AI applications for automating processes in clinical trials are among the most prominent AI applications for the pharmaceutical industry. AI vendors are currently offering software that allows pharmaceutical companies to leverage their scientists’ notes for data science projects regarding their future trials. Additionally, there are some applications which help companies segment their customers into easily navigable groups when finding patients for clinical trials.
In this article, we cover the most prominent AI software vendors are developing for pharmaceutical companies to help them plan, conduct, and review clinical trials. We make note of companies with a high likelihood of actually using AI in their software and discuss how pharmaceutical data may need to be prepared for the most effective implementation. The applications we cover are as follows:
- Text Mining for Clinical Trial Planning and Design: Applications for finding past trial information to inform current trial design.
- Matching Patients to Clinical Trials: Natural language processing (NLP) applications for extracting patient data and matching that patient to a clinical trial.
- Clinical Trial Design and Optimization: Applications for designing clinical trials, such as predictive analytics for genetic clustering.
We’ll begin with exploring the clinical trials applications of text mining:
Text Mining for Clinical Trial Planning and Design
Pharmaceutical research teams frequently review past clinical trial data for insights on how they might improve their clinical trial design in the future. AI software could help with this by aggregating all of that data and optimizing it for keyword search. Additionally, an AI application for clinical trial design could create visualizations of trends found throughout that clinical trial data.
Natural language processing (NLP) software is particularly helpful with clinical trial data in that the technology might be able to recognize words and phrases within clinical notes. This would allow research teams to locate clinical information that is more relevant to their current projects than it was when first discovered. This information could be digital lab notes, statistics, or dosage information from a pharmaceutical company’s database of clinical trial data.
This type of solution has the potential to save time when deciding which drugs to test and which experiments to conduct. A data scientist using an NLP solution to find notes about every chemical reaction associated with a given drug may realize they do not need to conduct the experiment.
This could be because they found the important information in the past and could use it to inform further experiments. Alternatively, this kind of discovery may prompt the company to move forward with a clinical trial for the given drug if there are no remaining concerns.
Lab notes and clinical trial data are typically saved in a specific database for keeping track of a pharmaceutical company’s experiments with certain drugs, molecules, and chemicals. These are written by the company’s scientists while they are conducting experiments. They also usually include healthcare and pharmaceutical jargon and some colloquial language.
In order to make sure an NLP software solution could recognize these types of words and information, the software developer would have to label each set of lab notes. Then, they use that labeled data to train the machine learning model behind the software to recognize individual fields on each type of document.
NLP software could also be trained on electronic medical records (EMRs) in addition to clinical trial reports to find information about a patient’s response to a drug. This type of software could identify notes about the patient’s experience and mark any relevant chemicals that may have played a role in that response. Leveraging EMR data along with clinical trial data can help pharmaceutical companies recognize any adverse effects their drugs may have.
That said, pharmaceutical companies typically do not have access to records from hospitals or healthcare providers outside of any partnerships they may have. This type of collaboration may help pharmaceutical companies access a hospital’s data and label it to use for training their machine learning model. This allows for more extensive training on more medical jargon and important topics such as disease and adverse effects of drugs.
We spoke to Amir Saffari, Senior Vice President of AI at BenevolentAI, about the future of AI and drug discovery. We asked how his company takes in all the published scientific data related to their drug discovery research. Saffari highlighted his company’s process of logging that data into their database before the entire research team has finished reading it:
One area our company focuses on is to machine read all the literature, patents, and documents. There is an enormous amount of research that gets published every day…So we machine read all available literature and pool it together in a database of facts that can be extracted from this literature. That forms the basis of the hypothesis that we generate to find therapeutic targets for diseases.
Here, Saffari shows the use of running all of this information through their machine learning platform. The amount of new clinical data published each day is too large for his company to keep up with, but their software can purportedly organize this data so it may still be leveraged despite that inconvenience.
When his company is choosing the best patients to test new therapy methods on, they combine the knowledge of the team with their large store of aggregated data. Text mining is one capability of NLP software that can be especially helpful in planning clinical trials. Text mining is the process of running a database through an NLP software to search for words, phrases, and general topics within that database.
The NLP software would then find all data related to the user’s desired topic and list the points as search results or through a user dashboard. Researchers may use text mining software to find evidence of chemical imbalances or dosage amounts that may have contributed to the results of a clinical trial. This could include the frequency of each dose and pre-existing chemical imbalances in the patients.
Linguamatics is one example of an NLP software developer that offers text mining solutions to pharmaceutical companies. They have a significant number of AI staff on their team that also has a background in AI. They document their clients’ success in case studies showing how their software is used and the benefits that purportedly come with it.
The company claims their text miner I2E helps pharmaceutical companies organize and search through research data on past chemical compounds and experiments. This would allow clients to access information about similar projects to gain a better understanding of how a chemical may react before they test it with another one.
