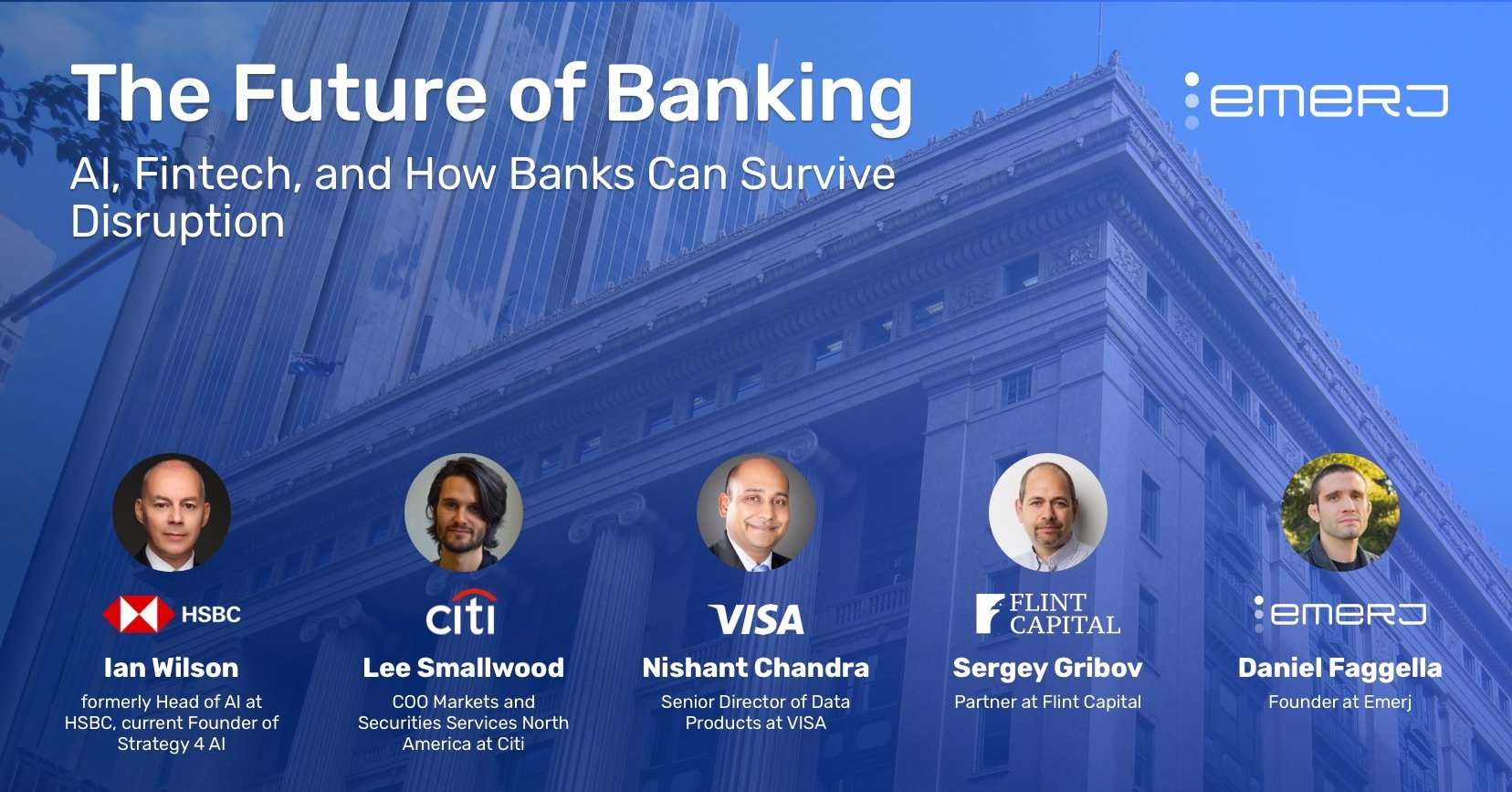
Artificial intelligence is transforming a variety of banking functions and allowing tech startups to compete with some of the largest banks for market share of key services, including lending and wealth management. Business news and media sites have been heralding the downfall of the banking industry as we know it because fintech companies are going to feel comfortable leveraging AI long before banks.
