Machine vision has numerous use cases within the healthcare industry, including clinical solutions such as medical imaging and medical diagnostics. There are also possibilities in white collar automation such as medical transcription, which was one of the main interview topics in our white collar automation interview with the Executive Chairman of CognitiveScale.
That said, there is a less common application for machine vision in optical character recognition (OCR), which can be used to digitize physical documents and transfer their data into digital storage such as Electronic Health Records.
In this article, we identify what is possible for machine vision and OCR software in healthcare document digitization. We highlight exactly which types of data can be digitized and what this could mean for healthcare networks and hospitals.
We explain how extracting each type of data from healthcare documents could be useful. We then discuss how accessible this may be to leaders in healthcare and how necessary it may be, and give an example of a vendor showing success with their software.
We then discuss how accessible OCR software might be to healthcare networks and hospitals in terms of adoption. Additionally, we discuss if adopting machine vision/OCR technology is even worthwhile for healthcare networks. Finally, we present an example of a document digitization vendor and provide a case study showing success with the vendor’s software.
The space for AI applications in healthcare document digitization is relatively nascent compared to that of other applications, such as medical diagnostics and medical transcription. We have included a section about why this might be at the end of this article to offer context into why there is comparatively less innovation in this area.
In this article, we’ll discuss:
- AI-based document digitization in healthcare – what’s possible, what’s successful, and what’s needed to make it work
- Why healthcare OCR is relatively underdeveloped
We begin our overview of the state of AI document digitization in healthcare by discussing the possibilities of the technology up front. We then look deeper into what data is necessary for healthcare companies to see success and how that success manifests in examples.
AI-based Document Digitization in Healthcare – What’s Possible
The most common artificial intelligence technology for document digitization is a type of machine vision software called optical character recognition (OCR). This software is made to analyze images of paper documents from an attached camera and recognize written characters and letters within them. It then records the recognized characters in the same sequence as they appear on the scanned image which can then be saved in a digital format.
This process effectively digitizes the information from the scanned document and allows the user to save it to a database for later use. This is particularly useful for companies with large amounts of data in physical forms that they wish to leverage for insights into possible business improvements. In the healthcare industry, OCR technology could help digitize document types including but not limited to:
- Clinical trial documents
- Patient reports containing clinical data or medical records
- Prescription slips or receipts that may be used to verify a patient received medication
- Lab notebooks from clinical trials or other experiments
To some, the idea of taking written information and automatically converting it to a digital format to be used for enterprise business intelligence would be a novel capability. However, to those in America the technology likely seems commonplace. Some industries like healthcare may have already been honing their process of digitizing documents since before the use case was considered for AI and OCR.
We spoke to Zhigang Chen, Director of the Healthcare Big Data Lab at Tencent, about how companies may respond to the challenges for AI in healthcare especially concerning data acquisition. When asked about the advantages in healthcare data the U.S. may have over other countries, Chen said,
So I think education, talent-wise, I think the US has a very strong advantage. The other is digitalization. It basically happened way earlier in various industries in the States. Also, I think the competition actually drives a lot of changes in the industry. For example, in the healthcare industry in the States, [digitization] had been around for many years, but that’s not the case in many other countries in the world. So I guess the digitalization put the US in a more advantageous position in the world.
We can infer from this quote that document digitization likely is not as widely considered to be a viable use case for machine vision in China. However American companies are now used to scanning data from printed documents and transcribing the data into word processors by hand.
These companies may want to stay updated with the latest in digitization technology. Some companies may want this even when it is not fully necessary for their business needs.
We caution readers not to adopt AI “toys,” or applications that are worked into their enterprise just because they use AI. Document digitization technology would only be necessary if the volume of documents to be digitized prohibits manual transcription.
Employing one of these applications requires an amount of research and development the typical healthcare company is not used to doing outside of the clinical healthcare space. They rely on that technology to work in order to accomplish their own research and development.
Physical documents from past clinical trials could be a good resource for healthcare companies to compare their current clinical trial operations in order to improve their best practices. For example, a hospital looking to gather data on the general health of their patient population may want to digitize documents from clinical trials conducted there in the past.
