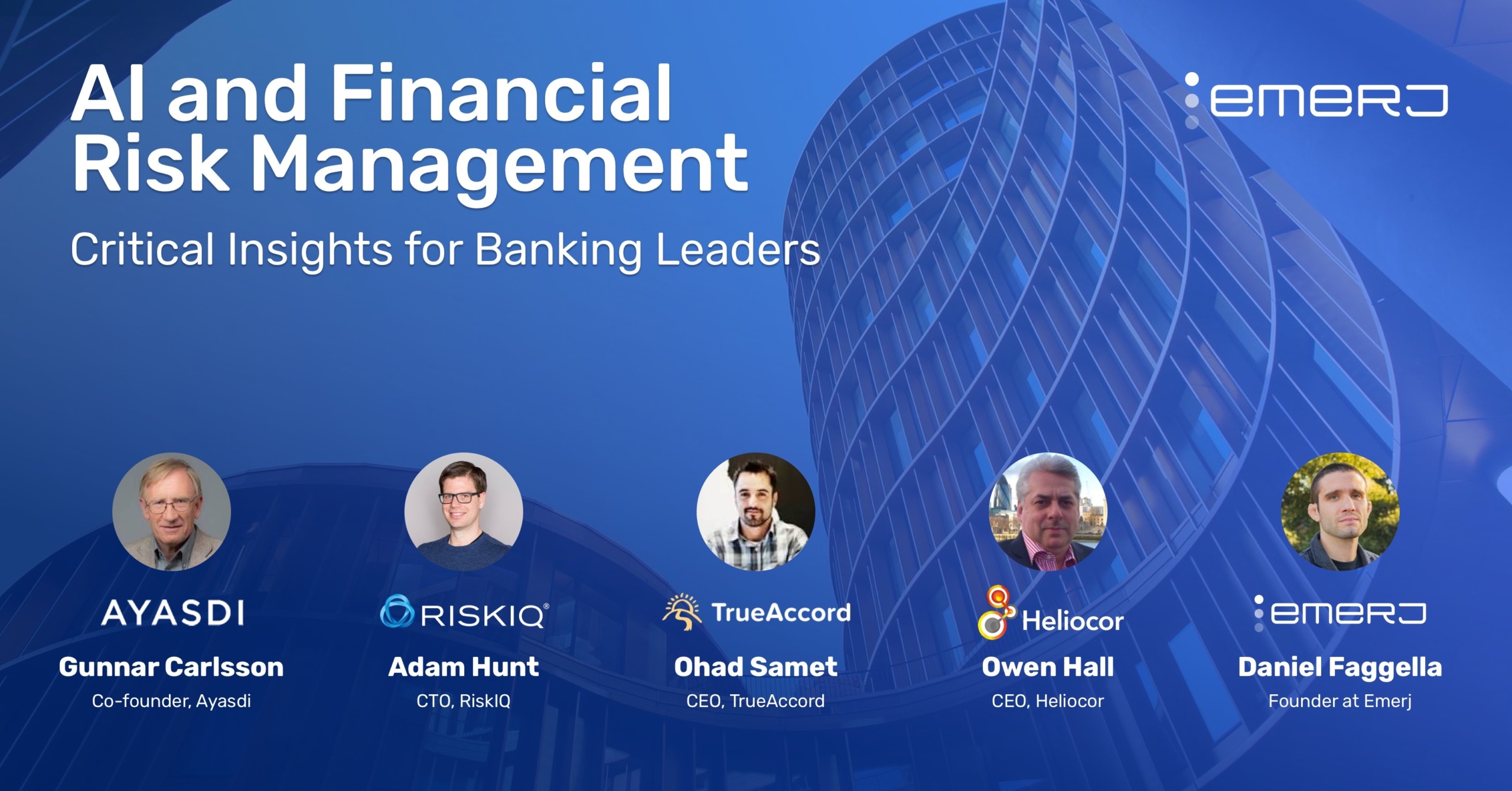
Our research indicates that AI applications for risk-related banking functions are more numerous than applications for other business areas. Fraud and Cybersecurity, Compliance, Loans and Lending, and Risk Management collectively made up 56% of the AI vendor products in the banking industry, as shown in the graph below:
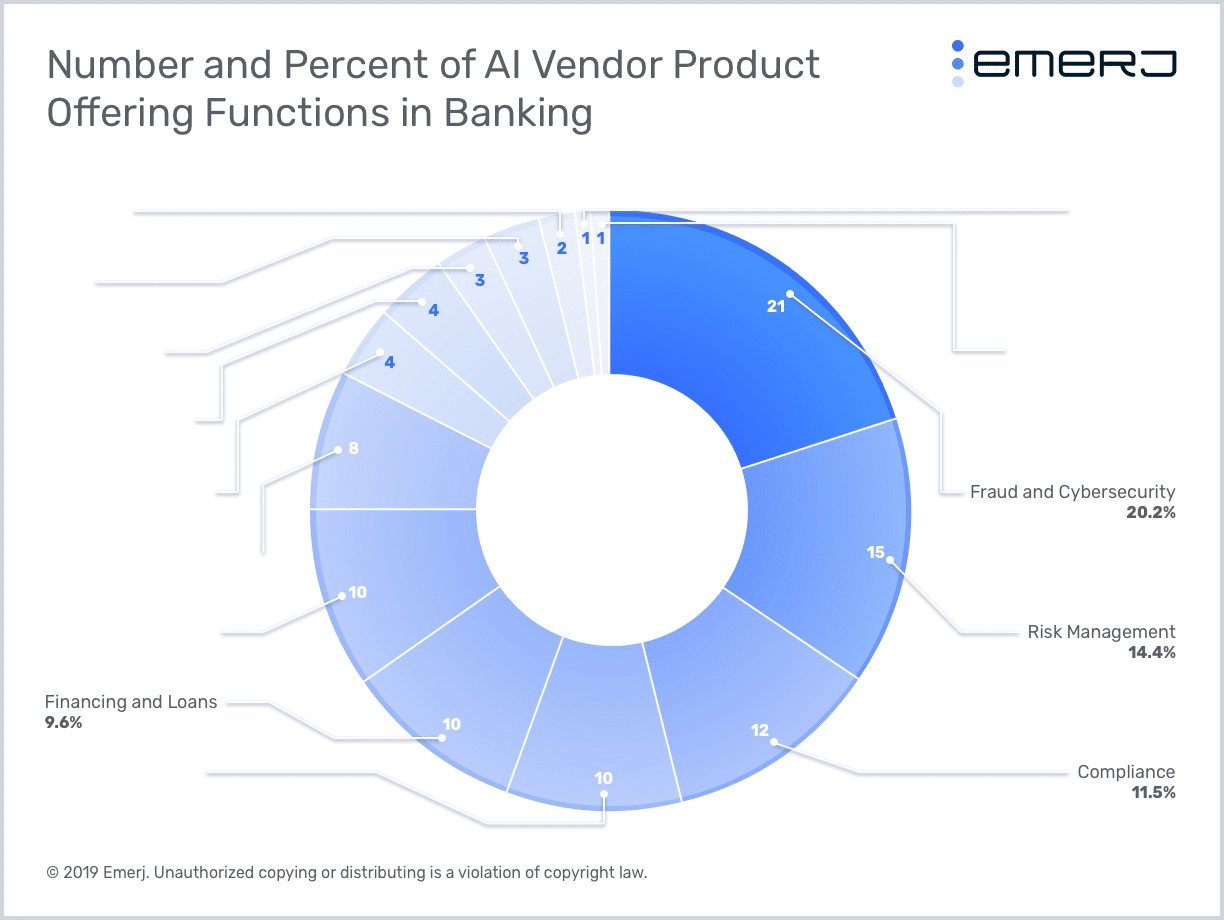
This indicates a clear fit for AI in the risk-related functions in finance, and as such, we focused this month’s AI in Banking podcast theme on risk.
We spoke with four experts on the state of AI in banking and finance and how banks can use artificial intelligence to mitigate various types of risks, including fraud risks, compliance risks, and cybersecurity risks.
In this article, we break down the key insights from the conversations we had with these four experts on our AI in Banking Podcast, including discussions about:
- Why Machine learning Is A Good Fit For Financial Risk Management
- The Weaknesses of Rules-Based Risk Management Systems
- How AI Algorithms Can Upgrade Existing Statistical Processes in Finance
- Financial Risk as an Early Area of AI Traction in Banks
We’ll also provide context around these points with data from our AI in Banking Vendor Scorecard and Capability Map report. Interested readers can download the executive brief.
