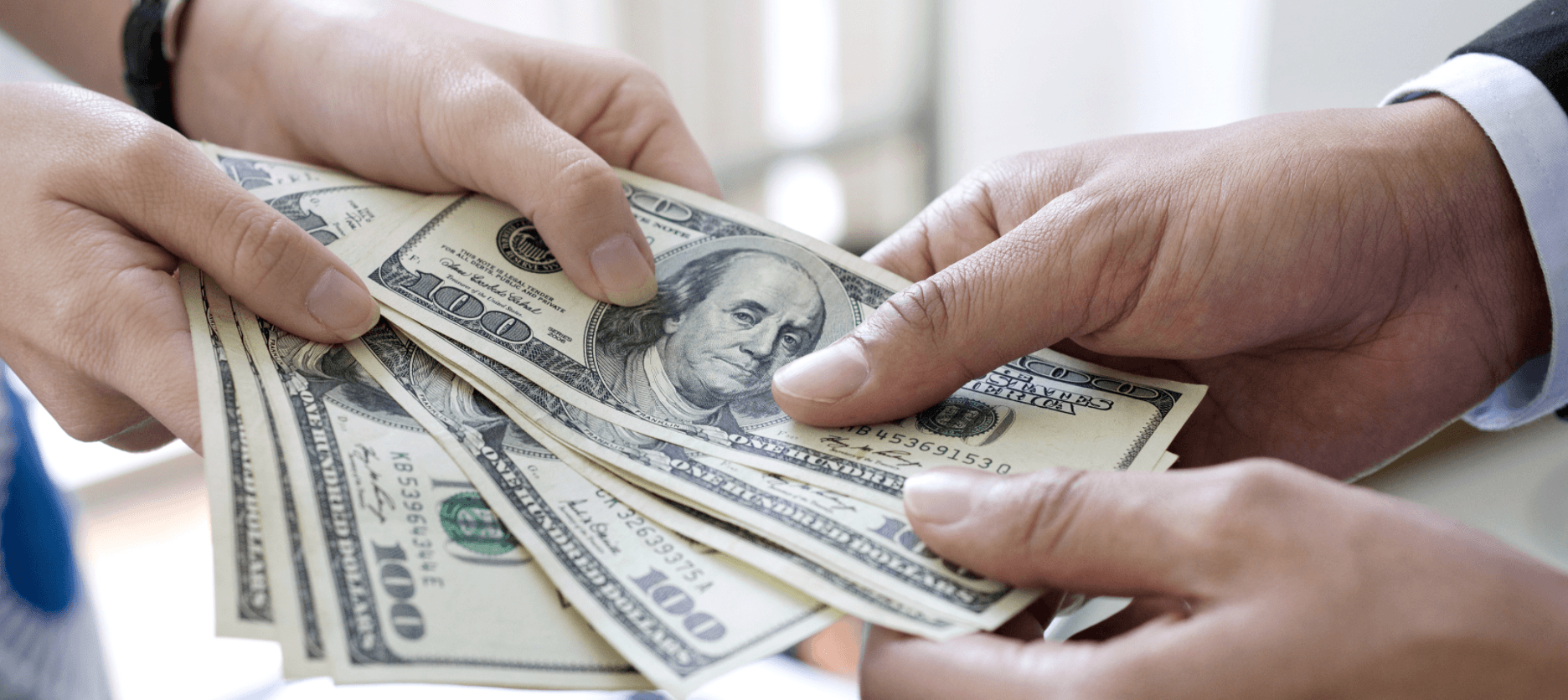
Lending is a massive business in the United States which directly and indirectly touches almost all parts of the economy. With tens of millions of Americans holding loans worth trillions of dollars, any technology that can make even a small improvement in a company’s returns on the loans they hold, or that can improve their share of the market, would be worth a significant amount of money.
That is why both established banks and startups in the field are constantly looking for ways to innovate – and artificial intelligence might allow for just that. In fact, our AI Opportunity Landscape research shows that approximately 15% of the venture funding raised for AI vendors in the banking industry is for lending solutions.
At its core, lending is a big data problem, making it a business naturally suited for machine learning. Part of the value of a loan is tied to the creditworthiness of the individual or business that took out the loan. The more data you have about an individual borrower (and how similar individuals have paid back debts in the past), the better you can assess their creditworthiness.
