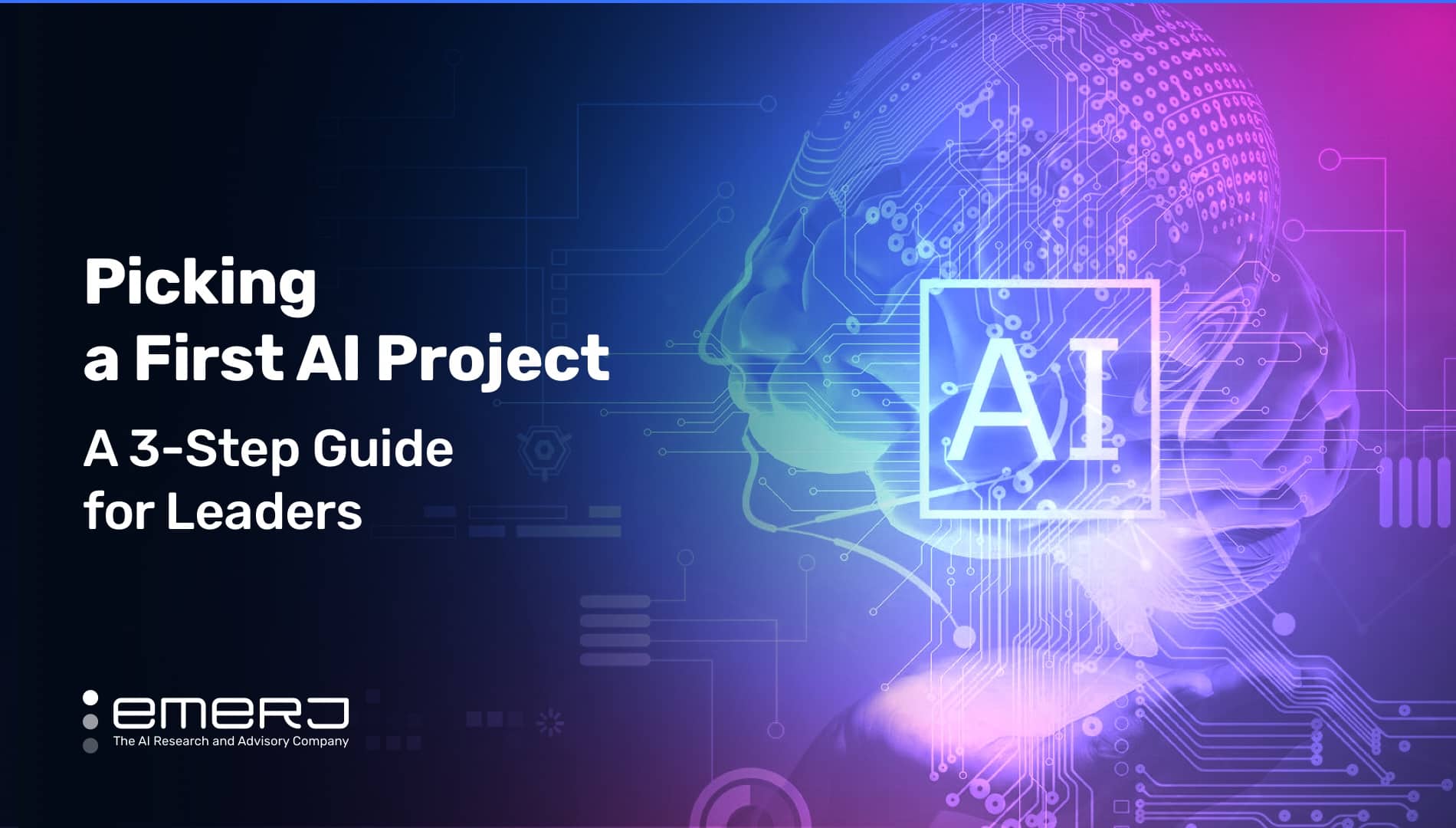
Artificial intelligence is poised to change every industry – and to create trillions in economic value over the coming decades.
However, 80-90% of initial enterprise AI projects fail.
Why?
Often, the wrong AI projects were selected in the first place.
But proper AI project selection isn’t a single skill – it is an outgrowth of executive AI fluency – the general ability of leadership to understand what AI does, how it works, and what long-term vision it should be contributing to (read our full Emerj Plus guide on Executive AI Fluency).
AI champions or C-level executives don’t simply learn to select projects. They must understand use-cases, and have at least a tertiary understanding of how AI works.
They must also have a framework for making project selection decisions, and that’s the focus of this article.
