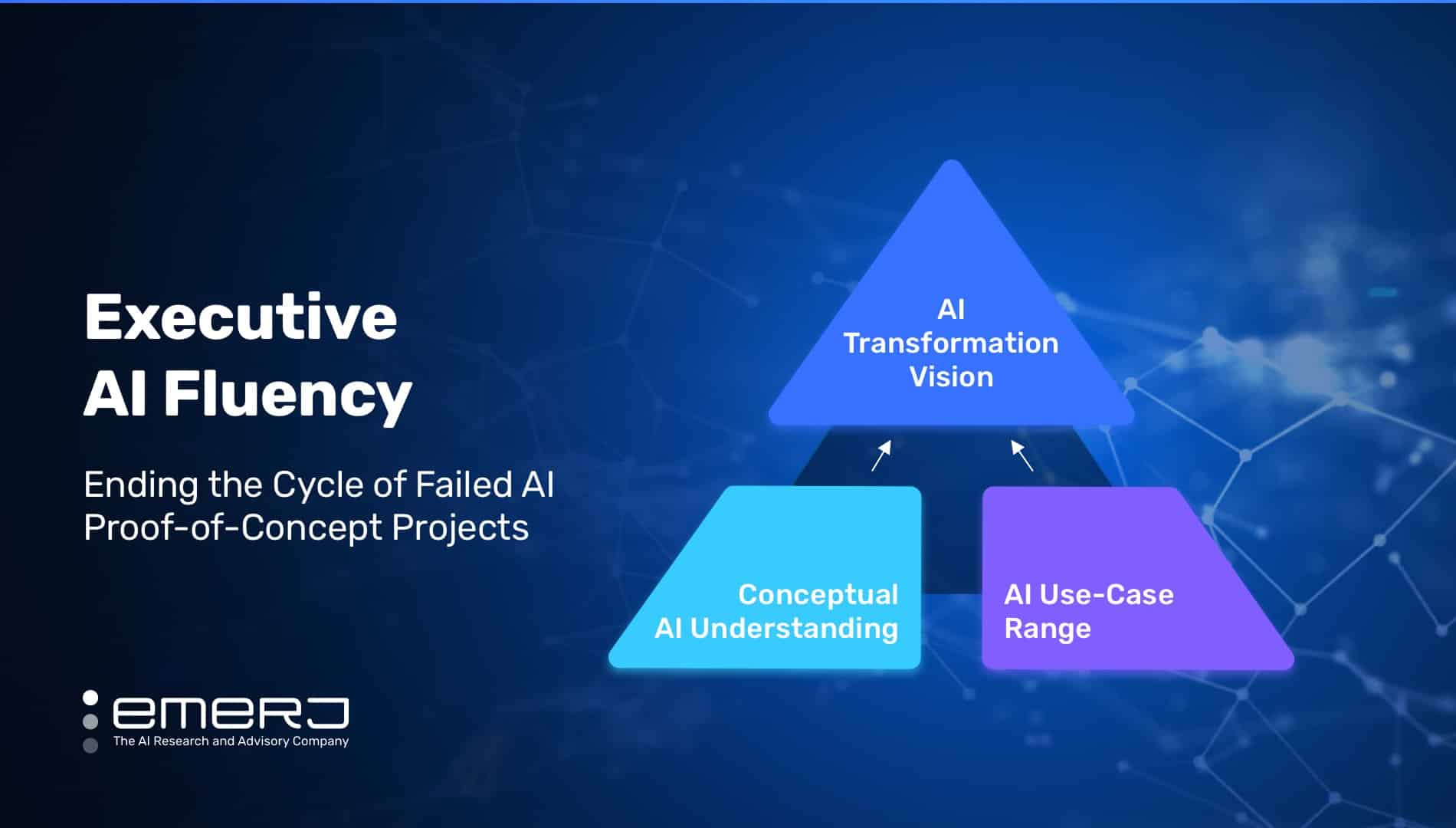
Despite the billions of dollars being poured into AI startups and applications – it is common knowledge that most enterprise AI initiatives fail.
While we could write an entire report about the reason that AI enterprise projects fail (Getting Started with AI is a good start), it’s important to note that most failures have the same origin:
Executive leadership doesn’t understand AI.
We could blame vendors for overselling their vision – but savvy executives would have known that their promises were unrealistic (and they would have asked for case studies with other clients).
We could blame the project’s failure on the disjointed state of our data infrastructure – but savvy executives would have done a small data audit to determine the feasibility of the project ahead of time.
We could blame the failure on team members who couldn’t work together across silos – but savvy executives would have built a dedicated project team committed (and accountable for) such collaboration.
Notice this has nothing to do with writing code. This has nothing to do with linear algebra. It has to do with contextually understanding what AI can do, and what the AI deployment roadmap looks like in practice.