The value of a loan is thus tied to assessments of the value of the collateral (car, home, business, artwork, etc…), the likely level of future inflation, and predictions about overall economic growth. The promise of AI is that theoretically it can analyze all of these data sources together to create a coherent decision.
Our Overview of AI in Lending
This article will look at some of the newest and most interesting attempts to use AI and machine learning in the lending business. It will not look at every single use of AI in the sector but will give an overview of major applications. Specifically, we’ll cover:
- How AI is being used to determine creditworthiness, particularly for those without credit histories
- How AI is being used to streamline the loan process
- How AI is being used to improve customer experience for borrowers
It is also worth nothing all of these goals also feed into each other. For example, the better you can determine an individual’s creditworthiness, the more easily you can streamline the internal processes. Similarly, the quicker and less hassle a process is, the more appealing it is to customers.
(Readers interested in a broader perspective on finance applications beyond loans can explore our full article on finance applications of AI.)
Determining Creditworthiness
The value of most loans is mainly based on how likely an individual or business is to pay it back, so determining how likely an individual is to default is critical for the whole sector. It can be a complex task even with perfect information, and often the information is incomplete or wrong. Individuals and businesses will sometimes lie. Several companies are using AI for risk assessment.
While in the past lenders looked at only a few metrics like FICO score and income, companies have started looking at an individual’s entire life and even their vast digital footprint to determine how likely they are to default. This is referred to as “alternative data” about potential borrowers. The idea is that extra data provides not just more insight into people with established FICO scores, but that it can be particularly useful for determining the creditworthiness of people without a traditional credit history.
Lenddo – Digital Footprint Analysis
One startup aggressively using advanced machine learning to comb through vast sources of alternative data to predict an individual’s creditworthiness is Lenddo. The company started in 2011 and focuses on emerging markets where rising middle classes often lack traditional credit histories or even bank accounts. They claim 5 million people receiving loans via their partners because their system was able to evaluate their creditworthiness.
As the video below explains, Lenddo looks at a potential applicants’ entire digital footprint to determine their creditworthiness by having individuals download their app. They claim it looks at over 12,000 variables including social media account use, internet browsing, geolocation data, and other smartphone information. Their machine learning algorithm turns all this data into a credit score, which banks and other lenders can use.
Lenddo claims not to share this personal data with lenders, only the final result of their analysis to protect individuals’ privacy. They claim their system has allowed their partners to approve up to 50 percent more applications.
Recently, FICO, the global credit agency, announced a major partnerships with Lenddo to use their technology as part of FICO’s new FICO score services in India. In addition earlier this year Experian partnered with Lenddo to use their technology in Indonesia and Vietnam.
ZestFinance – Artificial Intelligence and Search-Based Analysis
ZestFinance is another company using machine learning to process alternative data to get information on so-called “thin file borrowers” — those with no or little credit history. As they explain in this video, they provide companies with the tools to use data sources to do underwriting.
One of their most significant recent announcements was a strategic investment from Baidu, the leading Chinese internet search provider. ZestFinance will use Baidu search data to develop credit scores for individuals, giving them a massive amount of data for the large Chinese market where traditional credit score systems are mostly lacking.
ZestFinance will be able to make use of Baidu’s search, location, and payment data for individuals. ZestFinance is able to help lenders determine the creditworthiness of Baidu users – even if said users have very little credit history. According to ZestFinance, there are half a billion people in China with no credit history.
In many ways, this approach models some of the “individualized data” use-cases of AI in insurance that we see at firms like Progressive, where data is collected about individual drivers to better predict their risk of accident. It’s not surprising that similar approaches would be used to refine risk predictions for lending as well – and it’s a trend we expect to continue well into the decade ahead.
ZestFinance also recently finished a study with Ford Motor Credit Company. Based on the success of the study, Ford Credit is developing plans to use machine learning in their auto financing.
Equifax
It is not just startups using machine learning and some alternative sources to better determine an individual’s creditworthiness but the big established players as well. Equifax is one of the three big credit bureaus. Peter Maynard, Senior Vice President of Global Analytics at Equifax, in interview this year claimed their new “neural network improved the predictive ability of the model by up to 15 percent.” Using it to look back at recent decisions, they found that loans which were turned down could have been made safely.
