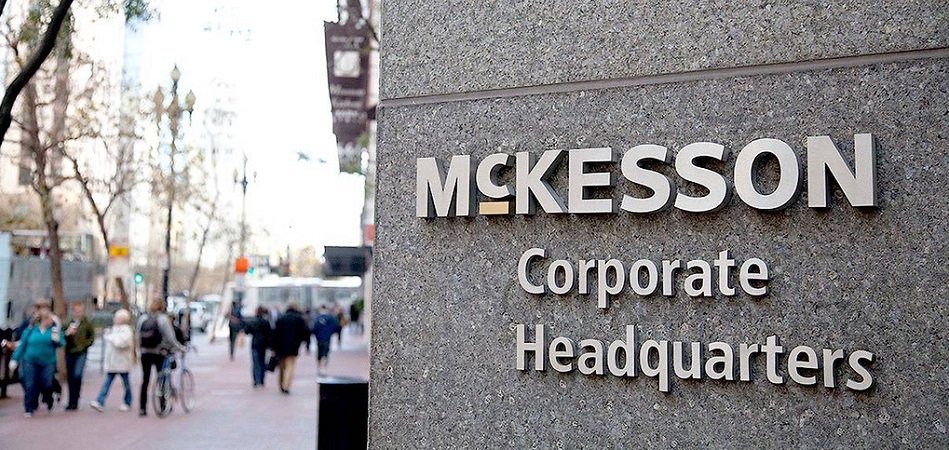
McKesson is a large pharmaceutical distributor that delivers health information technology, medical tools and supplies, and care management tools and software to healthcare providers in the United States. The company has been working to incorporate artificial intelligence solutions internally since 2012, but in more recent years they have adopted a few important automation tools.
These tools help them free up white-collar workers to take care of more specific work that only humans can do. Additionally, they help the company make a smooth transition into value-based care, which could result in higher annual savings.
In this article, we take a closer look at how McKesson facilitates healthcare AI through investments and building a new digital infrastructure internally. We also explore the tools McKesson is currently using to automate business areas that human employees may not be suitable for.
We discuss the following initiatives:
- Facilitating AI Inside and Outside the Enterprise:
- How McKesson helps AI firms develop healthcare applications they are interested in, such as Komodo Health.
- How the company makes it easier for all their employees to access and make better use of the AI tools they currently use.
- Internal AI Initiatives for White-Collar Automation and Finance Management:
- McKesson’s partnership with Genpact that allows them to automate numerous white-collar processes
- Their acquiring of Change Healthcare and how their predictive analytics software helps discern reimbursement rates for value-based care.
We begin our exploration of McKesson’s current AI initiatives with their investment in Komodo Health and adoption of the Google Cloud platform as flexible digital architecture.
Facilitating AI Inside and Outside the Enterprise
McKesson has made changes to allow their business to more easily adapt to new AI technologies. Their move to the Google Cloud platform is perhaps their most important change in recent years, because it enables faster implementation of new AI tools across all business areas.
Additionally, they look to smaller companies in the healthcare AI market to invest in so that they may have even more useful applications in the future. This is emphasized by their investment in newer companies with which they can build a relationship with early and possibly influence their approach to making their AI products.
Investing in Komodo Health
McKesson Ventures, the company’s own venture capital fund, invested in AI healthcare firm Komodo Health. Komodo claims their predictive analytics platform helps doctors diagnose and keep track of disease progression in patients. It purportedly can help doctors deliver individualized care by supplying insights about each patient or patient population. Komodo claims that all of this results in a shorter time between the patient’s check-in and their treatment.
Komodo most likely achieves this by training their machine learning model on a large amount of anonymized patient data. This way, patient names and other personal information will not be circulated and thus remain compliant with healthcare regulations. The data might involve various details about a patient’s health, such as:
- Medical history, including past injuries and ailments
- Past disease and ailment treatments that have worked for the patient
- Medications they are currently taking
- Any medication allergies
- The patient’s current symptoms
Each of these categories would then be labeled, and Komodo likely labels each piece of data under one of these categories. After Komodo’s data scientists expose the machine learning model to all of this data, it would then be able to discern correlations between symptoms, how they get worse, and which diseases or ailments they are most commonly associated with.
