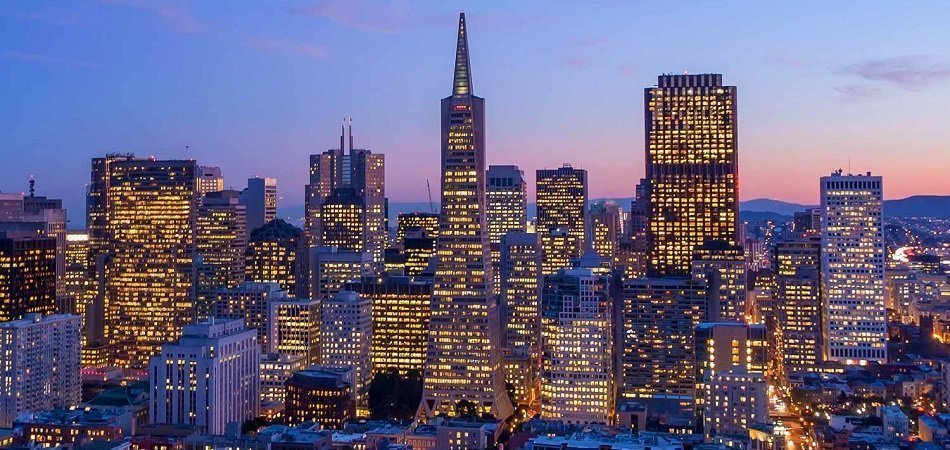
Episode Summary: If one wants to start a general search engine, they’re going to have to compete with Google. If one wants to start a general eCommerce platform, they’ll have to compete with Amazon. But the same dynamics play out on a smaller scale. There are going to be some established players, some big tech giant, be it IBM or someone else, who already has a product.
When it comes to getting a new AI product out to market, how does one compete with the big guys?
This week’s guest is Mike Edelhart, who runs Social Starts and Joyance Partners, seed stage investment firms out in the Bay Area. Edelhart has invested in a number of companies, and in this episode, we get his perspective on not only the patterns among successful AI startups and where AI plays a role in their competitive strategy, but what a “land and expand” strategy looks like for a new product that already has larger and more established competitors.
Subscribe to our AI in Industry Podcast with your favorite podcast service:
Guest: Mike Edelhart, Managing Partner – Social Starts and Joyance Partners
Expertise: media, internet, investing in tech startups
Brief Recognition: Edelhart has a long CEO history at numerous startups going back twenty years. He became a managing partner at Social Starts in 2014 and a Managing Partner at Joyance Partners in 2017.
Interview Highlights
(03:00) When you think about what makes AI either competitive or compelling in terms of an actual business model or a company, what are some of those factors? What makes AI click, not just as a label and as a hype term, but as something that actually brings value to a business model?
Mike Edelhart: Well, I agree with you entirely that artificial intelligence isn’t intrinsically valuable. It’s a set of techniques. In fact, at our fund, we remind ourselves of that by always referring to AI in the plural. It’s AIs, so there is no such thing as generic and flexible and universal artificial intelligence.
There are simply a range of techniques that, if applied well in specific situations, can help companies achieve benefits. And it’s the benefits were interested in, and it’s the companies were interested in, and the AIs, whether they’re derived from neural networks or from machine learning or deep learning or any other technique, are simply a means to an end.
And in terms of our funds, Join Us partners focuses on what we call the emerging science of health-driven happiness. And so there are a range of vectors that can drive that kind of outcome. We’re looking for the impact of science on the way the individual feels, the experience that person has a little happier, calmer, more competent, less anxious, less subject to pain.
And in that realm, there are a whole slew of ways AI can contribute. So one is in diagnosis. So if an individual can have a more precise, or an earlier sense of a problem of any sort, then that can be contributory to happiness, and AIs obviously can help there, and we look at that a lot.
So we have companies that have AIs that can mimic nurse behaviors or can deepen the capability of groups of diagnosticians to come up with better results. And another is improving care if those who provide care, if and when people need it, can be better at delivering the care more targeted, more effective—that too can contribute to happiness. And we have companies that are using neural networks in care settings to predict those patients most likely to have crisis situations, need care.
So the organizations can be more precise and maybe at the extreme end, AIs, along with techniques from neuroscience and other areas, can even begin to predict the future behavior of individuals to move individuals towards certain future behaviors. And an example might be to move them toward a reduction in the use of dangerous substances.
And Boundless Minds, one of the companies we’ve invested in, is using those kinds of techniques, so we see a whole range of ways that AI can contribute, but it’s a contributing factor. It isn’t the one and only element in any of the companies we invest in.
In many cases, it’s the quality of the corpus [of data]. So for AI companies that are using anything related to machine learning that machine learning is going to be valuable in contributing to the degree that it’s precise and effective at pointing towards certain outcomes. And that’s a function of corpus. So an example we’re seeing are US-based life science-oriented companies that are doing early work in China because of the density and depth of the corpus they can get.
(09:00) We’ve heard that it’s kind of the core competitive dynamic of AI. Maybe it’s not the only and maybe there’s detail to be added to it. I’m interested in your layered thought there.
ME: Well, it’s not just the biggest [corpus], it’s the densest. And so that whole set of techniques tends to work best in a situation where the outcomes that are being considered are very few and very dramatic. Will this person have a heart attack? Yes or no? Will this person get a certain disease in a set timeframe? Yes or no, or ranked probability of that happening?