Streamlining
Finding new and better ways to determining the creditworthiness of individuals is one way to increase business and gain customers. Eliminating administrative overhead and delays is a way to maximize the amount of profits for each loan created. For years banks and other lenders have being using computer systems to automate more and more of the loan process, but now some companies are trying to fully automate the process.
Upstart – Full Automation and AI Determined Creditworthiness
One of the most high profile startup companies using AI to determine creditworthiness and streamline the loan process is Upstart. Two of its co-founders are former top Google employees. Dave Girouard was formerly the President of Google Enterprise and Anna M. Counselman led Gmail’s consumer operations.
Upstart started by focusing on younger adults who lack much credit history. In addition to traditional FICO scores and years of credit, Upstart also takes into account education, SAT scores, GPA, field of study, and job history to use machine learning to predict an individual’s creditworthiness.
A major goal of Upstart is to use modern data science to automate the loan process. They claim to have been able to rapidly increase the amount of loans they are able to fully automate and as as of September 2017 the have reached 40 percent automation. While other companies have also automated some of the data entry, processing of paperwork, and verifying basic information most loan applications are still reviewed by a human underwriter before they can be approved. A human who checks that the information is accurate or the loan makes sense.
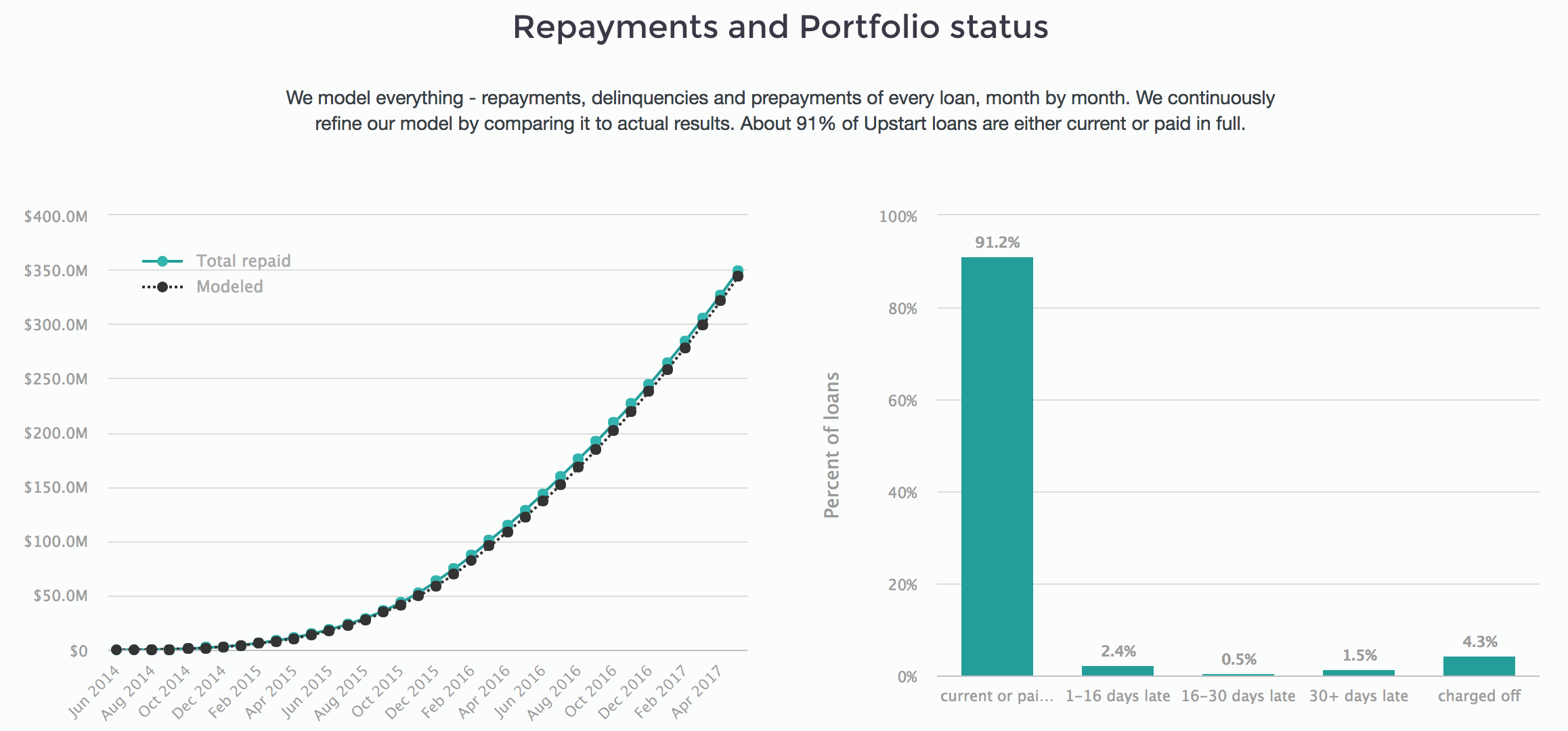
Upstart is a hybrid lender, which makes some loans directly and facilities loans for other lenders. This year it also started offering its technology to other companies via software-as-a-service.
