High-frequency retail (HFR) segments, such as groceries and drug stores, have ongoing challenges with profit margin – with grocery margins nearing 1% on average, according to an analyst from market research firm IBISWorld.
With inherent challenges to margin and constant encroachment from eCommerce giants like Amazon, retailers need to find any edge they can by optimizing their critical process (i.e. inventory management and pricing) in order to maximize profits. Artificial intelligence may be able to help with some of the previously intractable problems in the sector.
Daisy Intelligence is one such artificial intelligence vendor. The Toronto-based company focuses on retail merchandise planning: promotion, pricing and demand forecasting optimization. We spoke with Gary Saarenvirta, CEO of Daisy Intelligence with the intention of finding answers to the following questions:
- Why might grocery vendors or supermarkets need AI?
- How can retailers leverage AI for optimizing merchandising decision making?
In the sections below we highlight Gary’s insights, and we also take a closer look at three Daisy Intelligence case studies, examining the focus and impact of artificial intelligence on price optimization and inventory planning.
Why Grocery Vendors or Hypermarkets Might Need AI
Traditionally, vendors in the grocery retail sector have focused their promotional efforts around a particular localized event or holiday – and typically these promotions are repeated annually (often without significant changes to the promotion). Retailers often lack the complete picture when it comes to understanding how effective promotional campaigns are, or if the promotion of certain products might only be ultimately reducing overall profits by causing sales of related category products to drop.
Gary explains that retailers aren’t often able to see beyond individual product sales and margins when it comes to inventory planning. By way of example, he went on to say most retailers might follow certain traditional marketing practices:
“Whether promoting chicken wings on Super Bowl weekend, or promoting turkey at Thanksgiving, their promotional activities seem to revolve around a dozen main products and these seem to be done with very little insight beyond unit movement numbers of the item, and this is done repeatedly, In most cases, year over year.”
Apart from the lower margins forcing a push for greater efficiency in operations, Gary believes that retailers need to take a closer look at how effective their promotional campaigns actually are. Retailers looking to improve these margins through promotions and marketing campaigns need to be aware of the following challenges:
- Identifying products that have many consumer uses and might quickly lead to improved cart value or improved overall margins. For example:
- A consumer buying product like ground beef (which cannot be consumed by itself) might also attract sales for hamburger buns or pasta and tomato sauce. On the other hand, a product like a water bottle might not have many use-cases of being combined with other purchases.
- The challenge here for retailers lies in identifying the products with multiple use-cases which appeal to many customers. Once these products have been identified, the next steps of promoting them, and simulating sales forecast and pricing are also enabled through AI.
- Keeping track of ‘displacement halos’ for products to ensure that the retailers are not just moving their business from one category to another. For example:
- A promotion for ground beef by a retailer might potentially aid sales of hamburger buns, but might also cause decreased sales for hot-dog buns thus displacing their sales rather than gaining a net-positive effect.
Here is where the promise of artificial intelligence lies — by digging deeper than individual product sales and determining the full bottom-line impact of promotions and inventory choices. This is a complex process that involves determining the ripple effects of inventory and pricing choices – not only within the category in question – but across other categories store-wide. Machine learning excels in finding patterns and correlations within data, and that’s exactly the kind of capability that this task requires.
We list out a few use-cases below where AI might help retailers to:
- Find patterns in years of customer transactions about a specific product (such as one gallon of whole milk), and determine the impact of various promotions and sales for that product on other products in the store. For example:
- Are juice sales impacted slightly when milk his heavily discounted?
- Are milk-related products (such as cereals or baking ingredients) impacted by promotional efforts to sell more milk?
- Determine possible promotional ideas that correlate to higher margins store-wide.
Certainly, human intuition and knowledge of products and brands are important. However it’s humanly impossible to measure all the ripple effects of every possible decision. AI does that:
- Determine which products to select as possible levers for improving margin:
- Help provide context for the findings of the machine. For example:
- Maybe the massive sales in sunblock over a two-year period is due to a prominent in-store placement, as opposed to the success of a sales campaign or flier
- Maybe the decreased sales in canned goods in a specific year were due in part to construction happening in part of the building which left certain parts of the store inaccessible to customers
- An algorithm looking at sales and promotion data would have no idea about these other factors of store placement and construction issues, and human context would be necessary.