Once trained, their predictive analytics model could be used to help physicians understand their patients’ illnesses. This software most likely uses the context it has on the medical history of past patients to predict what a doctor’s diagnosis will be on a current patient.
This could be especially helpful to McKesson because of its purported capacity to predict the progression of a patient’s disease, which would allow healthcare providers to more easily communicate to McKesson which products they need and when they need to arrive.
McKesson’s world cancer day update suggests that Komodo Health’s AI platform can also be used to accurately predict cancer relatively early compared to current diagnostics. They emphasize this with a quote from Dr. Arif Nathoo, CEO of Komodo Health.
With regards to the data used to create these predictions, Nathoo said, “Just as Amazon can tell you when you need more cereal or new running shoes based on behaviors, we’re learning from the journeys of patients across 50 billion clinical encounters how to train our algorithms to detect cancer well before symptoms manifest.”
While 50 billion clinical encounters may seem like an untenable amount of patient data to use, McKesson claims Komodo uses the medical history of over 300 million patients to train its software. If this medical history includes each and every doctor’s appointment and emergency room visit on each patient’s record, then it is possible their database reaches 50 billion individual encounters.
McKesson Moves to Google Cloud
McKesson recently moved away from other cloud-based technology platforms in favor of the Google Cloud Platform (GCP), allowing them to distribute new applications and tools across the company more easily.
They claim that it will also advance the development of their new AI technology, which includes improving their data analytics capacity. This primarily supports McKesson’s strategy for optimizing how they deliver healthcare and products more efficiently.
The company claims the move to GCP will ensure that their digital infrastructure will be flexible. This likely means each department will not need to invest hard drive space to constantly installing new AI tools and updates to make sure they are operating with the newest technology.
Instead, employees use Google Cloud to remotely access the applications they need to use for their jobs, and updates are run through the cloud so that nobody can miss an update.
McKesson also claims this flexible infrastructure will allow them to accomplish the following advancements to their technological resources:
- Create a scalable and agile database that is more useful for the company itself and the other healthcare businesses it works with. This likely means that medical data is stored in a directory where it is labeled normally, but not organized according to those labels. This allows for different ways to organize patient data and clinical data so that it can be used by anyone interfacing with McKesson’s Google Cloud applications.
- Modernize their solutions to common business problems such as product manufacturing, specialty product distribution, and pharmacy retail operations. This is purportedly accomplished through developing and testing new applications and software tools through GCP.
- Capture new medical data in real-time using cloud-based data analytics tools, which likely helps them develop and improve machine learning models. This could allow for more up to date insights on specific patient populations or how patients commonly react to certain diseases.
- Have a bigger capacity for integrating machine learning and AI into the enterprise. Because the AI software does not need to be installed to each computer, or even to a central computer onsite, integrating AI applications may take much less time. When combined with the real-time cloud analytics mentioned above, AI applications could likely continue updating automatically without a human monitor.
- Expedite their ability to deliver new software and services along with the scalability to handle big-picture issues as well as granular business problems. This may seem redundant, but McKesson likely included this statement because Google Cloud Platform allows them to accomplish this faster than they ever have before.
- Reduce the need for managers and administrators to always be checking on and updating the digital architecture. This allows data scientists to focus on new projects within an infrastructure they can already rely on to be flexible enough to integrate their projects.
Below is an introductory seminar about using machine learning and AI on the Google Cloud platform. In this video, a Google cloud expert describes how businesses can use the platform to facilitate the use of AI across their entire enterprise. Because the video is quite long, we have included a list of the most useful moments below.
- 6:12 – Machine Learning as an API. GCP has 5 machine learning APIs that can enable AI tools that use natural language processing, translation, speech recognition, machine vision, and “video intelligence,” or insights from data gathered with machine vision.
- 21:56 – GCP tools for training machine learning models on a business’ proprietary data. These tools are called TensorFlow and ML Engine. TensorFlow is an open-source Google-created machine learning training tool, while ML Engine is a tool specifically for GCP.
