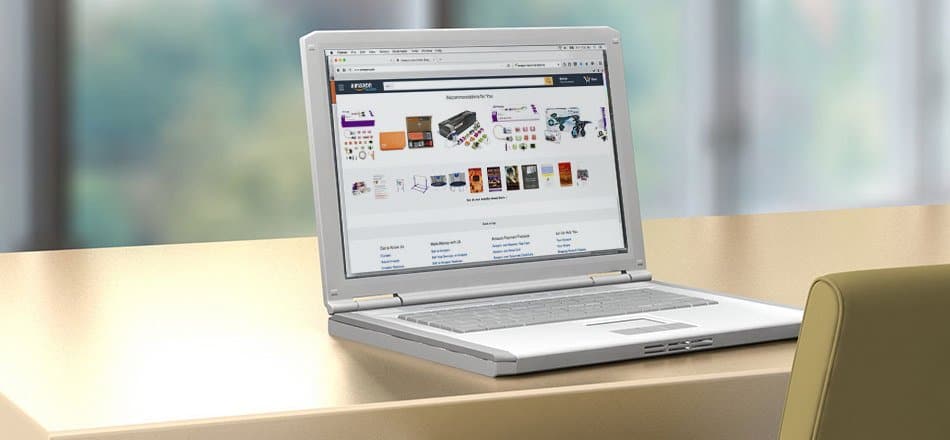
Episode Summary: When we think of recommendation engines, we might think of Amazon or Netflix, but while consumer goods and entertainment might be the most prominent domains for recommendation engines, there are others. This week, we speak with Madhu Gopinathan of MakeMyTrip, one of the few Indian unicorn companies, about recommendation engines for travel companies.
According to Gopinathan, MakeMyTrip’s recommendation engine has to figure out the best hotels for customer given their destination, but recommending hotels to first-time users and those who don’t frequent the site can prove challenging. How does a travel company’s AI-based recommendation engine start the process of making well-informed recommendations?
Gopinathan talks to us about how a recommendation engine might match people immediately with their preferred product or service when the on-site data does not exist to inform the AI-driven recommendations.
Subscribe to our AI in Industry Podcast with your favorite podcast service:
Guest: Madhu Gopinathan, Vice President, Data Science MakeMyTrip
Expertise: Natural language processing, machine learning, cloud computing, data analysis, software engineering, Python
Brief Recognition: Madhu is Vice President of Data Science at MakeMyTrip.com, where he leads initiatives in developing intelligent systems based on natural language processing and machine learning. Prior to MakeMyTrip, Madhu was CTO at VMobo, and Principal Architect at Analysis. He was on the product incubation team at Infosys, and he was an architect evangelist at Microsoft.
He also volunteered his skills in developing for fraud detection algorithms for the Unique Identification Authority of India. Earlier in his career, he served as a software engineer at TCSI and software architect at Covad Communications.
Madu earned his Doctorate in Mathematical Modelling from the Indian Institute of Science.
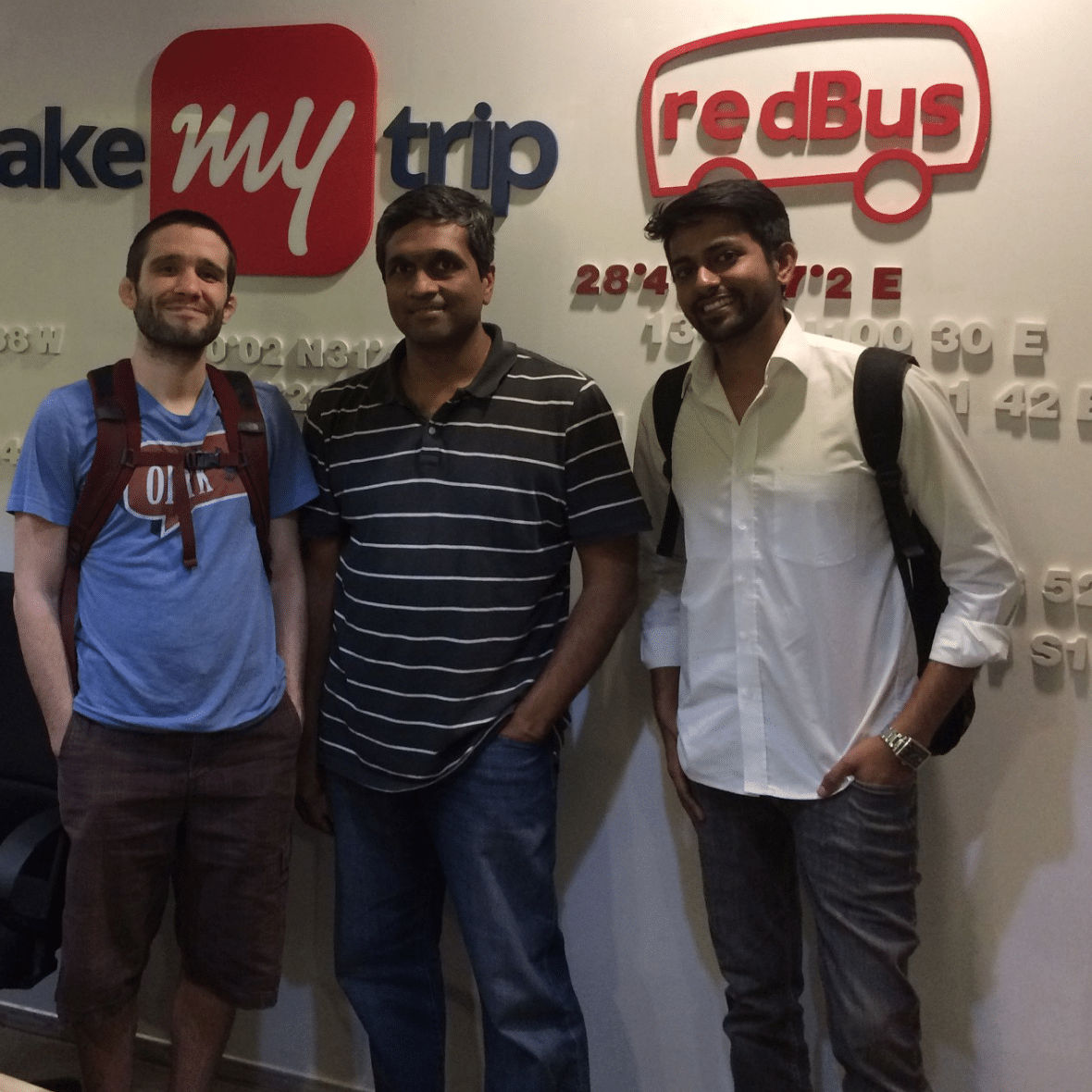
This article is part of a broad series of interviews for our in-depth AI in India analysis – read the full report here, and explore more quotes from AI execs and researchers from around India.
Interview Highlights
(03:25) You say that when a selection comes up, there has to be some kind of ranking because no one wants to go through a list of three thousand things. How do you explain to business people how that complex recommendation problem is solved?
Madhu Gopinathan: On one side, we have consumers, and on the other, we have hotels. From historical data, I can extract customer preferences in hotels. We collect a lot of data about customers, such as device type and their history. We utilize this information and match it with hotels.
For example, for someone with a top-end iOS device, we will probably book them in a four- or five-star hotel, higher than a lower-end Android. That’s one factor that helps us map it to the right hotels.
There are other factors, such as weekday bookings, the number of adults, length of stay. These pieces of information help us narrow down a reliable selection, and we constantly monitor and improve it.
(05:20) In some circumstances, you will have more data than others. You may only have the device they are using and from where they are accessing the internet. Others may have an account, with their travel history in the last eight months. Given the limited or excessive amount of information about this user, and their recent behavior, what can you present to them? Do you have a model for each kind of circumstance?