According to Gary, retailers cannot afford to promote campaigns without the complete view as they’re not as effective cannibalizing one category of products to another without supporting overall profitability. He adds:
“This is especially true since market pressure from large retailers like Amazon and Walmart is driving down prices and the ‘1% (average) net margin grocery industry’ is going to struggle to find profits unless they can find a way to become more efficient.”
How AI Can Help Retailers Optimize Inventory Operations and Pricing?
It is now possible for retailers to tackle their challenges by using AI, however they need to be well aware of what kinds of data may be required to help an algorithm “learn” to eventually recommend the most profitable pricing or promotional strategies.
Gary informs us that many retailers have an IT data warehouse where promotional and transactional data is stored, and technology vendors can gain access to the data through a one-time historical transfer. For applications like Daisy that also allow for real-time data feed, it is often possible for data to be sent daily in order to continue training and informing the algorithm.
The Use of Data
According to Gary, the data used to train Daisy’s AI could come from numerous sources including:
- Transactional data
- This includes two to five (sometimes up to 10) year historical transaction logs from retailers which include details of every single product purchased from multiple sales channels like stores in every or websites.
- Manufacturer cost data
- In order to arrive at the most ‘profitable’ pricing for different products, retail records about purchases from the manufacturers is intuitively critical. However, Daisy’s A.I. doesn’t recommend prices on products in isolation. Everything is optimized on a blended margin total basket to achieve the long term corporate goal of increased profitability
- Data about past promotions and marketing campaigns
- Gary says that from Daisy’s past experience with retail clients, promotional data is usually harder to track thoroughly and might have some inaccurate or missing data (like campaigns that were run in a single store or or last minute changes to promotions that were not recorded). He goes on to say that on average large retailers have 10 years of historical promotional data (which is 80% accurate) and smaller retailers might have records of the major promotions that were run, grocery fliers and circulars.
- Gary also says that it is still possible to infer and correct the operational intent even if promotional data is not as well tracked. For example, If there is a price drop noticed in one week for milk cartons from the historical data and corresponding quantity of milk cartons increase. In Gary’s words:
“We classify things that we believe are promotions even though promotional data doesn’t match this. We can infer placement and promotional elasticity. We correct transactions. If the store put a wrong price on Monday 1.99 on monday and sold 100. And Tuesday they put 2.99 and they sell 1000 units for the week. Pricing elasticity would say if I increase the price I’ll make more sales. But we go in we know the price should have been 2.99 on Monday and go fix these operational errors”
- Other market factors
- For brick and mortar retail stores, additional information such as the geographic location of the store, the location of competitor stores etc. might also be tracked by the AI model to improve the accuracy of predictions for potential new store locations.
- If retailers offer a loyalty program, they might also have access to customer data such as the locations of where many of them reside or what the average household size and income are; all of which adds more ‘degrees of freedom’ to the predictive model to help get a more accurate result towards personaliztion.
AI in Action of Retail Pricing – How it Works
To explain how an AI platform like Daisy might make use of the different types of data available from the retailers, Gary draws an analogy to Google DeepMind’s AlphaGo, the first computer program to defeat human world champion at the ancient asian board game Go:
- AlphaGo was designed under a very defined set of rules to play the game. Gary believes that there are a similar set of rules for the retail sector such as recognizing that products have a halo, or the fact that discounted products will lead to increased product sales etc. These rules can be assembled into a mathematical model and retailers can simulate which scenarios of products and pricing might lead to maximum profit.
- From the historical data, various factors can be analyzed by the algorithm, including: Price elasticity, price variation, promotional elasticity, displacement, forward buying.
- Daisy’s approach is centered around the reinforcement learning branch of AI. After measuring the data, their platform claims to run billions of simulations and, through trial and error, determines the products and prices that are most likely to deliver the maximum return. Sequences of prices and products are forecast in a huge number of scenarios – just like AlphaGo might look 10 or 100 moves ahead in the game to predict possible outcomes.
- Once the scenarios are tested, the AI model makes weekly decisions and deliver the recommendations about what a promotional flyer should look like and a list of most profitable pricing for key products.
Gary tells us that Daisy’s recommendations are delivered through a web portal and a merchant login where people then choose whether to execute the recommendations. Gary mentions that people are still a big part of the decision loop since there are still factors which are not accounted for in the raw transactional and promotional data, such as brand and product supply.