Patient reports, likely from outside clinics or physicians, may also contain helpful information about likely side effects of a drug and how individual patients may respond. It is particularly important to incorporate all relevant patient information when new side effects are discovered or if a patient has had allergic reactions to it in the past.
Healthcare companies can benefit from this information by having access to every past reaction to a given drug and using that awareness to improve patient care.
All procedures and results from pharmacology experiments and other healthcare fields of study are recorded in lab notebooks, which usually contain notes from multiple weeks of work. These notes would then be digitized and saved to a database as new results are found.
These notes are digitized so that new information can be added to the company’s corpus of healthcare data associated with the current experiments or their goals.
An example of this would be saving this OCR data along with any video recordings of the corresponding experiment to organize all findings. A company may choose to film an experiment to document any reactions or results they find and be stored within the dataset for that experiment.
One vendor that has found success in offering machine vision OCR software for healthcare is Apixio. The company’s software platform is made for capturing data from detailed documents with numerous specific fields.
This includes the previously mentioned document types along with patient records with International Classification of Diseases (ICD) codes.
Below is a graphic from Apixio that explains the four sections of its software solutions platform. It begins with data acquisition, where the OCR takes place and client documents are digitized. Then, Apixio can purportedly process that data and format it to be readable by a machine learning model.
The company states their software analyzes this data and creates individual care summaries for each patient. Then, those summaries are leveraged to create predictive models that can gauge patient health risk:
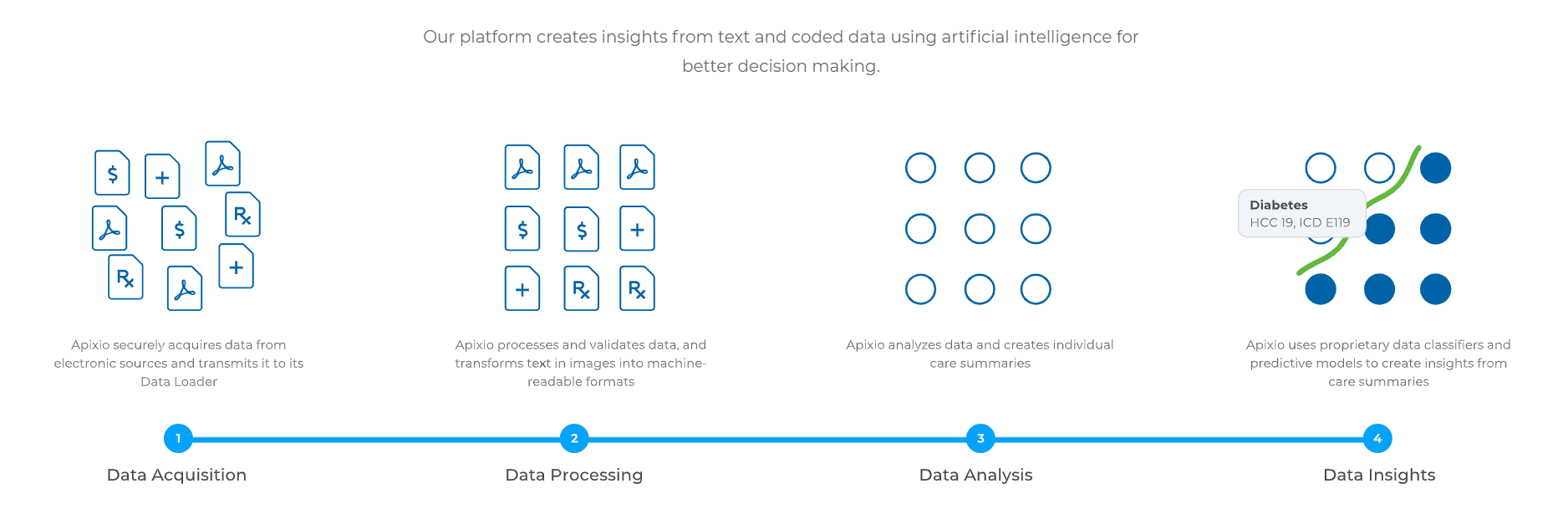
The company claims in a case study to have assisted Magna Health Plan convert their medicare advantage (MA) charts into a digital format. The Health Plan wanted to improve their regulatory compliance by moving away from relying on vendors to process their risk adjustment results accurately.
