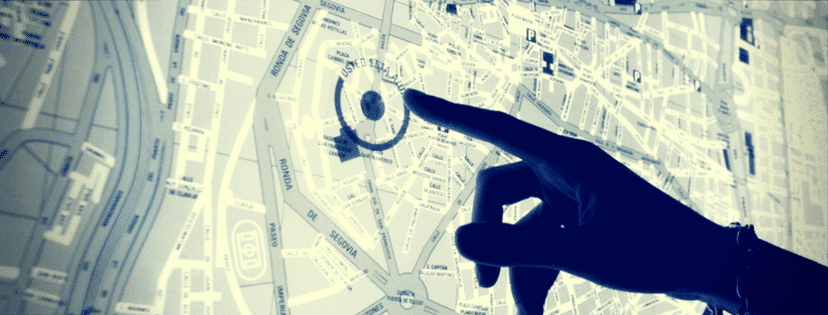
There is a certain level of stigma that exists around using machine learning and location data in business applications, understandably due to risks inherent in exploitation of individual privacy. But if we look under the hood of society’s daily web of interactions, we see that the location information economy—from GPS to radio signal based-triangulation to geo-tagged images and beyond—is now almost ubiquitous, from the moment we track our morning commute to the end-of-day search for healthy and convenient take-out for dinner.
As quoted by The Location Forum in its 2013 “Location Data Privacy Guidelines”, “location information…is rapidly becoming the newest “information weapon” used by CIOs, CMOs, COOs and digital strategists to gain a competitive advantage.”
Guidelines on how to collect, store, and use data are constantly evolving, quick enough that many companies need whole legal teams just to stay abreast of what’s kosher and what’s out of bounds on an almost daily basis. Being aware of data privacy laws is an essential component of leveraging information that is invaluable for today’s top business competitors. And while there are uses that consumers love to hate (mobile advertising, for example), there are also applications that have the potential to help protect both consumers and businesses on an unprecedented scale, such as purchase fraud protection.
The uses of machine learning in location data for business and industry are far more diverse than we can cover in one article; however, we’ve collected and expanded upon some of the current approaches that companies and organizations have taken in applying this technology.
Current Machine Learning and Location Data Applications
Geolocation for Preventing Fraud
In our most recent interview with Cloudera’s CTO and Co-founder Amr Awadallah, we learned that MasterCard uses location data to help prevent fraudulent transactions in real-time. To date, the payment processing company has built a data hub (i.e. data lake) the size of 10 petabytes, which includes stored transactions across their network over many years, and more recently information like a customer’s location when using the bank’s mobile application.
This information can tell where a customer is standing at the time of a transaction—are they actually at the ATM where money is being withdrawn—and use real-time analytics to prevent fraudulent transactions. As Awadallah noted, “Four or five years ago, whenever you would travel, your first transaction would always be blocked…now it’s less likely your first transaction will get blocked, it’s very rare today.”
In October of 2016, U.S. Bank also made a public announcement of its use of the opt-in Visa-developed Geolocation Service to help protect consumers from both credit card theft and unnecessary headaches when dining out at a new location.
Cloud-Based Sensor Technology for Tracking Mobile Fleets
Businesses small and large now have access to GPS and sensor-based technology to track vehicles in their delivery and service fleets. Fleetmatics, which is owned by Verizon, has introduced GPS-powered fleet tracking solutions for service companies who want to, for example, track the average “speeding, idling, miles driven and engine-on or off times,” any of which can trigger programmed alerts on a mobile device.
AT&T has been a pioneer in fleet-tracking technologies over the past five years. A 2016 Emerj interview with Mazin Gilbert, assistant vice president of Intelligent Services Research at AT&T Labs, provided insights into how the telecommunications company has used cloud-based sensor technology and predictive analytics to prevent vehicle failures – with the goal of improving the customer experience, preventing unnecessary costs, and maintaining employee morale.
Geolocation & Social Check-Ins for Recommendations & Sales Projections
A consumer’s location, often cross-channeled via mobile geolocation and social check-in technologies (such as Facebook and Twitter), has become essential for retail and service applications wanting to provide customized recommendations and advertisements. Restaurant applications such as EatStreet or DoorDash (to name a select two) can provide personalized recommendations based on user location and historic behavioral preferences, and others like OpenTable can provide a list of recommended venues and even reserve table space by modeling probable locations that a user might visit at any given time.
Open-source platforms like Mapbox are making it easier for developers to integrate evolving location data technologies into mobile and online applications across industries, from agriculture to real estate. A next-step application currently being studied and engineered is predicting future locations based on past places visited, a difficult problem to solve that requires “identifying the structures and routines hidden in users’ past locations.”