The analogy with AlphaGo is simply a convenient one, in that many people who follow technology in the news will be familiar with the AlphaGo story. The use of AI in retail pricing and product placement might also by likened to applications of artificial intelligence for finding customer segments for marketing purposes. Finding unique customer groups can similarly be overwhelming for people.
Do customers who buy a specific set of jewelry items become more or less likely to purchase clothing in the next month? Are customers who have been inactive for 6 months likely to respond to discount promotions, and if so, which discount promotions?
These questions are endless, and AI systems identify patterns of customer behavior that allow sellers to more accurately group their customers and find ideal opportunities for promotions based on historical purchase and promotional data. The process Gary describes is similar to what we describe above, only related to the unique world of grocery pricing and inventory.
In the sections below, we take a deeper look at how platforms like Daisy can help optimize promotions and pricing for retailers. We use one hypothetical use case, and two case studies of Daisy’s work with its retail clients.
Hypothetical Use Case – Grocery Flyer Design
Challenges for Marketing Teams in Food Retail
In the grocery space, marketing managers frequently need to make decisions of what products to promote every week. Grocers face a number of unique challenges, including:
- Making decisions of which products to promote every week
- Making a decision on the pricing of these products – this would include any sales or discount prices
- Understanding what kind of consequent inventory allocations might be needed based on promotions, seasonality, and other factors
The complex dynamics of the relationships between product promotion, pricing, customers, inventory management are difficult for human analysts to model and predict behavior accurately.
Example
Going Beyond Human Capacity
The answers don’t reside in the data – they reside where the data has never been. Simulations is the key to unlocking more revenue and increasing profitability.
Consider this:
A retailer with 50,000 SKUs requires 6×10 to the power of 3600 calculations* every week to simulate all the options available the selection of products in a typical weekly promotional flyer.
The time it would take the merchant to truly dig into every piece of data for every product promoted- week in and week out—would be mind-boggling.
Now factor in there are 100 stores in 7 price zones with pricing changes happening thousands of times a week.
Now factor the ripples of each decision. The fact is, traditional approaches can’t come close to handling this degree of complexity when it comes to data analyses.
Is it any wonder that 80% of the items promoted each week are decided are repeats from the previous year?