The majority of our Emerj Plus members are AI strategists and CEOs of AI and IT services companies, and they all struggle with finding executives who “get it.” The AI vendor companies we interview deal with the same problem. Some executives think AI will work like magic – and most of them have stunted and limited ideas of what the technology is capable of.
The Bad News: In the aftermath of the COVID-19 pandemic, enterprises will be more afraid than ever to run AI pilot projects, and to risk wasting money on technology they don’t understand. Sales will not be getting easier for most AI service providers.
The Good News: The AI service providers who can educate their buyer and help to mold a realistic idea of what AI can do, and how it can be deployed sustainably, will build trust and win deals in a critical era when automation and AI have the ability to add tremendous value to enterprises (or even save them from bankruptcy).
We can count on executives to “get it,” we have to make them “get it” as part of the strategic planning process or sales process – and that’s the focus of this article.
Below, we’ll explore a concept we call “Executive AI Fluency.” If you work within an enterprise, or sell to enterprises, and you need to make the business case for AI – this article will help you succeed with AI pilot projects, while most firms simply waste money spinning their wheels.
Three Components to Executive AI Fluency
Problems with AI initiatives often start at the top.
The executives that agree to fund these projects are often under the impression that AI is like IT. In IT, we can define our goal, define the functionality we’re looking to build, and scope the time it will take to achieve it. In AI, much more iteration and exploration are required, and time-to-ROI isn’t nearly as predictable. The upside is massive transformative potential, the downside is that most executives can’t stomach this new “R&D-like” investment – especially when they expect it to be an “IT-like” investment.
Halfway into a project, the leadership – unprepared for massive investment in data cleaning or the need for new data science talent – gets flustered, demands unrealistic deadlines or ROI goals, and then cancels the project. Millions of dollars are wasted because of this mismatch in expectations – and this lack of AI fluency.
When we can help executives to understand how AI works and how it is deployed, the benefits include:
- Better Project Selection: When we educate executives to understand AI, they’re more likely to put resources behind the projects likely to win (rather than the projects that their competitors are pushing press releases about, or whichever vendor had the nicest slide deck).
- Realistic Expectations: When we educate executives to understand AI, they’ll realize the initial hurdles to AI deployment, and they’ll agree to near-term and long-term measures of success that the team can actually achieve.
- Well-Resourced Projects: When we educate executives on how AI works, they’ll put the appropriate team members, funds, and IT resources behind a project to make it succeed – instead of relegating AI project teams to an isolated sandbox where they have no context to learn.
These factors are often the difference between success and failure of an AI project – and early projects are often the difference between positive or negative momentum for AI adoption. Nothing kills future investments like seeing three proofs-of-concept fall flat. That means internal innovation leaders don’t get to drive their transformation vision forward, and AI vendors and service providers lose future revenue (or worse, come out with a black mark on their reputation).
Executive AI Fluency is what needs to be developed – and it’s made up of three components:
How AI Works:
Key Questions:
• What are the team, data, and resource requirements for AI projects?
• What does a typical enterprise AI deployment roadmap look like?
How AI enterprise solutions work, which expectations are realistic, and which are not.
Enterprises can benefit from this because they will be less likely to fall for hype – and more likely to realistically consider AI’s near-term and long-term impact on the company. This makes executives more likely to understand the fundamental changes that real AI deployments will take, the need for better data infrastructure and cross-functional teams, and other factors we consider Critical Capabilities for deploying AI software.
AI Use-Case Range:
Key Questions:
• What are the most important AI use-cases at work in my industry today?
• How is the data used – and how is success measured – for these applications?
Executives cannot understand AI in a purely conceptual fashion. They need practical use-cases for the types of AI projects they are brainstorming – and it is even better (at least initially) to have examples within their industry or related industries.
One example of a strong AI use-case in banking is fraud detection. Some banks and AI vendors report to have lowered their rate of false-positive results for financial fraud using predictive analytics solutions. A wide range of use cases allows leadership to better detect where AI opportunities might lie within the company and decide which projects deserve the most attention of the many that could be applied.
Banking leaders should be able to expect a chatbot solution to provide their customers basic answers to common and simple questions. Bank leadership should not expect their chatbot to be able to handle complex conversations, or draw upon rich context from previous email or phone conversations with the client. The technology is simply not at that level today.
In this way working with AI is more strategic than the “plug and play” nature of IT solutions. There are more opportunities to think about the long term ROI of a given project, and that ROI can be measured in education and growth in addition to financial gain. Errors at this stage are the cause of millions of wasted dollars on AI projects that were doomed to fail from the beginning.
AI Transformation Vision:
Key Questions:
• How does AI align with our 3-5 year goals?
• How might AI unlock new business models – or new ways to deliver value for our customers?