Geolocation and beacon intelligence merged with predictive analytics has also been used by companies to help predict quarterly sales results, like when Foursquare’s CEO (accurately) predicted a 30 percent reduction in Chipotle Q1 sales for 2016.
Geolocation & Geofencing for Online Retail Experience
The State of Retailing Online (SORO) 2016 report, a joint study by Forrester Research and Shop.org, found that less than 10 percent of retailers have a focused plan to invest in location technologies for 2016, a statistic that seems to scream emerging opportunity for online retailers looking for an edge in the market.
Opt-in push notifications and GPS-enabled content, including store updates on things like sales and promotions, help retailers and stores leverage consumers’ increasing acceptance of using geo-location-based apps. In an article for Forbes, Michael Jones, senior vice president of retail and brand solutions at RetailMeNot, Inc., recommends giving customers control over these options in order to see increases in a company’s bottom line. Starbucks’ Mobile Order and Pay app, for example, uses geolocation technology to allow customers to place orders ahead of time, and more local restaurants and chains are following suit.
Location Data “Cocktail” — Providing the “Smart” Customer Experience
A Blue Nile Research report from 2015 found that upwards of 70 percent of B2C and 76 percent B2B buyers use three or more research channels before making a purchase. This type of information, combined with location data, is a powerful cocktail in providing a smarter customer experience, from pre- to post-purchase. In the same Forbes article mentioned above, Jones identifies trending cross-channel attribution, which combines several machine learning and location-based tactics, to help retailers create a 360-degree view of the buyer’s journey. Jones gives some great examples as to how retailers and service providers can use this information, including United Airlines’ efforts at helping consumers navigate airports with beacon technology, an application that also boosts brand awareness for cooperating vendors in the airport ecosystem.
Satellite Imagery & Predictive Analytics for Location Scouting
Real-time satellite imaging and predictive analytics for tracking and identifying optimal retail and other locations has increased in the last decade. Start-up Orbital Insight, which counts Google and Bloomberg Beta as investors, has used years’ worth of commercially-available satellite imagery and deep learning techniques to quantify retail traffic patterns in the parking lots of over 90 retailers. This data can also be use to analyze “company-specific and macro-level events” on car traffic.
Location-based social networks, including user mobility and location popularity, is also used to determine optimal retail store placement. Studies using popularity-prediction algorithms have found that models blending user mobility information from check-ins with geographic location data of users performed better than those using only geographic location data. This information is valuable for pinpointing a next office location, studying land prices, and better understanding ongoing use of heavily-trafficked spaces over time.
Satellite Imagery & Situational Awareness for Market-Based Decision Making
Earlier this February, Planet Labs announced its agreement to buy Terra Bella, an advanced imaging satellite platform, from Google (which will thereafter purchase Earth-imaging data from Planet Labs). This purchase will add steam to Planet Labs’ current suite of services, which includes progress tracking, competitive intelligence, and situational awareness technologies applied to the finance and business intelligence sectors.
Planet Labs describes companies’ abilities to use real-time imaging data to make better-informed decisions to inform supply chain management and track progress based on “global situational awareness and same-day competitive intelligence.” Its satellite imaging technologies allow, for example, the monitoring of global crop yields, mine outputs, and port traffic—information that is of immense value to financial companies and markets forecasting agricultural crop yield and availability, for example.
Other Cutting-Edge Uses & Closing Thoughts
Increasingly sophisticated mobility data models allow urban planners and developers the ability to find solutions for problems that require analyzing placement data, such as pinpointing the best intersection for a particular brand of business, the ideal street for a bike-share location, or reconfiguring roadways for optimal traffic flow. “Visit-pattern-aware mobile advertising” may soon provide smartphone-toting shoppers with more relevant, behavioral- and location-driven mobile ads, furthering shaping consumer purchasing habits.
One of the most significant disruptions is likely to be felt in healthcare, where diverse sets of data — from current medical results, historical records, and streaming information from mobile- and wearable-devices — will allow machine learning applications to produce both intelligent personalized and generalized analyses and recommended actions.
Programs for individuals with specific illnesses or conditions will shift from being based solely on aggregate data to incorporating personal variables, everything from a person’s genetics to their behavioral patterns, including location-relevant behavior. Creo, a healthcare technology company, illustrates a number of cutting-edge healthcare applications that incorporate location data, such as Text2Move, a program that has been used in diabetes management and prevention, with text-based extensions for things like sunscreen use and smoking cessation.
Machine learning has opened windows and allowed researchers to view and analyze human movement patterns and satellite imagery like never before. In turn, the rising influx of location data from smartphones and IoT-connected wearables is helping drive the next generation of machine learning and location-data-based applications. From smarter planning in long-term business strategy and development, to more individualized and interactive B2c and B2B services, it seems likely that no industry will be left untouched by the incorporation of location data into machine learning applications over the next decade.
Image credit: Geographica