The more varied the set of activities being considered it might be the harder and harder it is to get that kind of competitive value you’re talking about. So we’re seeing these kinds of AIs emerge where the situations are very directed and very structured. Are we looking at the x-ray of someone who has early onset lung cancer? Yes or no? Are we a triage nurse?
The next question is known. It’s always the same next question because you’re following a tightly defined triage procedure and that means every answer to every question is very predictable. So when that’s the case, the AI can contribute a lot. If you’re going to ask what somebody’s going to do next. It’s essentially impossible to predict.
(12:30) Is it unreasonable for companies to shoot for competing with Google and Amazon anymore or do you still think that there’re some big wide vistas like that where there’s going to be big huge companies?
ME: Yeah, I think they’re going to be huge companies, and I want to go back to what you talked about. So the biggest impact of this, and again, don’t think about universal AI. Think about a lot of AIs. There’s an AI that’s very good at predicting a disease. There’s an AI that’s very good at predicting the patterns and the way you spend money. There are hundreds of them, but what those AIs do if they’re effective and they’re going to become effective is they’re predicting what you’re going to need in the very near future.
Well, if those systems are predicting what you’re going to need in the very near future, you are not going to need to search for what you need in the very near future. It’s going to be suggested to you. The most obvious example of that right now is [Amazon’s] Alexa.
You say, “What’s happening in the news?” and an AI is then making decisions and predicting things. It may even make suggestions to you like, “Do you want to turn the oven on, it’s 6 o’clock?” Google’s going to have to change and deal with a whole different universe where many of the things people are now looking for they aren’t going to have to look for because AIs are going to be rather effective. Not one AI, but a whole range of AIs suggesting to folks things they might need, reminding folks of things I might do, presenting them with options.
We probably reached the peak dominance of search. In other words, products being proposed to you, things that you might like being proposed to you and the basis for that is becoming more and more sophisticated. Not just looking at the records or the songs you listened to before to predict a song, but looking at the songs you listened to before and the songs that people like you listen to before and the songs that seemed to be producing the most delight right now, and a whole range of factors being analyzed.
And that’s getting more and more powerful. So why does Google, with all that dominance, have a deep learning group that’s beavering away in the background? They recognize this. Why are they getting more and more involved in health? They recognize this and we’re right at the edge of these kinds of techniques beginning to have deeper impact.
Human beings will interact with one another more actively in different areas. In other words, we go back a few years, the level of interaction about health was very sparse compared to the level of interaction in other areas. But with AIs and data in the phone and on our scan, or on our heads or in our earrings, the amount of interaction, individual they’ll have about health we believe is going to go up massively.
They’ll still be looking for next songs to listen to and, “Will I like these shoes?” But these much more important, deeper, more personal, more consequential actions we think are going to become much more prevalent.
This is my third tech revolution. I got started doing this before the IBM PC and I have seen a number of generations of companies that didn’t exist and then were purported to have technological and market advantages so tremendous that they will never be supplanted, already supplanted. The current generation has similar vulnerabilities. They’re going to have to fight for their lives and they’re going to have to fight for their lives relatively soon.
(18:30) Do you see companies in the future starting with kind of a pinhead point that they can really nail and then expand in capability from there? Do you think there will be some folks who try to dominate a very broad world, let’s say Amazon, with anything you can buy online or Google with anything you can search?
ME: Amazon started and continued With books for a good long while and we’re seeing the same thing here. So an example in our world is a company called Lark. Lark is an AI that can replicate the behaviors of a registered nurse in the face of a specific medical situation.
So the one that we’re focusing on in the beginning is type two diabetes, so when that AI is facing type two diabetes, it can essentially create all of the interactions, the conversational interactions, the information flow that the individual would have with an RN, and it works.
And it’s been proven out in the market and there’re insurance codes for it that are just like the insurance codes for RN and obviously, much less expensive and much more available because that RN is there all day, every day, wherever you are, close to you, monitoring things, and that company’s doing incredibly well. And this is just the first of the medical situations where it could have an impact.
That’s a huge worldwide benefit right there. And then there are hundreds of other similar areas and every single patient and every single situation makes the AIs driving those RN-like behaviors more and more precise and more and more capable. So it has the potential to just kind of grow.
Start with benefits, use early benefits to get big. Use that early bigness to get even bigger and potentially transform the way individuals interact with medical professionals, the way individuals interact with diseases and then if you can do that, you can start, one believes, moving it forward and say, “Well, why wait until this person gets the disease? Why don’t we start interacting with the person long before the disease ever emerges,” and start reducing the rates at which the more overt manifestations of this problem occur, and then you really are starting to change the way society works.
For early stage companies, in particular, doing one thing really well is very hard, so do one thing really well, get the benefits from that. Why diabetes? Because it’s so widespread and the impacts of diabetes are so significant. So now you have relationships with a lot of individuals who have this problem with insurers, with doctors, with this whole universe and you know a lot about it and from that you can then say, “Well, people who have type two diabetes also may have a tendency towards being overweight, or a need to exercise more, or there may also be a correlation with say rheumatoid arthritis,” and you can take advantage of the relationships, data, and infrastructure you already have to spread out.
Then you just have that much more data and more relationships and deeper relationships and just keep expanding in that way. Sort of swinging from tree to tree.
Subscribe to our AI in Industry Podcast with your favorite podcast service:
Header Image Credit: Bay City Guide