AI transformation is a thorough understanding of how a company can leverage its strengths and position in the market alongside new AI capabilities in order to unlock new ways of serving its customers or methods of serving new markets. Once a business leader is familiar with how AI works and a good range of use-cases for their industry, they can begin to build an AI transformation vision for their company.
An executive that has taken the time to educate themselves on this will be able to consider their long term goals alongside their use-cases to find ways to differentiate their company in the future. Only then can they be most effective at making AI-related decisions responsibly. Without a good base of knowledge about the first two factors, it is impossible to build an AI transformation vision. An executive team is nearly destined to make rash or ill-informed decisions about the value of AI if they do not have a clear AI transformation vision.
Getting to Fluency
The process of getting to AI fluency will involve many departments including media and market research, vendors and service providers, and executive teams themselves. For the sake of this article, we will discuss the role AI leadership plays in this process as well as AI vendors and advisers. These are the two parties that will be working closely together most often to bring the company to a higher level of AI fluency and so we will explore them in depth.
The Role of Enterprise Leadership
Executives and AI leadership within the enterprise would do well to attend events, keep up with reliable resources, and speak with their peers as often as possible.
Our AI strategy reports are distilled from dozens of interviews with top execs and AI innovators and are a great place for our readers to start. It is imperative to remember that although AI is not rocket science, it is rather complicated. An executive would be incapable of allocating money responsibly toward AI innovation without understanding it’s broad opportunities and clear challenges in the enterprise. Instead, they might continue to use AI similarly to IT projects and move forward with a narrow view of the purpose of AI which will harm their long-term strategies.
Perhaps most important is an executive’s ability to consult with their peers who have attempted AI projects in the past and learned important lessons to start building a knowledge base. AI is still nascent in many industries, and so most executives will be at a beginner level. However, the ability to share knowledge with each other allows each company to move toward AI fluency.
In addition, leadership will need to factor AI into their long-term strategic planning once they have a firm understanding of how AI works with a list of representative AI use cases. We find that the process of strategic planning and thinking about where AI fits in the long term provides motivation for executives to get a thorough understanding of how the software works in their industry. This helps them determine the best use-cases to try and use within the enterprise.
AI is just another trend to learn for executives that do not have a firm understanding at the heart of their strategic planning to use as a guiding key. That said, executives have much more motivation to learn when they can tie what they learn to a compelling future vision (rather than a plug-and-play IT-like solution).
The Role of AI Vendors and Advisors
Enterprises have the luxury of being able to choose not to innovate or digitally transform and still pay the bills provided there are no major disruptions.
Their executives are more concerned with making a safe decision for the company than moving digital transformation forward in the enterprise. AI vendors do not have such a luxury, and so they need to convince companies to innovate using their products.
While this may lead some unethical vendors to overpromise and underdeliver, it also forces ethical and informed vendors and AI advisors to become educators in their space. These people bring the fundamental information about AI to the C-suite, including the transformative potential of the technology and the challenges and prerequisites for deployment.
The Transformative Potential of AI: This involves teaching the C-suite an understanding of AI by demonstrating use cases, laying out known instances of ROI, and understanding how that ROI is measured. This information is hopefully based in truth from a vendor that wants to see genuine success with their product within their client’s business. The ability to flesh out this transformative potential with observable use-cases is the first step for teaching executives what they need to know, and much of this information can be found in our AI Opportunity Landscape for banking and financial services.
The Challenges and Requirements for AI Deployment: Initial AI deployment asks a lot of companies that are just starting out including an overhaul of their data infrastructure and development team structure, building a culture that values data, and AI fluency in leadership. This puts leaders in AI services in a challenging position because they need to address the realities of AI adoption if they expect to make promises about their products, and some executives are unprepared or unwilling to digest that difficult kind of information right now.
That said, there is an opportunity for leaders in professional services with strong business use-cases and a strong understanding of the AI transformation journey. This is because they will be able to differentiate themselves from vendors and consultants who are armed only with buzzwords – or compelling “plug-and-play” claims that inevitably fall flat on delivery. Successful vendors have the potential to make long-term partnerships with their clients as opposed to delivering a series of ill-fated projects that likely never had much hope of success from the beginning.
An AI vendor that knows what they are talking about will see their role as helping to educate decision-makers in the enterprise. Long term success and funding for their AI product will genuinely depend on the enthusiasm and understanding of the buying stakeholder. Making a case to that stakeholder will be the difference between winning and losing vendors during this time, and we hope that the right vendors are the ones that help to bring enterprise clients into a position of greater AI fluency.
Emerj for AI Services Leaders
CEOs of IT and AI services companies join Emerj Plus in order to access our full repertoire of AI use-cases, best practices, and ROI insights for the enterprise.