Improving Customer Experience and Finding Customers
This section will look at only loan specific-uses of AI to attract and engage customers. Obviously, big banks along with a whole range of sectors are adopting general AI-powered tools such as chatbots, customer relationship management tools, and advertising analytics.
Personetic – Loan Repayment
Personetic is a cognitive banking company that provides AI applications to major banks (such as Royal Bank of Canada and Ally Bank). A recently unveiled application from the company is a tool, Personetics Act, to help individuals save money.
The video on their homepage shows how Personetics Act works for customers.
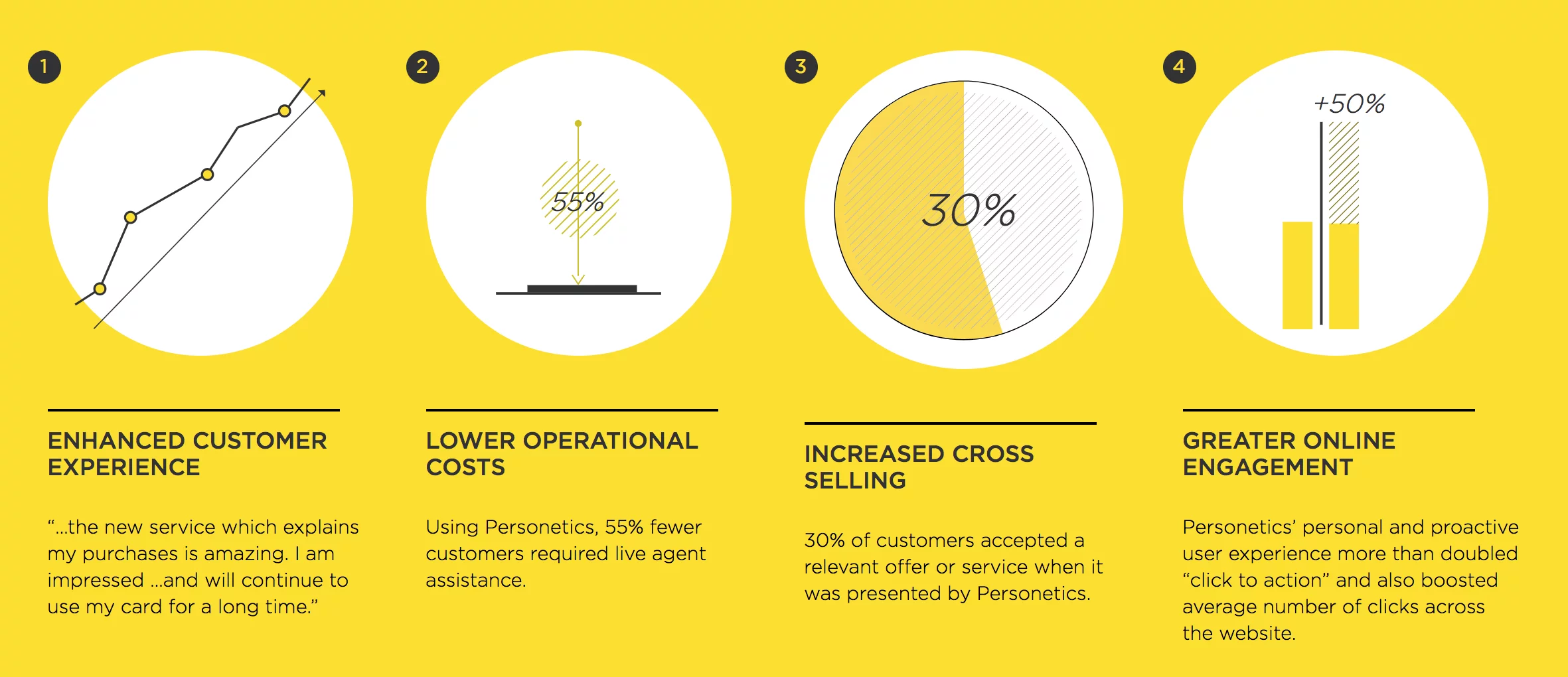
They use the same basic technology to help individuals pay off their student loans faster. They claim their system uses machine learning to determine analyze individual’s financial habits to determine if they can afford to pay back their student loans more quickly. The system can then automatically suggest to individuals how much more they should contribute.
