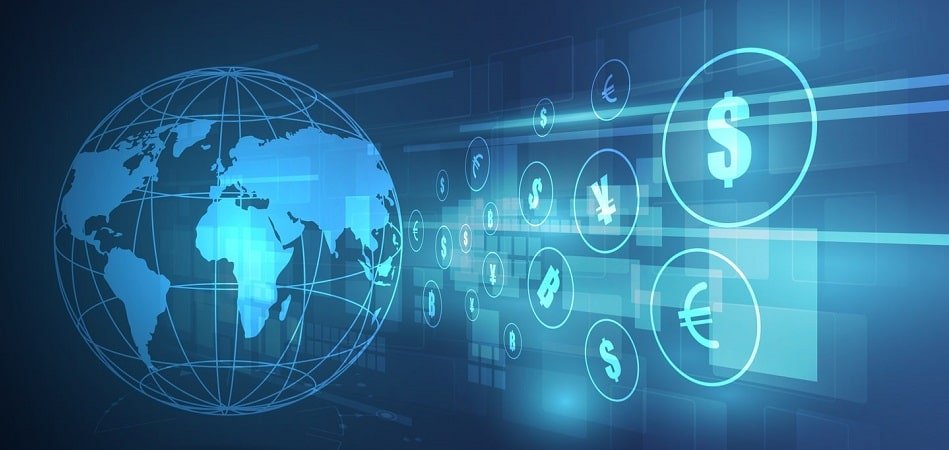
AI applications for the banking and finance industry include various software offerings for fraud detection and business intelligence. There are also predictive analytics applications outside of these that help banks automate financial processes and services that they offer their customers and provide internal analytics.
In this article, we identify three ways predictive analytics software could be leveraged by banks and financial institutions for automation and business intelligence purposes. First, we explain how data analytics could be used to better understand customer behavior and then provide an example of how that behavioral information could benefit banks. We then look a bit deeper into how this technology could be applied to predict outcomes across a longer period of time.
The following is a list of the banking possibilities of predictive analytics software covered in this article:
- Customer Analytics: for product creation and improving the customer experience
- White-Collar automation: particularly, accounts receivable software for matching corporate clients to invoices
- Credit Scoring: Banks could use predictive analytics to calculate makeshift “credit scores” for people that don’t have a credit history based on behavioral traits such as social media posts and spending habits. They could use these scores to determine whether or not to lend to someone
- Trading Insight: leveraging big data to inform which stocks to buy, trade, or sell
The first capability of predictive analytics we cover in this article is the ability to understand customer behavior and detect patterns within it.
Customer Analytics
We’ve previously written about predictive analytics software for marketing, sales, and customer behavior analytics within the context of either a single financial institution or a single institution-vendor relationship. Customer behavior data points may include spending habits, geolocation, and recurring payments such as gym memberships or online services.
In contrast, we speak more generally about how that software could benefit the general banking enterprise in this section.
In order to have a fully-functioning predictive analytics application for discerning and analyzing customer behavior, a bank must use their customer data to train a machine learning model. Customer data can come from various sources and include various types of information, including:
- Spending patterns, usually over the course of weeks or months.
- Channel usage, or how the customer is accessing their banking information, such as on mobile, desktop, or at an ATM
- Bank interactions such as emails with bank representatives or documented in-person visits
- Customer shopping behavior
- Services the customer is already using or receiving
- Customer credit score and credit history
- Customer profitability, including their likelihood to request loans, which might be discovered using another machine learning model.
- Social media data, such as “liking” multiple products on Facebook and posting about wanting or needing different products.
Usually, banks looking to adopt this type of software have large stores of big data of most of these types. Enterprise banks often have vast quantities of data that they aren’t always sure how to use even if they want to, and it can be challenging for them to garner insight from this data. Predictive analytics could help with this in some situations.
Much of a customer’s spending history, credit history, bank interactions such as transferring money from one account to another, and customer lifetime value will already be labeled.
More unstructured data types, such as social media data, will need to be labeled or formatted in some other way before predictive analytics software can recognize individual points within it.
An AI application that mines social media data would necessarily involve natural language processing (NLP). Banks could use NLP-based sentiment analysis software to determine a customer’s emotional response to a product in a social media post.
A bank could use this customer data to determine the best services and products to offer their customers via their mobile banking app or email promotions.
These could include new bank account deals for more family members, services such as overdraft protection, and special interest rates on loans.
Additionally, these services could be more easily integrated into the channels most often used by those customers, and thus improve the user experience.
Below is a short demonstrative video from IBM Analytics that details how AI-based analytics software could benefit banks. While the video does not explain exactly how the software works, it provides a clear explanation of the value the software may offer banks:
White-Collar Automation: Accounts Receivable
A press release from Cash and Treasury Management File details Citi Bank’s success with an AI software solution built by AI vendor HighRadius. The vendor specializes in cloud-based payment receivables, which help organize and keep track of accounts receivable with an application in the cloud. They claim to have used HighRadius’ predictive analytics technology to improve their Smart Match platform for invoice and payment matching for corporate clients.
The press release also states that Citibank’s corporate clients were seeking innovations in the following business areas:
- Reducing costs through automation of manual processes
- Decreasing the daily number of outstanding accounts receivable
- Managing exceptions quickly, and thus increasing the efficiency of payment processing operations.