That said, the future of banking has yet to be decided, and banks still have many advantages over fintech companies they can leverage to survive in the next decade. We spoke with four experts on the state of AI in banking about how banks can use artificial intelligence and machine learning to both retain their market share and win new customers over their competitors, including millennials who are used to online banking.
In this article, we break down the key insights from the conversations we had with these four experts on our AI in Banking Podcast, including discussions about:
- Preparing for the Future of Banking
- Overcoming Challenges to AI Adoption in Banking
- How Banks Can Compete With Fintech Companies
Special thanks to our four interviewees, whose interviews are embedded throughout the article:
- Ian Wilson, former Head of Artificial Intelligence at HSBC
- Lee Smallwood, COO Markets and Securities Services North America at Citi
- Nishant Chandra, PhD, Senior Director of Data Products at VISA
- Sergey Gribov, Partner at Flint Capital
Wilson, Smallwood, and Chandra also all served as research advisors for our AI in Banking Vendor Scorecard and Capability Map report.
You can listen to our full playlist of episodes in our “Future of AI in Banking” playlist from the AI in Banking podcast. This article is based in large part on all five of these interviews:
Subscribe to the AI in Banking Podcast wherever you get your podcasts: iTunes, SoundCloud, Stitcher
We’ll begin our analysis of the future of banking with how banks should go about their all-important AI strategies, those that will steer how they invest in and deploy artificial intelligence to stay competitive.
Preparing for the Future of Banking
Recently, the enterprise AI conversation has begun including discussions about AI strategy. We do our own AI strategy work with large organizations. Although AI strategy should be on the minds of business leaders across industries, the way it’s currently framed makes it seem like a general, “one-size-fits-all” initiative that needs to be rolled out across a business at all levels. This, however, is not the best way of thinking about an AI strategy.
Wilson discusses the importance of the Chief AI Officer at a company, defining their role as “strategically defining what each business line should be looking at in terms of AI strategy and how to execute that.” He stresses: businesses don’t need an AI strategy. Businesses need multiple AI strategies for each functional division at their company. What works for customer service isn’t going to work for anti-money laundering. Although it sounds obvious, the general discussion on AI strategy is far from accepting this.
This is likely a symptom of the way business leaders think about AI today: As regular IT, as plug-and-play software. AI requires an overhaul of a company’s business processes, culture, and technology infrastructure. Each of these facets of a business varies greatly from department to department, business function to business function. As such, each of them requires a separate strategy to tackle the unique problems within them, problems AI may or may not solve now and may or may not solve in the coming decade.
Businesses also need to align their Ai strategy with their business strategy within each functional division. According to Wilson, “If you’re building out an AI strategy, it’s not an independent item.” Wilson gives the example of a commercial bank:
If our strategy is to recommend better products to existing customers and grow our customer base by finding customers that are already doing business with our existing customers, what capabilities can AI offer to enhance that strategy that were never available before?
This gets to one of Wilson’s core points about AI adoption in banking: “It’s about understanding the capabilities of the technology.”
This contrasts with the way banking leaders today are talking about AI in the C-suite. Instead of asking whether or not AI is right for solving their particular business problem, they’re asking where they can integrate AI into their business. Wilson provides further detail to this dynamic when he says:
The key question you’ll be hearing at the C-suite in a bank particularly is ‘What’s next? What comes next? Where is the ROI?’ And often there is no answer to that question. There is no ‘what’s next’ because there was nothing strategic put in place. It was just throw some mud at the wall and see what sticks.
Banking leaders largely believe just adopting AI is enough to achieve an ROI. This couldn’t be further from the case.
In the coming ten years, banking leaders need to learn about what’s possible with AI, what we call the “capability space,” if they’re to develop strategies that are likely to garner an ROI from their artificial intelligence initiatives. In doing so, they’ll be able to encounter a business problem within a functional division and have an idea of whether or not AI could help to solve that problem, and if so, how.