Below is a short introductory video on text mining from Linguamatics. The following is a list of steps in the demonstration:
- 1:00: Effectively using search engines and keywords with text mining
- 3:23: Interpreting the meaning of the text found
- 5:10: Extract data relevant to the keywords used
- 5:33: Comparing these approaches
According to one of Linguamatics’ case studies, their I2E software helped AstraZeneca interpret data from studies and clinical trials to “find high-value information.” This refers to the two examples detailed in the case study.
The first involves identifying the status of clinical trials as either “blind” or “open label” when the dosage between patients differs. This means that some trials obfuscated which patient received which drug or dosage level, and others did not.
The second involves the duration of continued dosages during follow on clinical trials, or trials that extend past the original experiment window and focus on a few specific patients. Finding this information may be helpful in predicting the effects of prolonged exposure to the drug in question.
The case study states AstraZeneca researchers were able to search specific queries with as many as three variables at a time. It was purportedly essential to AstraZeneca’s ability to search through all of their clinical data for the most valuable information from it. However, it is important to note that the case study did not offer any numerical statistics pointing to AstraZeneca’s ROI regarding I2E.
Matching Participants to Clinical Trials
Business leaders in pharmaceuticals could benefit from AI software to help them match patients to a clinical trial based on physician’s notes and past trials. This type of automation has the potential to significantly reduce friction in getting a drug to market.
NLP software is currently the most commonly found solution for this problem because the technology can determine the best-suited patient for the trial based on medical history and patient files. Finding the right patients comes with its own set of challenges, but NLP software could improve on how companies handle them.
The following four challenges reflect the types of problems these applications may be able to solve:
- Keeping the personal information of the patients safe while handling their medical histories
- Recognizing International Classification of Diseases (ICD-10) to determine which ailments are discussed in a given document
- Identifying patient experiences with adverse effects of drugs they have tried in the past
- Utilizing unstructured data to find insights about the correct patients
Some patients’ personal information may be protected by law or a prior agreement and thus cannot be disclosed in a way that would link that information back to the individual. Few vendors offer solutions with this as part of their value propositions, but some claim to be able to obfuscate that information.
This would allow pharmaceutical companies to hide any identifying information while still using their clinical and medical information. This may be possible with visualizations such as graphs that do not detail individual patients but create relevant statistics.
ICD-10 codes are among the most important details when determining how viable a patient is for a clinical trial. Each standardized code denotes a single possible disease, illness, or injury a patient may have or have had previously.
A machine learning model for trial matching would need to be trained to recognize the ICD-10 codes associated with each patient and determine how related they are to the drug being tested. This might include how the injury or ailment was sustained, such as an injury from operating heavy machinery or lung disease from prolonged exposure.
An important factor in making sure the right patients are chosen for a clinical trial is their history with adverse effects of drugs. A patient may be less likely to respond well to the drug being tested if their medical history indicates an unwanted side effect from drugs with similar ingredients. Other indicators of a low chance of success are adverse effects of drugs that were made to treat the same illness as the drug being tested.
In order to extract information from this type of file format, the machine learning model behind the software would need training on pharmaceutical data from those documents. Software developers would need to label each individual field in each type of document. Then they would run tens of thousands of clinical trial reports through the model along with every ICD-10 code.
This would gradually train the machine learning model on the ability to detect which fields hold which information types. This includes the classification for any ailments the document may refer to.
Deep6 AI is one company that claims to be able to hide sensitive patient data while matching them to clinical trials. They offer NLP software that purportedly detects individual traits associated with patients including symptoms, past diagnoses, genomics, or test results.
The image below is a graphic from the Deep6 AI website which lists the proposed benefits of their solutions:
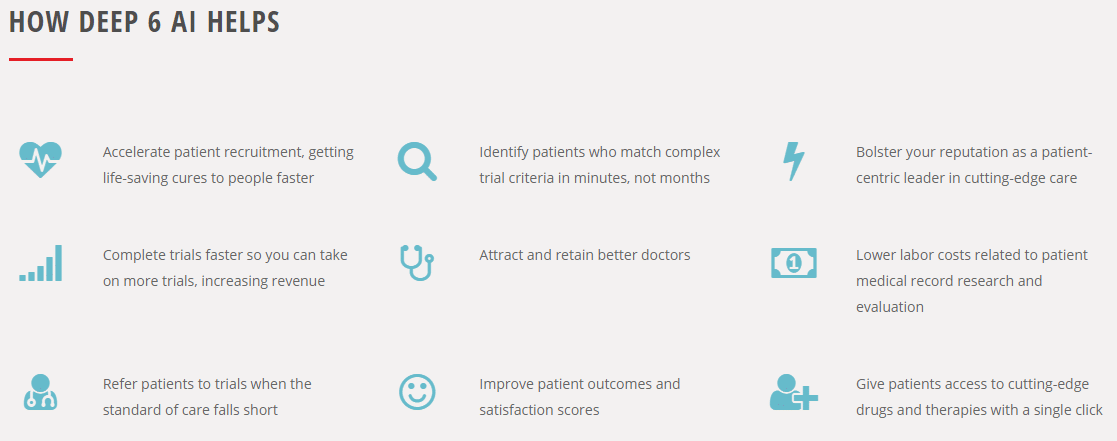
They also claim their software helps their clients find more viable patients for each clinical trial than they normally would. This is evidenced by their case study covering their project with Cedars-Sinai Institute. The case study states they helped Cedars-Sinai Heart Institute improve their clinical trial matching process for a drug called Udenafil. The drug is made for patients with a rare birth defect of the heart, and Cedars-Sinai was having trouble finding more than two patients for the study.