*As FYI, 103600 is an astronomical number; if you added up each grain of sand on the planet, it’s less
Considering the flyers below from Sobeys and Walmart, which were sent out a couple of days before Mother’s Day, 2017, a few days in the following week leading up to Victoria Day. These examples can be used to highlight some of the potential ripple or halo effects of promotional efforts – and some of the considerations that marketing managers might need to take into account.
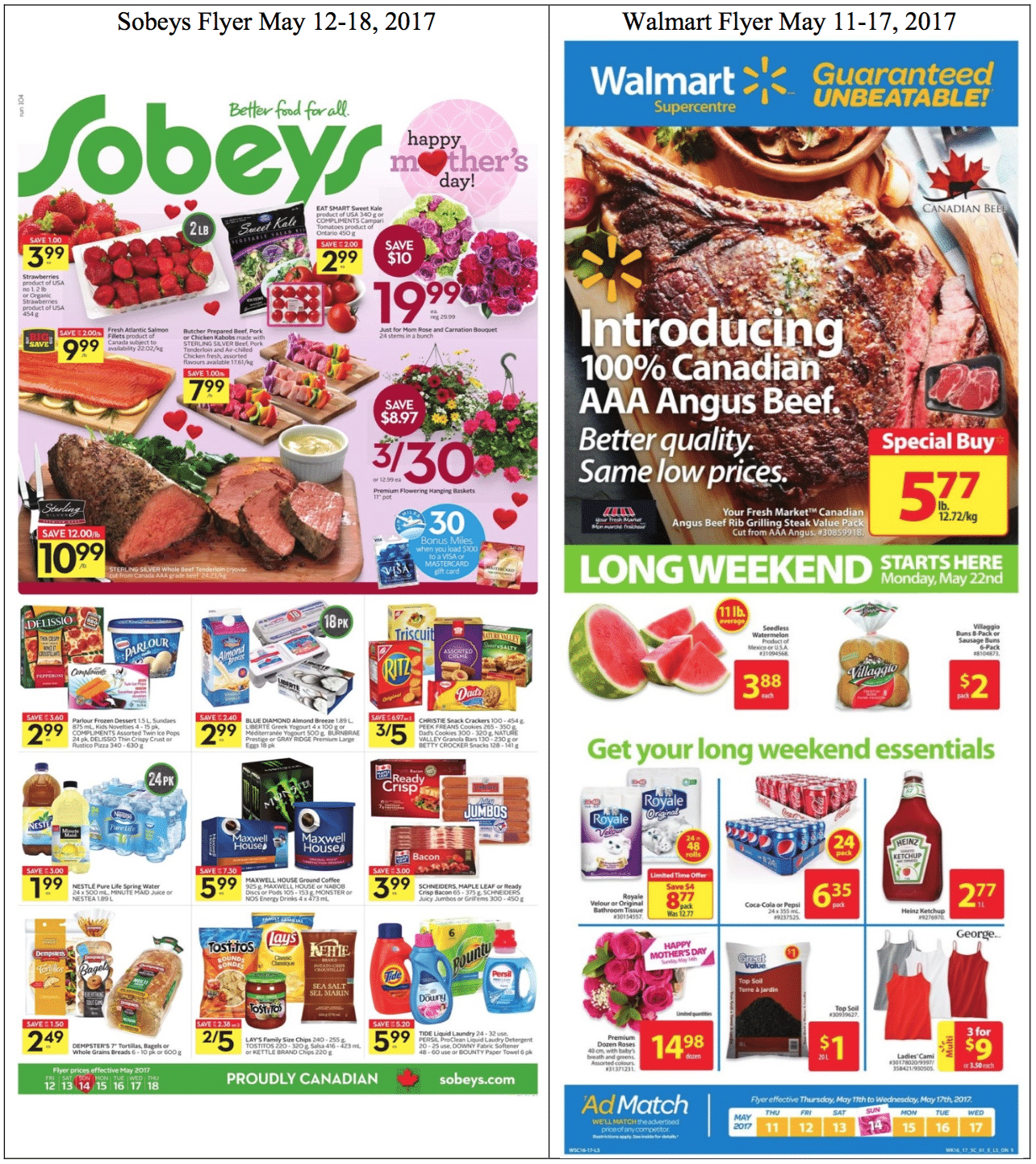
In the flyers above, a marketing manager might have to look at several factors to ascertain the impact of ripple-effects between the different products being marketed on the flyer:
- Walmart seems to be promoting grilling steak in the top section, but also has specials on ketchup and hamburger buns. Marketing managers might need to know what the “ripple effect” of these specials might be, since one possible result could be to drive customers to buy ground beef at regular low prices, and thus, moving customers away from grilling steak – ultimately displacing customers rather than gaining a net positive increase in sales.
- Sobeys might have offered the same promotion one year ago in 2016 just before Mother’s day (Gary informs us that such repetitive promotional use is the common promotional practice in grocery). Repetitive promotions like these might not be leading to incremental growths in margin, and managers may need to consider the impact of different promotional offerings.
The Impact of AI
When large retailers were to engage with Daisy, the process would typically work as follows:
- The retailer’s massive trove of historical data transactional data, client information, promotional records, social and demographic data might be collected and then processed (cleaned and tagged) in a way that makes it fit for an AI platform to analyze.
- The AI platform runs billions of GPU-powered simulations to identity which products will deliver the most and, therefore, should be promoted in the flyer and recommend prices by taking into account cost prices of the products and product supply levels.
- Using the recommendations the retailer might potentially avoid any negative consequences from ripple effects of marketing promotions – or at least give them a best chance of a successful promotion.
Case Study – Grocery and Produce – Earth Fare
Challenge
Daisy Intelligence worked with North Carolina-based Earth Fare, an organic grocery store with 46 locations in the US. Earth Fare’s value proposition meant that their produce had to be “70% organic, humanely raised, antibiotic-free and hormone-free poultry, grass-fed meats and sustainably sourced seafood.”
The stringent standards resulted in their marketing team spending a significant amount of time each week on promotion planning – mostly in the form of the price-based promotion through weekly ads. Earth Fare needed a way to cut down on planning time and improve the results for the promotions.