Special thanks to our four interviewees, whose interviews are embedded throughout the article:
- Gunnar Carlsson, Co-Founder at Ayasdi
- Adam Hunt, CTO at RiskIQ
- Ohad Samet, Co-Founder and CEO at TrueAccord
- Owen Hall, CEO at Heliocor
You can listen to our full playlist of episodes in our “AI and Financial Risk Management ” playlist from the AI in Banking podcast. This article is based in large part on all five of these episodes:
Subscribe to the AI in Banking Podcast wherever you get your podcasts:
We start our analysis of AI for financial risk management with why machine learning is such a good fit for processes such as fraud detection and cybersecurity.
Why Machine learning Is A Good Fit For Financial Risk Management
Risk-related banking functions, particularly fraud and cybersecurity, in large part involve discovering unusual behavior as it appears. Fraud and cybersecurity analysts monitor digital systems for activity that is outside of the norm and then flag this activity for further review.
Traditionally, fraud and cybersecurity analysts have used rules-based systems for determining whether or not to flag activity as suspicious or not. We discuss the weaknesses of these systems in further detail later in this article, but artificial intelligence is simply better-suited for detecting deviations in normalcy.
In particular, anomaly detection is one AI approach that, as its name implies, automatically flags activity that breaks patterns of normal.
Businesses have been using anomaly detection applications for their fraud and cybersecurity processes for the better part of the last decade; this stands in contrast to the relative infancy of other AI applications.
AI vendors selling anomaly detection products into banking have raised just as much money as AI vendors selling natural language processing (NLP) products ($723 million), but there are half as many anomaly detection vendors than there are NLP vendors.
In addition, Anomaly Detection as a category scored highest among the top three AI Approaches (Anomaly Detection, NLP, Predictive Analytics) on its Average Evidence of ROI Score (2.1 out of 4.0).
All of this points to anomaly detection’s maturity as a technology and as an asset within key banking processes. Banks are stating more results with anomaly detection than any other AI approach, and venture capitalists are eager to invest in vendors that use it.
Businesses can install anomaly detection-based fraud detection or cybersecurity software into their digital ecosystems so that it can start “learning” what constitutes normal activity in a business.
For example, an anomaly detection system might “learn” that typical, legitimate transactions are in between two particular dollar amounts, come from certain georegions, and are on particular types of credit cards.
Although these criteria may sound workable with traditional rules-based systems, such systems are only as nuanced as what the people that create the rules for them can come up with.
Fraud analysts are only going to be able to create rules for fraud detection based on instances of fraud in the past. As a result, rules-based systems are not good at detecting new methods of fraud as they happen, and fraudulent transactions using these methods can easily enter the system undetected.
Hall seems to reference this in part when he discusses how fraudsters can use people’s digital footprints to defraud them, mentioning AI as one possible way to combat this:
As people go through their daily lives, they leave more and more ‘breadcrumbs’ about themselves and those become open for people to use in the areas of defrauding people of their money. You’ve…got a whole area in terms of the banks looking at ‘how do I really know that the person who sat online doing a transaction is the person I really think they are. I think AI will come into there.
When an anomaly detection software “learns” a digital ecosystem’s state of normalcy, it can flag anything that deviates from that normalcy even if that deviation isn’t immediately obvious to fraud analysts.
It might be that a transaction wouldn’t be suspicious except for the fact that it came in during a particular time of day or that it’s combination of georegion, credit card number, credit card brand, and dollar amount is so outside the norm that it warrants review.
As such, anomaly detection is a natural fit for fraud and cybersecurity use-cases in banking. To further illustrate this point, we’ll run through two hypothetical use-cases for AI in banking: wealth management and payment fraud detection.
Wealth Management
Increasing customer lifetime value is a key objective for wealth managers. In theory, machine learning software could be used to determine what a wealth manager should send to a customer and at what time to maximize the customer’s lifetime value (LTV).
The trouble is the factors that go into getting a customer to trade, buy, or sell are not easily measured in a digital space.
For example, a customer might make the decision to invest in a particular company after speaking with a wealth manager about a personal experience they had. This isn’t quantifiable, but it played a major role in getting the customer to invest.