Through interviews with AI leaders at global firms – and through AI project selection experience with enterprise innovation leaders in our hands-on market research services – it’s clear that there is no single playbook for project selection. However, we have distilled three distinct phases or steps of AI project selection that are almost always in place when project selection (i.e. the answer to the question “Where do I start with AI?”) is done right.
It would benefit leaders to pay attention to the advice, particularly towards the latter steps because it might be a bit of a surprise for business leaders, consultants, and AI services leaders who are looking for “low hanging fruit.”
Whether you’re an internal AI champion or an AI vendor, these hard-won lessons should be useful for simplifying your project selection process, and achieving a genuine ROI.
Step 1: Align Key Priorities and AI Opportunities
When it comes to aligning AI priorities and opportunities, the leadership needs to have an understanding of artificial intelligence itself, and of their own business priorities.
We’ll talk about both components to this mix, and it’s important to note that discussions that cross both of these domains will almost inevitably have to involve multiple stakeholders such as internal data science leadership, business leadership, and a variety of subject matter experts. They will often be critical for clarifying AI potential and business priorities the right way. Often we’re brought in to facilitate these kinds of conversations in order to keep them in line and steer them in the right direction.
AI Understanding and Possibilities
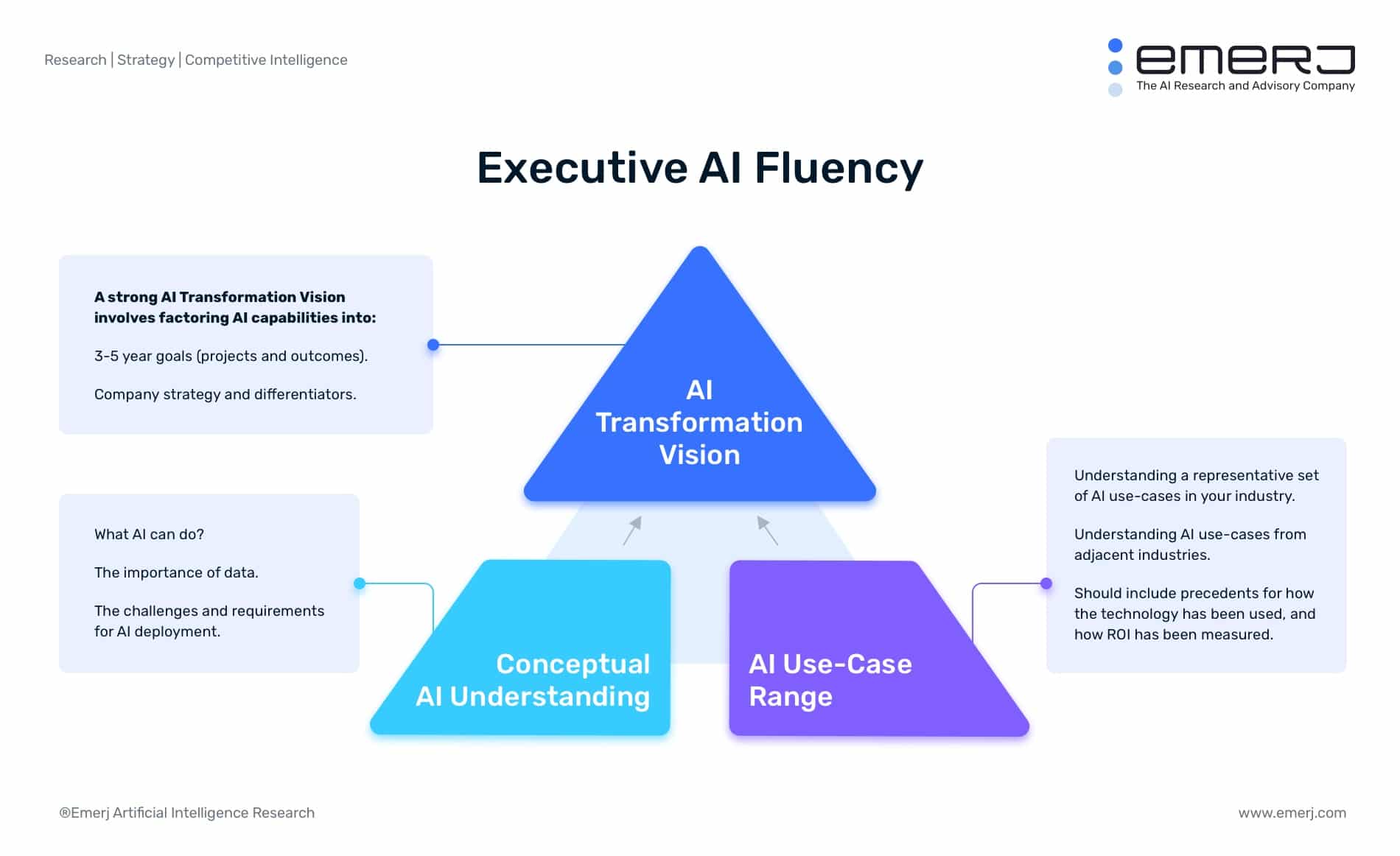
Leadership needs to:
- Understand how AI works
- Understand the use case range of AI
- Develop an AI transformation vision
Understand how AI works
Functional leaders don’t need to learn how to code, but they should understand what makes their company’s data valuable and why its format matters. They should understand how algorithms leverage data and what kinds of results they might expect from those algorithms.
