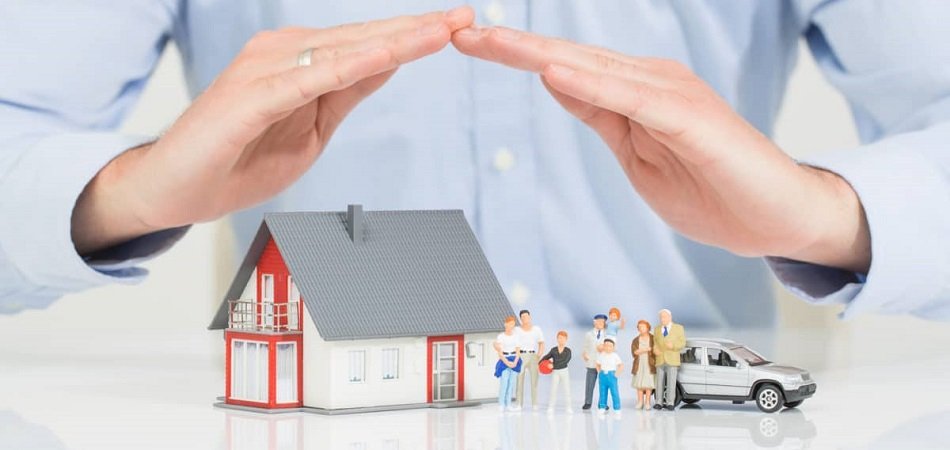
Even during a time when the buzz around AI and digital data storage is prominent, there are still some companies with large amounts of backlogged data that are taking up space in their databases, but not providing enough value. Large stores of enterprise and customer data can be valuable to insurance companies for optimizing their business operations and gaining analytical insights on how their business decisions affect company growth.
These monolithic databases cataloging nearly every insurance policy or claim issued or processed by the company can be instrumental in training machine learning models. In this report, we’ll investigate how a company might begin to sort and structure their big data and then put it to use with AI applications that can facilitate many insurance departments.
We will start our report with the possibilities present in data centralization, or the structuring of vast databases of documents and customer information to be made useful for solving business problems. Then we will investigate how AI applications can help insurers facilitate the following business processes:
- Claims Processing
- Fraud Detection
- Customer Engagement
- Determining Customer Lifetime Value
Our investigation starts at data centralization and how an insurer might approach formatting their big data into usable information”
Data Centralization
Big data often comes from large CRMs or other data storage options, such as databases for insurance policies and claims. Insurers may also have access to large amounts of unstructured data, or data formatted in a way that is impossible for a machine learning model to process as is.
This could include images of damaged cars associated with insurance claims or IoT sensor data from a mobile app or separate telematics devices. Another form of unstructured data that a company may not keep a database, but nonetheless has access to is social media data regarding customers’ interactions with advertisements.
There are numerous AI software vendors offering data integration services to centralize certain categories of that data for it to be used for business intelligence or AI applications. Companies selling into insurance typically also offer software that allows companies to interact with this data in a user-friend and productive way. This could be in the form of a user dashboard that allows insurance agents to find the information they need with relative ease.
Additionally, vendors offering data centralization solutions may also offer AI-driven data analytics software to solve various business problems. In insurance, the most common problems these vendors claim to solve are automatic quoting, claims filing automation, and fraud detection.
GrayMatter is one such vendor that offers data integration services along with various user interfaces clients may choose from to suit their needs. In addition, they also offer business intelligence analytics solutions to insurance companies and other industries. It is important to note that not all AI vendors offering AI application software will also offer data centralization solutions. That said, most vendors that do offer data centralization typically also offer software for other AI applications.
“The business management dashboard developed by GrayMatter is a powerful performance management tool empowering the user with business-critical information for sales management and business planning across the distribution channels,” said Teh Kim Leng, CTO of AIA Bhd, the largest publicly-listed life insurer in Asia. Here we can infer that he is referring to a GrayMatter dashboard interface that his company used to leverage their big data to track sales and plan business operations.
According to a case study conducted by GrayMatter, their data integration services were able to help another insurance software provider offer analytics services to their clients. Software developers at Sircon worked with GrayMatter to create a customized AI-based business intelligence application for data analytics.
The software leveraged architecture which allowed multiple users across an organization to use the software, accessing data from all business sectors at each user’s convenience.
While separate organizations did not need their data centralized with their fellow Sircon clients, the application was able to access that data concurrently. Each client would have then been able to access more data from this centralized location, allowing them to better train future machine learning models for their own purposes.
Below is an image depicting the typical flow of data within GrayMatter’s Big Data structuring approach. From left to right, data from various sources is acquired by GrayMatter’s various processing tools, then moved into management infrastructure which could categorize data points to ready them for use in AI software. Finally, the data is ready to be used in various AI and analytics solutions, notably predictive analytics.
These types of data integration services are for companies who are in need of data centralization in order to have large stores of data ready for whatever type of AI software they want to use. In order to train a machine learning model for maximum effectiveness, a business needs as much data as possible that is related to their desired function. Having a large aggregate of data in one place is typically ideal for this.
Through the next two capabilities covered in this report, we’ll look at the possibilities AI software can offer insurers once they have centralized their big data.
Claims Processing
In claims processing, companies can use predictive analytics software to determine the amount to be paid out to repair damage to a car or a house. In addition, this type of software can calculate changes to a customer’s premium payments or future deductibles as a result of a given incident or claim.
This information is typically presented to the insurance agent on a dashboard for either their desktop or web browser and can either automate the process entirely or serve as an assistant tool for suggestions. Most often, human insurance agents will still need to sign off on any conclusions made by the software.