Wilson believes that this “knowledge” is one of two major factors that will allow banks to transition from thinking about how they can use AI to save money to thinking about how they can use AI to find new opportunities and win market share.
Right now, banks are in a phase in which they’re wary about experimenting too much with AI. During this phase, which we call “Emergence,” banking leaders don’t know what AI is capable of and don’t understand AI’s time to value.
The latter is one of the key challenges banks will need to overcome in the coming years if they want to compete, and we’ll discuss this later in the article. The former results in a general trepidation for experimenting with AI, and banks are now more inclined to leverage AI for automating processes they’re already doing.
For example, Wilson discusses how banks struggle to deal with all of their paper documents. Banks may want to look for NLP and machine vision software for digitizing paper documents and allowing for document search capabilities. Such an application could achieve impressive ROI, but it’s not going to drastically change a bank’s core workflows.
As banks become more comfortable with adopting AI and become used to developing AI strategies for a variety of functional divisions, they’ll start experimenting with AI applications that are going to allow them to compete with other banks and fintech companies, particularly customer service applications that far exceed the abilities of the chatbots banks are trying to implement today. We discuss the important role the customer experience will play in determining the future of banking later in the article.
Overcoming Challenges to AI Adoption in Banking
Our interviewees highlighted three core challenges to adopting AI in banking:
- It’s unclear whether or not AI will generate an ROI
- Banks and their leaders don’t foster a culture that allows data scientists to experiment with AI so that it does generate ROI
- Banks have legacy IT and data infrastructures that weren’t organized for using AI
Challenge: ROI Uncertainty
AI initiatives come with a lot of uncertainty, and banks are not used to experimenting to achieve ROI. Banks that want to survive in the coming years will need to cultivate a culture of innovation within their business, starting with their leadership teams. Wilson cites a “comfort factor” as one of the biggest hurdles banks need to overcome if they want to adopt AI and, ultimately, compete with fintech companies that aren’t cautious about implementing new technologies. Banks want to know that AI will work, but according to Wilson:
It’s difficult with machine learning to give very simple, clear answers, and that’s a big stumbling block right now.
Wilson believes banks will overcome this hurdle when vendors can start telling them their competitors are using particular AI applications. Banks won’t likely have much of a choice but to adopt AI in the next few years as their competitors do the same.
Challenge: Developing a Culture of Innovation in Banking
We surveyed bankers for our AI in Banking Vendor Landscape and Capability Map report and found that the number one challenge to adopting AI in banking is a bank’s lack of a culture of innovation. What this means is that banks are so risk-averse that they aren’t willing to invest in experimentation with new technologies, and this is required for successfully adopting AI.
In the next few years, banks will need to learn to accept the risk associated with AI if they intend to compete with other banks and fintech companies. They need to understand that, as previously discussed, an AI initiative may not ever generate an ROI let alone generate one in several years.
To do this, they should focus on both hiring data scientists in-house and educating their leadership teams on AI adoption best practices. Our interviewees mentioned the importance of leadership in cultivating the company culture required for successful AI implementation. Chandra, in particular, said the following:
Leaders make sure the stars get aligned. That is the core premise of a great leader. They become the guards who align the stars in the organization. What we have to do at [the leadership] level is basically find out the top 5 challenges and the top 5 things we can do to [overcome] those challenges…When you rate the top 5 on your left and top 5 on your right, you are basically matching columns.
What you’re trying to find out is what the things are that you can do in terms of execution that will achieve the maximum amount of outcome for you. The culture of everyone taking ownership and doing something like this in every level of an organization, that is the culture which will basically transform the companies. The companies are not transformed by the leaders, the company is transformed by the culture which leaders bring in.
Smallwood echoes this sentiment and emphasizes the importance of AI-focused talent at the leadership level:
The number one thing for me is talent. At more senior levels, it’s important as a demonstration that the company has invested in that direction and that there are champions for this technological adoption and usage.