They don’t need to know how to code in Python, but they do need to know that Python is a coding language. They need to know that Python can do certain things that C++, for example, cannot do. They should understand what natural language processing is and perhaps a little bit of the difference between machine learning and deep learning. If they don’t even know these fundamentals, it’s going to be very hard for them to pick a first AI project properly.
Understand the use case range of AI
Functional leaders also need to understand representative AI use-cases in their industry. If it’s eCommerce, for example, they should know what Amazon or Wayfair are doing with AI. When we talked to Ben Lorica, PhD, Chief Data Scientist at O’Reilly Media, he said that overall, a strong case study will enable a business leader or decision-maker to look at a project’s detailed end to end process .
Essentially, you are learning from the mistakes of others before you. Looking at as many relevant use cases as possible for a specific AI technology in your industry will give you a pretty good idea of what you’re getting into before getting into it, and your probable level of success.
Develop an AI transformation vision
According to Salesforce, digital transformation is “the process of using digital technologies to create new — or modify existing — business processes, culture, and customer experiences to meet changing business and market requirements.”
When it comes to using AI to affect this creation or modification, while it can yield some impressive results when it comes to digitizing processes that still involve paper and reducing the time customer service agents spend searching for customer information, leaders are perhaps too excited to jump into AI without knowing the fundamentals of what it entails.
All too often, leaders view AI as a goal, and not a tool. The current focus and excitement surrounding AI can compel leaders to develop a digital transformation strategy around it rather than casting it in a supporting role to achieve the big picture goals of the company. Leaders that understand how AI works and use case range for its industry would be able to develop an AI transformation vision that can work in a real-world scenario.
When these three things are in place, the next step would be assessing if the company should really be moving forward with an AI project. The leadership of the company or the ultimate party that’s signing off on this project should be the executive champion of this AI project only if they understand those three points. Otherwise, we can realistically expect to see not even a short term ROI, never mind long term ROI.
We’ll almost always select AI projects that are destined to fail when leadership has a limited understanding of the challenges of deploying AI, and will almost always become frustrated prematurely with AI projects. We cannot overstate the importance of these three points as there really is no substitute to having leadership that has a firm understanding of these points. For their part, AI services leaders absolutely need to convey their business case in a way that helps the leadership understand these points clearly.
Ideally, only with these three factors in place should we move forward with an AI project.
Business Priorities
The next important thing that leadership should understand is the internal business priorities and to account for any shifting that might occur due to external factors. For example, your business strategy and 3-5 year goals have probably changed pretty drastically since COVID-19. Some businesses are only just now going to be clarifying what their actual forward-looking strategy is and others may not feel secure in their current strategy as of yet.
Business strategy
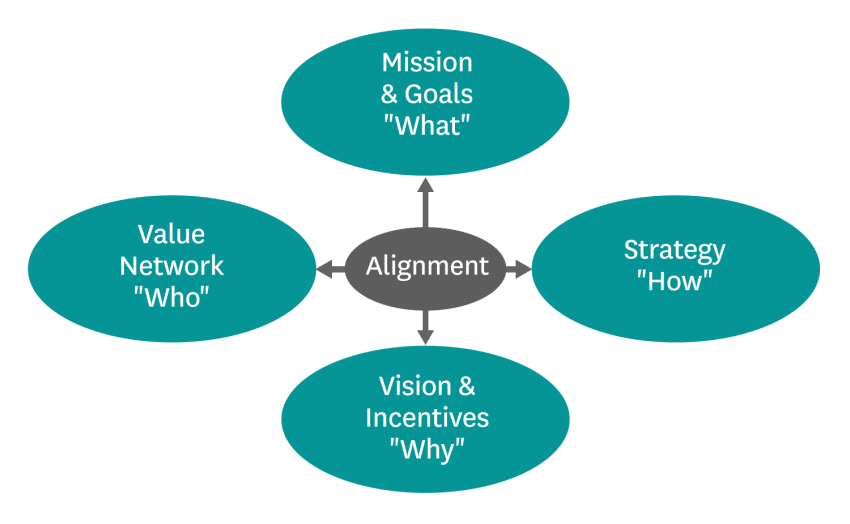
There is much confusion about what a business strategy is with respect to achieving business priorities. Many people believe that a business strategy is an overarching plan that will inform other elements of the business organism, when in fact it is what develops when these other elements are in place, namely, the mission and goals, vision and incentives, and value proposition.