Machine learning doesn’t fit so naturally into this particular use-case, and this is the case for many applications in the customer-facing functions, including wealth management and customer service.
In addition, it’s difficult to measure the ROI of such an application. Even if all of the data that a wealth manager uses to determine when to send to a customer could be digitized and fed into a machine learning algorithm, the firm wouldn’t have an idea of the customer’s LTV for years, sometimes decades.
As such, the firm wouldn’t know if the AI software was any better than traditional methods for a very long time; it could turn out that it didn’t help at all.
Payment Fraud
In contrast, as we’ve discussed, anomaly detection fits in well with existing fraud detection processes. When a payment comes into the system, anomaly detection software can determine whether or not the payment is fraud based on data that is readily available as the payment comes in: the payment’s amount, its location, its card type, its date.
The software can get more granular than any rules-based system.
As a result, the ROI on anomaly detection software for fraud detection can be almost immediately positive. Fraud teams can reduce false positives and false negatives, decreasing the number of payments that enter the system undetected, and they can do this as soon as the software is properly trained. The ROI can be measured in cost reduction and increases in productivity.
Fraud analysts spend far less time and effort reviewing false positives and are also able to identify cases of fraud that may have been overlooked using traditional fraud detection systems.
The Weaknesses of Rules-Based Risk Management Systems
Most traditional fraud detection systems are rules-based systems. These systems are good at identifying and stopping known threats that security analysts have seen before. For example, if a customer whose transaction history have always been around a particular date and at a particular location is suddenly found to have made a significantly higher transaction in different locations, the system can create a red flag.
Typically fraud and cybersecurity analysts at financial firms spend a lot of time designing rules-based threat detection systems. These systems need to include every rule that the analysts can think of in terms of denying security access to attackers.
But even when analysts design a system that prevents a majority of security attacks, these rules need to be constantly updated.
This is because once attackers find a loophole in a rules-based system, they can exploit that opening until the breach is discovered and the rule is updated. In addition, fraudsters are beginning to use AI themselves, making AI adoption all the more necessary for fraud departments at banks in the coming years.
Hunt stated:
Where AI systems can really help is in allowing security analysts to design rules that are more dynamic and far broader. This makes it such that attackers need to put in a lot more effort to find a workaround for these rules.
In general, traditional security rules are predefined rules for which cases might be classified as “benign” or “suspicious.” These rules cannot account for all threats, especially new threats that haven’t been encountered before.
Banks usually employ these traditional tools to introduce some level of automation into fraud analyst workflows, enabling them to do more. However, they can sometimes prove to be inefficient if they flag too many false positives.
The Advantage of AI
AI software can automatically categorize a list of threat incidents on the basis of which threats are more immediate. Prioritizing these threats effectively allows human security analysts to focus only on the most important cases.
That said, AI systems are a lot more difficult to deploy and require different skill sets to maintain than regular IT. When implemented correctly, however, they can ensure more dynamic threat responses, adapting to new cybersecurity threats in real-time.
As a result, they can flag transactions as fraudulent even when they employ new methods of fraud unknown to fraud analysts.
How AI Algorithms Can Upgrade Existing Statistical Processes in Finance
There are numerous areas of finance in which statistical methods are already used to determine best practices. We’ll discuss two in particular: loans and lending and debt collection. Artificial intelligence could serve as an upgrade to these statistical models, allowing for more nuance and granularity in ways that can drive revenue.
Loans and Lending
The linear regression-based credit score revolutionized lending processes at banks. They aim to be objective and accurate representations of a loan applicant’s creditworthiness, solving bias problems that came with allowing underwriters to be the sole determinant for approving a loan.
Although algorithm bias is a concern with machine learning due to the “black box” problem, banks and other lenders are finding success with AI for loan and lending processes.
Traditional credit scores may factor in some two dozen variables into their calculations, but some AI-based underwriting software can take hundreds, even thousands of variables into consideration. This is especially helpful for applicants that lack a robust credit history, a segment of the population that banks are less eager to approve for loans because of the uncertainty that comes with them.
While some AI vendors offer software for credit underwriting that factor in variables such as an applicant’s digital footprint (social media data, browsing data), others use data such as the make and model of the car for which the applicant needs a loan, for example.
Feeding data like this into a machine learning algorithm could generate a more nuanced credit score that gives a more accurate picture of whether or not an applicant is likely to pay back their loan regardless of their previous credit history.