Fraud Detection
Insurance fraud detection can be accomplished with multiple AI approaches, including anomaly detection and predictive analytics. Anomaly detection software is built around a machine learning model installed within a client’s claims processing system and allowed to detect new claims coming into the system to establish what a “normal” claim looks like. The software is then able to detect aspects of claims that deviate from that established measurement of “normal” and label them as anomalies.
As the software is exposed to more deviations and anomalies within each claim, those anomalies will affect what the machine learning model recognizes as “normal.” This way, the machine learning model begins to accept reasonable deviations from a standard insurance claim to avoid false positives. Although the system still flags any potentially fraudulent data points for a human employee to review.
Predictive analytics operates differently from anomaly detection when used for insurance fraud detection. This is because the way machine learning models are trained to work between the two applications comes from entirely different approaches. Data scientists will need to label a large volume of insurance claims as legitimate in order to establish what a normal claim looks like for the machine learning model.
They will also need to label a large volume of claims as fraudulent, to signal the machine learning model that there are fraudulent data points within the claims that it needs to find. As the software processes these labeled claims, it will be able to discern which data points correlate to a fraudulent or legitimate claim.
Most insurers will find that they already have historical claims data labeled as fraudulent or not, but if any of the claims are documented physically the company may require an AI solution to digitize them. This can be handled with machine vision software capable of optical character recognition (OCR) to detect the chains of text on a printed document and replicate them in a digital database.
Friss, a predictive analytics software provider selling into insurance, published a customer success story in which they claim they helped Anadolu Sigorta transform their fraud detection process. Anadolu Sigorta claims they had 25,000 to 30,000 claim files to check for fraud every month. Before choosing Friss, the company handled all fraud detection manually with a team of 50 people following procedures based on loose rules and individual employee experience.
Below is a video showing Faith Gören, Deputy Chief Officer of Anadolu Sigorta, describe his company’s experience with choosing Friss and how they benefited from leveraging their data with an AI fraud detection solution:
Customer Engagement
Another possibility of AI in insurance made possible with big data is in customer engagement. Companies can use predictive analytics to create models of “what-if” marketing scenarios to determine the best course of action for their new marketing campaigns and promotions. This allows them to access customer profiles and compare them with past marketing campaigns to see which demographics respond the best to which types of marketing.
While some conclusions may seem obvious, such as millennial customers being more receptive to mobile insurance apps, more specific insights can be found. This could include the reasons why individual customers from older generations have trouble with the mobile app, which allows the insurer to look for ways to make the app more user-friendly. It is this ability to find the source of a business problem with enough detail to strategize a solution that can make big data analytics powerful for retaining a good relationship with customers.
In our example of the mobile app, an insurer could give their customer service department special instructions on how to help customers use the mobile app, with emphasis on the most common issues customers are having. This way, the company can be making sure their customers have support while waiting for an update to the mobile app that will resolve the issue entirely.
Determining Customer Lifetime Value
Big data could also play an important role in predicting customer lifetime value (CLV), the measurement of how long a customer will stay insured with the same company and how much revenue they will bring throughout their lifetime. This determination needs to be made based on a large amount of historical data from the customer’s profile or customer profiles of people of similar demographics.
An insurer with structured big data, however, could gain an even more detailed look at the potential revenue projected from one customer’s lifespan. This could take into account their credit score, past insurance policies, geolocational data, and statistical averages of customer lifetime value based on the company’s business experience with customer behavior. For insurance, this can allow companies to facilitate their customers’ experience while tracking their account activity for patterns that correlate to how much value a customer might offer over time.
Pegasystems is a software vendor that claims to help insurers improve their customer experience with AI powered decision assistance tools. These tools purportedly give suggestions to insurance agents on when they should try to upsell or when it may be time to give the customer time to think. The software may even give an on screen prompt for what to type back to a potential customer in either an email or chat client, such as suggesting to tell the customer “thank you.”
According to one of Pegasystems’ case studies, they helped CSAA Insurance rebuild their customer engagement process with their insurance agents, which changed it from an entirely transactional experience to one that focused on customer satisfaction and engaging them with their product. Because the client company had over 2 million AAA members to service, they needed to increase loyalty within their customer base by improving the customer experience of interacting with AAA agents.
By working with Pegasystems, CSAA was able to bring in data from numerous administration systems for insurance policies and create a “single view” for each customer. Using this administrative data in conjunction with the customer’s historical data, CSAA could track each customer interaction to monitor satisfaction. The case study states that CSAA improved performance across their net promoter team by giving them access to detailed context on the customers before they begin speaking with them.
“We weren’t able to acknowledge how long a customer had been an AAA member, and now we can. We are leveraging information more effectively so we can deliver that perfect customer experience,” said Steve Rowbury, the Business Owner of CSAA Insurance Group. He claims his company was able to define a perfect customer experience with CSAA, and achieve that with all of their customers using Pegasystems’ solution.
Pegasystems claims CSAA exceeded their initial goals in about six months, but they do not offer statistical results showing a numerical increase in customer lifetime value.
Below is a video explaining how Pegasystems’ software can give an employee a detailed view of how a customer is navigating the internet for information about a product in question. In the video, this product is a promotional credit card from the customer’s bank. However, this could also be used if a customer was searching for a temporary insurance policy such as one for a vacation:
Customer Lifetime Value is an attribute that is subject to change from customer to customer and does not account for emergent problems or changes to a customer’s lifestyle. Insurers may need to update their customer profiles and records based on any new information they find, as this will help their software’s machine learning algorithm factor in the abnormal occurrence.
If a customer recently changed career paths and saw an unprecedented increase in income, it would be useful to create a data point in their customer profile regarding that. The customer may be able to afford a better insurance policy or pose the risk of switching to another insurer.
Header Image Credit: Herndon Carr & Co