According to this Harvard Business Review article, a good business strategy “…provides a clear roadmap, consisting of a set of guiding principles or rules, that defines the actions people in the business should take (and not take) and the things they should prioritize (and not prioritize) to achieve desired goals.”
The business strategy is the how in the overall alignment of resources to achieve desirable results. When it comes to AI in business strategy, the goal is to use AI as a tool to minimize risk and maximize the bottom line in alignment with the mission and goals, vision and incentives, and value proposition of the enterprise.
In some cases, Ai might not be the best way to forge ahead in uncertain times. Leadership with the requisite understanding of AI and its possibilities in the current context will realize that it would make sense to hold back on deploying artificial intelligence until a forward-looking business strategy and longer term goals can be set with reasonable confidence. Otherwise, the long-term strategic investment in AI might be reshuffled and the relative progress may be lost as priorities pivot in a time of great change.
Long-term goals
A key part of developing an AI business strategy is determining and solidifying long term tangible outcomes and measurable goals, such as the launch of specific products, potentially rebranding, profitability, market share, projected revenues for specific products, and so on.
This also involves key initiatives that would align with the strategy and those three to five year goals. It’s important to note that it’s often easiest to unleash an artificial intelligence budget for projects that are related to existing key thrusts within the company.
For example, if a firm is already investing a significant amount of money, effort, and resources to overhauling its customer service experience, it would make sense to look for AI opportunities in that space because the resources and the efforts are already being dedicated there.
So it’s important to know what those upcoming key thrusts are going to be in the one-year and two-year time horizon. It’s often not a good move to invest outside of the key thrusts of the company.
That said, in some cases for businesses with more AI maturity – that is, they have a good understanding of AI deployment and a strong AI transformation vision to have confidence behind that vision – it might make sense to invest in a divergent AI direction. However, this is not usually the case for enterprises picking a first AI project.
When hoping to accomplish 3-5 year goals with AI, it is absolutely critical that executive leadership plays a part in molding a transformation vision and has a deep understanding of the role of artificial intelligence for the future of the company.
Note: Learn more about delivering AI ROI and framing the strategic value of AI in our Emerj Plus article titled: “How to Succeed with AI Projects – Lead with Strategy.”
Step 2: Determine AI ROI Opportunities
The second key priority in picking a first AI project is determining ROI opportunities for viable potential projects. This involves exploring the map of possible use cases and can be done in two different ways
Known Use-Cases Within Your Own Industry
Exploring use cases in one’s own industry would involve looking at the various companies in the startup ecosystem and sorting them by their credibility and capabilities. It does require experience and a market research methodology to make an effective assessment of the vendor landscape.
However, on a tertiary level, companies can often do this for themselves. It is important to note that when looking at one’s own industry, you should pay particular attention at the leading companies in that industry.
Within banking, within insurance, and within brick and mortar retail, the global top 20 are often going to be the firms that have the most artificial intelligence investments. Studying the use cases of these top companies would theoretically provide you with ideas and direction for initial AI initiatives that could be worth considering.
Known Use-Cases in Adjacent Industries
It is also often worth looking at adjacent industries. For example, a bank may learn from e-commerce artificial intelligence use cases when it comes to online recommendations, or it may learn from insurance AI applications when it comes to backend paperwork processing. Limiting your scope to within your own industry can hold your company back in terms of unlocking the full capabilities of the technology and the full range of ideas that are worth potentially adopting.
Getting a sense of what a technology can purportedly do can inform executive leadership and subject matter experts who are assessing the landscape. They can achieve a firm understanding of the relative challenges and the relative difficulty in deployment for different AI application categories.
It is important to note that not all AI use cases are equal. Some require vastly different data infrastructures in house talent or data volumes than others. Understanding this as you collect different use cases will be critical for prioritizing them and determining what to study.
Step 3: Determine Key AI Initiatives and Outcomes for ROI and Capabilities
The next and final step in picking a first AI project is to determine key initiatives and outcomes for ROI and capabilities for potential AI project opportunities.
