a regular basis? What is the level of completeness and granularity associated with this data? How far away is it from ground truth? If complete and accurate data piped into a cognitive procurement system yields improved decision making capability, then it will be important to understand how incomplete and inaccurate data may produce unintended consequences.
- Analytic Integration: To what extent is spend data already integrated into enterprise decision making? Do protocols exist for data-driven decision making, e.g. how to balance insights from spend data against stakeholder preferences or local conditions? What is an enterprise’s confidence that it is measuring the correct key performance indicators? If cognitive procurement begins guiding controversial management decisions, then it will be important to understand exactly how and to what extent spend data should inform executive decision making.
- Supply Network Harmonization: To what extent do suppliers and partners have the ability and willingness to provide or interface with cognitive procurement, instead of human employees? If there are infrastructural or cultural barriers that make automated communication or data transfers difficult, then it will be important to understand what the risks and opportunities that exist along the way to transforming an enterprise’s digital supply network
Image credit: Dynamic Web USA