Cedars-Sinai purportedly used the Deep6 AI cohort builder tool. This is a type of application for organizing and identifying patient profiles from a database. The case study states the client company was able to validate 16 of the 19 newly found patients as eligible for the Udenafil trial. Deep6 AI claims the process took less than an hour. However, it is unclear if that is indicative of most customers’ experience.
Clinical Trial Design and Optimization
Another use case for AI in clinical trials is in the design and optimization of practices, procedures, testing methods, and the variables tested in each trial. AI solutions may be able to analyze patient profiles and medical histories to determine which patients will have the best response to the drug being tested. This might save pharmaceutical companies some time when trying to match patients to a trial and augment the trial to allow for more viable patients.
Predictive analytics applications could accomplish this with genetic clustering. This is the process by which an AI software segments a customer base into smaller groups that reflect each patient’s chance of responding well to the drug.
Pharmaceutical companies can also incorporate the medical histories of their trial participants into the genetic clusters. This is because there are also solutions that let companies detect possible adverse effects of certain drugs before they occur during trial.
For example, a patient could have a history with a drug that is known to have a volatile reaction with the one being tested. Similarly, another patient could have an allergy that increases their chances of responding negatively to the drug.
Predictive analytics solutions could also be used for identifying and establishing the best practices for clinical trials. This is based on the steps taken during past trials as they are represented in the company’s database of past trial data. These documents include information about clinical trial operations, procedures, and whether the company considers the trial a success.
Another AI application could then run through all of this data to find instances of similar trials or situations within a trial with varying levels of success. This allows business leaders to compare their new plans for a trial with the operations of one that may not have been successful for the same type of drug.
A business leader might also discover a new approach to clinical trials they may want to adopt. Some predictive analytics solutions will identify similarities between past and current trials for the user. Others may allow users to create a predictive model that could illustrate them clearly.
Perhaps most important to clinical trials is finding the trust indicators that show the drug will fulfill market demand for the treatment in development. Pharmaceutical companies may want to use data analytics software to gauge market demand and how likely doctors are to prescribe their drug. This can be done with EMR data, physician’s notes data, and sales data.
The following is a demonstrational video from AI vendor Dataiku. It demonstrates how their DSS platform could help data scientists predict doctors’ prescriptions. We have found the most important sections of this process and list them here:
- 0:00 – Finding the necessary datasets for the prediction they want to create.
- At 2:30 – The goal of this analytics experiment and combining data to find any contradictions or incongruencies.
- At 6:30 – How the user combines datasets and “cleans” any incongruencies between them. This exercise requires the user to make sure all of the physician ID numbers appear accurately or find out why certain ones may be missing.
- 8:25 – The user populates all relevant data into a single table. Here they can find rows that can be combined into a single one for less granular categorization.
- At 9:58 – Taking all the cleaned and organized data to utilize it for generating a predictive model.
As explained in the video, it is important that pharmaceutical companies understand how to prepare and clean their data for machine learning models. In order for an AI application to work as intended, the machine learning model behind it must be trained on data that is properly labeled, edited to have less redundancy or inconsistency, and organized by the appropriate groupings for the project.
We spoke to Madhusudan Shekar, Principal Evangelist of Amazon Internet Services, about determining the best AI application areas for a business. When we asked him about how he emphasizes the importance of data cleaning and organization to his clients, Shekar explained,
When a business faces challenges in data organization, we start looking at the data lake to determine what is clean and unclean data. Once the data is cleansed, the business now has the ability to process it. Put the data in a single space and define schema as you start building and reporting from that.
Machine learning, for all the coolness it is known for, first is about data. Eighty percent of the work in machine learning is getting the data organized, structured, trustworthy. Because the machine is now going to make decisions for you.
Shekar is direct in his statement that most of the work to get an AI application running comes in preparing, cleaning, and leveraging the data to train the machine learning model. This is because once the training is done, the AI application can work on its own and will not need constant input from an employee.
Our research did not yield any results when we searched for case studies showing a pharmaceutical company’s success with any kind of data analytics software for clinical trials. Some may see this as a sign the use case is relatively nascent compared to the others described in this article. However, we are confident that the companies offering solutions like this are somewhat likely to be truly using AI.
Header Image Credit: Marketing China