In order to be in compliance with healthcare coding (HCC) regulations, a company must have patient conditions marked with the corresponding ICD code.
This coding would also affect a health plan’s risk adjustment results, as personnel validating risk adjustments need to have the right code to officially discern the nature of the patient’s ailment.
According to the case study, Magna Health Plan was able to identify 584 HCC deletes, or instances where the HCC on a chart had been deleted, that would have impacted their risk adjustment scores. This likely resulted in more accuracy and transparency when reporting on their patient visits.
Apixio also claims Magna Health Plan was able to identify 2,171 HCC deletes that did not impact their risk adjustment scores because they were either intentional or accurate and had been replaced. Apixio purports that Magna Health Plan agreed with 95.3% of these deletes, meaning they only needed to make final decisions or changes to the last 4.7%.
Why Healthcare OCR is Relatively Underdeveloped
Document digitization in healthcare is nascent compared to other use-cases such as medical imaging, telepathology, and patient population segmentation.
Most document digitization vendors in healthcare are unlikely to be using AI, even if they claim to do so. Many companies do not list any case studies documenting a healthcare client’s success with their software.
Additionally, the vendors we found had little in terms of venture funding and dedicated AI talent. Low venture funding and low AI talent density is generally a bad sign for a vendor’s ability to deliver a result for their clients with AI.
We caution readers to be aware of companies that claim to use AI but do not indicate AI talent, case studies, or venture funding.
The healthcare industry is seeing AI applications developed and sold for not only machine vision technology but other types of software at almost every level of business.
That said, document digitization remains relatively nascent even compared to other data acquisition applications such as text miners and voice recognition note recorders. This is likely the case for three main reasons:
- The technology is primarily seen as a transitionary tool
- In many cases, the technology requires multiple AI applications to fully utilize the data captured
- AI is not a fundamental requirement of accurate document digitization at this time
Many companies may interpret the value of document digitization as a method of converting all their paper documents into data for later use. This is true even if they do not intend to use an AI-based analytics application to analyze that data.
That said, companies capable of becoming entirely paperless have likely done so already or have been looking for these types of solutions for a long time. Healthcare companies that already have a reliable digitization method may not choose to upgrade it with AI.
A document is digitized once an OCR software detects all the letters and typed characters in it and saves them to a file. However, in order to leverage these files in any helpful way, healthcare companies will likely need to use another AI application to analyze them.
This was true for our example of Apixio in that the company’s platform also offered predictive analytics applications based on the client’s chosen data.
It is important to note that moving to fully digital documents may still be a helpful choice for business leaders who still rely on physical forms. Even without the ability to use these digital formats for data analytics, the technology still offers an important value to healthcare networks that need more digital documentation.
Understanding and maintaining one machine learning model may be more than enough for a healthcare company to handle if they are not experienced with implementing this much data. To ask them to adopt two services in order to accomplish what they may perceive as a simple task, scanning documents into their system, may deter them from moving forward with a solution.
Healthcare companies may also have other types of unstructured data they want to leverage when using the solution, such as stores of old PDF scans of medical documents. These could be as organized as possible or stored within such a large database as to be hard to identify.
We spoke to Will Jack and Nikhil Buduma, co-founders of Remedy Health Inc, a medical AI firm specializing in predictive analytics.
When asked how healthcare companies may handle large amounts of unstructured or poorly structured data and be able to make use of it long term, Will Jack said,
This idea of systems getting jumbled up and becoming cludges is not unique to healthcare. If you look at almost any industry this happens. … Innovation rarely happens from within, it’s usually a party stepping in form the outside. I don’t think healthcare systems are going to be able to dig themselves out of this current hole they’re in, it would be exceptionally expensive, it would probably incur a lot of downtime. If you have multiple payers trying to do this, it would be very difficult to agree on a common set of standards and protocols.
Finally, AI may also not be fully necessary for providing healthcare companies with accurate document digitization. While human error is still a challenge to overcome in this business area, document digitization may be too intense of a solution for a business that does not have a relatively high volume of documents to be digitized.
Companies concerned about the long term use of these applications may consider AI more necessary in fields other than document digitization. At the same time, they can wait for larger corporations to innovate on healthcare OCR before taking that risk.
Header Image Credit: Revalton