Chandra also gives an example of how the leadership team can steer a company away from embracing the culture necessary for adopting AI. He gives an example of a company in which the technology infrastructure was ready and capable of handling an AI upgrade, but the leadership team would not pull the trigger on an initiative:
In this case when the technology was available but the leader was not, that’ the classic case of the leader getting distracted. An organization had to have spent a lot of money to be ready for transformation or…the inception of the organization happened in the digital domain…The leadership may fail to create value out of data: how do I monetize it? How do I leverage it toward barriers for entry for my competitors? How do I use this for a target advertisement for our product?
So the question becomes the leadership is sitting on this massive amount of data. which is the oil in the industry, and they don’t know how to use it or…fine tune [it] in terms of [answering] ‘why did I fail and where and how, and if I do it differently what would I do differently this time?’
This scenario harkens back to the importance of a coherent AI strategy for a particular business function. Leaders need to educate themselves on the capabilities of AI so they can craft these strategies and be able to answer the kinds of problem-solving questions necessary for working with their data science teams to iterate and experiment with AI. Only then will a culture of innovation start taking hold within an organization.
Challenge: Legacy IT Infrastructure
Chandra points at how the current approach to integrating new technologies, AI in particular, needs to change in the coming years for banks to remain competitive. He uses pizza as an analogy for the state of IT infrastructure at banks, suggesting that AI and data science capabilities are thrown onto a bank’s core IT infrastructure as “toppings.” As a result, with enough toppings, existing IT infrastructures overload and can’t handle the weight of what’s been thrown on top of them.
He suggests transitioning to what he refers to as “lasagna,” an IT infrastructure that is embedded with layers of AI and data science-related capabilities. One way of going about this could be embracing open source technologies.
Banks are used to using licensed software from software vendors, but open-source software is becoming more ubiquitous, and it may save banks on cost and on the valuable time they could be spending catching up with their competitors. According to Nishant Chandra:
Now [banks] realize that working on legacy domains limits their capabilities in various ways. It limits their capabilities in terms of using the power of their big data. It limits…the speed at which they can respond. It also ties them to certain organizations and their release portfolios. A company’s growth is limited by how fast new features can be released by their vendors.
Chandra points out how open source technologies are in many cases releasing faster than licensed software, and they also tend to be more agile and flexible. Transitioning to open source software may allow banks to respond to their competitors’ newfound capabilities and technological advancements, as well as cybersecurity threats, sooner. According to Chandra, “some of the early threats in terms of security are taken care of in the open-source domain.”
That said, banks face serious challenges when transitioning from their legacy licensed software to open source software. In many cases, this transition relates to a cultural challenge at the bank, as discussed previously. Leadership teams are often reluctant to invest in workflow overhauls that may not result in any ROI or cost savings for a very long time. Chandra illuminates this point when he says:
The potential payoffs would take up to 5 years if not a decade. You don’t want to be so much behind that you realize you absolutely cannot catch up. Its an investment but its an investment that would be continued, meaning new open source software will continue to come up
Large, risk-averse institutions such as banks generally don’t’ want to take on costly IT infrastructure projects that both won’t garner an ROI for years to come and require continued investment in upskilling and technology upgrades. Unfortunately, that’s exactly what AI and machine learning are, and banks need to understand this if they intend to survive in the next ten years.
How Banks Can Compete with Fintech Companies
AI is in large part the reason fintech companies get to compete with banks for market share of key banking functions. Banks are no longer the only place consumers can go to receive banking services. Numerous tech startups are offering financing options and money transfer services to customers entirely over the internet. Gribov believes:
We have a lot of things to be afraid of because a lot of the things banks are doing can now be done without any bank. You can send money with messaging apps.
This poses a threat to banks that have historically relied on face-to-face interactions with customers at local branches, and this threat will grow in such a way that it will force banks to not only adopt AI but to become leaner in the next decade.
Customer Experience May be a Losing Battle
Banks may not be able to compete with Fintech companies in the customer experience domain. Fintech companies may be able to offer better customer experiences to younger customers used to digital banking and digital communications. According to Gribov, fintech companies built on AI algorithms can:
…do a lot of stuff on different segments in banking. If you’re thinking about customer-facing stuff, you can do obviously, right now you have agents talking to customers, but AI can do a way better job in doing this interfacing kind of work because the AI machine will know not just the number of customers that the agent talked to, it will have all of the information of every single customer the bank talked to. It will know what kind of transactions the customer did five years ago, ten years ago, what kind of stuff they’re interested in on their facebook page, etc. AI can provide way better service than a human ever could.