Actions Taken
Earth Fare had access to large amounts of historical data which was taking a long time to analyze and then determine actionable insights towards improving promotional activities. Earth Fare used Daisy Intelligence to automate the selection of products that needed to be promoted prominently:
- Earth Fare first provided Daisy with four years of historical transaction data and promotional history through an FTP transfer process which doubled as the portal where Earth Fare’s store managers could log in to see promotional recommendations each week.
- Daisy claims that their AI platform was first able to ‘clean and tag’ the transactional and promotional data and members from both Daisy and Earth Fare teams collaborated to resolve any discrepancies in the data (like missing promotional records).
- After this, Daisy’s AI engine determines relationships among product affinities, promotion cannibalization, promotion and purchasing cadence, competitive store openings and weather-related events.
- A team from Daisy then worked with the Earth Fare leadership to understand what the optimization goals (sales, margin, traffic, etc.) of the company were. This also included understanding factors like how many products did Earth Fare want to promote in each category or through which marketing channels.
- Earth Fare’s promotional strategy process was then analyzed by both teams working together with the aim of determining which parts could be optimized using the Daisy platform. This included determining areas where Daisy could potentially optimize Earth Fare Promotion planning with the end goals being reduced planning time for promotion and increased returns on promotions.
Results
According to Earth Fare, Daisy Intelligence’s AI system helped Earth Fare to analyze the halos for each product and understand what the ‘ripple effects’ of promoting one product were on other products and what it might be in the future. We take a closer look at some of the results of the integration and how it impacted Earth Fare’s business.
We first define the term net promotion effectiveness (NPE) which is an indicator that Daisy uses to measure the impact of a promotion. According to the case study from Daisy, NPE is measured as “lift” in sales of promoted products over their baseline sales plus incremental sales of associated products less any cannibalization or forward buying done by customers’
- Earth Fare’s promotion optimization collaboration resulted in increases of 3% to total top line sales.
- Prior to the integration, the items promoted by Earth Fare’s marketing team contributed around 20 percent of total sales. Post the collaboration Earth Fare claims that the products promoted after recommendations from Daisy led to the NPE increasing to around 40 percent of total sales from 2016 to 2017 — doubling the impact of promotions. (See Figure Below)
- “Without a doubt, Daisy has driven a ‘meaningful lift’ in incremental same store revenue and trips without any additional margin cost,” said Scott Little, Earth Fare’s Chief Financial Officer.He also said the collaboration has cut down on the market team’s planning time.
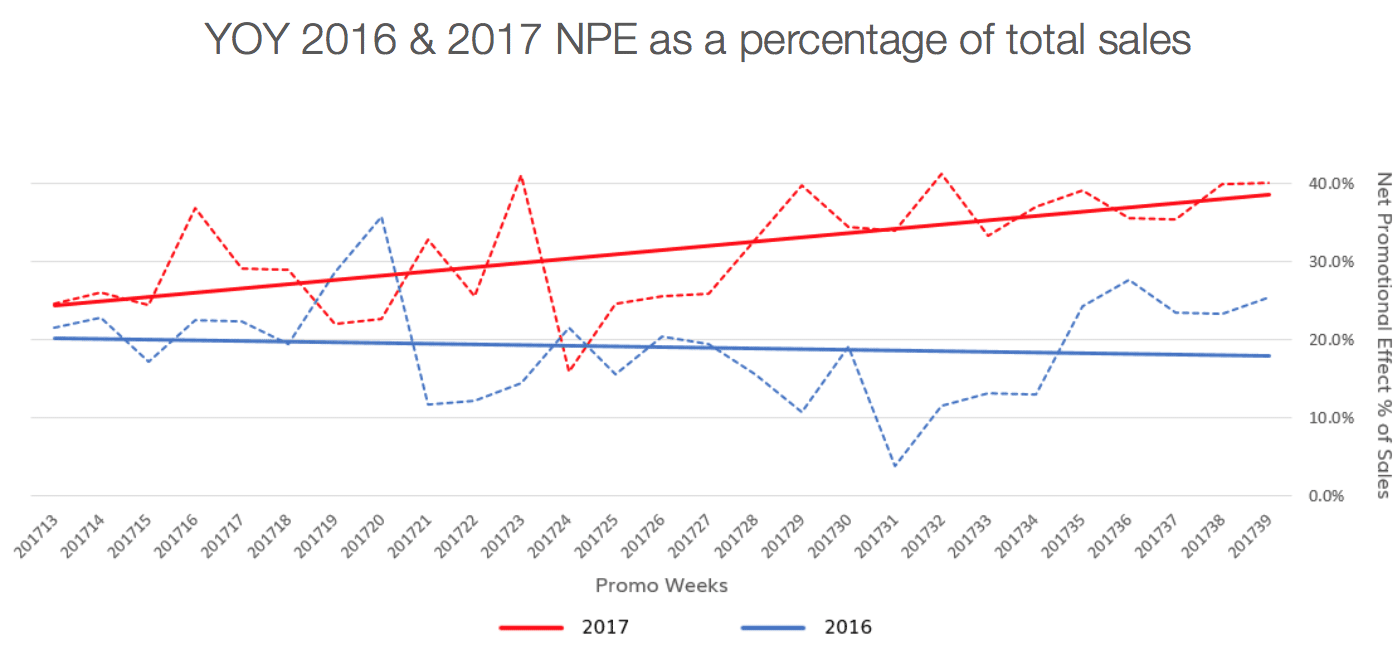
Optimization.