Amazon – Small Business Loans
Given the dominant role Amazon plays in online retail, it has a huge amount of proprietary information on what products are sold on their site, how customers feel about those products, the economic status of the companies which make those products, and the likely future demand for these products.
Amazon is using this data in machine learning models to find companies to offer small business loans to. The program is invitation only. Amazon finds companies to give loans to and makes the application very easy. Their proprietary source of data can might provide Amazon a better understanding of what some specific companies might want a loans and their relative creditworthiness than other traditional lenders. It is possible Amazon could realize a small business could use a loan and offer them one before the small business owner even does.
The program is invitation only. Last year, Amazon lent out roughly $1 billion to small businesses that use its marketplace.
Conclusion Thoughts and Future Trends for AI in Financial Lending
The use of machine learning to analyze alternative data in loans and credit rating is going to raise some privacy, ethical, and legal concerns. Many people might not feel comfortable with a company having access to all of this sensitive information about their life. Even if all these companies behave ethically, the more data they hold the more that can be stolen by malicious hackers in a data breach.
The use of “big data” also runs the risk of companies accidentally or purposely discriminating against groups. For example, a program might not deny applications from protected minorities, but it might deny applications for individuals who have a dozen data markers that just happen to highly correlate with those groups.
Even with these concerns the use of machine learning to process alternative data to determine creditworthiness is likely to grow significantly. There are billions of people without real credit histories that companies may one day want to offer mortgages, payment plans for products, credit cards, or other loans. The financial appeal of these tools is obvious. It is reasonable to believe that the more information you gather about an individual, the more likely you would be able to predict their behavior, including how diligently they would pay back a loan.
One big caveat is that any system is only as good as the people who set it up. Many of these applications are new and have only really existed during a time of modest economic growth. It is easy to appear right about the worthiness of loans during good times; the real test is often how they do in a downturn.
Even if machine learning can accurately use an individual’s digital footprint (purchase history, app use, search history, social media activity, etc) to determine their creditworthiness, it doesn’t necessarily that machine learning systems will always yield better results than traditional credit measures (though even those traditional methods for credit analysis may be low-hanging fruit for “white collar automation”).
It is possible to design a program to give you the answer you want instead of the correct answer. As we saw with the crash of the credit default swap market, if the incentives are wrong, companies can create reasonable sounding justifications to give bad loans the seal of approval. It is possible some companies might accidentally or intentionally use new machine learning approaches to play into the same errors.
Instead of finding more people who are a low risk for loans, AI companies might just create a plausible excuse to provide loans to those who are a bad risk. It is possible that the success of some of these startups could lead to other less scrupulous entries into the market.
Emerj for Financial Services Leaders
Emerj, the AI Research and Advisory Company, works with financial services firms to assess where AI can drive value at their companies. We help lenders find out if they can leverage AI to increase customer lifetime value, underwrite more loan applicants, reject riskier applicants that traditional underwriting methods may not catch, and comply with regulations.
Leaders at large lenders use AI Opportunity Landscapes to develop winning long-term AI strategies that allow them to pave the way toward data dominance in their industry. Contact us to learn more.