While a good cross-functional AI team will select a portfolio of potential AI projects (using a stepwise process as we’ve outlined here) – it is often best to be conservative about how many of those projects can be pursued at once. For most firms, selecting four or five AI projects at once is likely to set teams up to fail. The prime goal is to learn all you can about these opportunities to rank them based on their alignment to establish strategic goals, talent and resource requirements, and alignment with AI transformation vision.
Alignment to Established Strategic Goals
Generally, as we move into a phase I project, we want to find one that aligns very powerfully with current strategic initiatives and critical capabilities of leadership.
Aligning with current strategies can get a tremendous amount of buy in because of its potential financial and strategic value to executive leadership which is why we need to focus on assessing just one or two projects. The focus on learning would lead to the understanding that while short term ROI might be challenging, it is possible to adapt nimbly to artificial intelligence technologies moving forward and put ourselves in a better position in the years ahead.
Alignment with critical capabilities of leadership is a prerequisite to AI the appointment. You want to understand the financial outcomes to expect from this particular project such as efficiencies, revenues, and risk reduction the tangible output of this project and projecting ROI.
Talent and Resource Requirements
You want financial understanding as well as get the direction to take in improving critical capabilities to better adopt AI in the future. You should put particular focus on answering the following questions, which is discussed in detail in our article on Critical Capabilities:
- What talent or skills do we need to develop?
- What data understanding do we need to develop?
- What data infrastructure do we need to overhaul?
The talent or skills you need to develop by the end of this project will depend on the goals of the AI initiative. For example, if an eCommerce company has a lot of problems with fraud and international purchases and is looking to solve those issues, they would want to have a greater understanding of payments fraud data. That would indicate a need to develop the skills of data scientists and hiring subject matter experts to identify and analyze the fraud data on hand and get actionable insights from it.
Understanding data is a tough nut to crack, so it is important to narrow your focus on current issues you want to address. In retail, the issue might be with logistics, so you would benefit from a deeper understanding of logistics data instead. Better access, clarity, and transparency to logistics data can help you make more informed decisions about what to do with that data.
As to the data infrastructure, overhauling it will depend on the current requirements of the company as well. For example, if a large bank wants to move their customer service data from chats, from phone calls, and transcripts from email from divergent silos into one place. Data infrastructure that aligns customer data on a single platform allows us to draw upon multiple kinds of customer touchpoints at one time. In this case, succeeding in upgrading that core data infrastructure would be an actual win from the project.
Artificial intelligence will absolutely be revolutionizing various industries, but it needs to be approached with the right caution.
I’ll frame the importance of this third phase succinctly:
AI initiatives should build value as they go – but they should ultimately serve the purpose of furthering AI maturity, and driving a company towards an AI transformation vision.
Projects done without this eye on the long-term will fall into a trap of plug-and-play promises of near-term value. AI is challenging, AI involves uncertainty, and unless it is seen as an investment in learning and in Critical Capabilities (i.e. the elements of AI maturity), sustained investment and actual transformation is extremely unlikely. Yes, we need near-term wins whenever possible – but we can’t count on every AI experiment to be a win. Every experiment can, however, be a lesson – and an exercise in building the skills, resources, and culture that will bring us to success in the long run – and allow us to pull ahead of our competition.
Takeaways for Consultants: There’s both risk and reward for AI services and consulting leaders and there’s very little benefit to pretending that adopting artificial intelligence in the enterprise is easy. The consultants and service providers that will have the best long-term relationships will be those that can frame the challenges and requirements of artificial intelligence while also having a rounded person perspective on its actual strategic and financial return on investment. The actual process of AI deployment is extremely challenging, but for the companies that succeed, it will be extremely rewarding.
Takeaways for Enterprise Innovation Leaders: Similarly, internal innovation strategy leaders and anyone that try to sell AI to executive leadership should not frame it as plug-and-play technology. That kind of framing is bound to backfire and get a project canceled halfway through its deployment because leadership is going to be surprised that this project requires so much more than an average project would allow.
Alignment with the AI Transformation Vision
The potential of AI for transforming businesses is huge, but it has its own dynamic from other transformative factors. It’s critical that executive leadership has a shared understanding of the teaching value of AI and realistic considerations of deployment when it comes to picking a first AI project.
Without the buy-in from that champion in the beginning of a project, it’s unlikely that we’ll ever get to see even so much as a near term ROI. If you can do that well, then you can position your company, not only to gain a financial benefit from AI, but, be more nimble in adopting and deploying these technologies into the future and pulling ahead of the pack when it comes to AI transformation.
Note: Readers with an interest in molding their own AI transformation vision may benefit from our full report titled Generating AI ROI – Best Practices and Frameworks.