- 24:18 – How custom machine learning models work on GCP using TensorFlow and ML Engine.
Internal AI Initiatives for White-Collar Automation and Finance Management
McKesson has made some important partnerships and mergers in the past few years that have improved its business processes using machine learning and AI. They have partnered with the AI firm Genpact in order to automate white-collar pharmaceutical work and free up employees to focus on delivering care.
Additionally, McKesson acquired Change Healthcare and are utilizing their predictive analytics software to provide value-based care and automate claims management.
White-Collar Automation at McKesson and Their Partnership With Genpact
McKesson has been working on data analytics systems and finding better and better ways to manage their data since 2016. This has allowed them to try out different approaches to artificial intelligence in various business areas, but most recently they have leveraged the technology for numerous white-collar use cases. They take advantage of these use cases through their use of AI applications provided by Genpact, a partnered company.
The white-collar business areas that McKesson has been able to automate include:
- Order to cash: The process of aggregating sales orders for pharmaceuticals and medical services, and taking in payments for those sales. Could come from individual patients, insurance providers, or hospitals in the case of medical tool sales.
- Record to report: The collection and distribution of important information, ideally in a timely manner. This includes communicating pathology and radiology information about patients to the correct doctors and pharmacists. Alternatively, this can be useful in a medical testing lab where results may need to be distributed to many departments at once.
- Contract management: The process by which written and signed contracts are distributed, then sent back to the relevant departments. These departments will need to know the contract has been signed in order to fulfill a task or ship a product. Additionally, data analytics systems may take any currently active contracts into account when recommending the best course of action for a business leader.
- Master Data management: An automated process that manages a company’s store of big data and where that data is stored within the enterprise. This is most likely in reference to patient data and electronic health records (EHR) stored somewhere in McKesson’s database.
- Source to pay: Managing the company’s financial resources in order to reduce company spending in frivolous areas. This helps business leaders decide the areas that require the most funds and prioritize based on large amounts of enterprise data. This is most likely accomplished using predictive analytics to create a budget while taking patient data into consideration when calculating possible resource utilization in the future.
McKesson’s “source to pay” and “record to report” processes are the most likely to be automated through predictive analytics. This is because managing and recommending good business spending habits requires using historical spending data and the ROI yield from past expenditures.
The image below is an example of one of Genpact’s AI software solutions, APFlow. The company claims APFlow is their recommended solution for the source to pay process.
The user is able to visualize all invoices within a system and group them by document type and payment status. The dashboard allows the user to bring up recently viewed graphs and refresh the visualizations based on any changes to the data:
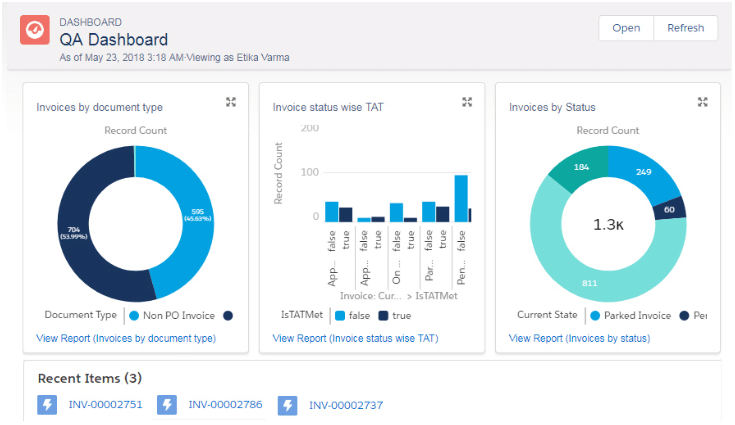
Additionally, predictive analytics software can predict where a certain type of document or memo needs to be sent based on information on past data transfers within the enterprise.
In the case of new personnel or a change in which departments are prioritized to receive information, however, this may be bypassed by directing the software to send the information to certain people.
