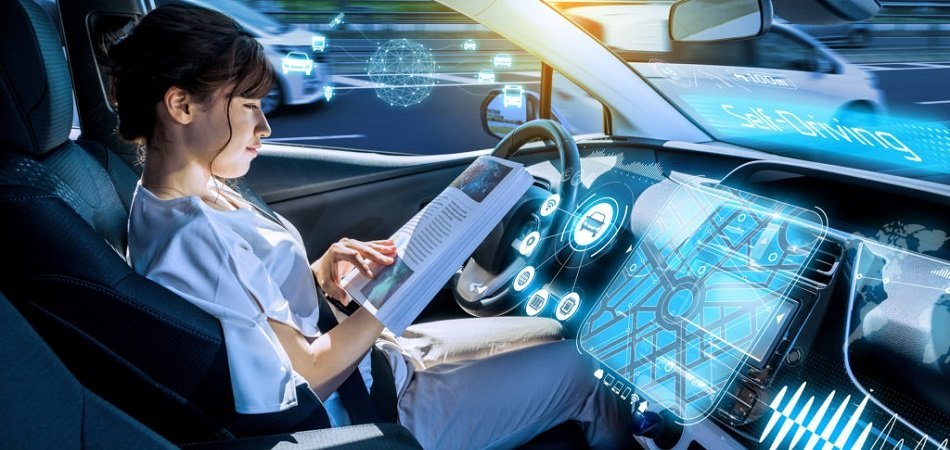
Danny Lange heads up the AI efforts at Unity, one of the better-known firms in terms of simulations and computer graphics. They work in several different industries, but this week we speak mostly about automotive.
This is a man that has been in the AI game since before it was cool, and now he is working on some cutting-edge projects with Unity. In this interview, we speak with Danny about where simulated environments are becoming valuable.
We hear about simulations mostly in the context of video games, and of course, Unity does apply their technology in that domain, but what about a space like automotive, where navigating within an environment is important?
Certainly we need to have physical cars on the road to drink in data from physical roads and physical environments, but is it possible to splinter some digital cars into digital environments that model the physics, that model the roads, that model the same number of pedestrian risks, and see how well they succeed in all these different environments with no real physical risk of damaging an actual vehicle or an actual person on the road?
As it turns out, there’s value there.
We are put in touch with Lange by the folks at the BootstrapLabs Applied AI Conference 2019 in San Francisco in April 19th. I spoke there last year and I know the folks at BootstrapLabs who run the event. Before they put it up they gave me a list of interesting folks to speak with, and that’s what gave me the chance to speak with Lange this week.
Subscribe to our AI in Industry Podcast with your favorite podcast service:
Guest: Danny Lange, VP of AI and Machine Learning – Unity Technologies
Expertise: computer science/AI and machine learning
Brief Recognition: Lange earned his PhD in Computer Science from the Technical University of Denmark in 1993. Prior to Unity Technologies, he was Head of Machine Learning at Uber, General Manager of Machine Learning at Amazon, and Principal Development Manager of Big Data Analytics with Hadoop at Microsoft.
Interview Highlights
(04:30) Could you give us a sense of where the simulated world environments and physical forces are playing a role in automotive?
DL: If you take a little step back and think about gaming, when you play games you are in this 3D world with physics; you collide with things, you fall down, you try to survive, things like that.
Then think about the automotive world, where I can now drive a virtual vehicle on a road where you can drive it through a city. I can see how the environment inferences the decisions of that vehicle, say it’s a self-driving vehicle, that’s basically a game-changer, and that is basically something that happens today, that you can basically simulate vehicles in traffic and learn from that.
(05:30) What makes that possible?
DL: There is a lot of what we call “world building” in it, so you have to build cities, buildings, trees, and sidewalks and stuff like that. And it doesn’t stop there; you also have to build what we call the “dynamics of the episodes,” which is pedestrians, bicyclists, other cars moving around, traffic lights…time of day…different weather.
Then quickly you have a virtual world in which your vehicle now has to drive through traffic and using machine learning to train the vehicle, using machine learning to actually generate the dynamics of the environment to challenge the self-driving vehicle.
(07:00) So the idea is that you can run that car through 2000 permutations of traffic in different environments and weather at once hypothetically if you have the compute power and that if we do enough of that… we can train a new model of car on all the normal road conditions and in a faster time than if we had 200 cars driving in the real world. I guess that’s the promise, right?
DL: Yeah. Think about it this way. If you take Alphabet’s Waymo company, they are building a self-driving vehicle. Up to today, they have been driving about 10 million miles on real roadway with those vehicles. In the US, we have about one fatality per one hundred million miles, so just driving 10 million miles is actually really not gonna tell us much about how safe that vehicle is.
