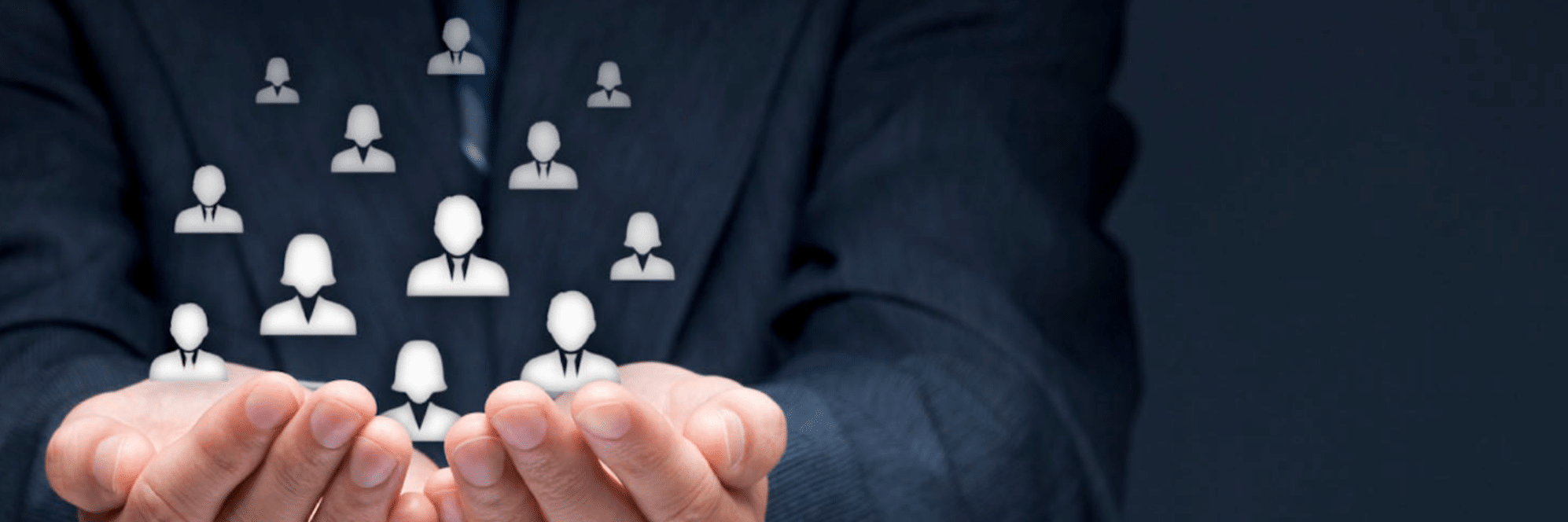
A myriad of customer service channels exist today, such as social media, email, chat services, call centers, and voice mail. There are so many ways that a customer can interact with a business and it is important to take them all into account.
Customers or prospects who interact via chat may represent just one segment of the audience, while the people that engage via the call center represent another segment of the audience. The same might be said of social media channels like Twitter and Facebook.
Each channel may offer a unique perspective from customers – and may provide unique value for business leaders eager to improve their customer experience. Understanding and addressing all channels of unstructured text feedback is a major focus for natural language processing applications in business – and it’s a major focus for Luminoso.
Luminoso founder Catherine Havasi received her Master’s degree in natural language processing from MIT in 2004, and went on to graduate with a PhD in computer science from Brandeis before returning to MIT as a Research Scientist and Research Affiliate. She founded Luminoso in 2011.
Subscribe to our AI in Industry Podcast with your favorite podcast service:
In this article, we ask Catherine about the use cases of NLP for understanding customer voice – and the circumstances where this technology can be most valuable for companies.
Why Customer Voice Needs Artificial Intelligence
Making sense of the meaning in customer or user feedback (through phone calls, chat, email, social media, etc) is valuable for nearly any business. The challenge lies in finding this meaning at scale, and across so many different data formats.
Catherine tells us that, historically, businesses manage these different customer interactions by putting them into appropriate “buckets” or categories. For example, if there are 70,000 customer support email messages received in a particular month, the company might have a manual process of flagging each message as “refund request,” “billing inquiry,” “purchase request,” etc.
However, manual categorization becomes nearly impossibly challenging at scale, for a number of reasons:
- While all customer service emails and call center calls might be labelled manually by the customer support rep who handles then, other kinds of data (tweets, chat messages, comments on online forums) may never receive the same kind of labelling.
- A company with pre-determined “buckets” (categories) for customer service inquiries is unlikely to be able to pick up on new, emerging trends in the particular words, issues, or phrases used in customer requests. This inability to adapt and find new patterns could limit the company from seeing new opportunities for improvement, or new emerging issues for important customer segments.