As a result, banks can approve more applicants while taking on less risk. An AI algorithm might reveal that an applicant a bank might otherwise have rejected because they lacked a credit history or had a blemish on their record is in fact likely to pay back their loan, and the bank could approve them, directly increasing revenue.
At the same time, a bank can reject applicants they would have taken on had they used traditional credit modeling to vet them, thus reducing risk.
Debt Collection
Debt collection is another area where AI might upgrade existing statistical models. Traditionally debt collectors use phone calls, letters, emails and send notices to the credit bureaus alerting them of customer debts.
The existing models at debt collection departments seek to determine when debt collectors should reach out to customers to maximize the chance that they can get the customer to pay their debt.
Samet discusses how machine learning upgrades to existing debt collection processes might allow for more granularity in making the most of these efforts.
He gives the example of debt collection operations in credit lending, explaining how AI software might add value to existing workflows by improving customer experiences. He says:
There is a lot of thinking from financial firms about how to acquire customers, and AI vendors are offering finance firms a solution in this context where the better the machine learning algorithms get, the better the system’s prediction of what might be the right time, the right data through the right channel with the right content and with the right payment offer to get them to pay for their debt.
This may prove useful for driving revenue for banks. There seems to be some evidence that contacting customers in the way that they prefer significantly improves debt collection numbers.
Financial Risk as an Early Area of AI Traction in Banks
Experts we’ve spoken to have pointed to risk-related banking functions as areas where AI is providing value by way of cost reduction. Carlson echos this when he says:
From supply chain to the internal guts of the bank—AI can theoretically be applied to clean these processes to be more seamless and optimize those processes in real time, driving down cost and improving output…which means customers get served faster.
Although AI-use in banking is still nascent on the whole, financial crimes and AML are where some banks are currently deploying AI solutions. This is because trying to assess customer behavior based on transaction histories and other customer specific data is hard.
In many cases, this data is very complex and there are lots of fraud investigators at banks working on this, making it a highly labor intensive task.
Banks won’t likely focus on building robust customer-facing applications before they’ve automated key processes that don’t drive them any revenue, namely compliance.
Although many banks claim to have their own chatbots, these chatbots are unsophisticated.
Banks likely only built them in response to their competitors’ press releases. This is evidenced by a key discrepancy: 38% of the AI products that banks discuss in press releases are conversational interfaces, much more than any other AI capability.
At the same time, AI vendors offering conversational interfaces have raised only 5.5% of the total funds raised by AI vendors in banking. One would expect chatbot vendors to raise a greater share of venture capital if banks were actually focusing on chatbots at this time.
The money is in the risk-related functions. AI vendors offering products for AI’s Risk Management capability raised 29% of the total venture capital funding for AI vendors in banking, far more than any other Capability category.
This is perhaps unsurprising; banks have been investing in automation efforts in areas such as compliance and fraud for many years now. They are eager to automate these processes because they don’t drive any additional revenue.
As such, banks have IT departments dedicated solely to fraud and solely to compliance. If they want to invest resources at these departments into building AI, they can do so much more easily than in areas where dedicated automation efforts don’t exist.
In addition, the leadership teams in these departments are used to thinking about automation and streamlining processes with technology.
This will make the transition into AI easier, even if none of these leaders are data scientists or machine learning engineers. Their frames of mind are at the very least ahead of their peers in other departments.
All of this, in tandem with what banking experts are saying about where banks are currently investing in AI, leads us to believe that risk-related functions are most likely to be automated in the next five years.
Related Articles
AI-Based Fraud Detection in Banking – Current Applications and Trends: An analysis of predictive analytics- and anomaly detection-based fraud detection software for the banking industry, including specific use-cases for both.
AI for Compliance in Banking – Where Banks Should Focus Next: A discussion on machine learning use-cases for compliance processes in banking and finance and AI adoption best-practices, including insights on why banks are eager to automate compliance processes with AI.
Artificial Intelligence For Risk Monitoring in Banking: An exploration of how AI is making an impact on broader risk monitoring processes in banking.
Artificial Intelligence in Cybersecurity – Current Use-Cases and Capabilities: A breakdown of some of the most common use-cases for AI in cybersecurity broadly, including a discussion on how anomaly detection works.