HighRadius’ platform uses predictive analytics to match open invoices with received payments from corporate clients.
According to the press release, Citi Bank was able to help their corporate clients improve their reconciliation rates and straight-through processing (STP), or automated payment processing system. This would indicate that Citibank’s STP system could more accurately match payments to the correct deficit and thus reconcile the debt.
In the coming years, this and other types of AI-based automation may come to replace many roles in banking and finance. This might include marketers and financial advisors whose job it is to find these trends and capitalize on them.
We spoke to Alexander Fleiss, CEO, Chairman, and co-founder of Rebellion Research about how AI is “eating” finance, or replacing the jobs of more and more employees in banks and financial institutions.
That said, while AI could prove disruptive in finance, readers should be aware that Rebellion Research is also likely trying to drum up hype about automation in order to sell their products. We discuss this notion further in our article – Will Robots Take Your Job? Don’t Trust Startups and Enterprises to Tell You.
Rebellion Research develops AI applications for quantitative analysis used to decide on investments. When asked about which roles he thought were most likely to be automated, Fleiss said:
I think we’ll see a lot of brokers losing their jobs, a lot of financial advisors, bankers are going to get hit. In terms of the number of jobs, it’s going to be the retail banks that will fire the most people. They’re going to have fewer people at the window, fewer people in the back office. The big city banks are going to fire tens of thousands of people in operations and accounting; a lot of paper pushers.
Predictive Analytics for Credit Scoring
Predictive analytics can also be used in credit scoring applications for client banks and enterprise creditors to more accurately estimate the risk associated with a potential customer. Most credit scoring methods consider the potential customer’s credit and financial history, but this may still leave some people without credit even if they are able to pay their loan payments on time.
In order to determine a credit score, the software runs all available information about the given customer through its algorithm. It then calculates how big of a risk the bank would take if they chose to underwrite that customer.
This application may allow banks or creditors to base their credit scoring on alternative data types such as social media posts and interactivity. This could include what sites a potential customer visits, what they purchase via eCommerce, and what they say about those sites and purchases on social media. The online behavior of a potential customer can indicate the likelihood that they will pay back their loans and make payments on time.
It is important to note that in order to extract data from social media posts, such as whether a person felt positively or negatively about a purchase, NLP technology would be necessary. This is because NLP is the only AI technology be able to estimate the sentiment of a social media post. The sentiment becomes a data point indicating a “positive” or “negative” experience, which can then be recognized by a predictive analytics application.
This has the potential to allow banks to accurately score individuals who normally would not have access to credit. Those without credit histories would be able to leverage their social media activity and eCommerce internet history to show their fiscal responsibility and thus get lent to by a bank.
SAS is a large tech firm that offers a predictive analytics application they call Credit Scoring for SAS Enterprise Miner, which they claim has helped Piraeus Bank Group. The case study detailing their partnership states that SAS helped the bank speed up their data analysis and report generation processes. With regards to data analysis, Piraeus Bank Group used the software to optimize the development of their risk prediction models.
This means that the bank group found the best possible way for their enterprise to project their predictions into the future, and this likely includes being able to cleanly move between variables to test. The case study also states that Piraeus Bank Group was able to improve data analysis speed by 30%.
Prescriptive Analytics for Trading Intelligence
The difference between predictive and prescriptive analytics is mainly that prescriptive analytics takes the technology a step farther to recommend the next best course of action. Once the software finds all viable next steps for the user, it recommends one with the highest likelihood of success. Often, predictive analytics will simply allow the user to more cleanly plug different variables into situations they need to have information on before they can make a decision.
Predictive analytics software correlates the goal of the data science experiment with data points that have lead to similar results to that goal in the past.
For example, if a data scientist wanted to test the best way to improve ROI on changes to their customer smartphone app, the system would correlate popular app updates with ROI. The data scientist would then be able to see which updates to the mobile banking app elicited the most customer satisfaction.
Banks could use trading insight found using prescriptive analytics to help their clients who buy and sell stocks make more informed decisions.
For banking customers, this information could be channeled into a mobile banking app and delivered through a section about stocks and trading. Alternatively, they could use this intelligence internally to have a more detailed image of the banking stock market and further understand what is leading people to buy stock in their company.
We spoke to Ann Miura-ko, co-founder and partner at Floodgate, about how prescriptive analytics software could benefit financial institutions by being “self-driving.” In this case, she refers to the software always determining the next probability as new data enters its purview. When asked if prescriptive analytics software could be used to recommend business operations to various departments throughout every process, Miura-ko said:
My belief is that the data actually already exists out there in terms of how all of this information ought to be tied together, so when I talk about probabilistic inputs, it’s not just around things we’re never certain about…there’s also things about the future that we should be able to predict and we should know that there’s some sort of newsworthy event that then is going to have trickle-down effects upon my business.
It is clear from this quote that the possibilities of prescriptive analytics within the enterprise may be vast. It is important to recognize the amount of automation already possible with prescriptive analytics, as companies may continue to innovate on it for the banking space.
Our research did not yield any results showing a bank’s success with a vendor’s software for trading intelligence. Because of this we can infer that the landscape of applications for trading and stock intelligence may be relatively nascent compared to other banking solutions.
This could be indicative of major banks prioritizing innovation outside of this type of intelligence. Other, possibly more important areas for innovation include loan and credit intelligence, fraud detection, and prevention.
Header Image Credit: Admiral Markets