So what we have to do is try to move into the virtual world because as you said we can have 2000 parallel driving experiences happening. Well, 2000 is actually low, make it 10 or 20 thousand, there are many servers in the different cloud services and we have made Unity able to actually execute in cloud services, so you can really scale out.
And then secondly, you no longer have to run on wall clock time. Think about how all games are made for people, and people play games in human time, but if I wanted to train a vehicle, I can actually just speed it up and basically have it drive at some virtual time that is way faster than wall clock.
That is where that amount of training helps one particular aspect of AI systems which is the ability to generalize, so if the system hasn’t seen enough, crazy, unlikely episodes…then it’s really hard to throw a real-world crazy episode at it, where it hasn’t seen anything like that in the virtual space.
(10:30) What are the parts that in virtual space we can model very close to reality? And what are the parts that are harder?
DL: What we have found is that there is a shift happening today where we are starting to realize that going into more and more precise, more and more accurate physical models…trying to model the real world as close as possible, there seems to be another trend [instead]. [This] is …model[ing] the world with a lot of noise instead.
So instead of precision, we just throw a lot of noise in there, so things like friction, we let it have a lot of variation, gravity, we let that have variation, and then what we do instead is that we ramp off of the amount of training data to basically now train…machine learning models.
They…have seen more variation, so when you bring that into the real world, there’s this greater likelihood that the real world is now within your distribution. So instead of doubling down on precision, we have to double down the amount of data, throw noise into it, and basically get more robust systems out of it, and that’s a fairly new trend.
There has been this attempt for the longest, it has almost been on for decades where we try to implement stuff in very deterministic code and very finite terms, if statements in there…and true and false and all that stuff.
The world is actually, if you go really deep down at the atomic level, well it’s going to mechanics which is basically statistics. So nothing’s quite 100% anywhere, and I think a bunch of companies…are really successful today because they realize that customers are not that deterministic.
You operate in a statistical world, and when you look at customers, when you look at self-driving vehicles, when you look at robots, there are all these other things going on in the environment, that if you tried to model too accurately, you’re just gonna miss it, you’re not going to be able to predict that.
So we see this massive shift of people using the term “big data” all the time. I’m not gonna go down that path, but it is kind of big simulation then. With a lot of noise in…you have a system that basically has seen most of what’s possible.
(15:30) Where are the business areas where we want to run simulations to get value?
DL: The foremost one is when it comes to autonomous vehicles or self-driving cars. That is the number one area where we see the kind of simulation that I just described. There are also a lot of other simulations going on, and some of them you may not think of them as just strictly simulations, but…imagine the signing, the interior of a vehicle, and you have that entirely done in software.
Now you put on your virtual reality goggles, your headset, and you can sit down in a chair, but you can see the interior of the car in front of you, you can try to figure out if you can reach out and touch all of the buttons with it, make sense whether it is too far from yo. So actually you are simulating the experience of the in-vehicle experience before you ever put one piece together.
[The value] is actually in a pretty wide array. Some of it is visual, basically being able to train the computer vision technology behind cameras. We call that the cognitive side of it, seeing what’s going on around the vehicle and navigating. But there are also things like lidar and radar.
We also simulate lidar and radar, and the whole purpose of this is to actually build these machine learning models that are able to interpret the lidar signal, the radar signal, and the visual signal, and put it all together and build the most perfect perception of the world around the vehicle.
That stuff cannot happen on the street. When you drive on the street it’s too dangerous, and most of the time there is not really a whole lot of stuff going on, you are just following the car in front of you.
I can tell you 98-99% of the time nothing interesting is going on because you are really just trailing the car in front of you.
(19:30) What do you think could be the future of this technology in automotive?
DL: That would obviously be when the vehicles can cooperate. When the vehicles can cooperate with each other, they can cooperate with the traffic lights, they can cooperate with the city that they drive in. That’s not just for automotive vehicles, it’s just the whole world.
We’re going to see these AI systems initially be very tailored towards specific needs, but when they are able to communicate between themselves and orchestrate solutions to problems that we don’t implement, so you can say multiple AI systems collaborate to solve a task, that’s when we’re really gonna see a change, whether its self-driving cars or many other aspects of society.
We’re talking about not a lot of smart, very perceptive individual vehicles, but a smart web of intelligences that are able to interact to make the whole system of moving people and things better.
Subscribe to our AI in Industry Podcast with your favorite podcast service:
This article was sponsored by BootstrapLabs, and was written, edited and published in alignment with our transparent Emerj sponsored content guidelines. Learn more about reaching our AI-focused executive audience on our Emerj advertising page.
Header Image Credit: Innovation at Work – IEEE