- Human beings don’t categorize content in the same way – and discrepancies and misunderstandings in categorization can make customer feedback useless.
Many companies look to technology that can detect common patterns for these messages, create categories for each found pattern, and flag them appropriately for the attention of the business owners (which includes finding new patterns). This is a job for machine learning.
“Sentiment analysis” – the process of computationally identifying and categorizing opinions expressed in a piece of text – has become a somewhat familiar term. Catherine tells us that truly understanding customer voice involves much more than simply detecting emotions within text, and includes:
- Finding new “entities” (products, brands, people) which are gaining or losing frequency in customer feedback
- Determining customer sentiment – not just overall – but in relation to specific entities or types of customer issues
- Showing changes and trends in customer feedback over time
- Understanding the different patterns of feedback across unique channels (call center, chat messages, social media, etc)
The problem is many text analytics techniques in the past require a significant amount of data and effort in building rules and anthologies in the beginning, and still be unable to provide a true picture of what is actually being said by the customers.
This can be daunting for many companies because of the high costs often involved in streamlining and cleaning company data. According to Catherine, voice of the customer AI applications involve less fuss – and potentially a more clear ROI – than many other business applications of AI and machine learning:
“If a company is looking working in AI and doesn’t really know where to begin, voice of the customer is a great place to start once you’re doing it right because you can get a real impact and you don’t have to explain a lot about what’s going or why things are possible and things like that. You can just get results that allow you to get more AI buy-in throughout an organization.”
Below are a few of the use cases Catherine discussed for NLP technology for “making meaning” of customer communication at scape and across channels:
Use Case: Telcom Industry
Employee satisfaction and retention is a critical issue in the tech sector because of the high churn cost (nobody wants to hire and fire $200,000/year engineers rapidly, retention is key). Catherine tells us that telecommunications firm in the UK received many complaints from employees about the food quality and other small aspects of the company that they believed contributed to the high turnover rate of the company.
The company sought to resolve these issues at face value, expecting that it would reduce the turnover rate. However, it did not reduce turnover in the slightest, and the reason for this is that the complaints they addressed did not necessarily align to the reasons people were leaving the company.
The company failed to look at the context of the complaint and how it aligned with the culture of the company. Many people in the some tech industries like to complain, and complaining can actually lead to retention. Catherine recounted her own experience:
“You find example complaints about food quality and other factors where – everybody complains about it, but nobody leaves because of it.
Sometimes complaints are from people who want to stay and see improvements… people love to be there are often complaining. I went to MIT so I know all about this phenomena, there’s a social thing about complaining, so these are things you need to understand if you’re a company.”
The result of this lack of insight into the culture was wasted effort in addressing non-issues, and none at all on issues that mattered to their employees.
The company would have gotten better insights by looking at individual complaints, subsequent actions of the employee to see how it tied into their retention, and discerning patterns of behavior that would have provided them with the information they needed to increase employee satisfaction.
Tying employee feedback (in the form of unstructured text) to actual data on employee performance and churn potentially allows companies to get at the heart of why people are leaving, not just what they socially complain about on Slack or group chat.
Use Case: Focus Product Improvements on What Matters to Customers
Consumer electronics is a highly competitive sector, and companies battle fiercely to win over customers and market share. The challenge for a consumer electronics manufacturer is to figure out the features that matter to potential customers that would drive them to purchase a product.
People looking to buy televisions, for example, are most likely not aware of the latest features available in the newest models in the market, and may have difficulty in making sense of all the buzzwords. Winning over these discerning customers is a challenge.
Catherine tells us that one particular manufacturer sought out a large number of reviews on televisions to understand what distinguished the brand in the mind of a wide range of customers, its perceived strengths and weaknesses, and the features which potential customers wanted to have in their televisions.
The information derived from this text analysis of those reviews helped the manufacturer make more informed decisions with its expensive engineering resources. Catherine claims that the company developed and put in the features that resonated with consumers, which likely contributed to more television sales. Catherine states:
“People can really align what is going on in the unstructured data with what’s driving sentiment, what’s driving these KPIs, and saying these things really matter.”
In advising companies about where to focus NLP voice of the customer insight, she recommends identifying critical business metrics that matter for a company’s strategy, growth, or profitability, and gleaning customer insight on these areas specifically (basically asking: “What customer insights will move the needle on our most important metrics?”).
Use Case: Making Meaning of Customer Complaints
In another case, a company was getting a high volume of returns (refunds) for a particular computer model from various parts of the world. The customers returning the product complained about various things regarding the way the computer connected to the power. Catherine explains why NLP might be useful in discovering this issue:
“This is where having an NLP system can be important – there’s a million ways that customers might refer to an adapter than plugs into the wall. They might call it a ‘cord’, a ‘plug’, a ‘connector’, and these might be impossible to pre-program into a system upfront.”