That said, Smallwood doesn’t think the future of banking is a “millennial game.” He believes the customer experience is “what wins:”
Customer experience is really what wins regardless of the age or profile of who that consumer is. With some of the examples we’re talking about, student loans is going to skew younger and maybe people who are more digitally native. Yes, in some aspects of finance, that may be a factor. But in other parts, maybe asset management, I don’t think it’s millennial-driven, but its customer experience-driven. I think it’s good because it drives innovation [at banks]…because…they’re putting more focus on what the customer experience looks like regardless of what that customer profile looks like.
Banks are only now trying to deploy AI-based chatbots, but our interviewees believe that banks will focus on automating compliance before they focus on improving the customer experience using AI. Banks take on greater risk than small fintech startups; as such, compliance is a much greater concern for banks right now, probably as it should be.
What this means, however, is that fintech companies will be able to divert more resources to optimizing their customer experience because they offer less services. They’re leaner; they offer one or two services and can master them early, allowing them to divert the AI talent they often deploy to customer service applications.
Banks likely won’t do this for several years, well after they’ve adopted AI applications for risk-related functions such as fraud, cybersecurity, and compliance.
Banks Can Leverage Existing Assets to Win
Banks still, however, have several advantages over fintech companies they can leverage to remain competitive. There are
some banking functions that are likely to be left to full-fledged banks in the coming years because fintech companies simply can’t compete with banks when it comes to their access to resources and data. Smallwood gives an example:
If you think about asset management, pension funds—getting access to these people is phenomenally difficult for startups. If you’re a company that already has good access to these people, you can get a one-on-one conversation pretty easily.
Gribov echoes this sentiment with his own example:
There is functionality that banks provide that you need to be a bank to provide…[such as] the maturity of money. If you have money which you put in a bank for a long period of time, it’s there for a long period of time, and you can take loans. [Banks] are able to give you these loans using money that people are putting in for months. Because the banks are so big, they can provide this functionality, and someone has to provide it. It will be very hard for fintech companies to provide this unless they become a bank.
In order to survive in the coming decade, banks need to understand what kinds of data they have access to that fintech companies don’t and what kinds of problems they can solve for customers that fintech companies can’t because they don’t have access to that data.
Banks can leverage their large existing customer bases as low-hanging fruit for thinking about which products and services to offer and which they may need to cut back on in order to conserve resources and match fintech companies in how lean they are. This will be one of the only ways banks will be able to compete with fintech companies that are digitally native and are able to provide customers better, more convenient experiences.
Banks Can Learn From the Startup Ecosystem (and Acquire AI Firms)
Smallwood suggests banking leaders learn about what’s going on in the startup ecosystem for two reasons:
- Generating ideas about what AI capabilities are available
- Scouting potential acquisition targets
Many fintech companies have the advantage of digitally-native AI talent on their leadership teams, but they don’t have the funds nor the lure of stability to onboard more talent quickly. Banks, on the other hand, can buy entire companies, onboarding their AI talent and the products they’ve built in the process.
In doing so, they can iterate on the startup’s algorithms with the piece of mind that their competitors won’t be able to do business with them anymore. In effect, they can buy their competitor’s technology options. They may also be able to beat out less developed fintech startups that might pose a threat to them in a few years if they were able to win market share.
By building their own AI in-house, they can race alongside these fintech startups on the back of the talent of their acquisition target.
That all said, it will be very difficult for banks to retain the talent they acquire past their initial contract periods if they do not first cultivate that culture of innovation throughout their business. AI talent is almost by default more likely to pursue careers at large tech firms known for AI (Google, Amazon, Facebook) because everyone at those companies know how to speak the “language of data science” and respect how important they are to the future of the business.
A data scientist is likely to leave a bank even if the bank offers them more money than they’d get working at Google if the bank fails to put that data scientist to work on valuable projects that matter to the business and fails to surround them with subject-matter experts that can facilitate their work.