This is different from a non-AI analytics engine that simply shows the most current trends in a company’s data. We spoke to German Sanches-Trilles about the differences between analytics and predictive analytics in our interview about data science terms that people commonly get wrong.
When asked about what makes predictive analytics different from more common analytics engines, Sanches-Trilles said:
If we compare it with normal analytics, when I listen to the term analytics, the first thing that comes to my mind is Google Analytics. [In Google Analytics,] you look at the traffic of your website, perhaps the purchases of your eCommerce, looking at the data and trying to understand it.
When you are doing predictive analytics, there is this predictive part in which you think of the data you have, you build something with that data, an algorithm or a model or whatever, and try to infer something that is going to happen in the future based on the past.
Predictions from AI software are reviewed by a human employee before a final decision is made. Because of this, predictive analytics software can help business leaders manage finances using all of their enterprise data with the freedom to accept or reject what the results may imply.
Acquisition of Change Healthcare: Claims Management and Predictive Analytics
Recently, McKesson acquired Change Healthcare, an AI firm that has allowed them to access their predictive analytics technology for value-based care and medical reimbursement. Value-based care is a business model that charges the patient based on the value of care they received.
This functions differently from individually priced medicines and services as products based on a typical supply and demand market strategy.
Change Healthcare has AI analytics software that can possibly allow McKesson to ensure the accuracy of payments and revenue by reducing finance errors like missed payments and chargebacks.
One of McKesson’s chosen solutions from Change Healthcare is their recent software called Claims Lifecycle Artificial Intelligence. This is part of Change Healthcare’s “Intelligent Healthcare Network,” or its AI platform specifically for solving business problems in healthcare.
The company claims the Claims Lifecycle solution can help optimize claims processing for both healthcare providers and payers such as patients or their health insurance provider.
The “claims lifecycle” referred to in the name of the solution refers to the chain of events that starts when a claim is initially filed and ends when it is paid off or rejected by the company.
The software purportedly detects claims that will be denied while the claim is submitted. The machine learning model behind the software likely compares new claims with past ones that have been accepted. This allows it to discern if the claim is accurate or not.
The graphic below from McKesson illustrates how Claims Lifecycle Artificial Intelligence observes details about input claims and then accepts or rejects them:
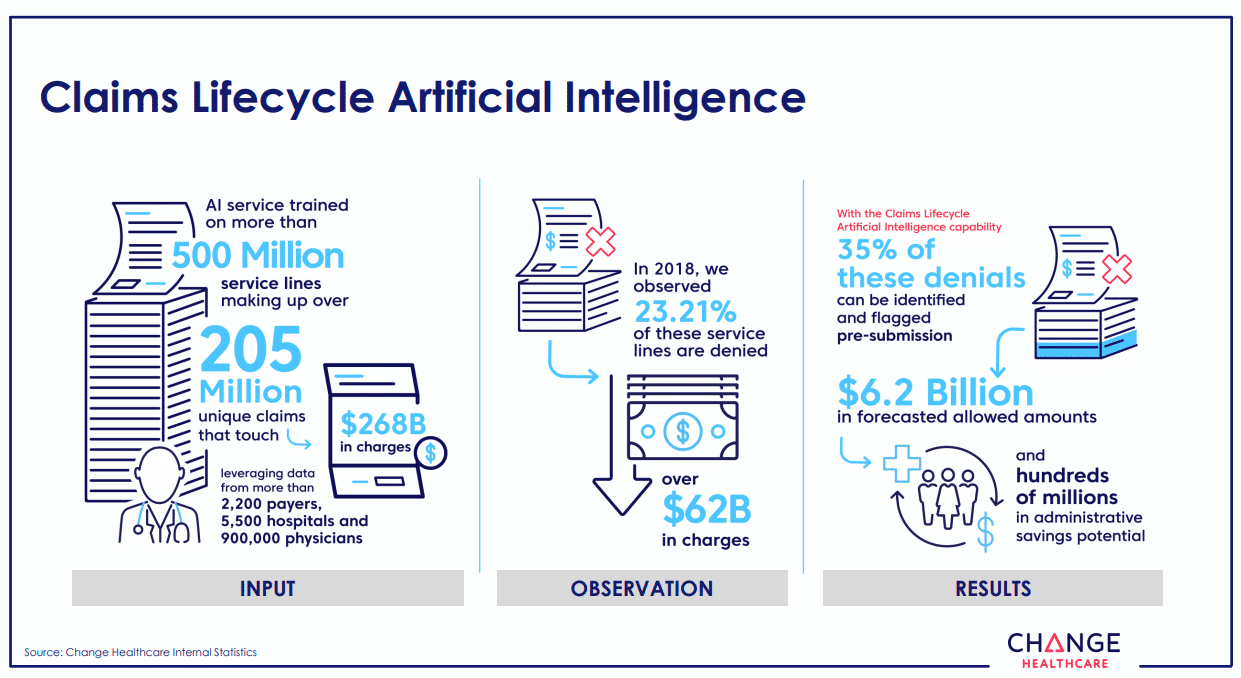
Claims Lifecycle Artificial Intelligence can reject claims based on the signature on the form or the dollar amount the claim is for, however, these are just some of the possible parameters. A denied claim could get sent back to the claimant so that they may complete any parts of the claim they may have missed.