Case Study – Supermarkets – Harps Food Stores
Challenge
Daisy worked with Harps Food Stores, a chain of supermarkets based Arkansas. Harps needed to measure the impact of their pricing strategy and promotion which was important to understand the relationships between their products and customers. In addition they were also unable to track product halos and measure ripple effects of their promotions from traditional methods used in the grocery industry (much of which, Gary tells us, is based more on gut feeling than on statistical significance).
- Harps Food Stores needed to identify which products to promote on their weekly circular
- Additionally, they needed to understand what pricing and promotional ripple effects might be impacting their overall margins
Actions Taken
In 2016, Harps enlisted Daisy Intelligence to help them analyze two to five years of historical transactional data, and then run simulations to find the most profitable promotional decisions. According to the case study, Daisy’s AI model was able to analyze the data and recommend which products should be featured in Harps’ weekly print circular – which was their largest advertising expense.
Results
Harps shared that Daisy’s AI enabled their merchandising and marketing personnel to rapidly analyze transactional data on a massive scale, simulate potential strategies and ultimately improving the process by which the company makes promotional planning and pricing decisions.
“Daisy Intelligence now gives us the ability to see all of the associated sales related to every item. We can look across all transactions and see what is the impact of that one item in the basket.” – David Ganoung, Vice President of Marketing at Harps Foods.
The case study states that Harps tangibly improved their promotional efficiency with the implementation although further details on robust measurable results were not shared with us for this piece.
Takeaways for Retailers
Retailers may use AI for pricing and promotion optimization in retail for the following reasons:
- Margins in the retail industry are very small (as low as one percent) and retailers need to gain every edge they can to optimize their operations for sustained profits.
- Retailers need to know which products to promote every week (or month, or season) for maximizing returns.
- Grocers and retailers need to understand the ripple effects of what promoting one product might have on the overall net margins, and how some product purchases displace others. These relationships might be highly complex and time consuming for humans to model accurately.
The goal of most high-frequency, high volume retailers applying AI for pricing and promotional optimization can be summarized as follows:
- Using AI to identify the right products to promote, in order to maximize profit margin.
- Getting actionable insights for pricing of various products each week, and understanding the impact of promotional efforts in real time.
- Predicting inventory allotments in order to better prepare for promotions and sales.
The analysis of halo effects in retail is a perfect example of a problem that might otherwise be unsolvable without machine learning. Factoring for promotions, seasonality, and the impact on other sales – and looking at all of this data year-over-year – allows for an almost unlimited number of hypotheses to test.
For example: Are sales of a new brand of candy bars reducing sales of other candy bars? Does candy bar sales impact the sales of other sweets? Does it impact the sales of other items that children enjoy? Does the sale of sweets impact other product categories?
The questions are endless, and rather than assessing the data question by question, AI offers the promise of coaxing out the patterns from the raw data, and presenting them for a user to explore.
This article was sponsored by Daisy Intelligence, and was written, edited and published in alignment with our transparent Emerj sponsored content guidelines. Learn more about reaching our AI-focused executive audience on our Emerj advertising page.
Header image credit: Citipost Mail