MG: The principle is that the more information you have, the more reliably you can narrow down [the recommendation]. When you have less information, you can pool that information with people who have similar profiles. In case you have no information about the customer or these methodologies, you can always recommend the popular hotels.
We also discovered nuances. The popular weekend hotels are different from the popular weekday hotels. If people are traveling on business, they prefer hotels in certain neighborhoods. This is also different from the preferred hotels and neighborhoods on weekends. Again, there are other conditions like time-of-day bookings, date of check-in, number of people
(06:47) In a newly developing city for which you don’t have historical information related to hotel bookings, how do you start to get proper hotel recommendations?
MG: We typically do some exploration and exploitation. Just like visiting casinos in Las Vegas. You don’t stick to just one slot machine. You will try to experiment. But when you find a good one, you play it more times. In the same way, we recommend some of the usual hotels, but also recommend new ones to find balance.
To find the new players in the league, you would want to give them chances, then evaluate which similar groups of people are likely to agree with us.
(10:55) Where do you see recommendations improving as the AI technology progresses?
MG: One is in collecting more information about people and hotels. As the integration with hotels becomes stronger, we can collect more information. We also collect information from customers through their reviews. We can get people to contribute more content through images and videos as machine learning gains more traction. In this area, called aspect-based sentiment analysis, people are typically used to relying on the average rating in a review. We are going one level deeper.
If a reviewer writes “Hotel is great. But food is not very good,” some aspects provide a positive evaluation, while some aspects are not very good. This is useful for us. We can extract dimensions from the text data. We score hotels based on these dimensions from multiple reviewers. These can be used to aid the discovery process.
These can also be useful to hoteliers, to see what aspects customers are complaining about and improve on those aspects. So we’re helping both sides, customers and partners.
(14:30) Your company is moving into chat and considering voice technology. What is the vision for these technologies?
MG: For the post-sales services in travel such as to check status for booking, e-ticketing, changing dates, etc., chat is great. But in a country like India, chat is only useful or relevant for one segment of the population. There could be a larger segment that is more comfortable with natural language, with voice as the primary medium. How can we help them? That’s how we are building voice models and finding partners.
For the customers on the voice channels, instead of recommending 10 hotels, we can recommend only three. We can ask them a question about their preferred location, have a conversation, and help them book the right hotel.
(17:05) Similar to Amazon’s virtual assistant Alexa, it might involve a good amount of experimentation to see how [voice] will click.
MG: It’s clearly an investment in the future. But we want to put it out and see how people will adopt it.
Subscribe to our AI in Industry Podcast with your favorite podcast service:
Header Image Credit: University of Pennsylvania