Upon analysis of various ratings and reviews using advanced NLP to understand the context, it turns out that the problem causing the most returns and refunds with the computer was that the device connected to an app store, which did not accept foreign credit card payments.
People that purchased the product could do so using a foreign credit card, but could not purchase anything on the app store, making the item far less useful than they had anticipated. The licensing restriction was the real reason for the returns of the product, and the company failed to pick up on it.
Pre-set customer service “buckets” might not pick up on a trend of this kind, but “listening at scale” with NLP was able to do so – according to Catherine. She described the flaw in the process:
“…there isn’t a set of six key words that describes this problem. It’s a much more sort of effused problem, right, it’s a much more hard to put your hand on it, and that’s really interesting. And that’s what modern NLP lets you do.”
Different customers wording the same frustration in different languages and in different ways is hard to detect even with AI, and it’s promising to think that these new kinds of issues can be unearthed with the pattern recognition of NLP.
Use Case: Automotive Manufacturing
The automotive manufacturing industry can save a significant amount of money by optimizing and automating its customer service workforce. Catherine tells us that a Japanese company decided to redesign its customer service workflow by using Luminoso’s software to analyze its customer support tickets.
The company did not have a particular customer problem to solve – Catherine says the firm simply wanted to improve its customer service system.
The company already had a system they used to categorize customer complaints that they expected to integrate with the AI system. However, there was one category where the customer service agents would put in customer complaints they could not address or understand. Company employees considered many of these complaints to be ridiculous or frivolous, and did not know how to productively use the feedback.
The AI looked at all the categories, and automatically analyzed how each one could be broken out and used in the workflow, including the problem category. The AI system helped the client find some interesting new insights to help diagnose an unknown issue.
It found that a group of people were talking about bad smells in a particular model of car, describing it as a “dog” smell. Customers from different geographic regions were bringing their car to service centers and complain about this unusual smell. The service center staff would then take note of this interaction with the customer and enter it into the system – but there was no way for dispersed human service staff to “connect the dots” on the “dog smell” issue.
The computer also noted another group of people that complained that there was dew or water in their car in the morning, and it was the same car model. Catherine claims that the AI detected a pattern between these two groups, and concluded that the presence of moisture in the car and the dog smell were related factors.
Given this information, the company investigated and discovered that the air-conditioning hose had come loose in that particular car model, and the water was accumulating underneath the dashboard. Over time, it started growing mold, which would account for the “dog” smell complaints.
Using unstructured text data, the company had discovered a connection between “dew” (wetness) and “dog smell” (mold) – and finally, the company had an actionable step to take to improve their product and their customer’s experience.
The company issued a dealership memo, directing service centers to fix the hose problem in that particular car model every time one came in. Catherine summarized the situation:
“So none of these customers were nuts. There was actually something wrong with their car. But it wasn’t at all what they were looking for… And so they were doing all that kind of stuff, and in the meantime they found hey there’s something big wrong with this model of car.”
The company was addressing potential issues in the future, not knowing they already had an ongoing issue that was adversely affecting customer satisfaction with their product. Common sense NLP, which tied in two related patterns, showed them that. They were then able to address the issue before it became a major problem.
Closing Thoughts on Making the Anecdotal, Measurable
According to Catherine, clients are interested in tuning into the voice of the customer for a variety of reasons, including:
- Exploring a particular set of known customer issues in greater depth (for example: An insurance company gaining more clarity on why customers are switching to their main competitor)
- Exploring customer sentiment and customer feedback to find new patterns (such as the story of the auto manufacturer discovering the mildew problem in the example above)
- Seeing trends in customer support issues over time (a hotel chain that implements a new and improved room cleaning process may look to see if customers are complaining less about room cleanliness)
- And more.
While the particular insights that clients seek are sometimes different, the goal of these various NLP initiatives generally boils down to one of two goals which resonate with any business leaders:
- Improve products and services
- Improve customer experience and customer lifetime value
As Catherine mentioned in the telcom example, improving employee satisfaction (understanding the “voice of the employee”) is another application for NLP, and one that she believes is becoming more popular.
About Luminoso
Luminoso helps customers improve the customer experience by understanding and quantifying customer feedback at scale, including:
- Extracting value from untapped data sources
- Moving from anecdotes to quantifiable insights
- Lowering the time and cost of processing unstructured data
- Gaining visibility into key topics and track trends over time
This article was sponsored by Luminoso, and was written, edited and published in alignment with our transparent Emerj sponsored content guidelines. Learn more about reaching our AI-focused executive audience on our Emerj advertising page.
Header image source: Infotech