Additionally, this allows the claimant to correct any information that the system rejected. According to Change Healthcare, this system has resulted in $6.2 billion in savings within all companies that use this solution.
It is very possible that this software uses predictive analytics to determine if the information within each claim is accurate or complete. This inference comes from the fact that Change Healthcare is a company focused on data analytics and predictive analytics, and this was true even before they were acquired by McKesson.
Unfortunately, there is currently no information on this solution that confirms it as predictive analytics.
Predictive Analytics Software
Change Healthcare’s analytics platform is called HealthQx, and it purportedly can help healthcare providers make the transition to value-based care and reimbursement. These are required under the Affordable Care Act (ACA), the solution uses predictive analytics to determine the value of the care each patient received.
Additionally, automating this process facilitates reimbursement in the elimination of any possibility for human error. When a human employee cannot retain all of the financial information at once and makes a mistake, it could lead to less reimbursement for their company, which affects the total revenue for that company.
HealthQx uses a technique called “episodic claim grouping” to detect when business costs rise and fall. The software can then take the time period between when costs initially spike and when they fall back down as an “episode” so that it can focus in on a specific time where costs surged to find exactly how much a provider needs to be reimbursed.
HealthQx can use the data from each of these episodes to gather more insight to use in future analytics queries and projects. Change Healthcare refers to this data utilization as “episodic analysis.”
However, episodic claim grouping is just one technique a predictive analytics software could use to find this type of insight that a client company could not find prior to adoption. We spoke to Steve Gullans, Managing Director at Excel Venture Management, about investing in AI healthcare applications.
While our interview mostly deals with why doctors don’t want to be fully replaced by AI, Gullans made an important observation regarding white-collar and financial automation in healthcare:
…Looking for diamonds in the rough or opportunities that are undervalued, by looking through the patent histories and seeing what’s out there that people don’t appreciate, on a global basis…you could actually find things that were invisible before.
It would seem that even investors at large venture funds are interested in how patient history can help determine the value of their care. Because of this, business leaders should keep an eye out for AI firms that purport to solve value-based care problems if that is a business area they are considering for integration.
It is likely that the machine learning model behind HealthQx was trained on thousands of records from client healthcare providers such as EHRs, healthcare claims, and financial data. It would need to be relevant to the client company’s resources and financial plans, patient health levels and satisfaction, and the list of services that can be reimbursed.
Additionally, it is possible that the software has a natural language processing (NLP) section. This is because EHRs contain unstructured written information that NLP can process whereas predictive analytics cannot.
Both Change Healthcare and McKesson claim that HealthQx is being leveraged by health plan providers that cover 33% of commercially insured citizens in the United States. However, they do not mention any of these providers by name.
It would appear that McKesson is seeing some success with the software as a value-based care solution, but they do not state any savings figures in regards to their transition into AI-powered value-based care.
Header image credit: Medium